Abstract
Many mountain pastures consist of a mosaic of grassland and shrub communities. Ongoing changes in mountain agriculture have affected the balance between the two elements of the mosaic. In order to understand the consequences of these changes for ecosystem functioning, we studied patterns in vegetation, root structure and soil properties along transects of varying grassland-to-shrub proportions. Our hypothesis was that differences in the vegetation aboveground are accompanied by differences belowground, related to soil properties and depth. The research was conducted at a subalpine site in the Trentino region (South-eastern Alps), consisting of Nardus stricta grasslands alternating with shrub patches of Rhododendron ferrugineum. Our investigation showed that the composition of vegetation was mainly governed by R. ferrugineum cover and less by soil properties. Plant species richness peaked at low to intermediate degrees of shrub cover and composition between transects became more similar with increasing shrub cover. Where R. ferrugineum cover was higher, Hemicryptophytes caespitosae were replaced by Nano-phanerophytes with consequences for belowground structures. At increasing shrub cover, root length density decreased, especially in the top soil, while root weight density remained stable and C content increased insignificantly. We discuss that theses structural changes along the gradient of R. ferrugineum cover affect a number of ecosystem services. The presented evidence suggests that maintaining grasslands with a low cover of R. ferrugineum balances a number of services, namely plant species diversity, carbon stabilization in soil and the prevention of soil erosion.
Introduction
Subalpine pastures are created and maintained by extensive ruminant husbandry and are frequently composed of a mosaic of shrubland and grassland (Mucina et al. Citation1993; Tasser & Tappeiner Citation2002; Koch et al. Citation2015). The reduction of traditional management or the complete abandonment of pastoralism in areas below the treeline results in the expansion of woody plants, especially in inaccessible and steep areas (Tasser & Tappeiner Citation2002; Galvánek & Janák Citation2008). In many cases, such homogeneous shrub or forest vegetation is much less biodiverse than grassland vegetation or mosaics of both (MacDonald et al. Citation2000; Pornaro et al. Citation2013; Koch et al. Citation2015).
Mountain grassland provide a number of services such as the conservation of unique biodiversity, the regulation of physical and chemical fluxes in ecosystems, the mitigation of pollution and the preservation of landscapes (e.g. Gibon Citation2005; Lemaire et al. Citation2005). Shrubland and grassland differ in ecosystem functioning and, hence, shifts in the mosaic structure may affect the provision of ecosystem services. Several of these services are modulated by belowground components of the ecosystem, for example, the retention of water and nutrients in the soil, the sequestration of carbon (C) and soil stability (Guidi et al. Citation2014). However, vegetation studies are usually restricted to aboveground components. Van der Maarel and Franklin (Citation2012) suggested that “it would be logical, although technically complicated, to include belowground components in the definition and description of plant communities” since belowground components are part of the community. Structural analyses of aboveground plant parts should therefore be complemented with an analysis of the belowground parts.
Above and belowground vegetation structure has been jointly studied in cropland with regards to soil water infiltration and soil structural stability (Mamo & Bubenzer Citation2001; Joseph et al. Citation2003), which regulate soil erosion (Klima Citation2007). In grassland, Guidi et al. (Citation2014) emphasized the importance of the stable storage of carbon. Compared to forests, they found that managed and abandoned grasslands store substantially more C in stable mineral soil aggregates and as much C in total. In addition, the depth and the density of plant roots are important soil stability factors and are sensitive parameters in most hydrological models (Beven & Kirby Citation1979; Tasser et al. Citation2003). This shows that above and belowground vegetation structure is crucial in the global C and water cycles. However, only a few studies reported the effects of semi-natural vegetation on soil properties (e.g. De Baets et al. Citation2006; Montagnoli et al. Citation2010; Głąb & Kacorzyk Citation2011) or how changes in root density and morphology in gradients from grassland to shrubland affect soil functioning (Tasser et al. Citation2003).
One of the most widespread semi-natural habitats in temperate mountain regions are species-rich Nardus stricta L. grasslands codified as H 6230 in the Natura 2000 list (Galvánek & Janák Citation2008). They are extensively managed and occur in large parts of Western and Central Europe (Krahulec Citation1985; Stieperaere Citation1990; Peppler Citation1992). In the Italian Alps, this habitat is located in alpine and subalpine zones on a wide range of different soil and climate conditions, which result in a wide number of sub-types (Lüth et al. Citation2011; Pignatti et al. Citation2013; Gennai et al. Citation2014). Especially, in the upper subalpine zone, Nardus grasslands are frequently interspersed by Rhododendron ferrugineum L. dwarf shrub communities, forming a mosaic of both communities. The portion and distribution of grassland and shrub patches primarily depend on management factors such as the intensity of grazing by domestic animals. Grazing intensity has been reduced in many areas of the Southern Alps and caused the expansion of dwarf shrub communities (Pornaro et al. Citation2013). As reported by the Trento Regional Rural Development Programme (2014–2020), 67% of the regional territory were covered by forest in 2005 (407.531 ha), and the area of forest expanded by 18% on former open grassland in marginal areas in the past 50 years. Such a strong change of land cover may have large-scale consequences for the provision of ecosystem services.
We, therefore, studied how plant species composition, as well as soil properties, root density and root size distribution vary along grass–shrub gradients (open N. stricta grassland to R. ferrugineum shrubs) in the South-eastern Alps. We hypothesized that along these grass–shrub gradients a concurrent change of both vegetation and belowground patterns occurs. In particular, as root distribution of herbaceous and shrubby plants differs, we also expected that patterns vary significantly with soil depth. To the best of our knowledge, this is one of the first detailed assessments of root characteristics in species-rich Nardus grassland. The objective of our research was to assess aboveground species composition and belowground soil and root characteristics and consequently derive recommendations for urgently needed cost-effective management strategies in this fragile ecosystem.
Methods
Description of study site
The research was conducted in the Trentino region, a mountain area in the Central and Eastern Italian Alps (Blasi et al. Citation2014). The study site was located on the Trenca summer farm (latitude 46°08′N; longitude 11°39′E; elevation 1700 m asl.) in the south-eastern part of the Lagorai massif. The climate of the site is temperate oceanic with an annual mean temperature of 4.4°C (derived from the high-resolution Alpine temperature interpolation by Hiebl et al. Citation2009) and rainfall of 917 mm year−1 (data derived from the Meteotrentino data weather station nearest to the site). Monthly precipitation exceeds 80 mm between May and October. According to the world reference base classification, the soil at the site is an Umbric Leptosol.
In 2009, when the field work was carried out, the pasture site was grazed by 85 dairy cows, 50 goats and 70 sheep. The stocking rate was adjusted by the owner to achieve optimum pasture productivity (around 0.6 livestock units ha−1). However, in the twentieth century, the number of grazing animals was higher and continuously reduced from 2000 to 2009. Cows were kept on the pasture during the entire grazing season (from June to September). They were milked twice per day and were taken to areas further away from the stead after milking. The study site was located in the flatter portion of the whole grazing area and consisted of heterogeneous vegetation (Figure (a)): it can be described as a gradient of vegetation between typical Nardus grassland on one side and areas completely covered by shrubs (R. ferrugineum) on the other.
Figure 1. The six transect lines (along which vegetation and soil samples were collected), plotted over an ortophoto of 2006 with contour lines at 10 m interval (a), and the distribution of shrub patches in 2000 (b) and 2006 (c) in the immediate surroundings of the transects (indicated by the blue rectangle in panel (a)). The inlay in panel (a) shows the location of the study site at Trenca farm in Italy.
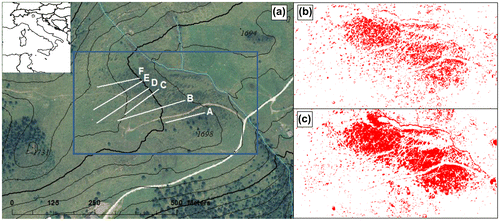
Air photos of the area captured in 1954, 1973, 1998 and ortophotos of 2000 and 2006 were analysed in order to distinguish shrub patches on grassland using different pixel colours. The distortion of air photos was corrected by overlapping them to ortophotos in ArcMAP 10.1 (ESRI, Redlands, CA). The poor resolution of air photos and their range of grey colouration impeded the analysis. Therefore, only the two ortophotos were analysed quantitatively using Sigmascan Pro v. 5.0 (SPSS Inc., Chicago, IL), for estimating the percentage of image pixels referable to shrubs and trees ground cover through analysis of image spectrum. The Tinitaly 10-m elevation data (Tarquini et al. Citation2007) was used to relate the percentage of shrub cover to terrain slope and topographic position index that is the difference between the elevation of a focal cell and the average elevation of its eight surrounding neighbours.
Vegetation survey, root sampling and analysis
Six linear transects across the grassland–shrub mosaic were established (Figure (a)). Five plots each measuring 1 m by 1 m were located along each transect resulting in a total of 30 plots. Plots were chosen along each transect in order to be as close as possible to 0, 25, 50, 75 and 100% of shrub cover. Plant species composition was assessed in each plot in July 2009. Presence/absence of species in each plot was recorded, using the taxonomy of Pignatti (Citation1982). The total number of species was calculated for each plot. In addition, the life form of all vascular plant species was reported according to Raunkiaer (Citation1934) and Pignatti (Citation1982). The similarity of vegetation in each plot i and the five other plots j in the same class of shrub cover was expressed as follows:
where dij is the Bray and Curtis (Citation1957) distance calculated as follows:
where Ni and Nj are the number of species on plots i and j, respectively, Nij is the number of species shared between plots i and j. The similarity is 0 when no species are shared and 1 when species composition is identical.
Soil samples from depths 0–5 cm and 5–20 cm were collected and analysed following Italian standard soil analysis guidelines (G.U. Citation1999) for total nitrogen (N) content (Kjeldahl total nitrogen [g kg−1]), content of organic C (Springer–Klee method [g kg−1]) and pH (1:5 soil–water solution). Additionally, one soil core (47 mm in diameter and 20 cm in length) was taken from each plot using a split-tube sampler (Eijkelkamp Agrisearch Equipment, Giesbeek, the Netherlands) to determine main root parameters for different soil depths. Cores were divided into three sections: 0–5 cm; 5–10 cm; and 10–20 cm depths, and then frozen. Before washing, a solution of 2% oxalic acid was used to disperse soil particles (Heringa et al. Citation1980). Roots were then separated from soil and organic debris using sieves, and samples were cleaned by hand to remove the remaining soil and organic impurities. After cleaning, samples were temporarily stored in a 12% ethanol solution. Total length and average diameter of root samples were measured by means of winRHIZO™ version 2003b (Regent Instruments Inc., Quebec). Results were grouped into diameter classes ranging from 0 to 2 mm (fine roots) in increments of 0.2 mm, and roots over 2 mm were grouped together. Roots were then dried at 105°C for 36 h to determine biomass dry weight. Root weight density and root length density of each core section were calculated based on soil volume. The total root weight density and the total root length density of each core were calculated using the densities found at each of the three soil sections, while average diameter were calculated as the ratio between the scanned projection area and the length of roots.
Statistical analysis
In order to investigate how vegetation composition changed along transects, a constrained correspondence analysis (CCA) was carried out with inclusion of shrub cover and topsoil properties (0–5 cm depth) as explanatory environmental variables using package vegan in R 3.1.3 (R Development Core Team). Differences in the ordination analyses using properties of topsoil or deeper soil (5–20 cm) were negligible.
In order to investigate the effects of shrub cover on vegetation, root and soil properties, generalized linear-mixed models were fitted to the data. A Poisson distribution with a log link function was used for species richness, and a binomial likelihood with a logit link for similarity index and proportions of life forms. Overdispersion was not present. Root weight density, root length density and average diameter as well as soil pH, N and C content were always positive but sometimes close to 0. We, therefore, used a Gamma likelihood with a log link. Since data exploration often suggested hump-shaped relationships, R. ferrugineum cover and its square were included as fixed effect. Soil proprieties were also added to the model as fixed effects but they didn’t improve the models as assessed by Akaike’s information criterion (AIC), which penalizes goodness of fit with the number of parameters. The transects were included as random intercepts and slopes, but did not improve the AIC. Hence, the final models were estimated using computationally simpler generalized linear models (GLM) in R 3.1.3 (R Development Core Team).
The dependence of shrub cover on terrain slope and topographic position was estimated in a GLM with a binomial likelihood and a spatially structured random effect to account for spatial autocorrelation using integrated nested Laplace approximation (Rue et al. Citation2009; Homburger et al. Citation2015).
Proportional data on life forms were analysed using multinomial log-linear models with the “nnet” package in R (Venables & Ripley Citation2002). Again, cover of R. ferrugineum and its square were used as explanatory variables and significances were calculated based on likelihood ratio tests.
Results
Aboveground patterns in grassland–shrub mosaic
Ortophotos revealed that from 1973 onwards the study area was characterized by the presence of shrub patches on the grassland. However, the shrubland mosaic evolved first slowly until 2000 (Figure (b)) and then increased from 11% of ground cover in 2000 to 16% in 2006 (Figure (c)). Shrub cover was significantly increased by slope (95% of credibility interval is excluding zero) but not by topographic position.
Vegetation at the site was a mosaic of N. stricta grassland intermingled with shrubland patches dominated by R. ferrugineum accompanied by Vaccinium species. N. stricta was by far the most frequent grass species and was found in all sampling plots with 0, 25, 50 and 75% of R. ferrugineum cover, but not in those with 100% cover (Table ). The grass species Phleum alpinum L. was recorded in few plots, all with 0 and 25% of shrub cover. The sedge Carex sempervirens Vill. was found in all plots with cover below 50%, in some plots with 50 and 75% of shrub cover and was absent where shrub cover was above 75%. Lathyrus montanus Bernh., Festuca ovina group, Luzula campestris (L.) DC. and Vaccinium vitis idaea L. were often present in plots with 25, 50 and 75% of shrub cover, but were not recorded at 100%. Species found in plots completely covered by R. ferrugineum, namely Homogyne alpina (L.) Cass., Maianthemum bifolium (L.) Schmidt, Soldanella alpina L. and Vaccinium myrtillus L., were not exclusive of plots with 100% of shrub cover, but they were also recorded in plots with lower cover values.
Table I. Species composition in a subalpine grassland–shrub mosaic (Trenca farm, South-eastern Alps).
The correspondence analysis constrained by R. ferrugineum cover and soil properties displayed differences in the influences of soil properties and shrub cover on plant species composition (Figure ). Variation in vegetation was largely due to different percentages of R. ferrugineum and much less by soil properties, with the exception of pH in one of the transects. The spread of ordination scores between transects decreased with increasing values of shrub cover, indicating a homogenization on the composition. The large spread of plots at 0% shrub cover was mainly associated with soil pH.
Figure 2. Canonical Correspondence Analysis of 1-m2 vegetation samples constrained by Rhododendron ferrugineum cover and soil properties (pH, nitrogen content [N] and carbon content [C]) of the upper (0–5 cm) soil layers. Full dots are plots with 0% of R. ferrugineum cover; empty dots are plots with cover between 25 and 100%. Dots of each transect are joined by a line along the cover gradient. Arrows indicate correlation vectors of soil properties used in the analysis. Abbreviation of species names: Ao: Anthoxanthum odoratum; Am: Arnica montana; Lc: Lotus corniculatus; Pa: Phleum alpinum; R: Rhododendron ferrugineum; Tr: Trifolium repens; Vm: Vaccinium myrtillus; Vv: Vaccinium vitis-idaea.
![Figure 2. Canonical Correspondence Analysis of 1-m2 vegetation samples constrained by Rhododendron ferrugineum cover and soil properties (pH, nitrogen content [N] and carbon content [C]) of the upper (0–5 cm) soil layers. Full dots are plots with 0% of R. ferrugineum cover; empty dots are plots with cover between 25 and 100%. Dots of each transect are joined by a line along the cover gradient. Arrows indicate correlation vectors of soil properties used in the analysis. Abbreviation of species names: Ao: Anthoxanthum odoratum; Am: Arnica montana; Lc: Lotus corniculatus; Pa: Phleum alpinum; R: Rhododendron ferrugineum; Tr: Trifolium repens; Vm: Vaccinium myrtillus; Vv: Vaccinium vitis-idaea.](/cms/asset/d225e5f9-4650-42ba-8ebe-70b3c28c9e4c/tplb_a_1187679_f0002_b.gif)
A total of 41 plant species was found in the entire data-set. The cover of R. ferrugineum had a significant hump-shaped effect on species richness ( < 0.001 for both, single and square term; Figure (a)). The average number of species per 1 m2 sample was 13 and 14 at 0 and 25% shrub cover, respectively, decreasing to 5 species at 100% shrub cover. The calculated index of similarity was also significantly affected by cover and its square (
< 0.001 for the single and
< 0.01 for the square term): values were the lowest for plots without R. ferrugineum and increased with shrub cover (Figure (b)). The increase of similarity index occurred primarily at intermediate shrub cover between 0 and 50%.
Figure 3. Effects of Rhododendron ferrugineum cover on the number of species (a), the similarity index of species composition (b), percentage of Hemicryptophytes caespitosae (c) and of Nano-phanerophytes in relation to the total number of species (d). Black lines represent predictions of generalized linear models for all plots.
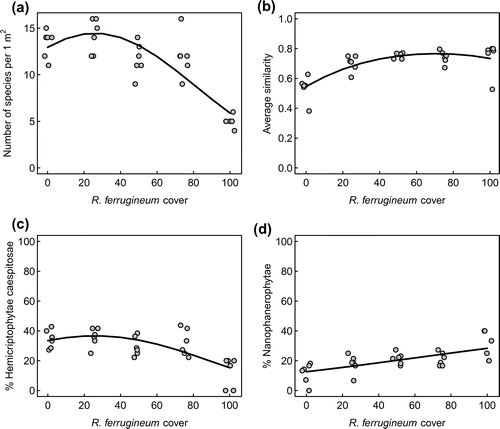
The main Raunkiær life forms in the grass–shrub mosaic were Hemicryptophytes rizomatosae, Hemicryptophytes caespitosae and Nano-phanerophytes, accounting for 32, 29 and 20% of all species on average, respectively. While the percentage of Hemicryptophytes rizomatosae was not affected by cover, the share of Hemicryptophytes caespitosae in the species assemblage showed a hump-shaped response to shrub cover ( < 0.001 for the single and
< 0.05 for the squared term; Figure (c)), decreasing from 36% at a cover of 25% to a share of 17% at 100% cover. Nano-phanerophytes displayed a significant positive relationship with cover (
< 0.001; Figure (d)), increasing from 14 to 33% as shrub cover increased from 0 to 100%. The multinomial log-linear model indicated that the probability to fall into a life form was significantly affected by cover (
< 0.05), but not by its square. Other life forms recorded were Geophytes rizomatosae (13% in average), Geophytes bulbosae (2% of all species in average) and Chamaephytes rizomatosae (4% in average).
Belowground patterns in grassland–shrub mosaic
Soil pH values ranged from 3.2 to 4.3 in the top soil layer, and from 3.4 to 4.9 in the lower, 5–20 cm (Table ). The majority of the samples collected in the top layer had an N content of more than 10 g kg−1, with a minimum of 3.9 and a maximum of 16.6 g kg−1, while in the deeper layer N content ranged from 1.3 to 7.4 g kg−1. Both, pH and N content were not related to R. ferrugineum cover. In contrast, C content of the upper and deeper layer displayed a significant positive relationship with shrub cover ( < 0.05 and
< 0.01 for the upper and lower layer, respectively). It is interesting to note the variability between transects of top soil C content increased with shrub cover (Figure (a)).
Table II. Average soil pH and N content at two depths of a mountain pasture in the South-eastern Alps at different percentage of Rhododendron ferrugineum cover.
Figure 4. Effects of Rhododendron ferrugineum cover on soil carbon content (a), root weight density (b), root length density (c) and root average diameter (d) at different soil depth layers. In figure a, full symbols and black line represent samples collected at 0–5 cm of soil depth; empty symbols and gray lines represent samples collected at 5–20 cm of soil depth soil depths. In figures b, c and d, dots and solid lines represent the upper (0–5 cm) soil layer, square symbols and dashed lines represent the middle (5–10 cm) soil layer and rhombi and dotted lines represent the lower (10–20 cm) soil layer. Lines represent predictions of generalized linear models for all plots.
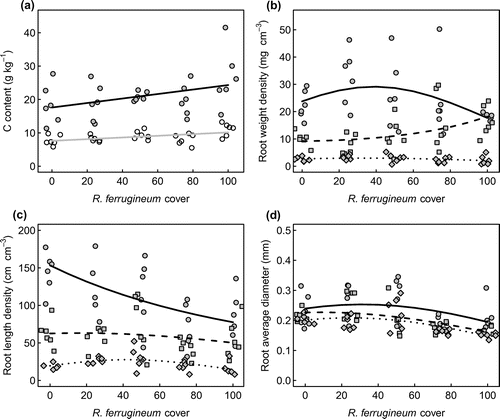
Total root weight density across the entire investigated soil column (0–20 cm) showed no significant relationship with shrub cover. This was due to contrasting responses of root weight density in the three depths (Figure (b)). In the top layer, which contained the major root mass, a hump-shaped but statistically non-significant relationship was found which contrasted with a significant increase ( < 0.05) in the middle layer (5–10 cm depth) and no change in the deepest layer. In contrast, total root length density significantly decreases with increasing shrub cover (
< 0.01) due to a strong and significant decrease with cover (
< 0.01) in the quantitatively important top layer (0–5 cm depth) and a significant hump-shaped effect of squared cover (
< 0.05) in the quantitatively marginal deepest 10–20 cm layer (Figure (c)). For both root metrics, including soil properties did not improve models.
Figure (b) and (c) also show important changes in the depth distribution of roots along the shrub cover gradient. While the major root mass and root length was always located in the top 10 cm of the soil, the strong quantitative dominance of the top layer attenuated with shrub cover.
The analysis of average root diameter revealed a significant hump-shaped effect of shrub cover ( < 0.001 for the single and
< 0.05 for the square term) but minor effects of soil depth. Due to variability in the data, a trend could not be documented in the upper layer, whereas the effect of shrub cover in the middle layer (
< 0.001) and the effects of shrub cover (
< 0.001) and its square in the deeper layer (
< 0.05) were significant.
Interesting patterns were found if information on root length and diameter were combined and root length density was analysed separately for diameter classes (Figure ). In all cores, more than 85% of total root length could be attributed to fine roots with diameter of less than 2 mm in diameter. Length density of fine roots (<2 mm) showed a negative relationship with cover in the top soil layer ( < 0.01) and a significant hump-shaped effect of squared cover (
< 0.05) in the lower layer (Figure (a)). It is also interesting to note that the density of coarse roots (>2 mm) was positively related to shrub cover (
< 0.05) only in the middle layer (Figure (b)).
Figure 5. Depth distribution of the length density of roots with diameter less than 2 mm (a) and diameter higher than 2 mm (b) as affected by Rhododendron ferrugineum cover. Note that the scales of the response axes of the two panels differ by a factor 100. Symbols are observed values, lines show predictions of generalized linear models. Dots and solid lines represent the upper (0–5 cm) soil layer, square symbols and dashed lines represent the middle (5–10 cm) soil layer and rhombi and dotted lines represent the lower (10–20 cm) soil layer.
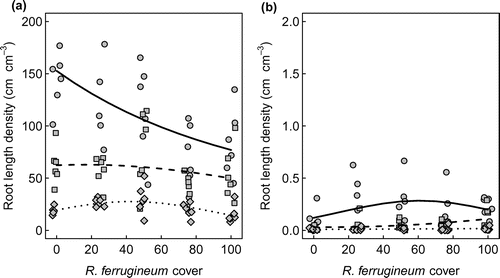
Discussion
Our study revealed distinct effects of shrub cover on above and belowground patterns in a mosaic of N. stricta pasture and R. ferrugineum shrubs. We found that a high cover of shrubs was detrimental to species richness as well as to the heterogeneity of species composition and reduced the density of fine roots in the top soil layer. These differences have important implications for ecosystem functioning and associated services.
Shrub cover reduces species richness and heterogeneity
A change in plant species composition was found along the gradient of R. ferrugineum cover, together with a hump-shaped response of species richness to shrub cover. These findings are in line with earlier investigations of plant biodiversity along shrub gradients (e.g. Anthelme et al. Citation2001; Kesting Citation2009; Pornaro et al. Citation2013; Koch et al. Citation2015). Results of the vegetation analysis agreed well with releves of 100 m2 plots randomly distributed across the study site at a continuous range of R. ferrugineum cover (Pornaro Citation2012). It is interesting to note that 50 out of a total of 98 species recorded by Pornaro (Citation2012) did not reach 1% of abundance. Even though these low-abundance species were rarely detected in 1 m2 samples, the shape of the response of species richness to shrub cover was similar.
Besides the fewer plant species in samples with high shrub cover, we detected a noteworthy homogenization of the species composition at higher degrees of shrub cover as indicated by the Bray–Curtis distance and the CCA. Looking at species data in more detail reveals that the homogenization was due to the fact that few of the species recorded at intermediate percentage of R. ferrugineum cover were able to grow at higher percentages of cover. The specialist species coping with high competition by R. ferrugineum are obviously few in number and the same in all six gradients, in line with recent findings (Parolo et al. Citation2011; Koch et al. Citation2015). Our results further showed that when R. ferrugineum cover was high, Hemicryptophytes caespitosae were replaced by Nano-phanerophytes with consequences on canopy and belowground structure. The Nano-phanerophytes, in fact, are long known to have a deeper root system than the Hemicryptophytae or Chamaephytes (Dierschke Citation1994).
Our study revealed that changes in plant species composition were not related to soil properties, but to other factors, primarily topography and grazing management. As recently demonstrated by Homburger et al. (Citation2015), stocking rate alone is not sufficient to understand local grazing pressure. Environmental conditions, mainly terrain slope and vegetation influence grazing activities and cause under- and over-grazed areas. This likely explains the significant effect of slope on the distribution of shrubs at the study site. Moreover, Julitta et al. (Citation2014) demonstrated the important role of microtopography of subalpine grassland on plant species phenology and distribution by affecting snowmelt, nutrient and water availability.
Cover of R. ferrugineum is related to the distribution of fine roots in the soil
Results of CCA demonstrated that the composition of vegetation along shrub cover gradients was not related to soil properties, neither of the upper nor of the deeper soil. The only soil property correlated with R. ferrugineum cover was C content. Other studies also reported higher contents of organic C in forest than in grasslands (Guo et al. Citation2007; Guidi et al. Citation2014). As a consequence of reduced soil temperature and moisture following forest succession (Hiltbrunner et al. Citation2013), the decomposition of organic matter is slowed down, leading to a decreased incorporation of C into soil aggregates (Gregorich et al. Citation2006). As discussed by Guidi et al. (Citation2014), such an increase of C content in the soil is a typical positive effect of transitional phases between grasslands and forest. However, Guidi et al. (Citation2014) found that less organic C is allocated to stable soil aggregates in forests than in grasslands. This evidence agrees well with our finding that the mass and length of fine roots (<2 mm) decrease as shrubs progress into grassland. Fine roots of grasses have high turn-over rates (Schneider et al. Citation2006) and a positive effect on aggregate stabilization (Jastrow et al. Citation1998). Their lower length, weight density and turnover in forests compared with grasslands can therefore reduce aggregate stability as found by Guidi et al. (Citation2014).
Above and belowground pattern have consequences for ecosystem functioning
Fine roots forming a very dense root system characterize grasses (De Baets et al. Citation2006), in contrast to the root system of trees and shrubs, which consist of fewer thick roots. It is reasonable to attribute the negative effect of shrub cover on root length density in the top soil layer to a decrease of herbaceous components in the vegetation. In the middle soil layer, however, root biomass increased with higher R. ferrugineum cover, whereas root length density did not change. Nano-phanerophytes roots likely influence the weight of root system but not its length. At high levels of shrub cover, roots will henceforth be coarser and deeper in the soil.
As demonstrated by Morgan (Citation2005), the effectiveness of plant cover in surface runoff and erosion depends on the height and continuity of the canopy, plant density and ground cover. The capability of grasses to reduce superficial erosion is well known (Gyssels & Poesen Citation2003; Morgan Citation2005). Succession by R. ferrugineum at our site replaced this herbaceous layer at our site by a shrub layer. It is doubtful whether the shrub cover layer will be as effective as grasses in controlling soil erosion. Furthermore, roots and especially the fine ones are important factors that account for the reinforcement of the soil against erosion (Canadell et al. Citation1996; Jackson et al. Citation1997; Van Beek et al. Citation2005). The reduction of root systems with increasing R. ferrugineum cover suggests that soil, especially the top 5 cm of the soil surface, is more susceptible to superficial erosion in shrubland than in Nardus grassland.
Conclusions
The vegetation of the analysed mosaic of R. ferrugineum shrubland and Nardus grassland changed along the shrub cover gradient towards a less species-rich and more similar composition at high shrub cover. In line with the observed patterns in canopy structure and life forms, alternations of roots characteristics were found. The effects of the cover of R. ferrugineum on patterns below ground was stronger than the effect explained by the quantified soil properties, which only showed an insignificant trend towards higher C contents at high shrub cover. Changes in belowground structures may alter ecosystem functioning. Differences in the distribution of fine roots corroborate evidence of higher C stabilization in soils of open grassland. In addition, the study suggests that surface soil erosion may be affected by a dense presence of R. ferrugineum cover in a subalpine pasture. Hence, we recommend maintaining grasslands with a low percentage of R. ferrugineum in order to balance a number of ecosystem services, namely plant species diversity, carbon stabilization in soil and the prevention of soil erosion.
Acknowledgements
Dr Rossana Sallenave’s assistance with the manuscript is gratefully acknowledged. We would also like to thank Malga Trenca Association and the Roncegno Terme municipality. We are grateful to Lorenzo Adami for his help in soil sampling and data collection and to Stefano Bilato for assistance in laboratory analyses. The authors are indebted to Professor Umberto Ziliotto for his suggestions and helpful comments on the project and to two anonymous reviewers for comments on the manuscript.
References
- Anthelme F, Grossi J, Brun J, Didier L. 2001. Consequences of green alder expansion on vegetation changes and arthropod communities removal in the northern French Alps. Forest Ecol Manag. 145: 57–65.10.1016/S0378-1127(00)00574-0
- Beven KJ, Kirkby MJ. 1979. A physically based variable contributing area model of basin hydrology. Hydrol Sci Bull 24(1): 43–69.10.1080/02626667909491834
- Blasi C, Capotorti G, Copiz R, Guida D, Mollo B, Smiraglia D, et al. 2014. Classification and mapping of the ecoregions of Italy. Plant Biosyst 148(6): 1255–1345.
- Bray J, Curtis J. 1957. An ordination of the upland forest communities of southern Wisconsin. Ecol Monogr 27: 325–349.10.2307/1942268
- Canadell J, Jackson RB, Ehleringer JR, Mooney HA, Sala OE, Schulze ED. 1996. Maximum rooting depth of vegetation types at the global scale. Oecologia 108(4): 583–595.10.1007/BF00329030
- De Baets S, Poesen J, Gyssels G, Knapen A. 2006. Effects of grass roots on the erodibility of topsoils during concentrated flow. Geomorphology 76: 54–67.10.1016/j.geomorph.2005.10.002
- Dierschke H. 1994. Pflanzensoziologie [Phytosociology]. Stuttgart: Ulmer.
- Galvánek D, Janák M. 2008. Management of Natura 2000 Habitats. 6230 Species-rich Nardus Grasslands. European Commission.
- Gennai M, Foggi B, Viciani D, Carbognani M, Tomaselli M. 2014. The Nardus-rich communities in the northern Apennines (N-Italy): A phytosociological, ecological and phytogeographical study. Phytocoenologia 44(1): 55–85.10.1127/0340-269X/2014/0044-0574
- Gibon A. 2005. Managing grassland for production, the environment and the landscape. Challenges at the farm and the landscape level. Livest Prod Sci 96: 11–31.10.1016/j.livprodsci.2005.05.009
- Głąb T, Kacorzyk P. 2011. Root distribution and herbage production under different management regimes of mountain grassland. Soil Till Res 113(2): 99–104.
- Gregorich E, Beare M, McKim U, Skjemstad J. 2006. Chemical and biological characteristics of physically uncomplexed organic matter. Soil Sci Soc Am J 70: 975–985.10.2136/sssaj2005.0116
- G.U. (Gazzetta Ufficiale dello Stato Italiano [Official Gazette of the Italian state]). 1999. Approval: Metodi ufficiali di analisi chimica del suolo [Approval: Official methods of soil chemical analysis]. D.M., 13th September 1999, suppl. G.U., 248, 21 October 1999.
- Guidi C, Magid J, Rodeghiero M, Gianelle D, Vesterdal L. 2014. Effects of forest expansion on mountain grassland: Changes within soil organic carbon fractions. Plant Soil 385(1–2): 373–387.10.1007/s11104-014-2315-2
- Guo L, Wang M, Gifford R. 2007. The change of soil carbon stocks and fine root dynamics after land use change from a native pasture to a pine plantation. Plant Soil 299: 251–262.10.1007/s11104-007-9381-7
- Gyssels G, Poesen J. 2003. The importance of plant root characteristics in controlling concentrated flow erosion rates. Earth Surf Proc Land. 28(4): 371–384.10.1002/(ISSN)1096-9837
- Heringa JW, Groenwold J, Schoonderbeek D. 1980. An improved method for the isolation and the quantitative measurement of crop roots. Neth J Agr Sci 28: 127–134.
- Hiebl J, Auer I, Bohm R, Schoner W, Maugeri M, Lentini G, et al. 2009. A high resolution 1961–1990 monthly temperature climatology for the greater Alpine region. Meteorol Z 18: 507–530.
- Hiltbrunner D, Zimmermann S, Hagedorn F. 2013. Afforestation with Norway spruce on a subalpine pasture alters carbon dynamics but only moderately affects soil carbon storage. Biogeochemistry 115: 251–266.10.1007/s10533-013-9832-6
- Homburger H, Lüscher A, Scherer-Lorenzen M, Schneider MK. 2015. Patterns of livestock activity on heterogeneous subalpine pastures reveal distinct responses to spatial autocorrelation, environment and management. Movement Ecol 3(35): 1–15.
- Jackson RB, Mooney HA, Schulze ED. 1997. A global budget for fine root biomass, surface area, and nutrient contents. Proc Natl Acad Sci USA 94: 7362–7366.10.1073/pnas.94.14.7362
- Jastrow JD, Miller RM, Lussenhop J. 1998. Contributions of interacting biological mechanisms to soil aggregate stabilization in restored prairie. Soil Biol Biochem 30: 905–916.10.1016/S0038-0717(97)00207-1
- Joseph L, Pikul J, Kristian JA. 2003. Water infiltration and storage affected by subsoiling and subsequent tillage. Soil Sci Soc Am J 67: 859–867.
- Julitta T, Cremonese E, Migliavacca M, Colombo R, Galvagno M, Siniscalco C, et al. 2014. Using digital camera images to analyse snowmelt and phenology of a subalpine grassland. Agr Forest Meteorol 198–199: 116–125.10.1016/j.agrformet.2014.08.007
- Kesting S. 2009. Shrub encroachment of temperate grasslands: Effects on plant biodiversity and herbage production. PhD thesis, University of Göttingen.
- Klima K. 2007. Soil-protecting capability of plants cultivated in mountain regions. Probl Manag Mountain Regions 54: 131–137.
- Koch B, Edwards PJ, Blanckenhorn WU, Walter T, Hofer G. 2015. Shrub encroachment affects the diversity of plants, butterflies, and grasshoppers on two Swiss subalpine pastures. Arct. Antarct. Alp. Res. 47: 345–357.10.1657/AAAR0013-093
- Krahulec E. 1985. The chorologic pattern of European Nardus-rich communities. Vegetatio 59: 119–123.10.1007/BF00055681
- Lemaire G, Wilkins R, Hodgson J. 2005. Challenges for grassland science: Managing research priorities. Agric Ecosyst Environ 108: 99–108.10.1016/j.agee.2005.01.003
- Lüth C, Tasser E, Niedrist G, Dalla Via J, Tappeiner U. 2011. Classification of the Sieversio montanae-Nardetum strictae in a cross-section of the Eastern Alps. Plant Ecol 212(1): 105–126.10.1007/s11258-010-9807-9
- MacDonald D, Crabtree JR, Wiesinger G, Dax T, Stamou N, Fleury P, et al. 2000. Agricultural abandonment in mountain areas of Europe: Environmental consequences and policy response. J Environ Manag 59: 47–69.10.1006/jema.1999.0335
- Mamo M, Bubenzer GD. 2001. Detachment rate, soil erodibility and soil strength as influenced by plant roots: Part 1. Laboratory study. Trans Am Soc Agric Eng 44: 1167–1174.
- Montagnoli A, Di Iorio A, Ceriani RM, Scippa GS, Chiatante D. 2010. Root seasonal pattern, spatial distribution, and C: N ratio of matgrass pasture (Nardus stricta L.) in the Lombardy Prealps. Plant Biosyst. 144(2): 463–470.10.1080/11263501003731979
- Morgan RPC. 2005. Soil erosion and conservation. 3rd ed. Oxford: Blackwell Science.
- Mucina L, Grabherr G, Ellmauer T. 1993. Die Pflanzengesellschaften Österreichs, Teil I [The plant communities of Austria, Part I. Jena: Gustav Fischer.
- Parolo G, Abeli T, Gusmeroli F, Rossi G. 2011. Large-scale heterogeneous cattle grazing affects plant diversity and forage value of Alpine species-rich Nardus pastures. Grass Forage Sci 66(4): 541–550.10.1111/gfs.2011.66.issue-4
- Peppler C. 1992. Die Borstgrasrasen (Nardetalia) Westdeutschlands [Matgrass pasture (Nardetalia) of Western Germany]. Diss Bot 193: 1–404.
- Pignatti S. 1982. Flora d’Italia. Bologna: Edagricole.
- Pignatti E, Pignatti S, Isenberg P. 2013. Plant life of the dolomites: Vegetation structure and ecology, Vol. 8. Sudtirol: Springer Science & Business Media.
- Pornaro C. 2012. Effects of wood establishment on plant biodiversity and herbage production of mountain pastures. PhD thesis, University of Padova.
- Pornaro C, Schneider MK, Macolino S. 2013. Plant species loss due to forest succession in Alpine pastures depends on site conditions and observation scale. Biol Conserv 161: 213–222.10.1016/j.biocon.2013.02.019
- Raunkiaer C. 1934. The life forms of plants and statistical plant geography. Oxford: Oxford University Press.
- Rue H, Martino S, Chopin N. 2009. Approximate Bayesian inference for latent Gaussian models by using integrated nested Laplace approximations. J Roy Stat Soc B 71: 319–392.10.1111/rssb.2009.71.issue-2
- Schneider MK, Luscher A, Frossard E, Nosberger J. 2006. An overlooked carbon source for grassland soils: Loss of structural carbon from stubble in response to elevated pCO2 and nitrogen supply. New Phytol 172: 117–126.10.1111/nph.2006.172.issue-1
- Stieperaere H. 1990. De heischrale graslanden van Atlantisch Europa [The Nardus grasslands of Atlantic Europe]. PhD Thesis, Ghent University.
- Tarquini S, Isola I, Favalli M, Mazzarini F, Bisson M, Pareschi MT, et al. 2007. TINITALY/01: A new triangular irregular network of Italy. Ann Geophys 50: 407–425.
- Tasser E, Mader M, Tappeiner U. 2003. Effects of land use in alpine grasslands on the probability of landslides. Basic Appl Ecol 4: 271–280.10.1078/1439-1791-00153
- Tasser E, Tappeiner U. 2002. Impact of land use changes on mountain vegetation. Appl Veg Sci 5: 173–184.10.1111/j.1654-109X.2002.tb00547.x
- van Beek LPH, Wint J, Cammeraat LH, Edwards JP. 2005. Observation and simulation of root reinforcement on abandoned Mediterranean slopes. Plant Soil 278: 55–74.10.1007/s11104-005-7247-4
- Van der Maarel E, Franklin J. 2012. Vegetation ecology. Oxford: Wiley.
- Venables WN, Ripley BD. 2002. Modern Applied Statistics with S. Berlin: Springer-Verlag.