ABSTRACT
With the rapid development of Internet and big data technology, it is common to apply Internet and big data in various fields. Education is also one of the important fields of Internet and big data application, which brings new power to promote China’s education reform and innovation. Nowadays, a large number of online learning systems have been developed and applied in the field of education in China. However, in the face of so many online learning resources, Chinese students often have cognitive loss or cognitive overload. Therefore, the application of adaptive learning model in online learning system provides key to solve these problems. Based on the characteristics of knowledge graph Association, this paper proposes a variable incremental adaptive learning model based on knowledge graph and applies it to online learning system. In this adaptive learning model, this paper studies the construction method and application of knowledge graph. On this basis, in the face of increasing data, this paper uses association rules algorithm to update the knowledge graph. Based on the shortcomings of the existing FP growth algorithm, which still needs to traverse the result set many times, this paper proposes an improved FP growth algorithm. Through the simulation analysis, it is found that the adaptive learning model, constructed by the knowledge graph and the improved FP growth algorithm, can achieve the accurate search of online learning system. And with the increase of students’ search times and time, the content of search will meet their learning needs more and more, complete the knowledge automatic recommendation of online learning system, and avoid the occurrence of network lost and cognitive overload.
Disclosure statement
No potential conflict of interest was reported by the author(s).
Additional information
Funding
Notes on contributors
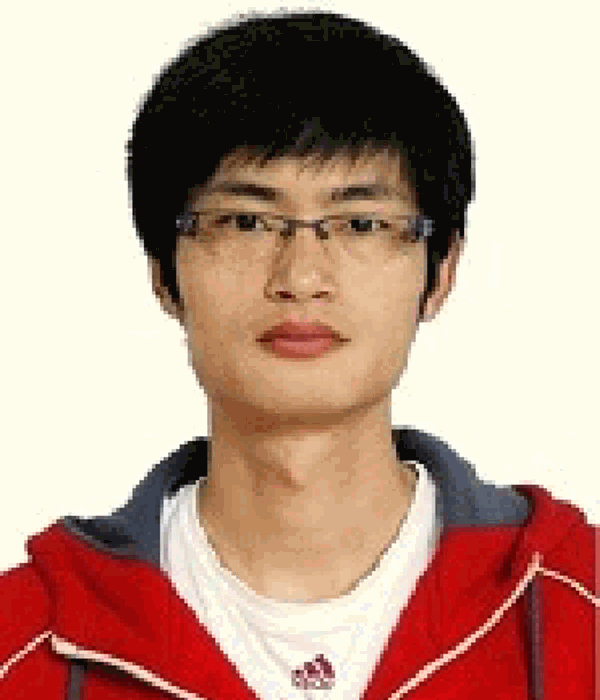
Zhongxian Bai
Zhongxian Bai was born in Jiaxing, Zhejiang, P.R. China, in 1985. He received the master’s degree from Zhejiang Normal University, P.R. China. Now, he works in Communication University of Zhejiang. His research interests include smart learning, Artificial intelligence and big data analysis.