Abstract
The emergence of online lending services such as peer-to-peer (P2P) lending has simplified transactions for lenders, eliminating the need for traditional bank intermediaries. However, accurately predicting potential lender defaults is a critical challenge in preventing financial distress, as lenders bear the burden of default risk. This challenge becomes even more complicate with P2P lending datasets that feature a multitude of complex attributes. To improve predictive accuracy, the study focuses on optimizing feature selection in this data-rich environment. Its main objective is to identify the most influential features for predicting default risk among P2P lenders through feature optimization techniques, leveraging the Genetic Algorithm (GA) in conjunction with ten different Machine Learning models. The study employed a hybrid approach of GA with three mutation rate levels, basic (MR=0), moderate (MR=0.5), and extreme (MR=1), to provide insights into model responsiveness and performance under various mutation scenarios. Notably, the results highlight GA+XGBoost as the best-performing model with a stable fitness score of 86.132% compared to others. This research holds significant potential for improving lender risk management in the P2P lending landscape by effectively identifying higher risk lenders. Ultimately, the findings contribute positively to the mitigation of financial risks for lenders within the P2P lending ecosystem.
Disclosure statement
No potential conflict of interest was reported by the author(s).
Additional information
Notes on contributors
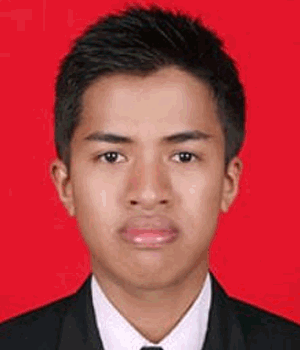
Muhammad Sam'an
Muhammad Sam'an received Bachelor Degree from Universitas Negeri Semarang and Master Degree from Universitas Diponegoro in Mathematics 2010 and 2016 respectively. His research interests are in optimization, fuzzy mathematics and computational mathematics. He can be contacted at email: [email protected].
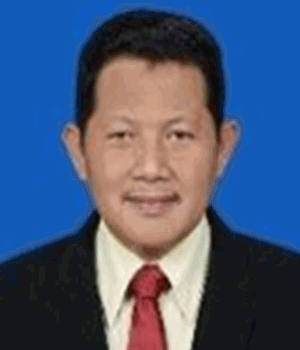
Muhammad Munsarif
Muhammad Munsarif received the Doctoral Degree in Computer science from Dian Nuswantoro University (UDINUS). Currently, he is a lecturer in informatics Engineering at Muhammadiyah University, Semarang (UNIMUS). His research interests include computer vision, data science and technopreneuership. He can be contacted at email: [email protected].
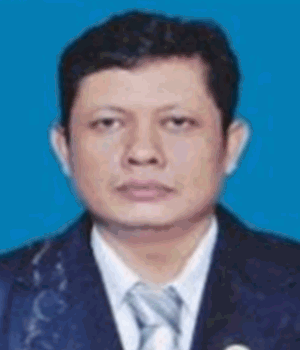
Safuan
Safuan received the Master Degree in Informatics Engineering from Dian Nuswantoro University (UDINUS) in 2015. Currently, he is a lecturer in informatics Engineering at Muhammadiyah University, Semarang (UNIMUS). His research interests include data mining, programming and web security. He can be contacted at email: [email protected].
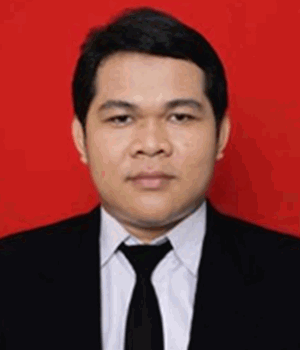
Yahya Nur Ifriza
Yahya Nur Ifriza received in Master of Informatic System from Universitas Diponegoro in 2017. Currently, he is a Lecturer at department of computer sciences, Universitas Negeri Semarang. He has interested research in data mining and wireless sensor network. He can be contacted at email: [email protected].