Abstract
In today’s digital landscape, the pervasive use of digital images across diverse domains has led to growing concerns regarding their authenticity and reliability. The potential for malicious manipulation of these images underscores the critical need to develop robust methods for detecting tampering and ensuring their integrity. Fragile watermarking has been found to have extensive applications for tamper detection and recovery. An image watermarking technique for tamper detection, correction, and recovery is presented in this study. The proposed method employs a self-embedding method to generate the reference watermark from the original image, which has the advantage of superior tamper detection, localization, recovery capabilities, and robustness against attacks. Set Partitioning in the Hierarchical Tree (SPIHT) algorithm is applied to generate a reference watermark of the original image. Low-Density Parity Check (LDPC) is employed for error correction, providing higher-quality reconstruction to recover the original image. Schur decomposition processed the watermarked image blocks to generate authentication bits for each block to enhance tampering detection. The proposed watermarking method was evaluated using PSNR, SSIM, BER, and NC metrics for grayscale and colored images. The technique demonstrated high robustness in tamper detection and recovery against various malicious attacks. Comparative analysis with existing methods shows the efficacy of the proposed method.
Disclosure statement
We have no conflict of interest related to any financial and personal relationships with other people or organizations that could inappropriately influence our work. We have not received any funds or grants from any organizations.
Data availability statement
Data sharing not applicable to this article as no datasets were generated or analyzed during the current study.
Additional information
Notes on contributors
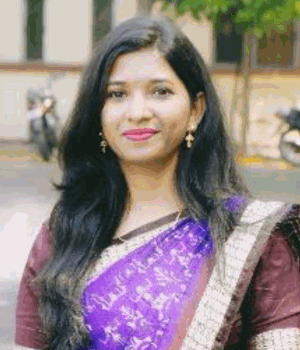
Priyanka Priyadarshini
Ms. Priyanka Priyadarshini completed her B.Tech. in Computer Science and Engineering from College of Engineering and Technology (CET), Bhubaneswar, Odisha, India, received the M.Tech degree in Computer Science and Engineering from the Veer Surendra Sai University of Technology, Burla, Odisha, India, in 2020. She is currently pursuing the Ph.D. degree with Veer Surendra Sai University of Technology, Burla, Odisha, India. She is working as Assistant professor in Sambalpur University Institute of Information Technology (SUIIT), Burla, Sambalpur, Odisha, India. She has published many research papers in reputed Inter- national conference and journals. Her main area of research include Cryptography and network security, Multimedia Security, Watermarking, Image processing, Steganography.
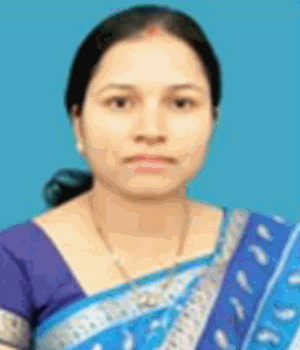
Kshiramani Naik
Dr. Kshiramani Naik is presently working as an Assistant Professor in the Dept. Information Technology, Veer Surendra Sai University of Technology, Odisha, India. She did her Ph.D. in Computer Science and Engineering from Indian School of Mines, Dhanbad in 2017. Her research interest includes Image Cryptosystem, Steganography and Watermarking. She has more than six years of teaching and research experiences. She has published more than ten papers of conference and journals of reputes. Currently she is guiding four Ph.D. students.