ABSTRACT
Participatory planning is a democratic spatial decision-making process involving multiple stakeholders. The integration of artificial intelligence (AI) methods in participatory planning has the potential to improve the decision-making process. However, there are challenges and limitations that need to be addressed. In this paper, we systematically review the progress of AI-enabled participatory planning, identifying strengths and weaknesses. We used a Strengths, Weaknesses, Opportunities, and Threats (SWOT) framework for our analysis, highlighting the opportunities for advancing AI in participatory planning and the potential threats that may arise. Our study provides valuable insights into the current state of AI-enabled participatory planning, paving the way for future developments and improvements.
Highlights
Deep learning elevates participatory spatial decisions.
AI’s strengths in urban planning are on data, communication, and automation.
Emerging AI tools support richer urban research contexts.
Challenges remain on digital divide, trust, privacy, and accountability.
AI’s potential is an ethical urban asset rather than a controversial adversary.
1. Introduction
As the need for equity in urban development is increasingly recognized, collaborative community-engaged processes (i.e. participatory planning) have been suggested as a better approach to local decision making than technocratic and authority-led models (Innes & Booher, Citation2018; Ye, Wu, Lemke, Valera, & Sackey, Citation2022). Arnstein (Citation1969) created a typology of citizen involvement in local decision making and identified the lack of participatory approaches to urban planning. In her typology from low level participation to high level participation, non-participation meant that public decision-making lacked community consultation; tokenism meant the power holders would listen to the participants, but the participants did not have co-decision making power; and citizen power was the ideal with community members engaged in co-production of plans and making decisions. Improving participation in urban planning has been promoted by organizations such as International Association for Public Participation (IAP2, Citation2023). Organization for Economic Cooperation and Develoment (OECD, Citation2021) and the United Nations’ in its sustainable development goals (United Nations, Citationn.d.).
Participatory planning involves many activities, including identifying participants, determining roles and potential contributions of participants, identifying shared goals and the current local condition, communication between stakeholders, and the planning procedure. These activities are usually carried out in organized workshops involving reviewing documents, listening to domain experts, interacting with information systems, writing reports, and discussion and dissemination of results (Haqbeen, Sahab, Ito, & Rizzi, Citation2021; Martinez-Lopez et al., Citation2019; Ye, Du, & Ye, Citation2021). Increasingly, planning activities are aided by participatory mapping practices involving on-line tools and data-driven workflows underpinning the concept of ‘smart cities’ (Afzalan, Sanchez, & Evans-Cowley, Citation2017; Jankowski et al., Citation2021).
The practice of participatory planning has been evolving with new conceptual frameworks and technologies, including collaborative spatial decision support and Artificial Intelligence (AI) (Cai et al., Citation2018; Gorsevski et al., Citation2013; Jankowski & Nyerges, Citation2001; Jelokhani-Niaraki, Citation2021). AI is described as ‘intelligence’ demonstrated by machines that can perform like humans on tasks such as recognizing and localizing objects in images, summarizing an article, or recommending commodities to customers, tasks which would typically require human intelligence to solve but now can be largely automated with AI (An et al., Citation2023; Dwivedi et al., Citation2021; Sanchez, Shumway, Gordner, & Lim, Citation2022).
Though the concept of AI can be traced back to 1943 (Hochreiter & Schmidhuber, Citation1997; Wiggins & Ferreira, Citation1992), for example, machine learning is generally considered a subset of AI, referring to mathematical models that can learn patterns from data and infer unknown outcomes based on the new input, such as regression analysis. AI research and applications have been recently expanding into various domains largely due to the availability of big data and increased computing power (Allam & Dhunny, Citation2019; Li, Citation2018). It is then critical to explore how modern AI can support or even transform the current planning practice from a traditionally technocratic expert-driven process to a more inclusive participatory process increasingly relaying on data, computing capability, and large models. Hence, a systematic review of AI in participatory planning is timely given the advances in both fields.
The aim of this systematic literature review is to evaluate the current state of AI integration into participatory planning, a democratic spatial decision-making process involving multiple stakeholders. We seek to answer the following research questions:
What are the key strengths and weaknesses of integrating AI into participatory planning processes as identified in the existing literature?
What opportunities and threats can be observed for the future development and application of AI in participatory planning?
How can these insights inform the advancement of AI-enabled participatory planning and mitigate potential risks associated with its implementation?
By addressing these research questions, our study aims to provide valuable insights into the current state of AI-enabled participatory planning, offering guidance for future developments and improvements in this interdisciplinary domain.
This paper is organized as follows: first, we outline the review methods used in this study. Then, we present our findings and discussions on the topic of AI-enabled participatory planning, using a Strengths, Weaknesses, Opportunities, and Threats (SWOT) framework to structure our analysis. In the findings section, we identify the benefits resulting from the integration of AI with participatory planning (i.e. strengths), as well as the limitations of the current state of integration (i.e. weaknesses). In the discussion section, we explore how new AI technology may further facilitate participatory planning processes (i.e. opportunities) and how the development of participatory planning can also advance the integration of AI. Finally, we consider possible negative aspects of such integration (i.e. threats).
2. Review methods
This paper investigates AI-enabled participatory planning in terms of the existing research, tasks, data, and methods. The review process at the most rudimentary level involves a four-stage analysis framework including literature search, screening process, selection of the screened literature, and adding referenced literature based on the screened results (Page et al., Citation2021). We limited our initial literature search to the SCI (Science Citation Index) and SSCI (Social Science Citation Index) databases to ensure the quality of the literature. Publications were retrieved from the databases using four groups of terms: (1) the paper must use AI technology; (2) the research needs to include multiple stakeholders; (3) the research question should be spatially defined; (4) the research topic must be related to participatory planning. While there has been a significant body of research focusing on spatial decision support systems (SDSS), relatively few research efforts have been dedicated to using SDSS in the collaborative planning context. In addition, only a few papers aimed at the level of participation between tokenism and citizen power based on Arnstein typology, while most papers focused on developing methods for delivering information to the public. We present the structured keywords lists and detailed review procedure in the appendix.
After conducting a thorough review of the literature, it was observed that the majority of the papers were from the Participatory Planning Support Systems (PPSS) literature. This finding is not surprising since AI models are digital models that can only exist in information systems such as PPSS. PPSS are valuable tools and processes that promote collaborative decision-making in planning by enabling multiple stakeholders to participate in the planning process. These systems usually leverage digital technologies such as Geographic Information Systems (GIS), simulation models, and web-based platforms to facilitate data sharing, communication, and decision-making. For this reason, our focus would be the recent deep learning methods and the outcomes of the participatory spatial decision-making.
SWOT (Strengths, Weaknesses, Opportunities, and Threats) analysis was chosen as the framework for this systematic review because of its suitability in evaluating complex, multidimensional topics. SWOT provides a structured approach for analyzing internal and external factors that can influence the effect of AI in PPSS. By examining strengths, weaknesses, opportunities, and threats, SWOT allows for a comprehensive understanding of this subject and helps identify areas where improvements can be made or potential risks mitigated.
We conduct the SWOT analysis in the following steps: Firstly, from the 97 papers included in the review, we extracted relevant information on the participatory processes, AI methods, and applications. Secondly, the extracted data were categorized based on the four components of the SWOT framework. Strengths and weaknesses focused on the current state of AI integration in participatory planning, while opportunities and threats considered future developments, potential issues, and external factors affecting the domain. Thirdly, for each SWOT category, the research team reviewed the categorized data, identifying common themes, trends, and patterns. Contradictions, gaps, and discrepancies in the literature were also noted to provide a more nuanced understanding of the topic. At last, the findings from the analysis were synthesized to provide an overview of the current state of AI-enabled participatory planning, as well as insights into potential future developments, challenges, and risks. Recommendations for improving the integration of AI in participatory planning were also drawn from the synthesis.
3. Findings
In , we present an annotated bibliography of selected case studies, curated based on our assessment of their relevance and contribution. This compilation serves as a reference, detailing the use of various participatory planning procedures and AI methods in urban planning.
Table 1. Example of case studies with both AI and participatory planning (full table see appendix Table A).
Based on the table, the most widely used participatory planning procedures in urban planning include collecting local knowledge, predicting outcomes of alternative plans and facilitating communication. Concurrently, the AI methodologies predominantly employed in these procedures range from diverse optimization and prediction algorithms to natural language processing and computer vision models. In the ensuing sections, we dissect the principal findings utilizing a SWOT analysis framework.
3.1. Strengths
3.1.1. Analyzing local knowledge through big data
Local knowledge is essential for planning. Traditional data were mainly acquired from structured data, such as from the census of population and housing. AI could better understand the fine-scale local environment from unstructured data, such as text and images (Ye et al., Citation2021). It might provide information to planners about residents’ place-based values and land-use preferences in the absence of well-attended community meetings. It could also offer scalability to infer the preferences and needs from a sample representing a much larger group of people. Some unstructured data types include:
3.1.1.1. Offline behaviour data
GPS trajectory data derived from smartphones, credit card records, and records from smart cards in transit systems present the human behaviour in the built environment (Goodchild, Citation2010). These human behaviour data can reflect the attractiveness and accessibility of places, which can advance our knowledge of the urban environment (Zhu et al., Citation2020). Various AI models can be designed to learn from the movement data and predict future traffic conditions (Li, Xia, & Chai, Citation2021; F. Liu et al., Citation2020; Schläpfer et al., Citation2021).
3.1.1.2. Online behaviour data
Social media platforms provide information on space, time, content (text, image, video, voice), and social network connectivity, which would be valuable for understanding citizen preferences within planning (Wang & Ye, Citation2018). AI models can help extract and analyze user preferences and opinions from the unstructured social media content and other online platforms (Ai, Comfort, Dong, & Znati, Citation2016; Bakht, El-Diraby, & Hossaini, Citation2018; Martinez-Lopez et al., Citation2019; Kaklauskas et al., Citation2021).
3.1.1.3. Street view imagery
Street-level imagery can be used to measure the human perception of a large-scale urban region (Biljecki & Ito, Citation2021). Zhang, Wu, Zhu, and Liu (Citation2019) created a dataset from street images labelled with human positive and negative perceptions such as safe, lively, beautiful, wealthy, depressing, and boring; then used AI models to segment street images into green infrastructure and other indicators to describe city features. Planners can thus enhance the design of the target area by taking into account people’s perceptions of the area (Zhang et al., Citation2019).
3.1.1.4. Air quality data
Air quality data have been one of the most important indicators in urban health research (Miranda et al., Citation2016; Sirbu et al., Citation2015). Sirbu et al. (Citation2015) adopted the AI models to predict the air quality of cities, showing that direct involvement of community members could enhance environmental awareness.
3.1.1.5. Sound Data
Environmental sound and soundscape have a great impact on the quality of human life (Stamatiadou, Thoidis, Vryzas, Vrysis, & Dimoulas, Citation2021). AI models have been utilized to forecast the noise levels of upcoming projects and to propose alternative design plans. For example, Stamatiadou et al. (Citation2021) used AI models to automatically label the crowdsourced soundscape data for the heritage preservation planning and recovery process.
3.1.1.6. Building information
AI models help capture the 3D information about built environments from various images, such as reconstructing height information from multiple angles. Aided by this information, equity building design can be realized such as promoting the equitable access to sunlight (Yasumoto, Jones, Yano, & Nakaya, Citation2012).
These AI techniques can help to provide richer, more nuanced insights into urban environments and community preferences, complementing the costly survey and participatory workshops. These data lay the foundation for participants to discuss design options in the planning process. Knowing the information from the data can make the planning discussion rational and may inspire alternative plans.
3.1.2. Predicting results of alternative plans
One of the strengths of AI is the ability to classify and predict given a sufficient amount of data. As more data becomes available from the current and past planning processes, AI should improve its ability to predict planning outcomes. This adaptability can be particularly valuable in the dynamic and complex context of urban planning. The participatory planning process requires that the alternative plans (planning scenarios) be discussed by stakeholders to reach rational decisions, where the AI prediction power can provide anticipated outcomes and inform the planning decision.
AI models have also been used for predicting the acceptance of alternative plans based on socio-demographic and economic characteristics of urban residents (Dong, Ratti, & Zheng, Citation2019; Lock, Bain, & Pettit, Citation2021). Climate change is factor planners need to consider for resilient and sustainable communities (Hu et al., Citation2018; Lieberknecht, Citation2021; Meerow & Woodruff, Citation2020). Abbot and Marohasy (Citation2013) highlighted the benefits of combining multiple non-linear relationships using neural networks to predict weather patterns, which can increase by 10% the absolute value of the prediction accuracy on rainfalls compared with previous models.
Transportation planning is an important domain of urban planning. AI algorithms have improved traffic prediction accuracy by about 20%∼50% in Google Maps. AI models demonstrated their effectiveness in helping users resolve over-subscribed scheduling problems and evaluate the robustness of existing solutions for urban transportation planning and transit system management. Those models can better inform traffic planning by incorporating traffic pattern changes (Du, Zhang, Du, & Liu, Citation2020).
AI models would estimate project costs. Uncertainty in cost estimation is bound to lead to participatory planning project failure (Nijkamp, van der Burch, & Vindigni, Citation2002). AI models were used to determine a property tax incentive programme eligibility and predict housing value increases based on different planning scenarios in Atlanta, outperforming traditional hedonic methods in prediction accuracy (van den Homberg, Gevaert, & Georgiadou, Citation2020). The community members were invited to discuss and provide information and opinions about the programme, which increased the estimation accuracy of the model and validated the modelling, leading to a novel integration of AI and participatory planning. By altering the input variables and inspecting the model output, planning participants can improve financial policy (Nousdilis et al., Citation2020). The co-design model in the participatory planning system allows users to discuss and annotate the prototype before being exposed to all the interactions available in the fully developed systems (Lock et al., Citation2021).
AI models could also be used for recommending alternative plans to acheive multiple goals. Molina (Citation2005) created an AI-based intelligent assistant for simulating the transportation decision process in Torino (Italy) and Vitoria (Spain), including diagnosis, prediction, and planning. The model supports generating feasible measures (e.g. financial support, zoning regulations) to accomplish the optimum long-term plans by formulating the planning problem in a mathematical format. Zhang et al. (Citation2018) developed the CityMatrix to let non-experts change land-use patterns and predict the corresponding traffic scenario. Using social media to communicate with residents, the output of AI models informs participants of the possible consequences of alternative emergency management plans (Ai et al., Citation2016).
3.1.3. Relieving the burden of plan generation
Besides the potential to generate alternative plan scenarios, AI models can partially automate the plan generation. This automation lowers the cost of participatory planning, making participation affordable even for low-income communities.
Urban planners can adopt AI models to generate maps of planned changes. For example, Yu, Zhang, Li, Montenegro-Marin, and Kumar (Citation2021) designed an AI model that optimized the logistics in a waste management system with multiple stakeholders, improving the planning and management performance, accuracy, and efficiency. Given the site boundary and the number of buildings, Dehaene (Citation2020) designed a model based on the ant colony optimization algorithms to automatically arrange the site planning by minimizing safety concerns and reducing construction costs. Participants can change the constraints or requirements based on the auto-produced plan to quickly see the revised plan, achieved by coevolutionary and genetic algorithm-based methods in such automated planning support systems (Quan, Park, Economou, & Lee, Citation2019). The AI provides the ability to maintain spatial relations among the plan’s structural elements as the design diagram is transformed, to recognize ‘emergent’ patterns and configurations in a diagram, to perform transformations that carry one diagram to another, to identify similarities and differences among diagrams, and to represent designs at varying levels of abstraction and detail (Do & Gross, Citation2001).
AI models can also retrieve relevant data to inspire designers (Q. Liu et al., Citation2020), making it possible to learn stylistic design criteria from existing urban designs and/or landscape architectural designs and transfer these styles to other designs. Ye et al. (Citation2021) developed an AI model that can automate the process of colouring masterplans and quickly make changes to the result. These applications learn the transfer patterns between the source and target data in the training process and then automatically apply them to a similar dataset.
3.1.4. Facilitating communications between stakeholders
Providing more information and reducing the plan costs support participatory planning in a general way, as these AI abilities would benefit the broader planning practice. There are also specific benefits of using AI in participatory planning. Auto-transcript and auto-translation services in online meetings can enable easier information access for other language speakers and people who need hearing aids (Tomašev et al., Citation2020). Here we discuss some detailed examples of how the integration of AI technology with a planning information system can facilitate communication between stakeholders.
The selection of participants is usually the first step in participatory planning. Ideally, the participants would encompass all stakeholders including residents affected by the proposed plan, professional planners, representatives of local government agencies, community leaders, consultants, and experts (Rodriguez-Soto, Velazquez, Monroy-Vilchis, Lemes, & Loyola, Citation2017). Yang et al. (Citation2020) classified the experts’ professional level by developing a machine-learning algorithm based on the indicators such as professional title, age, education degree, the field of expertise, number of published papers, and number of patents. The output of the AI model was used to identify the domain experts and how expert opinion should be weighted in the final decision.
When analyzing nine urban land-use and revitalization projects in the Netherlands, Nijkamp et al. (Citation2002) found a public-private partnership based on the joint-venture model has a higher chance of success. The participants first came up with many indicators for assessing the sustainability of communities, then they used the AI model to identify the redundant indicators (Kumar, Bhaumik, & Banerji, Citation2021). Escobedo, Bottin, Cala, and Montoya (Citation2020) employed the AI model to assess different stakeholders’ abilities in recognizing various landscape processes, and to design more context-relevant survey instruments for participatory planning.
AI models would extract the significant information nuggets from the participant's input and update those involved in the discussion. Topic modelling summarizes the main themes contained in user posts. Sentiment analysis models distinguish people’s attitudes (i.e. positive or negative) towards specific policies. Shin, Rajabifard, Kalantari, and Atazadeh (Citation2021) built a machine learning model to predict the level of property disputes. AI models can map the place mentioned by the participants, providing the spatial context for a discussion (Shin, Yuan, Siong, Zhang, & Phang, Citation2017).
AI models also could encourage people to share opinions. For example, the recent success of deep learning-based large-scale language models significantly boosts the performance in many languages understanding tasks such as reading comprehension, question answering, and chatbot systems (Brown et al., Citation2020). This enables AI such as a chatbot to automatically generate the most plausible response given a context. By responding to the reported issues by participants in the online forum, the chatbot can mediate the discussion by posting meaningful messages and replying to user posts based on the pre-defined answers (Haqbeen et al., Citation2021). Public participation experiments in urban planning in Afghanistan showed that the AI facilitator could achieve almost the same response rate as the human facilitator. Furthermore, AI paired with Blockchain technology can enhance the credibility of participants by recording the participatory process (Pournaras, Citation2020).
In addition to building trust by improving the credibility of participants, AI models can synthesize the discussions and help people reach the consensus with decision models if every participant agrees on a standardized decision procedure. There is a rich literature about standardized group decision models based on brainstorming, voting, and ranking (Jankowski & Nyerges, Citation2001; Jelokhani-Niaraki, Citation2021). Agent-based models can be used to predict the voting results (Aguirre & Nyerges, Citation2014). By changing the behaviour of automated agents, an alternative decision process pathway might be discovered.
3.1.5. Educating participants with scientific evidence
One benefit of participatory planning is shared understanding developed by all parties through communication and collaboration. If public participation is intended to produce systematic changes, all stakeholders need equal and equitable access to data and information resources (Rosen & Painter, Citation2019). AI models can make scientific evidence more accessible to the public involved in planning. Professional planner can use scientific evidence to inform the general public. For example, Walisadeera, Ginige, and Wikramanayake (Citation2015) developed an AI model to automatically collect knowledge from government websites, agriculture department leaflets, and radio and television programmes on agriculture for Sri Lankan farmers, enabling them to defend their interests.
Access to more information can boost the participation rate. After informing the public in detail, the participation rate increased by 3%∼17% in the household hazardous waste collection and recycling programme. Lim-Wavde, Kauffman, and Dawson (Citation2017). People involved in the community decision procedure gained access to scientific and technical guidance because they volunteered to collaborate with a local university and reach the disadvantaged community members (Girbes-Peco, Renta-Davids, De Botton, & Alvarez-Cifuentes, Citation2020). AI models also helped participants to understand science (Rivet & Krajcik, Citation2004) and increased their environmental awareness (Sirbu et al., Citation2015).
3.2. Weaknesses
3.2.1. Lack of documentation on AI’s impact on group processes and outcomes
Although there are claims in the literature about the usefulness of AI in participatory planning, very few of them document how the technology changes group process and outcomes. The goal of participatory planning is to empower community members by allowing everyone to contribute spatially-explicit values, preferences, and experiences to the decision making process Most of the reviewed papers in (and appendix Table A) described methods and their use in a participatory process but stopped short of reporting on how the used methods affected the decision making.
3.2.2. Data sampling biases
Looking at the AI methods, data sampling biases are a major concern because data shape the machine learning models. Incorrect or biased data leads to useless interpretation and analysis. The data and information contributed by participants may suffer from various biases including demographic, education, and spatial imbalance (Ibrahim, Khodursky, & Yasseri, Citation2021). The neighbourhoods with limited infrastructure and low-income populations produce relatively small amounts of digital signals in comparison to more affluent neighbourhoods (Long, Zhai, Shen, & Ye, Citation2018). Researchers should consider these data biases when collecting the data.
3.2.3. Lack of causal explanation in deep learning methods
New deep learning methods can boost prediction and simulation performance. However, most of them cannot explain the causal relationships among variables, making some prediction models difficult to be applied in planning practice. For example, the population is always a crucial independent variable in the housing price prediction model, but housing and population are co-dependent. Causal machine learning methods have a great potential to help planners and participants to understand the causal structure among different variables. Those methods would generate a series of possible causal relationships between the variables, then score the possibilities with a variety of methods or ran independent tests to get the most possible causal relation (Schölkopf et al., Citation2021).
3.2.4. Limited familiarity with AI methods
However, planners and urban researchers may not be familiar with AI methods. There still are challenges in sharing and using new data and methods across disciplines, as many argue that urban planning is siloed and lacks common methodology. For example, architects and planners infrequently collaborate in educational programmes (Malczewski & Jankowski, Citation2020). Urban planning education typically does not equip future practitioners with AI knowledge. AI-focused education should be added to urban planning curricula to prepare future planners for using AI techniques in planning practice and communicating about their results with the public.
3.2.5. Resource constraints
Limited resources in the planning field are another constraint. Municipal planning offices typically have limited budgets and tight work schedules to accomplish planning processes such as plan designs, public and stakeholder consultations, and institutional approvals. The extra costs of adopting AI technology in participatory planning need to be justified. The AI application costs include the software license fees and the hardware needed to deploy the application (Kontokosta, Citation2018). An AI chatbot usually charges its customers based on user numbers and provides only specific language services (Haqbeen et al., Citation2021). In addition, the code of online forums was developed with different programming languages, requiring multiple technicians to maintain. Planners may find it hard to adopt new AI technologies because of their lack of expertise and funding.
3.2.6. Ethical considerations
The use of AI in decision-making also raises ethical considerations. The potential for AI to perpetuate existing biases, unequal power relations, and exclusionary practices calls for careful consideration of the ethical implications of AI use in participatory planning (Gorodnova et al., 2020; Falco Citation2019; Cai et,al 2018). Ensuring transparency, accountability, and inclusivity in AI decision-making processes is crucial to promote more equitable and sustainable outcomes.
4. Discussion
The integration of AI in participatory planning has sparked a discourse on its comparative efficacy against traditional technocratic and authority-led models in local decision-making. Advocates posit that AI fosters a more transparent, inclusive, and accountable decision-making process by empowering diverse stakeholders to participate and share their knowledge and preferences. Conversely, critics caution about the potential for AI to reinforce existing biases, power imbalances, and exclusionary practices. Therefore, it is crucial to weigh the advantages and risks of AI in participatory planning, and to investigate how these systems can be designed and implemented to advance more equitable and sustainable outcomes. The insights presented in this section are derived from a systematic literature review, as summarized in , and they form the basis for our detailed discussion on the opportunities and threats of AI in PPSS.
4.1. Opportunities
Beyond the integration issues, we look for opportunities in planning and the AI research community to advance participatory planning further. How might advancements in AI research be applied to participatory planning? How does the development of planning theory and practice guide AI integration? We tackle these questions in this section.
The development of plans demands extensive knowledge of different technical and scientific domains and the comprehension of interactions between these domains (Austin, Delgoshaei, Coelho, & Heidarinejad, Citation2020; Jankovic & Zarate, Citation2011). It requires the collaboration of planning experts and stakeholders (Jankowski & Nyerges, Citation2001), which would be a core element in participatory planning. For example, climate planning asks for knowledge about environmental health, urban heat planning, and climate-related land use planning (Lieberknecht, Citation2021).
Recent advances in knowledge graphs have the potential to address the interdisciplinarity challenges. The vast heterogeneity of the disciplines involved in participatory planning ranging from the natural sciences to the social sciences (e.g. meteorology, environmental planning, urban planning, human geography) demonstrates new challenges in terms of data accessibility, reusability, and interoperability while geospatial semantics and knowledge graphs have the potential to overcome these challenges (Mai, Janowicz, Cai, et al., Citation2020, p. 2). A knowledge graph is a form of data representation that connects pieces of information together. In a knowledge graph, nodes represent entities (like people, places, or things), and edges represent relationships between these entities. For example, in a knowledge graph about a planning department, nodes might represent employees, departments, and projects, while edges might represent relationships like ‘works in’ or ‘manages’. Knowledge graphs are used in many applications, including search engines, recommendation systems, and AI systems, because they provide a structured, easily understandable way to represent complex relationships. Knowledge graphs have shown promising results in data integration & geographic entity alignment (Du, Wang, Ye, Sinton, & Kemp, Citation2021), geographic question answering (Mai, Janowicz, Yan, et al., Citation2020), and others. Utilizing knowledge graphs would be a possible solution to overcoming the interdisciplinarity challenge by providing a common knowledge base for planning. The ability to inform decision makers from the participatory planning process would be the key to overcome various bias (Innes & Booher, Citation2018).
Numerous AI models are freely accessible on the internet for planners to harness. They often provide user-friendly interfaces, such as that of ChatGPT(chat.openai.com), making these models readily usable without any financial cost. While it is important to take caution when using such models because those model are not open and they may try to collect sensitive information. Efficiently designed AI tools streamline the user experience by abstracting the intricate technical details. This approach makes AI capabilities more accessible, as it does not necessitate users to have extensive AI knowledge.
Not only many AI models are free, but also many models and their data are open sourced, as many developers choose to open-source their code on platforms like GitHub (www.github.com). This open policy tradition within the AI community not only democratizes access to both information and analytical tools but also fosters a larger pool of contributors, encouraging a wider range of ideas. This, in turn, facilitates continuous improvement and potentially catalyzes innovations in theory and practice (Wang & Ye, Citation2018). The transparency inherent in open-source AI models and data is unique. Unlike human actors, AI models ideally do not hold personal interests, and their decision-making processes are based solely on the data and algorithms they are trained on. This transparency can build trust among collaborators, as they can verify the data and algorithms used in the decision-making process. This trust, coupled with the shared understanding of the resources at hand, may foster cooperation and facilitate collective problem-solving.
The development of quantitative methods for evaluating diverse plans enhances the application of AI. This concept is illustrated in the work of Berke & French (Citation1994), where plan quality was assessed based on three aspects: (1) factual basis, (2) clear and comprehensive goals, and (3) action-oriented policies. Additional elements, such as plan implementation actions, monitoring strategies, inter-governmental coordination, and participation, were later incorporated (Berke et al., Citation2012). Rule-based models have already been utilized to verify whether a plan adheres to regulations for fire safety and yard setback requirements (Heikkila & Blewett, Citation1992). While these models can be effective in certain contexts, they also have limitations. For instance, they may oversimplify complex planning issues, overlook contextual nuances, and struggle to adapt to changing circumstances. However, with more attributes of plan quality are expressed in a mathematical format, AI models can capture more aspects of the plan and environment, thereby automating the evaluation of planning.
Falco (Citation2019) introduced the concept of participatory AI, envisioning the use of blockchain technology to ensure transparency in AI applications within smart cities. For example, the text mentions that if the community had commented on the algorithm behind the AI for the Chicago Police Department’s ‘strategic subject list’, the AI could have been less biased and more socially responsible. This technology provides a historical record of comments that remains accessible for future scrutiny of the AI’s decisions. Such emerging technologies could potentially bolster confidence in the transparency and security of future participatory planning practices.
4.2. Threats
Applying AI in participatory planning faces threats, many of which are beyond the planners’ control. Some fear that key individuals, including proponents and opponents of a plan, might game the system to achieve their ends. Some critics argue that participatory planning can be manipulated so it will only benefit powerful people. For example, automated social media accounts can be used to influence the land development and planning process by generating ‘an environment in which distortions were propagated’ (Hollander, Potts, Hartt, & Situ, Citation2020).
Applying AI in participatory planning faces legal challenges such as privacy agreements and accountability. The popular social media platform, Twitter, puts constraints on its policy for collecting user information, requiring detailed plans provided by any researcher who hopes to analyze the user-generated content. Still, people would worry their privacy might be revealed by AI models (Walter & Scholz, Citation2007; Wang & Ye, Citation2018). While humans traditionally held responsibility for their decisions, the advent of AI has introduced a potential shift in this dynamic, with individuals potentially attributing poor decisions to these technologies. This raises significant concerns about accountability, particularly among politicians (van den Homberg et al., Citation2020). Despite AI’s ability to perform certain tasks akin to humans, it is crucial to remember that ultimate decision-making responsibility still resides with humans.
The use of AI in planning poses the question of whether anyone would willingly surrender decision-making power in whole or in part to a machine. Put differently, can people trust AI? This is expressed in two distinct ways – people may think technology is unreliable or they may overtrust the machine. We found assertions in the literature suggesting that the general public welcomes various AI applications (Fagerholm et al., Citation2021; Lock et al., Citation2021; Sirbu et al., Citation2015).
However, skepticism may arise among non-experts when planners are unable to explain a model or adjust their expectations accordingly. In those cases, the AI models are an un-explainable black box (Frazier, Wikle, & Kedron, Citation2018). Research on explainable AI sheds light on a potential solution but still faces theoretical challenges (Slack, Hilgard, Jia, Singh, & Lakkaraju, Citation2019). Analyzing how humans build trust toward each other would also help to find ways for people to trust AI (Gillath et al., Citation2021).
Generally, AI models are very complex, and many are not mature. The short life of technology and a relatively small number of application domains cannot provide enough evidence for efficiency and good performance, making it difficult for people to build trust in AI systems. The reproducibility and replicability of the empirical studies of integrating AI with planning are problematic. Unlike algorithms, whose accuracy can be readily improved through data refinement and code optimization, replicating human behaviour in a participatory planning workshop presents a significant challenge (Wilson et al., Citation2021). This complexity arises from the unique characteristics of each individual and the constantly evolving dynamics of social interactions, both of which warrant further research.
Many deep learning models have been experimentally incorporated within the planning process as individual components, yet a plan created entirely by AI remains unseen. While AI can assist planners by facilitating the development of alternative designs, it cannot supplant planners in the current stage of AI development when it comes to creativity and understanding the physical, social, legal, and political characteristics of areas subject to planning practice. The integration of AI and participatory planning is still in its nascent stages, with only a handful of case studies addressing real-world planning problems identified in our literature review, such as those by Haqbeen et al. (Citation2021), Lock et al. (Citation2021), Auerbach et al. (Citation2020), and Escobedo et al. (Citation2020).
6. Conclusion
The manuscript’s contribution is to review recent deep learning methods and their outcomes in participatory spatial decision-making. The state of the current practice of AI in participatory planning can be characterized as an early stage of integration between the two fields. Our analysis showed the strengths of AI in participatory planning include collecting more local knowledge through unstructured data, facilitating communication between stakeholders, educating participants using scientific evidence, predicting results of alternative plans, and automating plan generation. The weaknesses of the AI models in participatory planning include the data sample bias, lack of the ability to model causality, and the costs of education, software, and hardware.
We identified opportunities for several potential applications in participatory planning based on the recent advances in planning and AI. For example, new deep learning models and knowledge-graph-driven applications can support planning decisions. New sources of data offer more information on the target participants, setting up a rich empirical context for urban research and policy interventions. Automated plan evaluation tools are enabled thanks to quantitative planning evaluation research and lay participants can be supported in contributing preferences and local knowledge by AI-enabled design tools. Lastly, we discussed the threats to the integration including the barrier of the digital divide, model trustworthiness, privacy issues, and algorithm accountability as serious impediments to progress in embedding AI in participatory planning.
This systematic literature review has surveyed recent publications on AI and participatory planning. Yet, due to the rapid development of both fields, some studies might have been overlooked. The field emerging at the intersection of planning and AI calls for more synthesis efforts and a comprehensive framework to realize the potential benefits of the synergy of participatory planning. Future research should focus on ways of making AI an ethical and trustworthy urban infrastructure asset rather than an adversary fraught with controversy and bias.
Data and codes availability statement
As this is a review paper, data and codes do not apply.
Disclosure statement
No potential conflict of interest was reported by the author(s). We wish to extend our sincere gratitude to the anonymous reviewers for their insightful comments, constructive criticisms, and invaluable suggestions, all of which significantly improved the quality of this paper. We also thank the editor for their guidance and support throughout the review process. Their collective expertise and dedication have greatly enhanced our work. Additionally, we are grateful for the discussions with Dr. Walter Peacock and Dr. Michelle Meyer from Texas A&M University.
Correction Statement
This article has been corrected with minor changes. These changes do not impact the academic content of the article.
Additional information
Funding
References
- Abbot, J., & Marohasy, J. (2013). The potential benefits of using artificial intelligence for monthly rainfall forecasting for the Bowen Basin, Queensland, Australia. Water Resources Management VII, 171, 287.
- Afzalan, N., Sanchez, T. W., & Evans-Cowley, J. (2017). Creating smarter cities: Considerations for selecting online participatory tools. Cities, 67, 21–30.
- Aguirre, R., & Nyerges, T. (2014). An agent-based model of public participation in sustainability management. In JASSS-The Journal of Artificial Societies and Social Simulation (Vol. 17, Issue 1). J A S S S. https://doi.org/10.18564/jasss.2297
- Ai, F., Comfort, L., Dong, Y., & Znati, T. (2016). A dynamic decision support system based on geographical information and mobile social networks: A model for tsunami risk mitigation in Padang, Indonesia. Safety Science, 90, 62–74. https://doi.org/10.1016/j.ssci.2015.09.022
- Allam, Z., & Dhunny, Z. (2019). On big data, artificial intelligence and smart cities. Cities, 89, 80–91. https://doi.org/10.1016/j.cities.2019.01.032
- An, L., Grimm, V., Bai, Y., Sullivan, A., Turner, B. L., II, Malleson, N., … Tang, W. (2023). Modeling agent decision and behavior in the light of data science and artificial intelligence. Environmental Modelling & Software, 166, 105713.
- Arnstein, S. (1969). A ladder of community participation. Journal of the American Institute of Planners, 35, 216–224.
- Auerbach, J., Blackburn, C., Barton, H., Meng, A., & Zegura, E. (2020). Coupling data science with community crowdsourcing for urban renewal policy analysis: An evaluation of Atlanta’s Anti-Displacement Tax Fund. Environment And Planning B-Urban Analytics And City Science, 47(6), 1081–1097. https://doi.org/10.1177/2399808318819847
- Austin, M., Delgoshaei, P., Coelho, M., & Heidarinejad, M. (2020). Architecting smart city digital twins: Combined semantic model and machine learning approach. Journal of Management in Engineering, 36(4). https://doi.org/10.1061/(ASCE)ME.1943-5479.0000774
- Bakht, M., El-Diraby, T., & Hossaini, M. (2018). Game-based crowdsourcing to support collaborative customization of the definition of sustainability. Advanced Engineering Informatics, 38, 501–513. https://doi.org/10.1016/j.aei.2018.08.019
- Barbosa, A., Martin, B., Hermoso, V., Arevalo-Torres, J., Barbiere, J., Martinez-Lopez, J., … Iglesias-Campos, A. (2019). Cost-effective restoration and conservation planning in green and blue infrastructure designs. Science of the Total Environment, 652, 1463–1473. https://doi.org/10.1016/j.scitotenv.2018.10.416.
- Berke, P., Smith, G., & Lyles, W. (2012). Planning for resiliency: Evaluation of state hazard mitigation plans under the disaster mitigation act. Natural Hazards Review, 13(2), 139–149. https://doi.org/10.1061/(ASCE)NH.1527-6996.0000063
- Berke, P. R., & French, S. P. (1994). The influence of state planning mandates on local plan quality. Journal of Planning Education and Research, 13(4), 237–250.
- Biljecki, F., & Ito, K. (2021). Street view imagery in urban analytics and GIS: A review. Landscape and Urban Planning, 215, 104217.
- Brown, T. B., Mann, B., Ryder, N., Subbiah, M., Kaplan, J., Dhariwal, P., … Askell, A. (2020). Language models are few-shot learners. ArXiv Preprint ArXiv:2005.14165. Retrieved from http://arxiv.org/abs/2005.14165
- Cai, L., Fan, L., Lai, W., Long, Y., Wang, P., & Xin, X. (2018). Definition, application and influence of artificial intelligence on design industries. Landscape Architecture Frontiers, 6(2), 56–63. https://doi.org/10.15302/J-LAF-20180207
- Dehaene, S. (2020). How we learn: The new science of education and the brain. London: Penguin.
- Do, E., & Gross, M. (2001). Thinking with diagrams in architectural design. Artificial Intelligence Review, 15(1), 135–149. https://doi.org/10.1023/A:1006661524497
- Dong, L., Ratti, C., & Zheng, S. (2019). Predicting neighborhoods’ socioeconomic attributes using restaurant data. Proceedings of the National Academy of Sciences, 116(31), 15447–15452. https://doi.org/10.1073/pnas.1903064116
- Du, J., Wang, S., Ye, X., Sinton, D. S., & Kemp, K. (2021). GIS-KG: Building a large-scale hierarchical knowledge graph for geographic information science. International Journal of Geographical Information Science, 36, 873–897. https://doi.org/10.1080/13658816.2021.2005795
- Du, J., Zhang, F., Du, Z., & Liu, R. (2020). Assessing vulnerability of road networks based on traffic flow betweenness centrality: A case study in Wuxi. Journal of Zhejiang University, Science Edition, 47(2). https://doi.org/10.3785/j.issn.1008-9497.2020.02.013
- Dwivedi, Y., Hughes, L., Ismagilova, E., Aarts, G., Coombs, C., Crick, T., … Williams, M. (2021). Artificial Intelligence (AI): Multidisciplinary perspectives on emerging challenges, opportunities, and agenda for research, practice and policy. International Journal of Information Management, 57. https://doi.org/10.1016/j.ijinfomgt.2019.08.002
- Elizalde-Ramirez, F., Nigenda, R., Martinez-Salazar, I., & Rios-Solis, Y. (2019). Travel plans in public transit networks using artificial intelligence planning models. Applied Artificial Intelligence, 33(5), 440–461. https://doi.org/10.1080/08839514.2019.1582859
- Escobedo, F., Bottin, M., Cala, D., & Montoya, D. (2020). Spatial literacy influences stakeholder’s recognition and mapping of peri-urban and urban ecosystem services. Urban Ecosystems, 23(5), 1039–1049. https://doi.org/10.1007/s11252-020-00962-y
- Fagerholm, N., Raymond, C. M., Olafsson, A. S., Brown, G., Rinne, T., Hasanzadeh, K., … Kyttä, M. (2021). A methodological framework for analysis of participatory mapping data in research, planning, and management. International Journal of Geographical Information Science, 35(9), 1848–1875. https://doi.org/10.1080/13658816.2020.1869747
- Falco, G. (2019). Participatory AI: Reducing AI bias and developing socially responsible AI in smart cities. 2019 22nd IEEE International Conference on Computational Science and Engineering (IEEE CSE 2019) and 17th IEEE International Conference on Embedded and Ubiquitous Computing (IEEE EUC 2019) (WOS:000521797300030; pp. 160–164). https://doi.org/10.1109/CSE/EUC.2019.00038
- Frazier, A. E., Wikle, T., & Kedron, P. (2018). Exploring the anatomy of Geographic Information Systems and Technology (GIS&T) textbooks. Transactions in GIS, 22(1), 165–182. https://doi.org/10.1111/tgis.12301
- Gillath, O., Ai, T., Branicky, M. S., Keshmiri, S., Davison, R. B., & Spaulding, R. (2021). Attachment and trust in artificial intelligence. Computers in Human Behavior, 115, 106607. https://doi.org/10.1016/j.chb.2020.106607
- Girbes-Peco, S., Renta-Davids, A., De Botton, L., & Alvarez-Cifuentes, P. (2020). The Montserrat’s neighbourhood dream: Involving Moroccan residents in a school-based community development process in urban Spain. Social & Cultural Geography, 21(5), 674–696. https://doi.org/10.1080/14649365.2018.1509112
- Goodchild, M. F. (2010). Towards geodesign: Repurposing cartography and GIS? Cartographic Perspectives, 66, 7–22.
- Gorsevski, P. V., Cathcart, S. C., Mirzaei, G., Jamali, M. M., Ye, X., & Gomezdelcampo, E. (2013). A group-based spatial decision support system for wind farm site selection in Northwest Ohio. Energy Policy, 55, 374–385.
- Haqbeen, J., Sahab, S., Ito, T., & Rizzi, P. (2021). Using decision support system to enable crowd identify neighborhood issues and Its solutions for policy makers: An online experiment at Kabul municipal level. Sustainability, 13(10), 5453. https://doi.org/10.3390/su13105453
- Heikkila, E. J., & Blewett, E. J. (1992). Using expert systems to check compliance with municipal building codes. Journal of the American Planning Association, 58(1), 72–80.
- Hochreiter, S., & Schmidhuber, J. (1997). Long short-term memory. Neural Computation, 9(8), 1735–1780. https://doi.org/10.1162/neco.1997.9.8.1735
- Hollander, J. B., Potts, R., Hartt, M., & Situ, M. (2020). The role of artificial intelligence in community planning. International Journal of Community Well-Being, 3(4), 507–521. https://doi.org/10.1007/s42413-020-00090-7
- Hu, Q., Tang, Z., Zhang, L., Xu, Y., Wu, X., & Zhang, L. (2018). Evaluating climate change adaptation efforts on the US 50 states’ hazard mitigation plans. Natural Hazards, 92(2), 783–804. https://doi.org/10.1007/s11069-018-3225-z
- Hu, Y., Deng, C., & Zhou, Z. (2019). A semantic and sentiment analysis on online neighborhood reviews for understanding the perceptions of people toward their living environments. Annals of the American Association of Geographers, 109(4), 1052–1073.
- IAP2. (2023). International association for public participation statement on diversity, equity and inclusion. Retrieved April 9, 2023, from https://www.iap2.org/page/IAP2-DEI-English.
- Ibrahim, K., Khodursky, S., & Yasseri, T. (2021). Gender imbalance and spatiotemporal patterns of contributions to citizen science projects: The case of Zooniverse. Frontiers in Physics, 9. https://doi.org/10.3389/fphy.2021.650720
- Innes, J. E., & Booher, D. E. (2018). Planning with complexity: An introduction to collaborative rationality for public policy. London; New York: Routledge.
- Jankovic, M., & Zarate, P. (2011). Discrepancies and analogies in artificial intelligence and engineering design approaches in addressing collaborative decision-making. International Journal of Decision Support System Technology, 3(2), 1–14. https://doi.org/10.4018/jdsst.2011040101
- Jankowski, P., Forss, K., Czepkiewicz, M., Saarikoski, H., & Kahila, M. (2021). Assessing impacts of PPGIS on urban land use planning: Evidence from Finland and Poland. European Planning Studies, 30(8), 1529–1548.
- Jankowski, P., & Nyerges, T. (2001). GIS for group decision making. Boca Raton, FL: CRC Press.
- Jelokhani-Niaraki, M. (2021). Collaborative spatial multicriteria evaluation: A review and directions for future research. International Journal of Geographical Information Science, 35(1), 9–42. https://doi.org/10.1080/13658816.2020.1776870
- Kaklauskas, A., Bardauskiene, D., Cerkauskiene, R., Ubarte, I., Raslanas, S., Radvile, E., … Kaklauskiene, L. (2021). Emotions analysis in public spaces for urban planning. Land Use Policy, 107, 105458. https://doi.org/10.1016/j.landusepol.2021.105458
- Kaklauskas, A., Jokubauskas, D., Cerkauskas, J., Dzemyda, G., Ubarte, I., Skirmantas, D., … Simkute, I. (2019). Affective analytics of demonstration sites. Engineering Applications of Artificial Intelligence, 81, 346–372. https://doi.org/10.1016/j.engappai.2019.03.001
- Kerebel, A., Gelinas, N., Dery, S., Voigt, B., & Munson, A. (2019). Landscape aesthetic modelling using Bayesian networks: Conceptual framework and participatory indicator weighting. Landscape and Urban Planning, 185, 258–271. https://doi.org/10.1016/j.landurbplan.2019.02.001
- Kontokosta, C. E. (2018). Urban informatics in the science and practice of planning. Journal of Planning Education and Research. https://doi.org/10.1177/0739456X18793716
- Kumar, S., Bhaumik, S., & Banerji, H. (2021). Methodology for framing indicators for assessing economic-socio-cultural sustainability of the neighbourhood level urban communities in Indian megacities: Evidence from Kolkata. Social Indicators Research, 154(2), 511–544. https://doi.org/10.1007/s11205-020-02559-6
- Li, C., Xia, W., & Chai, Y. (2021). Delineation of an urban community life circle based on a machine-learning estimation of spatiotemporal behavioral demand. Chinese Geographical Science, 31(1), 27–40. https://doi.org/10.1007/s11769-021-1174-z
- Li, W. (2018). Lowering the barriers for accessing distributed geospatial big data to advance spatial data science: The PolarHub solution. Annals of the American Association of Geographers, 108(3), 773–793. https://doi.org/10.1080/24694452.2017.1373625
- Lieberknecht, K. (2021). Community-centered climate planning. Journal of the American Planning Association, 88(0), 97–112. https://doi.org/10.1080/01944363.2021.1896974
- Lim-Wavde, K., Kauffman, R., & Dawson, G. (2017). Household informedness and policy analytics for the collection and recycling of household hazardous waste in California. Resources, Conservation and Recycling, 120, 88–107. https://doi.org/10.1016/j.resconrec.2016.10.007
- Liu, F., Andrienko, G., Andrienko, N., Chen, S., Janssens, D., Wets, G., & Theodoridis, Y. (2020). Citywide traffic analysis based on the combination of visual and analytic approaches. Journal of Geovisualization and Spatial Analysis, 4(2), 15. https://doi.org/10.1007/s41651-020-00057-4
- Liu, Q., Wang, K., Li, Y., & Liu, Y. (2020). Data-driven concept network for inspiring designers’ idea generation. Journal of Computing and Information Science in Engineering, 20(3). https://doi.org/10.1115/1.4046207
- Lock, O., Bain, M., & Pettit, C. (2021). Towards the collaborative development of machine learning techniques in planning support systems – a Sydney example. Environment and Planning B: Urban Analytics and City Science, 48(3), 484–502. https://doi.org/10.1177/2399808320939974
- Lock, O., & Pettit, C. (2020). Social media as passive geo-participation in transportation planning—How effective are topic modeling and sentiment analysis in comparison with citizen surveys?. Geo-Spatial Information Science, 23(4), 275–292. https://doi.org/10.1080/10095020.2020.1815596
- Long, Y., Zhai, W., Shen, Y., & Ye, X. (2018). Understanding uneven urban expansion with natural cities using open data. Landscape and Urban Planning, 177, 281–293. https://doi.org/10.1016/j.landurbplan.2017.05.008
- Mai, G., Janowicz, K., Cai, L., Zhu, R., Regalia, B., Yan, B., … Lao, N. (2020). SE-KGE: A location-aware knowledge graph embedding model for geographic question answering and spatial semantic lifting. Transactions in GIS, 24(3), 623–655. https://doi.org/10.1111/tgis.12629
- Mai, G., Janowicz, K., Yan, B., Zhu, R., Cai, L., & Lao, N. (2020). Multi-scale representation learning for spatial feature distributions using grid cells. International conference on learning representations. Retrieved from https://openreview.net/forum?id=rJljdh4KDH
- Malczewski, J., & Jankowski, P. (2020). Emerging trends and research frontiers in spatial multicriteria analysis. International Journal of Geographical Information Science, 34(7), 1257–1282.
- Martinez-Lopez, J., Teixeira, H., Morgado, M., Almagro, M., Sousa, A., Villa, F., … Lillebo, A. (2019). Participatory coastal management through elicitation of ecosystem service preferences and modelling driven by “coastal squeeze”. Science of the Total Environment, 652, 1113–1128. https://doi.org/10.1016/j.scitotenv.2018.10.309
- Meerow, S., & Woodruff, S. C. (2020). Seven principles of strong climate change planning. Journal of the American Planning Association, 86(1), 39–46. https://doi.org/10.1080/01944363.2019.1652108
- Miranda, A., Relvas, H., Viaene, P., Janssen, S., Brasseur, O., Carnevale, C., … Volta, M. (2016). Applying integrated assessment methodologies to air quality plans: Two European cases. Environmental Science & Policy, 65, 29–38. https://doi.org/10.1016/j.envsci.2016.04.010
- Molina, M. (2005). An intelligent assistant for public transport management. Advances in Intelligent Computing, PT 2, Proceedings (WOS:000232529000021; Vol. 3645, pp. 199–208).
- Nijkamp, P., van der Burch, M., & Vindigni, G. (2002). A comparative institutional evaluation of public-private partnerships in Dutch urban land-use and revitalisation projects. Urban Studies, 39(10), 1865–1880. https://doi.org/10.1080/0042098022000002993
- Nousdilis, A. I., Kryonidis, G. C., Kontis, E. O., Barzegkar-Ntovom, G. A., Panapakidis, I. P., Christoforidis, G. C., & Papagiannis, G. K. (2020). Impact of policy incentives on the promotion of integrated PV and battery storage systems: A techno-economic assessment. IET Renewable Power Generation, 14(7), 1174–1183.
- OECD. (2021). OECD report on public communication. Retrieved from https://www.oecd-ilibrary.org/content/publication/22f8031c-en
- Page, M. J., McKenzie, J. E., Bossuyt, P. M., Boutron, I., Hoffmann, T. C., Mulrow, C. D., … Whiting, P. (2021). The PRISMA 2020 statement: An updated guideline for reporting systematic reviews. BMJ, 372, 1–1.
- Pournaras, E. (2020). Proof of witness presence: Blockchain consensus for augmented democracy in smart cities. Journal of Parallel and Distributed Computing, 145, 160–175. https://doi.org/10.1016/j.jpdc.2020.06.015
- Quan, S., Park, J., Economou, A., & Lee, S. (2019). Artificial intelligence-aided design: Smart design for sustainable city development. Environment and Planning B: Urban Analytics and City Science, 46(8), 1581–1599. https://doi.org/10.1177/2399808319867946
- Rivet, A., & Krajcik, J. (2004). Achieving standards in urban systemic reform: An example of a sixth grade project-based science curriculum. Journal of Research in Science Teaching, 41(7), 669–692. https://doi.org/10.1002/tea.20021
- Rodriguez-Soto, C., Velazquez, A., Monroy-Vilchis, O., Lemes, P., & Loyola, R. (2017). Joint ecological, geographical and cultural approach to identify territories of opportunity for large vertebrates conservation in Mexico. Biodiversity and Conservation, 26(8), 1899–1918. https://doi.org/10.1007/s10531-017-1335-7
- Rosen, J., & Painter, G. (2019). From citizen control to co-production: Moving beyond a linear conception of citizen participation. Journal of the American Planning Association, 85(3), 335–347.
- Sanchez, T. W., Shumway, H., Gordner, T., & Lim, T. (2022). The prospects of artificial intelligence in urban planning. International Journal of Urban Sciences, 2, 179–194. https://doi.org/10.1080/12265934.2022.2102538
- Schläpfer, M., Dong, L., O’Keeffe, K., Santi, P., Szell, M., Salat, H., … West, G. B. (2021). The universal visitation law of human mobility. Nature, 593(7860), 522–527. https://doi.org/10.1038/s41586-021-03480-9
- Schölkopf, B., Locatello, F., Bauer, S., Ke, N. R., Kalchbrenner, N., Goyal, A., & Bengio, Y. (2021). Towards causal representation learning. ArXiv Preprint ArXiv:2102.11107.
- Sharma, A., Hamlet, A., Fernando, H., Catlett, C., Horton, D., Kotamarthi, V., … Wuebbles, D. (2018). The need for an integrated land-lake-atmosphere modeling system. Great Lakes Region. Earths Future, 6(10), 1366–1379. https://doi.org/10.1029/2018EF000870
- Shin, J., Rajabifard, A., Kalantari, M., & Atazadeh, B. (2021). Impact of ownership and architectural design on property disputes in multi-owned buildings. HABITAT International, 112, 102371. https://doi.org/10.1016/j.habitatint.2021.102371
- Shin, T., Yuan, Z., Siong, N., Zhang, Y., & Phang, V. (2017). Towards a deep learning powered query engine for urban planning. 2017 International Conference on Asian Language Processing (IALP) (WOS:000428370700024; pp. 99–102).
- Shuler, C., Brewington, L., & El-Kadi, A. (2021). A participatory approach to assessing groundwater recharge under future climate and land-cover scenarios. Journal of Hydrology-Regional Studies, 34. https://doi.org/10.1016/j.ejrh.2021.100785
- Sirbu, A., Becker, M., Caminiti, S., De Baets, B., Elen, B., Francis, L., … Van den Bossche, J. (2015). Participatory patterns in an international air quality monitoring initiative. PLOS ONE, 10(8). https://doi.org/10.1371/journal.pone.0136763
- Slack, D., Hilgard, S., Jia, E., Singh, S., & Lakkaraju, H. (2019). How can we fool LIME and SHAP? Adversarial attacks on post hoc explanation methods. Retrieved from http://arxiv.org/abs/1911.02508
- Stamatiadou, M., Thoidis, I., Vryzas, N., Vrysis, L., & Dimoulas, C. (2021). Semantic crowdsourcing of soundscapes heritage: A mojo model for data-driven storytelling. Sustainability, 13(5), 2714. https://doi.org/10.3390/su13052714
- Tian, Z., Zhong, R., Barenji, A., Wang, Y., Li, Z., & Rong, Y. (2021). A blockchain-based evaluation approach for customer delivery satisfaction in sustainable urban logistics. International Journal of Production Research, 59(7), 2229–2249.https://doi.org/10.1080/00207543.2020.1809733
- Tomašev, N., Cornebise, J., Hutter, F., Mohamed, S., Picciariello, A., Connelly, B., … Clopath, C. (2020). AI for social good: Unlocking the opportunity for positive impact. Nature Communications, 11(1), Article 1. https://doi.org/10.1038/s41467-020-15871-z
- United Nations. (n.d.). Goal 11: Make cities and human settlements inclusive, safe, resilient and sustainable. Sustainable Development Goals. Retrieved from https://sdgs.un.org/goals/goal11
- van den Homberg, M., Gevaert, C., & Georgiadou, Y. (2020). The changing face of accountability in humanitarianism: Using artificial intelligence for anticipatory action. Politics and Governance, 8(4), 456–467. https://doi.org/10.17645/pag.v8i4.3158
- Walisadeera, A., Ginige, A., & Wikramanayake, G. (2015). User centered ontology for Sri Lankan farmers. Ecological Informatics, 26, 140–150. https://doi.org/10.1016/j.ecoinf.2014.07.008
- Walter, A., & Scholz, R. (2007). Critical success conditions of collaborative methods: A comparative evaluation of transport planning projects. Transportation, 34(2), 195–212. https://doi.org/10.1007/s11116-006-9000-0
- Wang, Z., & Ye, X. (2018). Social media analytics for natural disaster management. International Journal of Geographical Information Science, 32(1), 49–72. https://doi.org/10.1080/13658816.2017.1367003
- Wiggins, L. L., & Ferreira, J. (1992). MIT’s computer resource lab. International Journal of Geographical Information Systems, 6(4), 299–307. https://doi.org/10.1080/02693799208901914
- Wilson, J. P., Butler, K., Gao, S., Hu, Y., Li, W., & Wright, D. J. (2021). A five-star guide for achieving replicability and reproducibility when working with GIS software and algorithms. Annals of the American Association of Geographers, 111(5), 1311–1317. https://doi.org/10.1080/24694452.2020.1806026
- Yang, Y., Bai, Y., Wang, X., Wang, L., Jin, X., & Sun, Q. (2020). Group decision-making support for sustainable governance of algal bloom in urban lakes. Sustainability, 12(4), 1494. https://doi.org/10.3390/su12041494
- Yasumoto, S., Jones, A., Yano, K., & Nakaya, T. (2012). Virtual city models for assessing environmental equity of access to sunlight: A case study of Kyoto, Japan. International Journal of Geographical Information Science, 26(1), 1–13.
- Ye, X., Du, J., & Ye, Y. (2021). MasterplanGAN: Facilitating the smart rendering of urban master plans via generative adversarial networks. Environment and Planning B: Urban Analytics and City Science. https://doi.org/10.1177/23998083211023516
- Ye, X., Wu, L., Lemke, M., Valera, P., & Sackey, J. (2022). Defining computational urban science. In Bin Li (Ed.), New thinking in GIScience (pp. 293–300). Singapore: Springer Nature Singapore.
- Yu, K., Zhang, Y., Li, D., Montenegro-Marin, C., & Kumar, P. (2021). Environmental planning based on reduce, reuse, recycle and recover using artificial intelligence. Environmental Impact Assessment Review, 86. https://doi.org/10.1016/j.ejar.2020.106492
- Yu, P., Williams, B., Fang, C., Cui, J., & Haslum, P. (2017). Resolving over-constrained temporal problems with uncertainty through conflict-directed relaxation. Journal of Artificial Intelligence Research, 60, 425–490 https://doi.org/10.1613/jair.5431.
- Zhang, F., Wu, L., Zhu, D., & Liu, Y. (2019). Social sensing from street-level imagery: A case study in learning spatio-temporal urban mobility patterns. ISPRS Journal of Photogrammetry and Remote Sensing, 153, 48–58.
- Zhang, Y., Grignard, A., Lyons, K., Aubuchon, A., & Larson, K. (2018). Real-time machine learning prediction of an agent-based model for urban decision-making. In Proceedings of the 17th International Conference on Autonomous Agents and Multiagent Systems (AAMAS’ 18) (WOS:000468231300357; p. 2173).
- Zhao, X., Ma, X., Tang, W., & Liu, D. (2019). An adaptive agent-based optimization model for spatial planning: A case study of Anyue County. China. Sustainable Cities and Society, 51.https://doi.org/10.1016/j.scs.2019.101733
- Zhu, D., Zhang, F., Wang, S., Wang, Y., Cheng, X., Huang, Z., & Liu, Y. (2020). Understanding place characteristics in geographic contexts through graph convolutional neural networks. Annals of the American Association of Geographers, 110(2), 408–420. https://doi.org/10.1080/24694452.2019.1694403
Appendix
Review method
The search query on the web of science:
(((ALL = (artificial intelligence) OR ALL = (machine learning) OR ALL = (AI) OR ALL = (deep learning)) AND ((ALL = (participatory) OR ALL = (collaborative) OR ALL = (group) OR ALL = (community) OR ALL = (public) OR ALL = (stakeholders) OR ALL = (involvement)) AND ((ALL = (spatial) OR ALL = (geographical) OR ALL = (geospatial) OR ALL = (location) OR ALL = (gis) OR ALL = (land) OR ALL = (site) OR ALL = (urban)) AND ((ALL = (planning) OR ALL = (decision making) OR ALL = (design)))))))
After thoroughly examining the full text of the remaining papers, we identified 87 relevant publications. To ensure comprehensiveness, we scrutinized the reference sections of these publications for additional studies related to our topic. This process led to the inclusion of 10 more conference papers from AI conferences, bringing the total number of reviewed papers to 97. The final dataset comprises review papers, opinion papers, and 45 empirical papers documenting specific use cases in the realm of AI-enabled participatory planning. Figure B presented our systematic review process.
Figure B. Systematic review workflow (after Page et al., Citation2021).
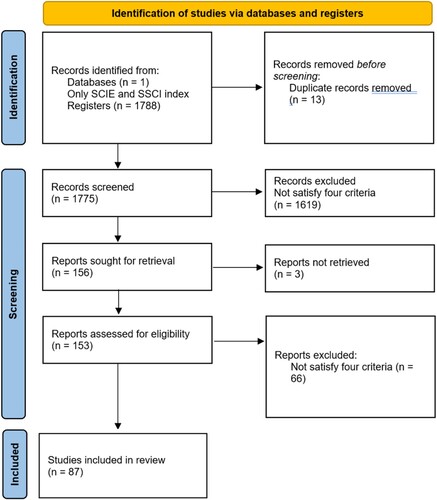
Full annotated bibliography table
Table A. Full annotated bibliography of case studies with both AI and participatory planning in chronological order.