Abstract
Data governance is an emerging subject in the information system (IS) field. In recent years, the volume of data used within organisations has increased dramatically, playing a critical role in business operations. This paper explores the current literature on data governance and is intended to provide a comprehensive analysis of the activities involved in data governance. Six major academic databases in the IS domain were searched using key terms to identify and analyse material reflecting the current state of knowledge. A systematic procedure was developed to identify 31 papers that explicitly mention data governance activities. Open coding techniques were applied to conduct content analysis, resulting in 110 data governance activities across five decision domains of the data governance framework. These data governance activities are understood as: ‘action’ plus ‘area of governance’ plus ‘decision domain’ e.g. (define data policies for data quality). Our analysis shows a high volume of data governance activities associated with the ‘defining’ action of the areas of governance across the decision domains with a lack of reported on the ‘implementing’ and ‘monitoring’ actions.
Introduction
In recent years, the volume of data used within organisations has increased dramatically, playing a critical role in businesses operations (Tallon, Ramirez, & Short, Citation2013). In particular, data influence both operational and strategic decisions. How to govern these data has also become critical, for data to be treated as a valuable asset (Khatri & Brown, Citation2010). Data governance has rapidly gained in popularity (Cheong & Chang, Citation2007; Khatri & Brown, Citation2010; Weber, Otto, & Österle, Citation2009) and is considered to be an emerging subject in the information system (IS) field (Hagmann, Citation2013). Practitioners also consider data governance as a promising approach for enterprises to improve and maintain the quality and use of their data (Otto, Citation2011a).
It can be argued that data governance, from both the academic and practitioner points of view, should be a universal approach to data accountability, fitting all data aspects and needs of an organisation (Weber et al., Citation2009; Wende, Citation2007). A survey of 200 organisations (Pierce, Dismute, & Yonke, Citation2008) found that 58% recognised data as a strategic asset. A recent study by Holt, Ramage, Kear, and Heap (Citation2015) indicated that 45% of their participants within the global community of database and data professionals did not have data governance policies in place. Hence, data governance requires more attention from stakeholders (Fisher, Citation2006).
This paper aims to contribute to the IS community through a categorization of current academic literature in the domain of data governance, which should assist researchers in understanding the research that has been published around the activities involved in data governance. These activities highlight the tasks that need to be performed in order to do data governance.
This paper is organised as follows: section 2 presents an overview of the data governance literature, section 3 describes the research approach used to conduct the literature review, including the paper selection strategy and the data analysis techniques applied; and section 4 presents the results of our analysis of data governance activities. We conclude by discussing the results and addressing the limitations and recommendations for future work in the area.
Data governance background
Data governance is defined as ‘A companywide framework for assigning decision-related rights and duties in order to be able to adequately handle data as a company asset’ (Otto, Citation2011b; p. 47). The main driver for data governance is considering data as an asset of the firm (Panian, Citation2010). Horne (Citation1995) connected governance with optimal uses of assets, then treated data and information as an asset, which drives the importance of the governance of the data within an organisation. The concept of data as an asset was developed in a report by the Hawley Committee in 1994, which defined data assets as ‘Data that is or should be documented and that has value or potential value’ (Oppenheim, Stenson, & Wilson, Citation2003).
It can be argued that ‘data governance’ is a new term with novel implications for data as an asset. However, there are many terms and approaches in the academic literature that deal with data and information under the IS field, such as total data quality management (TDQM) (see Wang, Citation1998). Another approach is data quality management (DQM) (see Wang & Strong, Citation1996), among many more different approaches and terms (Lucas, Citation2010; Otto, Wende, Schmidt, & Osl, Citation2007).
The main difference between the terms ‘governance’ and ‘management’ is that governance refers to the decisions that must be made and who makes these decisions in order to ensure effective management and use of resources, whereas management involves implementing decisions (Fu, Wojak, Neagu, Ridley, & Travis, Citation2011; Khatri & Brown, Citation2010). Hence, management is influenced by governance (Otto, Citation2011c). Therefore, we can distinguish between the activities for data governance and the activities required for data management.
The definition of data governance indicates who holds the decision rights and accountability regarding an enterprise’s data assets. Therefore, the decision domains should be identified in order to assign the right responsibilities and duties. In reviewing the literature relating to data governance frameworks, the framework proposed by Khatri and Brown (Citation2010) was selected to present the decision domains that should be considered for data governance. The framework contains five interrelated decision domains: 1) data principles, 2) data quality, 3) metadata, 4) data access, and 5) data life cycle as shown in Figure . These five decision domains follow a similar pattern to the IT governance decision domains to proposed by Weill and Ross (Citation2004). Each of the five decision domains addresses a set of core issues which are explained below.
Figure 1. Decision domains for data governance (Khatri & Brown, Citation2010).
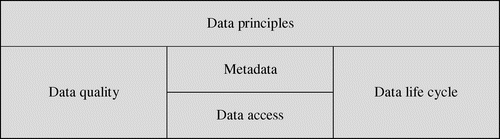
According to Khatri and Brown (Citation2010), data principles are shown at the top of the framework as they are intended to establish the direction for all other decision domains. Hence, the principles set the boundary requirements for uses of data assets, which addresses the enterprise’s standards for data quality. The data quality then refines the basis for how data are interpreted (metadata) as well as accessed (data access) by users. Finally, the data life-cycle decision defines the production, retention and retirement of data assets which plays a fundamental role in operationalizing the data principles into the IT infrastructure.
The focus of our literature review is to identify the data governance activities reported in the literature through the lens the of Khatri and Brown (Citation2010) framework.
Research approach
The purpose of this study is to identify and categorise the literature that explicitly mentions data governance activities. In order to conduct content analysis on a selection of papers, our research approach adopted steps proposed by Finney and Corbett (Citation2007). These steps consist of data collection and coding procedures and are explained below.
Step 1: Decide the level of analysis
This step involves deciding what level of analysis should be conducted. The level of analysis can be a signal word, a set of words, phrases, or an entire document (Finney & Corbett, Citation2007). For this research, the level of analysis considered the entire paper. The data collection phase was initiated by collecting papers through searches of the following databases: the AIS Electronic Library, CiteSeerX, EBSCO Online, Emerald Insight, ScienceDirect, and the ACM Digital Library. These 6 databases cover the majority of IS journals and conferences (Otto, Citation2011a). Search criteria were established in each database using the advanced search function. The keyword search criterion of having ‘data governance’ or ‘information governance’ in either the title or abstract was applied on the 16th December 2015, resulting in a total of 230 papers (Table ).
Table 1. Papers resulting from each database on the 16th December 2015.
The abstracts of these papers were reviewed; enabling the exclusion of those papers that are not related to the data governance domain (see Table ). It can be seen that 171 of the 230 papers were excluded as a result. The majority of the excluded papers were not peer-reviewed academic papers, but mainly practitioner papers.
Table 2. Initial excluded papers.
In addition, 66 papers were excluded, as they were not related to the data governance domain. They had been published to serve a different interest of study that was not related to data governance, although they mentioned data governance in the abstract. For example, the (Martin, Simons, Craven, & Betton, Citation2014) paper, where data governance is mentioned in the context of ‘there is a need for data governance in healthcare’, was not considered to be directly related to the data governance domain or focusing on the study of data governance activities.
Step 2: Decide how many concepts to code for
Here, researchers should decide whether to code text using a predefined set of concepts or develop a list of concepts incrementally during the process of coding (Finney & Corbett, Citation2007). For this research, due to the small number of papers that explicitly contribute to data governance activities, the researchers decided to code concepts inductively that can be interpreted as data governance activities. Therefore, all the concepts emerged incrementally through the processes of open coding.
Step 3: Decide whether to code for the existence or frequency of a concept
After a certain number of concepts have emerged, researchers should decide whether to code the concepts for existence or frequency (Finney & Corbett, Citation2007). If the concepts are coded for existence, this involves listing only the concepts that emerge. However, coding for frequency makes possible a discussion of saliency and emphasis (Finney & Corbett, Citation2007). For this research, it was decided to code for frequency in order to gain a deeper insight into the concepts that emerged.
Step 4: Decide on how you will distinguish between concepts
During this step, researchers should decide whether to code the concepts exactly as they appear, or if they can be coded in some altered or collapsed form (Finney & Corbett, Citation2007). For this research, it was decided to follow open coding analysis techniques suggested by Corbin and Strauss (Citation1990), whereby concepts that appear to be similar are grouped together under a higher-order, more abstract concept called a category.
Step 5: Develop rules for coding your text
It is necessary to define certain translation rules in order to ensure the consistency of the coding procedures (Finney & Corbett, Citation2007). The following translation rules were established and applied during the coding procedure:
• | All papers were read the first time in order to code data governance activities. There should be an imperative verb that indicates that an activity should be taken around data governance. | ||||
• | All the concepts that emerged from the papers were compared to identify similarities and differences in order for them to be labelled together in categories. | ||||
• | Once all the papers were coded, the researchers examined the categories that emerged and their properties within the actual text in order to ensure that they reflected the meaning of the actual text. |
Step 6: Decide what to do with ‘irrelevant’ information
This stage involved determining what to do with information in the text that was not coded (Finney & Corbett, Citation2007). Carley (Citation1993) suggested that deleting irrelevant information can facilitate content analysis procedures by generating simplified text. In this research, the remaining 59 papers received a more in-depth review in order to identify which of the papers explicitly mention data governance activities. Of the 59 papers, only 31 explicitly mention the required or recommended data governance activities. These activities are the conditions or things that need to be performed in order to be considered as doing data governance. In order to be coded as concepts, the sentence had to contain an action (imperative verb) such as, ‘define’, ‘establish’, ‘manage’ and ‘create’.
Step 7: Coding the text
The coding procedure should start following the decision related to irrelevant information and should follow the translation rules identified in step 5 (Finney & Corbett, Citation2007). As mentioned earlier, this research adopted an open coding analysis technique, which is part of a grounded theory approach (Corbin & Strauss, Citation1990). Open coding analysis is widely applied in conducting content analysis for a set of academic papers (see Finney & Corbett, Citation2007; Goode & Gregor, Citation2009; Grahlmann, Helms, Hilhorst, Brinkkemper, & van Amerongen, Citation2012) and is described as ‘the process of breaking down, examining, comparing, conceptualising, and categorising data’ (Corbin & Strauss, Citation1990, p. 61).
Open coding is a process that aims to identify the concepts or key ideas that may be hidden within data and are likely to be related to a phenomenon of interest (Bhattacherjee, Citation2012). Concepts and categories are generated in the open coding stage (Glaser, Citation1992) and, when the categories are developed, their properties and the dimensions of the properties are identified (Corbin & Strauss, Citation1990). Table shows the terms that are involved in open coding relevant to this study as defined by Corbin and Strauss (Citation1990). This is followed by a description of how open coding is operationalized.
Table 3. Definitions of the terms that are included in open coding (adapted from Corbin & Strauss, Citation1990, p. 61).
There are two analytical procedures that used to achieve the goal of open coding (Corbin & Strauss, Citation1990; Glaser,Citation1992): the first is the ‘making of comparisons’ and the second is the ‘asking of questions’. The ‘making of comparisons’ procedure concerns comparing each concept with the others for similarities and differences. Then, as Corbin and Strauss (Citation2008) argued, concepts that are found to be conceptually similar are grouped together under a higher-level descriptive category. However, each concept has the potential to bring out different aspects of the same phenomenon based on the proposed analytical procedures. This type of comparison allows the researcher to differentiate categories from each other and identify the properties and dimensions of each category (Corbin & Strauss, Citation2008; Glaser, Citation1992).
The second procedure for open coding involves asking questions, and refers to analysis of the emerged concepts that are conducted to establish what category, or property of a category, a concept describes (Glaser, Citation1992). This step requires close examination of the data through line-by-line coding, which forces the analyst to verify and saturate categories, thus minimising the possibility of missing an important category (Glaser & Holton, Citation2004). Application of these two procedures allows the analyst to conceptualise the data and categorise them according to the specific needs of the intended investigation.
Step 8: Analysing the results
After coding the data, researchers should decide how to review and present the results (Finney & Corbett, Citation2007). For this research, a frequency count was the principal method for representing the data governance activities.
Data governance activities analysis
A total of 59 articles were reviewed, 31 of which explicitly mention data governance activities. The 31 papers were classified by paper publication type, either a journal paper or conference proceedings papers, and are listed in Table .
Table 4. List of the papers selected.
While reviewing and applying the open coding analysis procedure to the 31 selected papers, an MS Excel spreadsheet was developed. The spreadsheet was constructed to include reference to each open coding stage, including referencing the original text using Mendeley, a document management application.
The open coding analysis procedure was conducted in an iterative manner (as described in step 7), starting with reading each paper and searching for any action (imperative verbs) (see step 6). These concepts were then compared for similarities and differences in order for them to be categorised in higher abstracted categories which are considered to be data governance activities. In maintaining the meaning of the categories, it was decided that each of the categories would consist of the following three constructs: 1) action, 2) area of governance, and 3) the decision domain. Table shows the terms used in the coding procedure associated with the number of results counted after reviewing the 31 papers. This is followed by an explanation of the three constructs.
Table 5. Terms included in coding procedures and the total number of results.
Reviewing the 264 concepts, three ‘actions’ against the ‘areas of data governance’ emerged. These ‘actions’ indicated the doing of data governance, and were named as follows: 1) define, 2) implement, and 3) monitor. The researchers found that all the imperative verbs in the concepts could be turned into one of these three actions. For example, according to Cheong and Chang (Citation2007, p. 1007), ‘The first step to setting up a formal data governance programme is to determine a Data Governance structure’, in which the verb ‘determine’ can be interpreted as ‘define’. Another example comes from Weber et al. (Citation2009, p. 4:2): ‘It establishes organisation wide guidelines and standards’, in which the verb ‘establish’ can be deemed to mean ‘define’. However, an interpretation of these actions relies upon the context itself. Therefore, each imperative verb could be interpreted as one of the three actions in one case and to another action in others, such as the verb ‘develop’ in some contexts means to ‘define’ and in others can mean ‘implement’. For example, in an excerpt from Weber et al. (Citation2009, p. 4:6), ‘data governance develops and implements corporate-wide data policies’, the verb ‘develop’ means to ‘define’. In contrast, in an excerpt from Panian (Citation2010, p. 943), ‘to establish data definitions and taxonomies, define master data, develop enterprise data models’, the verb ‘develop’ can be interpreted as ‘implement’, as it is related to implementing a data model.
Eight ‘areas of governance’ emerged during the comparison procedure for the concepts: 1) data roles and responsibilities, 2) data policies, 3) data processes and procedures, 4) data standards, 5) data strategy, 6) data technologies, 7) data guidelines, and 8) data requirements. Each of the 264 concepts could be placed into one of these areas of governance.
The third construct is the ‘decision domain’. The analysis found that honouring the five decision domains defined by Khatri and Brown (Citation2010) gave in-depth insights into the actual focus of the activity. However, some of the 264 concepts were reported to cover more than one decision domain, and in some instances all the five decision domains. For example, a concept labelled as ‘define data policies’ without any specified domain was considered to cover all five domains.
The illustrative example below shows how the concepts were placed into a category which was considered to be a data governance activity that consisted of the three constructs. Wende (Citation2007, p. 417) stated that ‘data governance defines roles, and it assigns responsibilities for decision areas to these roles. It establishes organisation- wide guidelines and standards for DQM’. Through coding this excerpt, four concepts emerged, which were placed into categories of data governance activities. Table illustrates the four concepts and the breakdown of the constructs.
Table 6. The concepts that emerged and their categories.
During the comparison procedure, using the schema outlined in Table , the 264 concepts were reduced to 110 data governance activities as categories.
Table illustrates the results of the open coding analysis, including the frequency of the ‘areas of data governance’ under each respective ‘action’ across the five ‘decision domains’ that emerged during the open coding process. In the event that a papers mentioned an activity more than once, the frequency was noted as one, unless that same area was mentioned with different actions or associated with another decision domain.
Table 7. Frequency analysis of the data governance activities mentioned in the selected papers.
Discussion
Our analysis shows that the highest frequency count for the ‘areas of data governance’ is against the ‘define’ action, specifically, definitions of the data roles and responsibilities, data policies, data processes and procedures, and data standards. The definition of the data quality decision domain is also the highest frequency count. This is not surprising as data governance is often cited as the key aspect of data governance. In contrast, ‘metadata’ has the lowest frequency count which suggests that a ‘how data is interpreted’ mindset has not received as much coverage in the academic literature to date.
Our analysis also points to a low frequency for ‘implement’ and ‘monitor’ actions this could indicate a lack of maturity around data governance in practice which penetrates through to our research activities to date, where the majority of the papers are focusing only on the ‘define’ action of the data governance areas. Although a ‘define’ action can be considered as a primary action of data governance areas, ‘implement’ and ‘monitor’ should also play an important role in data governance. For example, in terms of ‘data processes and procedures’ activities, a small number of papers explicitly mention implementing and monitoring data processes and procedures, however embedding and controlling data governance processes and procedures in any system should be considered as fundamental to a data governance programme in order to make sure these processes and procedures are followed.
In addition, defining data technologies as an activity for doing data governance has received an acceptable level of attention specifically for the data life cycle decision domain. However, none of the papers considers monitoring data technologies, which, from our point of view, is a shortcoming in the research, as it is critical to monitor the activities related to data technologies in order to manage a data governance programme successfully.
Finally, defining, implementing, and monitoring data roles and responsibilities across all the decision domain as a data governance activity has received high level of attention. Returning to the definition of data governance, this is about assigning decision-related rights and duties for handling the data in an organisation. Therefore, out analysis supports that actions related to data roles and responsibilities for all the decision domains should be viewed as the primary activity when considering doing data governance.
Conclusion and future work
Research in the data governance domain is growing in IS, as is the need for research in this area as more organisations consider data as a valuable asset. A review of the data governance literature shows that there is a lack of research that explicitly studies activities for governing data. Nevertheless, there is some research that contributes to our understanding of data governance through modelling (see Khatri & Brown, Citation2010; Otto, Citation2011b; Tallon et al., Citation2013). These studies reveal some progress in exploring the activities that are required for governing data.
This research study identified and analysed 31 peer-reviewed papers that focus on data governance activities. Using a systematic approach through eight steps adopted from Finney and Corbett (Citation2007), the selection process yielded 230 papers that were subject to selection and exclusion criteria, which led to the exclusion of 171. Following a more in-depth review of the remaining 59 papers, 31 were found to serve the research purpose explicitly. These 31 papers were analysed using an open coding analysis technique suggested by Corbin and Strauss (Citation1990). This technique was selected to conduct an in-depth content analysis of the data governance activities mentioned in these papers.
This research has two key limitations. Firstly, the focus of the research was only on peer-reviewed academic literature in the data governance area, ignoring practitioners’ publications specifically. Institutions such as DAMA and The Data Warehousing Institute (TDWI) may provide further insight into data governance programmes from a practitioner perspective, and future research may highlight differences in theory and practice (DAMA International, Citation2009). Secondly, the research presented in this paper concluded with a frequency count of data governance activities. There was no detailed description for each of the 110 activities identified in this paper due to page length limitations.
Finally, as recommendations for further research in the area of data governance activities, the above resulting activities should be validated by conducting field studies, as well as being described in greater detail, in order to be more valuable for researchers as well as practitioners. The results also, as discussed earlier, reflect the lack of research in the ‘implement’ and ‘monitor’ actions of the different data governance areas. Therefore, is it suggested that researchers in the domain of data governance focus more on the data governance activities related to implementing and monitoring over defining.
Disclosure statement
No potential conflict of interest was reported by the authors.
References
- Becker, M. Y. (2007). Information governance in NHS’s NPfIT: A case for policy specification. International Journal of Medical Informatics, 76, 432–437. doi: http://0-dx.doi.org.library.ucc.ie/10.1016/j.ijmedinf.2006.09.00810.1016/j.ijmedinf.2006.09.008
- Bhattacherjee, A. (2012). Social science research: Principles, methods, and practices. (2nd ed.). Textbooks Collection. Athens, GA: Global Text Project. Retrieved from http://scholarcommons.usf.edu/oa_textbooks/3
- Carley, K. (1993). Coding choices for textual analysis: A comparison of content analysis and map analysis. Sociological Methodology, 23, 75–126. doi:10.2307/271007
- Cheong, L. K., & Chang, V. (2007). The need for data governance: A case study. In, Proceedings of the 18th Australasian Conference on Information Systems, Toowoomba (Australia), 2007- 12-06, Pp. 999-1008
- Corbin, J. M., & Strauss, A. (1990). Grounded theory research: Procedures, canons, and evaluative criteria. Qualitative Sociology, 13, 3–21. 10.1007/BF00988593
- Corbin, J., & Strauss, A. (2008). Basics of qualitative research. (3rd ed.). Thousand Oaks, CA: Sage Publications, Inc.
- DAMA. (2009). The DAMA guide to the data management body of knowledge, Bradley Beach: Technics Publications.
- Donaldson, A., & Walker, P. (2004). Information governance – a view from the NHS. International Journal of Medical Informatics, 73, 281–284. doi:10.1016/j.ijmedinf.2003.11.009
- Finney, S., & Corbett, M. (2007). ERP implementation: a compilation and analysis of critical success factors. Business Process Management Journal, 13, 329–347. doi:http://0-dx.doi.org.library.ucc.ie/10.1108/1463715071075227210.1108/14637150710752272
- Fisher, T. (2006). Fourth Quarter 2006). Data monitoring: Add controls to your data governance and compliance programs. Business Intelligence Journal, 11, 51–57.
- Fu, X., Wojak, A., Neagu, D., Ridley, M., & Travis, K. (2011). Data governance in predictive toxicology: A review. Journal of Cheminformatics, 3(1), 1–16. doi:10.1186/1758-2946-3-24
- Gillies, A., & Howard, J. (2005). An international comparison of information in adverse events. International Journal of Health Care Quality Assurance, 18, 343–352. 10.1108/09526860510612199
- Glaser, B. G. (1992). Emergence vs forcing: Basics of grounded theory analysis. Mill Valley, CA: Sociology Press.
- Glaser, B. G., & Holton, J. (2004). Remodeling grounded theory. Forum Qualitative Sozialforschung/Forum: Qualitative Social Research, 5(2), Art. 4. Retrieved from http://nbn-resolving.de/urn:nbn:de:0114-fqs040245
- Goode, S., & Gregor, S. (2009). Rethinking organisational size in IS research: Meaning, measurement and redevelopment. European Journal of Information Systems, 18, 4–25. 10.1057/ejis.2009.2
- Grahlmann, K. R., Helms, R. W., Hilhorst, C., Brinkkemper, S., & van Amerongen, S. (2012). Reviewing enterprise content management: A functional framework. European Journal of Information Systems, 21, 268–286. 10.1057/ejis.2011.41
- Guetat, S. B. A., & Dakhli, S. B. D. (2015). The architecture facet of information governance: The case of urbanized information systems. Procedia Computer Science, 64, 1088–1098. doi: http://0-dx.doi.org.library.ucc.ie/10.1016/j.procs.2015.08.56410.1016/j.procs.2015.08.564
- Hagmann, J. (2013). Information governance – Beyond the buzznull. Records Management Journal, 23, 228–240. doi:10.1108/RMJ-04-2013-0008
- Holt, V., Ramage, M., Kear, K., & Heap, N. (2015). The usage of best practices and procedures in the database community. Information Systems, 49, 163–181. 10.1016/j.is.2014.12.004
- Horne, N. W. (1995). Information as an asset – The board agenda. Computer Audit Update, 1995, 5–11. doi:http://0-dx.doi.org.library.ucc.ie/10.1016/0960-2593(95)90246-510.1016/0960-2593(95)90246-5
- Kersten, S. (2013). Moving the needle toward a data-driven health care system: Optimizing the EHR through information governance. Journal of Health Care Compliance, 15, 45–62.
- Khatri, V., & Brown, C. V. (2010). Designing data governance. Communications of the ACM, 53, 148–152. doi:10.1145/1629175.1629210
- Kooper, M. N., Maes, R., & Lindgreen, E. E. O. R. (2011). On the governance of information: Introducing a new concept of governance to support the management of information. International Journal of Information Management, 31, 195–200. doi:10.1016/j.ijinfomgt.2010.05.009
- Lajara, T. T., & Maçada, A. C. G. (2013). Information governance framework: The defense manufacturing case study. Paper presented at Proceedings of the Nineteenth Americas Conference on Information Systems, 21 Chicago, Illinois. Retrieved from http://aisel.aisnet.org/cgi/viewcontent.cgi?article=1704&context=amcis2013
- Larkin, B. S. (2008). Increasing information integrity: Cultural impacts of changing the way we manage data. International Journal of Organization Theory & Behavior (PrAcademics Press), 11, 558–578.
- Lomas, E. (2010). Information governance: information security and access within a UK context. Records Management Journal, 20, 182–198. 10.1108/09565691011064322
- Lucas, A. (2010, June 16–19). Corporate data quality management: From theory to practice. Paper presented at 5th Iberian Conference on Information Systems and Technologies (CISTI), Santiago de Compostela, Spain. Retrieved from http://hdl.handle.net/10400.5/2089
- Martin, J. L., Simons L., Craven M. P., & Betton V. (2014). Towards a framework for the appraisal of digital products for mental health [Abstract]. International Journal of Integrated Care, 14(8), 1–2. Retrieved from http://doi.org/10.5334/ijic.1814
- Oppenheim, C., Stenson, J., & Wilson, R. M. S. (2003). Studies on information as an Asset I: definitions. Journal of Information Science, 29, 159–166. doi:10.1177/01655515030293003
- Otto, B. (2011a). A morphology of the organisation of data governance.
- Otto, B. (2011b). Organizing data governance: Findings from the telecommunications industry and consequences for large service providers. Communications of the AIS, 29, 45–66.
- Otto, B. (2011c). Organizing data governance: Findings from the telecommunications industry and consequences for large service providers. Communications of the Association for Information Systems, 29(3), 45–66. Retrieved from http://aisel.aisnet.org/cais/vol29/iss1/3
- Otto, B. (2012). Managing the business benefits of product data management: The case of Festo. Journal of Enterprise Information Management, 25(3), 272–297. Retrieved from http://dx.doi.org/10.1108/17410391211224426
- Otto, B., Wende K., Schmidt A., & Osl P. (2007). Towards a framework for corporate data quality management. 18th Australasian Conference on Information Systems. the University of Southern Queensland, Toowoomba, Australia, 109, 916–926.
- Palczewska, A., Fu, X., Trundle, P., Yang, L., Neagu, D., Ridley, M., & Travis, K. (2013). Towards model governance in predictive toxicology. International Journal of Information Management, 33, 567–582. doi:10.1016/j.ijinfomgt.2013.02.005
- Panian, Z. (2010). Some practical experiences in data governance. World Academy of Science, Engineering and Technology Management, 62, 939–946.
- Pierce, E., Dismute W., & Yonke C. (2008). Industry report: The state of information and data governance: Understanding how organisations govern their information and data assets. Baltimore, MD: International Association for Information and Data Quality.
- Rickards, R. C. & Ritsert, R. (2012). Data governance challenges facing controllers. International Journal of Business, Accounting, & Finance, 6, 25–42.
- Rifaie, M., Alhajj R., & Ridley M. (2009). Data governance strategy: A key issue in building enterprise data warehouse. Paper presented at the Eleventh International Conference on Information Integration and Web-Based Applications and Services, Kuala Lumpur, Malaysia. doi:10.1145/1806338.1806449
- Rosenbaum, S. (2010). Data governance and stewardship: Designing data stewardship entities and advancing data access. Health Services Research, 45, 1442–1455. 10.1111/hesr.2010.45.issue-5p2
- Shaw-Taylor, Y. (2014). Making quality improvement programs more effective. International J Health Care QA, 27, 264–270. doi:10.1108/IJHCQA-02-2013-0017
- Silic, M., & Back, A. (2013). Factors impacting information governance in the mobile device dual-use contextnull. Records Management Journal, 23, 73–89. doi:10.1108/RMJ-11-2012-0033
- Tallon, P. P., Ramirez, R. V., & Short, J. E. (2013). The information artifact in IT governance: Toward a theory of information governance. Journal of Management Information Systems, 30, 141–178. doi:10.2753/MIS0742-1222300306
- Tallon, P. P., Short, J. E., & Harkins, M. W. (2013). The evolution of information governance at intel. MIS Quarterly Executive, 12, 189–198.
- Vayghan, J. A., Garfinkle, S. M., Walenta, C., Healy, D. C., & Valentin, Z. (2007). The internal information transformation of IBM. IBM Systems Journal, 46, 669–683. doi:10.1147/sj.464.0669
- Wang, R. Y. (1998). A product perspective on total data quality management. Communications of the ACM, 41(2), 58–65.
- Wang, R. W., & Strong, D. M. (1996). Beyond accuracy: What data quality means to data consumers. Journal of Management Information Systems, 12(4), 5–33.
- Watson, H. J., Fuller, C., & Ariyachandra, T. (2004). Data warehouse governance: Best practices at blue cross and blue shield of North Carolina. Decision Support Systems, 38, 435–450. doi:10.1016/j.dss.2003.06.001
- Weber, K., Otto, B., & Österle, H. (2009). One size does not fit all–A contingency approach to data governance. Journal of Data and Information Quality (JDIQ), 1(1), 1–27.
- Weill, P., & Ross, J. W. (2004). IT governance: How top performers manage IT decision rights for superior results. Boston, MA: Harvard Business School Publishing.
- Weller, A. (2008). Data governance: Supporting datacentric risk management. Journal of Securities Operations & Custody, 1, 250–262.
- Wende, K. (2007). A model for data governance -Organising accountabilities for data quality management. Paper presented at the 18th Australasian Conference on Information Systems, Toowoomba, Australia. Retrieved from http://aisel.aisnet.org/cgi/viewcontent.cgi?article=1079&context=acis2007
- Wende, K., & Otto, B. (2007). A contingency approach to data governance. Iciq, 163–176.