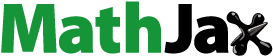
ABSTRACT
There is empirical evidence that decision makers show negative behaviours towards algorithmic advice compared to human advice, termed as algorithm aversion. Taking a trust theoretical perspective, this study broadens the quite monolithic view on behaviour to its cognitive antecedent: cognitive trust, i.e. trusting beliefs and trusting intentions. We examine initial trust (cognitive trust and behaviour) as well as its development after performance feedback by conducting an online experiment that asked participants to forecast the expected demand for a product. Advice accuracy was manipulated by ± 5 % relative to the participant’s initial forecasting accuracy determined in a pre-test. Results show that initial behaviour towards algorithmic advice is not influenced by cognitive trust. Furthermore, the decision maker’s initial forecasting accuracy indicates a threshold between near-perfect and bad advice. When advice accuracy is at this threshold, we observe behavioural algorithm appreciation, particularly due to higher trusting integrity beliefs in algorithmic advice.
1 Introduction
To derive advantages from the huge amount of information available in a digitalised world, algorithmsFootnote1 are employed in many task domains. Yet, algorithms provide advice in less a-priori determinable judgemental tasks such as in demand forecasting (Fildes et al., Citation2009), which was previously reserved exclusively for human judgement.
Algorithms have commonly been used in automation tasks that are standardised and a-priori well determinable. The output of an aid can easily be identified as right or wrong at the time of decision (Ord et al., Citation2017). Even without providing immediate performance feedback, the human operator gets a good impression of whether the aid is trustworthy or not (e.g. Dijkstra, Citation1999; Madhavan & Wiegmann, Citation2007). Bad advice can then easily be detected, resulting in trust adjustments towards the trustworthiness of the automation aid (Goodyear et al., Citation2016; Yu et al., Citation2019).
Empirical research indicates a perfect automation schema (PAS). It is an explanatory approach for two seemingly contradictory findings: the human operator exposes higher trust levels in the automated aid compared to the human aid, however, he/she is more likely to disuse the automated aid (Dzindolet et al., Citation2002). The PAS suggests that people have preconceived notions of perfect or near-perfect automation, leading to high initial trust levels (Dzindolet et al., Citation2003). In contrast, human aids are expected to be imperfect as erring is a human trait (Renier et al., Citation2021). Consequently, people expose higher trusting expectations towards the automation relative to a human aid. Witnessing bad advice leads thus to higher violations of trusting expectations in the automation. In addition, algorithmic errors are associated with a fundamental flaw, violating their consistency assumption. Humans are believed to learn from errors and thus their consistency assumption is not violated.
However, forecasts are by their nature uncertain in their future state. They are less a-priori determinable, errors less visible and performance feedback may not be readily available due to a time delay until the true value materialised. This makes perfection nearly impossible and algorithmic advice permanently runs the risk of violating the PAS. In such a task domain, the decision maker struggles to determine whether bad advice from an algorithm indicates a fundamental flaw or is due to a random error that was unavoidable.
There is empirical research that points to negative beliefs, intentions and behaviours towards the algorithm compared to a human, termed as algorithm aversion (for an overview see, Jussupow et al., Citation2020). In forecasting research, the behavioural trust measure weight on advice (WOA) is used to measure algorithm aversion (Logg et al., Citation2019; Önkal et al., Citation2009; Prahl & Van Swol, Citation2017). WOA origins in the advice research (Bonaccio & Dalal, Citation2006) and gives insights on how much the initial own judgement is updated by the exposure to external advice. This study therefore takes a trust theoretical perspective on algorithm aversion and refers to WOA as a synonym for behavioural trust. Linking WOA to cognitive trust seems natural because updating one’s own judgement due to an advice in a judge-advisor system (JAS) expresses the willingness to become vulnerable to the advisor, a prerequisite of cognitive trust. Prior research showed the important influence of cognitive trust on the decision to use a technology (Komiak & Benbasat, Citation2006; Li et al., Citation2008; Schmidt et al., Citation2020). We refer to trust as a generic term for cognitive and behavioural trust (WOA).
Experiments gained momentum, particularly in exploratory research designs (Obermaier & Müller, Citation2008).We therefore experimentally examine a potential threshold between near-perfect and bad advice. The latter is expected to violate PAS, leading to trust decreases. Yet, the threshold between near-perfect and bad advice is not examined in forecasting tasks. Based on a pre-test, we provide advice accuracy that is higher, equal or lower than the decision maker’s initial forecasting accuracy. Furthermore, we examine whether trust differences between an algorithm and a human already exist before performance feedback (initial trust). In addition, we broaden the analysis of WOA to its cognitive antecedents: trusting beliefs and trusting intentions. Doing so allows us to get a better understanding of the role of trust towards an advisor. Summarised, we answer the following research questions: (1) How does cognitive trust influence WOA in the source of advice? (2) Are there differences in initial trust between human and algorithmic advisors? (3) Are there differences in the development of trust after performance feedback? (4) When does an external advice lead to trust decreases?
This paper applies a fictitious context of the forecast of the demand of a product in two different countries. An auto-ARIMA model provided advice accuracy that is higher than the decision maker’s initial forecasting accuracy. The advice was merely labelled as coming from an algorithm versus from a human colleague in the same firm. We used the average forecasting accuracy based on a pre-test (n = 145) for advice accuracy that equals the decision maker’s initial forecasting accuracy. We manipulated this advice to generate advice accuracy that is worse than the decision maker’s initial forecasting accuracy.
Results of an online experiment at a public university in Germany show that there are no cognitive trust differences between a colleague and an algorithm. However, we observe behavioural algorithm appreciation when the accuracy of the advice equals the decision maker’s initial forecasting accuracy. One reason for this seem to be lower trusting integrity beliefs in the human after performance feedback. Thereby, we extend the findings from Prahl and Van Swol (Citation2017) and find the decision maker’s initial forecasting accuracy is a potential threshold between near-perfect and bad advice. Further, briefly framing that an advice comes from an algorithm results in behaviour that is not determined by cognitive trust before interaction. This contradicts the suggestion derived from our trust model.
We make three contributions: First, we apply a trust theoretic perspective and extend the scope of analysis within the algorithm aversion literature to a more holistic perspective on cognitive trust and WOA within a JAS. Second, we identify a threshold between near-perfect and bad advice in an inherently uncertain forecasting task. Third, based on the decision maker’s initial forecasting accuracy, we examine the influence of advice accuracy on trust development and observe algorithm appreciation when advice accuracy equals the decision maker’s initial forecasting accuracy.
2 Hypotheses development
2.1 Cognitive trust model
We define cognitive trust as a belief and intention towards the decisions of another party in a decision under risk where the decision maker is aware of the risk involved and is willing to take it. Within a JAS, risk is a relevant parameter because there is uncertainty about the advice accuracy and bad advice could be harmful. The higher the level of trust, the more risk the decision maker is willing to take in the relationship (Mayer et al., Citation1995). There are many different ways to measure cognitive trust with regard to the human-machine interaction (e.g. Jian et al., Citation2000; Madsen & Gregor, Citation2000; Xie et al., Citation2022). For operationalisation, however, we adapt the trust model commonly applied in the e-commerce trust literature due to three advantages. First, it deals with computer advisors that are closest to an algorithmic advisor. Second, empirically approved measures of trust can readily be adapted to a more technology-like JAS (Lankton et al., Citation2014). Third, based on the Theory of Reasoned Action, this model allows us to further split the antecedent cognitive trust into trusting beliefs and trusting intentions as important determinants of behaviour (Fishbein & Ajzen, Citation1977). Examining both preserves the dual meaning of cognitive trust (Rousseau et al., Citation1998). Trusting beliefs are important antecedents of trusting intentions (Lee & Turban, Citation2001) and in turn of WOA (Pavlou & Gefen, Citation2004).
Trusting beliefs are the extent to which one feels confident in believing that the advisor is trustworthy in a particular task (see, McKnight & Chervany, Citation2001). This term subsumes three components: trusting competence, benevolence, and integrity beliefs. Trusting competence beliefs mean that the advisor has the ability to perform the task and measure the perception of how knowledgeable the advisor was. Trusting benevolence beliefs express care about the welfare that motivates the advisor to act in the other person’s interest. They focus on characteristics such as being genuinely concerned or acting without opportunism. Trusting integrity beliefs capture the perception of honesty, truthfulness, sincerity, and keeping commitments. Trusting intentions refer to the willingness to depend on the other party in a specific situation (McKnight et al., Citation1998). Together they constitute cognitive trust (see, ). There is large evidence that cognitive trust is an important determinant of behaviour, i.e. of WOA (Komiak & Benbasat, Citation2006; Li et al., Citation2008).
Figure 1. Cognitive trust model and its influence on behaviour (adapted from McKnight et al., Citation1998).
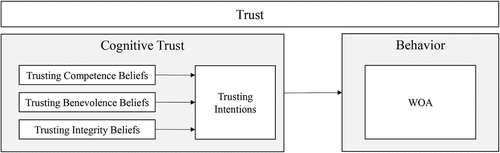
This model is consistent with the definition of algorithm aversion as negative beliefs, intentions and behaviours. For the purpose of this study, we distinguish between cognitive and behavioural algorithm aversion or appreciation, respectively.
There is evidence in the domain of e-commerce that trusting benevolence beliefs may be the main driver for trust towards the algorithmic advisor, while it has a major influence on developing distrust towards a human advisor (Komiak et al., Citation2005). Trusting benevolence beliefs, however, are omitted in the algorithm aversion literature so far (Prahl & Van Swol, Citation2017).
2.2 Initial trust before interaction
The beginning of any relationship is the most critical time frame to develop trust (McKnight et al., Citation1998) and therefore the understanding of how initial trust towards technology is formed is central to promoting the implementation and adoption of novel technologies (Gefen & Straub, Citation2003; Li et al., Citation2008; Siau & Wang, Citation2018). When the decision maker has no first-hand experience, initial trust serves as a mechanism to overcome perceptions of risk and uncertainty (Komiak & Benbasat, Citation2006; Mayer et al., Citation1995; Pavlou & Gefen, Citation2004). Still little is known about how initial cognitive trust affects WOA in a JAS. Therefore, we hypothesise the following links:
H1a: Initial cognitive trust towards the human advisor positively influences initial weight on human advice.
H1b: Initial cognitive trust towards the algorithmic advisor positively influences initial weight on algorithmic advice.
The PAS suggests even higher expectations in the algorithm than in a human. Before interaction, however, the level of trust towards a human and an algorithm might not differ. Decision makers share a relatively longer history with human advisors than with algorithms (Ring, Citation1996) and may believe in the good in people. Further, humans may implicitly believe that a perfect or near-perfect forecast is theoretically possible when the underlying processes can be known by greater knowledge at the time of decision (Einhorn, Citation1986; Highhouse, Citation2008), e.g. the ‘broken leg cues’ (Meehl, Citation1954). This is particularly true before interaction as there is no objective measure that indicates advice accuracy. We hypothesise:
H2a: Initial cognitive trust towards the algorithmic advisor is equal to initial cognitive trust towards the human advisor.
H2b: Initial weight on algorithmic advice is equal to initial weight on human advice.
There is little and inconclusive empirical research on initial trust towards an algorithm versus a human. Castelo et al. (Citation2019) asked participants, how much they would trust an algorithm or a ‘very well qualified person’ in a separate (Study 1) and a joint evaluation (Study 4). Results underscore the importance of the task domain and indicated relatively higher trust towards the algorithm before interaction only for more objective tasks where the customers were more familiar with the use of algorithms (e.g. weather or stock price forecasts). Also, Longoni et al. (Citation2019) queried the extent to which participants would follow the recommendation of the human (robotic) dermatologist, an item similar to initial trusting intentions. They found that algorithm aversion is already prevalent before interaction when offered a medical service operated by a computer, as indicated by differences in initial trusting intentions.
2.3 Dynamic trust after performance feedback
Trust develops after the decision maker gains first-hand experience with the advisor (Lewicki & Bunker, Citation1995) as trust levels result from an expectation-disconfirmation process (Lewicki et al., Citation2006). Thus, measuring trust levels at a single point in time is little informative. Hoff and Bashir (Citation2015) model the dynamic character of trust (see, ). Yet, little is known about trust development during the interaction as algorithm aversion is often measured as a one-time decision (e.g. Dietvorst et al., Citation2015, Citation2018).
Figure 2. Dynamic trust model (adapted from Hoff & Bashir, Citation2015).
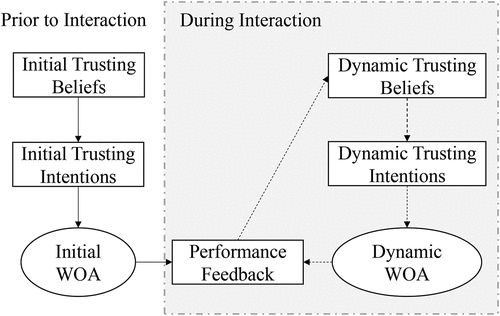
Link between cognitive trust and WOA (H3)
Analogously, we expect that dynamic cognitive trust is a good predictor for dynamic WOA. We hypothesise:
H3a: Dynamic cognitive trust towards the human advisor positively influences dynamic weight on human advice.
H3b: Dynamic cognitive trust towards the algorithmic advisor positively influences dynamic weight on algorithmic advice.
Advice accuracy and development of cognitive trust (H4) and WOA (H5)
After seeing the algorithm perform, the PAS suggests that only near-perfect advice quality maintains the human’s belief of perfection of the algorithm. This human belief implies that errors of automated aids indicate a fundamental flaw that will reoccur as erring is not an algorithmic trait (Renier et al., Citation2021). As a consequence, expectations in algorithmic advice are relatively even higher than in a human advisor. Erring as a human trait is accepted and limits the expectation of perfection. Humans are even believed to learn from experience and thus show a more consistent behaviour in the long run (Dijkstra, Citation1999). Based on the expectation-disconfirmation theory (Bhattacherjee and Premkumar Citation2004), expectations towards the algorithm are more violated than towards a human, even though advice accuracy is identical. Empirical evidence comes from Prahl and Van Swol (Citation2017) who documented algorithm aversion after providing bad advice. Bigman and Gray (Citation2018) documented that even when advice accuracy is identical for both advisors and known to the decision maker, they still prefer the human advisor. Similar findings come from Dietvorst et al. (Citation2015). We therefore expect that the development of trust is contingent on advice accuracy. This study focuses on the threshold between bad advice and near-perfect advice that is still vague.
Trust in automation literature points to a potential threshold of 70 % accuracy. Accuracy levels equal to or lower than this threshold seems to violate the PAS and are not perceived as near-perfect by the decision maker anymore (Madhavan & Wiegmann, Citation2007; Yu et al., Citation2019). Research on the PAS is primarily dealing with low levels of automation (e.g. a detection aid) that execute a-priori well determinable tasks (Byström & Järvelin, Citation1995). That is, an error in operation can be observed immediately at the timepoint of deciding to trust an automation.
However, this threshold in automation tasks is not transferable to a less a-priori determinable forecasting task where judgement is always subject to random errors (Dietvorst & Bharti, Citation2020). Outcomes in forecasting tasks are by their nature irreducible uncertain and can objectively be evaluated ex-post after a time delay until the true value materialised (Ord et al., Citation2017). Consequently, the human as well as the algorithm will not make perfect forecasts.
According to the expectation-disconfirmation theory, we expect that advice accuracy of 5.41% meets the expectation towards both advisors as a decision maker can improve his/her initial forecasting accuracy. However, advice accuracy of 10.70%, e.g. that is on average equal to the decision maker’s initial forecasting accuracy, does not meet the expectation of improvement, even more violating the relatively higher expectations towards the algorithmic advice. We argue that the initial forecasting accuracy of a decision maker is a potential threshold between near-perfect and bad advice, resulting in algorithm aversion. Finally, advice accuracy of 15.10% is on average much worse than the decision maker’s initial forecasting accuracy. Therefore, we expect trust decreases towards both advisors. We hypothesise that higher expectations towards the algorithm will lead to a sharper decrease in trust, leading to algorithm aversion (see, ).
H4a: After receiving advice whose accuracy is higher than the decision maker’s initial forecasting accuracy, cognitive trust towards the external advisor does not decrease, independent from the source of advice.
H4b: Receiving advice whose accuracy equals the decision maker’s initial forecasting accuracy leads to cognitive algorithm aversion.
H4c: After receiving advice whose accuracy is lower than the decision maker’s initial forecasting accuracy, cognitive trust will decrease towards both advisors.
H5a: After receiving advice whose accuracy is higher than the decision maker’s initial forecasting accuracy, WOA will not decrease, independent from the source of advice.
H5b: After receiving advice whose accuracy equals the decision maker’s initial forecasting accuracy leads to behavioural algorithm aversion.
H5c: After receiving advice whose accuracy is lower than the decision maker’s initial forecasting accuracy, WOA towards both advisors will decrease.
3 Method
3.1 Task and procedure
We conducted an online experiment with 389 participants (203 women; 184 men; 2 diverse; Mdn age = 22) at a public university in Germany. After successful completion of the experiment, the participant entered a raffle of three Amazon vouchers of 50 Euros each. Informed written consent to take part in this experiment was obtained before the commencement by each participant.
The task is to forecast the demand for a product in the upcoming week based on a bar chart of the past 14 weeks including all daily demands in two different countries (see Appendix A). No more extraneous information was disclosed to prevent potential biases. Participants got familiar with the forecast task in two training rounds including performance feedback on their forecast absolute percentage error for each round. Afterwards, the external advisor (human/algorithm) is introduced and participants are informed about the procedure in this JAS. Before forecasting the demand in country 1, initial trusting beliefs and trusting intentions were queried. For each country, participants are asked to make a one-week-ahead point forecast of the product demand. One group is told that the advice was generated by an algorithm and the other group is led to believe that it was presented with advice given by their colleague. We deliberately framed the human advisor not as an expert to avoid responsibility shifting (Armstrong, Citation1980). The participants were randomly distributed to these two groups receiving the same set of bar charts. Then the participants had the chance to adjust their initial forecast in light of the presented external advice if deemed necessary.
Advice accuracy was pre-tested to ensure that the advice is on average better (5.41%), equal (10.70%), or worse (15.10%), respectively, than a participant’s initial forecasting accuracy. Advice accuracy of 15.10% is designed to worsen the accuracy of the participant as it increases the discrepancy to the correct value in the same direction as the average initial forecast in the pre-test. We explicitly provided feedback on initial and final absolute percentage error rate in addition to the absolute percentage error rate of the advisor after each round. The experiment took about 12 minutes on average to complete.
3.2 Measures
We apply the WOA variable commonly used in advice research. The change in judgement is set in relation to the difference between the advice and the initial point forecast. That is, a WOA of 100 % indicates that the advice is completely adopted, a WOA of 0% indicates that the advice is ignored. In short, the lower the WOA, the more sceptical is the decision maker about the advice. WOA is computed via the following equation:
The initial own forecast is the participant’s forecast before receiving advice, the final forecast the participant’s adjusted forecast after receiving advice.
To measure cognitive trust, we adapted existing cognitive trust measures from Lankton et al. (Citation2014) to the context of a JAS. We ask participants to rate the advisor’s trusting competence, benevolence, and integrity beliefs as well as trusting intentions on a Likert-type scale ranging from 1 (‘strongly disagree’) to 7 (‘strongly agree’). As an example, ‘I expect the algorithm to be capable of making an accurate forecast’ is used as a measure for the trusting competence in an algorithm (see Appendix B).
4 Results
4.1 Manipulation check
First, we tested whether the manipulation of the advice accuracy was successful. Analysing the initial forecast of the participants, we find that 48 entries are due to entering typos as the forecasts are extremely low (forecasts in a range between 1 and 30,200 whilst the last available order quantity was 304,146). We omitted these values as they distort the mean initial error rate and tested whether the 10.70% error rate treatment was equal to the initial own error rate, and whether the 5.41% (15.10%) error rate treatment was better (worse) than initial own forecast.
Three one-sample t-tests were run to determine if there were differences between advice error rate and initial own error rate. The advice error rate in the 5.41% error rate treatment (n = 106) was lower than initial own error rate (11.06 ± 4.67), a statistically significant difference of 5.65 (95% CI, 4.75 to 6.55), t(105) = 12.447, p < .001. Also, the advice error rate in the 15.10% error rate treatment (n = 120) was higher than initial own error rate (11.95 ± 8.62), a statistically significant difference of −3.15 (95% CI, −4.71 to – 1.59), t(119) = −4.00, p < .001. Most importantly, the advice error rate treatment of 10.70 % (n = 115) was equal to initial own error rate (11.36 ± 4.71), a non-significant difference of .66 (95% CI, −.21 to 1.53), t(114) = 1.498, p = .137, two-sided. The manipulation of the error rate was successful.
4.2 Hypotheses tests
We follow prior literature (Logg et al., Citation2019) and winsorised any WOA values greater than 1 or less than 0. If not otherwise stated, we test for the 5% significance level in the following analyses to determine a statistically significant effect.
4.2.1 Initial trust (H1 and H2)
In , we show descriptive statistics of initial cognitive trust and WOA.
Table 1. Descriptive statistics on initial cognitive trust and WOA in the source of advice.
Two separate regressions were run to predict initial WOA from initial trusting beliefs and initial trusting intentions as mediator.
In the human advisor group (n = 193), the direct effect of initial trusting beliefs on initial WOA is positive and significant (b = .0590, s.e. = .0276, p = .0342), indicating that participants scoring higher on initial trusting beliefs are more likely to weigh the advice higher than those scoring lower on that measure. The indirect effect of initial trusting beliefs via initial trusting intentions (IE = .0370) is positive and statistically significant, 95% CI [.0071; .0669]. The direct effect of initial trusting intentions on WOA is also positive and significant (b = .0541, s.e. = .0224, p = .0165), indicating that participants scoring higher on initial trusting intentions are more likely to weigh the advice higher than those scoring lower on that measure. This supports H1a.
In a supplementary analysis of the three trusting beliefs towards the human advisor, we find that the direct effect of initial trusting competence beliefs on WOA is positive and significant (b = .0504, s.e. = .0208, p = .0166), as well as its indirect effect (IE = .0265), 95% CI [.0073; .0497]. There is also a direct effect of initial trusting benevolence beliefs (b = .0856, s.e. = .0274, p = .0020), as well as its indirect effect (IE = .0293), 95% CI [.0016; .0570]. However, there is only an indirect effect of initial trusting integrity beliefs via initial trusting intentions (IE = .0202), 95% CI [.0095; .0336].
In the algorithmic advisor group (n = 196), the direct effect of initial trusting beliefs on initial WOA is insignificant and even negative (p = .9637) as well as its indirect effect via initial trusting intentions, 95% CI [−.0112; .0594]. The direct effect of initial trusting intentions on WOA is also insignificant (p = .2033). H1b is not supported.
To identify drivers that influence weight on algorithmic advice, we run a correlations analysis of several contextual factors on initial WOA (see Table 4). Only the familiarity of the participant with the application of an algorithm in this particular task domain was significantly correlated with initial WOA (r = -.162, p = .023, two-tailed). However, the correlation is negative, i.e. the more familiar they were, the lower the initial WOA.
To test H2a, a one-way MANOVA was run with advisor as fixed factor and four dependent variables: initial trusting competence, benevolence and integrity beliefs, and initial trusting intentions. The combined values were used to assess initial cognitive trust towards the advisor. There was no statistically significant main effect of advisor on the combined dependent variables initial cognitive trust, F(4, 384) = 1.361, p = .247, Pillai’s trace = .014. This supports H2a.
Analysed separately, there is only one statistically significant main effect of advisor on initial trusting integrity beliefs, F(1, 387) = 4.243, p = .040, partial η2 = .011. Mean initial trusting integrity beliefs in the algorithm (4.628) was higher than in the human (4.306), a statistically significant difference of .322, 95% CI [.015; .629].
An independent-samples Mann-Whitney U test was run to determine if there were differences in initial WOA between the advisors. Distributions of the initial WOA in both groups were similar, as assessed by visual inspection. Initial WOA was not statistically significantly different between the human (Mdn = .4183) and the algorithm (Mdn = .4899), U = 19947.00, z = .938, p = .348. The results support H2b.
4.2.2 Dynamic trust
Link between cognitive trust and WOA (H3)
Again, two separate regressions were run to predict dynamic WOA from dynamic trusting beliefs and dynamic trusting intentions as mediator.
In the human advisor group (n = 193), there is a significant and positive indirect effect of dynamic trusting beliefs on dynamic WOA (IE = .0391), 95% CI [.0077; .0684]. There is a direct effect of dynamic trusting intentions on dynamic WOA (b = .0529, s.e. .0212, p = .0133). The results support H3a. In a supplementary analysis of the three trusting beliefs towards the human, we find a significant and positive indirect effect of dynamic trusting competence beliefs (IE = .0325), 95% CI [.0128; .0527], and a significant and positive indirect effect of trusting integrity beliefs (IE = .0334), 95% CI [.0149; .0559] on dynamic WOA.
In the algorithmic advisor group (n = 196), there is a positive and significant direct (b = .0520, s.e. = .0228, p = .0237) as well as indirect effect (IE = .0284), 95% CI [.0003; .0588] of dynamic trusting beliefs on dynamic WOA. There is a marginally significant direct effect of dynamic trusting intentions on dynamic WOA (b = .0399, s.e. = .0227, p = .0799). The results support H3b. In a supplementary analysis of the three trusting beliefs towards the algorithm, we find a significant and positive indirect effect of dynamic trusting competence beliefs (IE = .0340), 95% CI [.0121; .0574], and of dynamic trusting integrity beliefs (IE = .0318), 95% CI [.0159; .0497], as well as a positive and significant direct (b = .0378, s.e. = .0132, p = .0047) and indirect effect of dynamic trusting benevolence beliefs (IE = .0175), 95% CI [.0033; .0319] on dynamic WOA. Dynamic trusting benevolence beliefs seem to be an important driver of dynamic weight on algorithmic advice, however, not of human advice.
Cognitive trust development (H4)
To test hypothesis 4, two three-way mixed ANOVAs were run to understand the effects of advisor, error rate and time on trusting beliefs. Pairwise comparisons are shown below.
In the 5.41% error rate treatment, mean dynamic trusting beliefs towards the human advisor (4.72) were equal to initial trusting beliefs (4.92), a mean difference of −.196, 95% CI [−.524, .132], p = .241. Mean dynamic trusting intentions in the human advisor (5.22) were even higher than initial trusting intentions (4.71), a mean difference of .515, 95% CI [.165, .865], p = .004. In the algorithmic advisor group, mean dynamic trusting beliefs (4.72) were equal to initial trusting beliefs (5.04), a mean difference of −.280, 95% CI [−.642, .082], p = .129. Also, mean dynamic trusting intentions (5.18) were higher than initial trusting intentions (4.77) in the 5.41% error rate treatment, a mean difference of .411, 95% CI [.025, .796], p = .037 (see, ). In sum, this supports H4a.
Figure 4. Changes in trusting beliefs (Panel A and B) and trusting intentions (Panel C and D) after performance feedback.
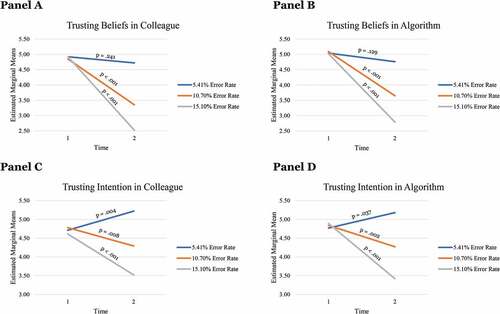
In the 10.70% error rate treatment, mean dynamic trusting beliefs towards the human advisor (3.35) were lower than initial trusting beliefs (4.85), a mean difference of −1.497, 95% CI [−1.838, −1.156], p < .001. Analogously, mean dynamic trusting intentions (4.29) were lower than initial trusting intentions (4.78), a mean difference of −.492, 95% CI [−.855, −.129], p = .008. This partly contradicts H4b. In the algorithmic advisor group, mean dynamic trusting beliefs (3.65) were lower than initial trusting beliefs (5.09), a mean difference of −1.438, 95% CI [−1.769, −1.107], p < .001. Analogously, mean dynamic trusting intentions (4.27) were lower than initial trusting intentions (4.84), a mean difference of −.567, 95% CI [−.920, −.215], p = .002. This partly supports H4b.
In the 15.10% error rate treatment, mean dynamic trusting beliefs towards the human advisor (2.52) were lower than initial trusting beliefs (4.92), a significant mean difference of −2.403, 95% CI [−2.747; −2.059], p < .001. Analogously, mean dynamic trusting intentions (3.52) were lower than initial trusting intentions (4.61), a significant mean difference of −1.097, 95% CI [−1.463; −.730], p < .001. In the algorithmic advisor group, mean dynamic trusting beliefs (2.79) were lower than initial trusting beliefs (5.02), a significant mean difference of −2.228, 95% CI [−2.545; −1.911], p < .001. Analogously, mean dynamic trusting intentions (3.42) were lower than initial trusting intentions (4.89), a significant mean difference of −1.466, 95% CI [−1.803; −1.128], p < .001. This supports H4c.
In supplementary analyses, an independent-samples Mann-Whitney U Test identified higher dynamic trusting integrity beliefs towards the algorithm (Mdn = 4.00) than towards the human advisor (Mdn = 3.00), U = 2617.00, p = .016.
WOA development (H5)
To properly test hypothesis 5, pairwise comparisons of a three-way mixed ANOVA were used to understand the effects of advisor, error rate and time on trusting beliefs.
In the 5.41% error rate treatment, mean initial weight on human advice (.296) was equal to dynamic weight on human advice (.392), a mean difference of .095, 95% CI [−.005, .195], p = .063. Analogously, mean initial weight on algorithmic advice (.383) was equal to dynamic weight on algorithmic advice (.416), a mean difference of .033, 95% CI [−.078, .143], p = .558 (see, , Panel A and B). This supports H5a.
In the 10.70% error rate treatment, mean initial weight on human advice (.440) was higher than dynamic weight on human advice (.277), a mean difference of −.163, 95% CI [−.267, −.059], p = .002. In contrast, mean initial weight on algorithmic advice (.424) was equal to dynamic weight on algorithmic advice (.406), a mean difference of −.018, 95% CI [−.119, .083], p = .728. This contradicts H5b. As a supplementary analysis, pairwise comparisons between human (.277) and algorithmic advisor (.406) indicate a mean difference in dynamic WOA of −.129, 95% CI [.018, .240], p = .023.
In the 15.10% error rate treatment, mean initial weight on human advice (.422) was higher than dynamic weight on human advice (.277), a significant mean difference of −.145, 95% CI [−.250; −.040], p = .007. Analogously, mean initial weight on algorithmic advice (.452) was higher than dynamic weight on algorithmic advice (.278), a significant mean difference of −.174, 95% CI [−.270; −.077], p < .001. This supports H5c.
In a supplementary analysis, a two-way ANOVA was run with advisor and error rate as fixed factors and the change in WOA as dependent variable. There is no significant interaction effect, p = .107, but there is a main effect of error rate, F(2, 383) = 9.395, p < .001.
Interestingly, we find a significant mean difference in the change of WOA (.145) between the human (mean = −.163) and the algorithm (mean = −.018) in the 10.70% error rate treatment, 95% CI [.000; .290], p = .050 (see, , Panel C).
5 Discussion
Initial trust
Rational decision makers are assumed to equally weigh external advice and their initial forecast in order to maximise forecasting accuracy, when no accuracy information is available (Dawes & Corrigan, Citation1974). In our experiment, participants initially discounted external advice, which indicates irrational behaviour.
At the beginning, we find no trust differences (cognitive trust and behaviour) towards human and algorithmic advisors, i.e. we do not observe algorithm aversion nor appreciation. This is in contrast with Longoni et al. (Citation2019) who observe algorithm aversion before interaction, i.e. lower initial trusting intentions towards the algorithm. However, their medical task domain differs in many aspects from our economic forecasting task domain. Therefore, this task difference needs to be discussed. For example, consequences of bad advice in medical healthcare would probably be more severe for participants than bad advice towards a product demand forecast. Furthermore, in our experiment participants had the opportunity to adapt their trust level due to repeated interactions. In the medical field, an error could potentially be lethal. Another explanation might be the divergent framing of the human advisor. As responsibility shifting might be caused by an advisor’s expert image (Armstrong, Citation1980), we deliberately informed the participants in our experiment that they will receive advice from a colleague of the same firm. As human advisors constitute the benchmark for comparisons in the algorithm aversion literature, examining the effect of advisor framing might be a fruitful avenue for future research.
Analysing the influencing effect of cognitive trust on behaviour, we separated between human and algorithmic advisors. When participants find themselves in a setting where they get advice from a human advisor, cognitive trust positively influences their behaviour. This was also proposed by our initial trust model (see, ). Surprisingly, this initial trust model does not hold when interacting with an algorithmic advisor. We find no influence of cognitive trust on behaviour in the case of an algorithmic advisor framing. We suspect that there might be other factors, such as distrust, that initially influence behaviour. In a more practical JAS setting, we expect the influence of cognitive trust on behaviour in the case of a human advisor even stronger. The result might be initial algorithm aversion. Still, such settings are currently little explored and seem to represent another promising direction for future research.
Dynamic trust
Rational decision makers are assumed to adapt their trust levels according to the performance feedback available (Deutsch, Citation1958; Kramer, Citation2010). Initial trust levels are adapted due to this expectation-disconfirmation process, resulting in dynamic trust levels. The PAS suggests no trust decreases if initial trust is confirmed by performance feedback. However, after exceeding the threshold between near-perfect and bad advice, initial trust is disconfirmed and thus decreases. Due to relatively higher expectations towards the algorithmic advisor, we hypothesized harsher trust decreasescompared to a human advisor. Vice versa, we expected trust towards the human advisor to decrease less strongly when providing the same advice accuracy due to a general acceptance of human errors (Renier et al., Citation2021). To accurately examine trust development (cognitive trust and behaviour), we therefore separated between source of advice and advice accuracy.
We find that behavioural changes are dependent on advice accuracy but also on the source of advice. The decision maker’s initial forecasting accuracy seems to be an important indication for the threshold between near-perfect and bad advice. Interestingly, after receiving advice whose accuracy equals the decision maker’s initial forecasting accuracy (i.e. a deviation of 10.70% from the true value in this experiment), we observe behavioural algorithm appreciation. At this threshold, dynamic weight on algorithmic advice is maintained at its initial level, however, dynamic weight on human advice decreases. This finding contradicts the PAS. We find that participants receiving algorithmic advice behave more rational to maximise their forecasting in situations where the accuracy of an external advice equals the decision maker’s initial forecasting accuracy. Averaging two judgements is at least as accurate as the average forecasting accuracy of a decision maker and is even more accurate in the case where the two judgements bracket the true value (Soll & Larrick, Citation2009). Consequently, averaging equally accurate forecasts would be rational, but this was not the case here.
As our results contradict the PAS, we would expect that initial expectations towards advice accuracy might have been even higher towards the human advisor than towards the algorithmic advisor. Paradoxically, participants with the human advisor framing initially expected a significantly lower forecasting accuracy (mean = 10.91% absolute error, n = 61, SD = 11.62) compared to the algorithmic advisor framing (mean = 8.46% absolute error, n = 65, SD = 13.07).Footnote2 These observed higher expectations towards the algorithmic advisor are still in line with the suggestion of the PAS, but we do not observe algorithm aversion as the hypothesised consequence of diverging levels of expectations. Instead, participants decrease weight on human advice relatively harsher, even though on average the initial expectations towards the human advisor were met. In contrast, despite relatively higher initial expectations towards the algorithmic advisor, that were not met, we do not observe any decreases in weight on algorithmic advice. It seems that the accuracy of the human advisor is assessed less accurately than the accuracy of the algorithmic advisor. Alternatively, participants might be more tolerant of deviations from expectations towards an algorithmic advisor.
When forecasting accuracy of the external advice is below or above this threshold between near-perfect and bad advice, we neither find algorithm appreciation nor algorithm aversion. Yet, it remains unclear whether algorithm aversion occurs when forecasting accuracy decreases further than we tested in this experiment. This might be one reason why our experiment did not replicate the findings of Prahl and Van Swol (Citation2017). Another reason could be that Prahl and Van Swol (Citation2017) may have provoked algorithm aversion resulting from a break in consistency due to a sudden bad advice and not due to bad advice itself. However, in a forecasting domain, barely any advisor can always provide constant accuracy. Therefore, reactions to inconsistent advice accuracies and the timing of bad advice are another promising field for future research.
Analogously to behaviour, we find cognitive trust differences only at the threshold between near-perfect and bad advice. Comparing cognitive trust indicates that trusting integrity beliefs, i.e. beliefs in an error-free analysis of the provided data set, are significantly higher towards the algorithmic advisor, which might be one reason why we observe behavioural algorithm appreciation.
Our results are in contradiction with Ring’s (Citation1996) concept of fragile and resilient trust. According to the resilient trust concept, participants would be more resilient to errors made by a human advisor as they share a relatively longer history. Maybe this has changed somewhat over the last decades with more human-machine interaction taking place in everyday life.
According to the dynamic trust model, we examine the influence of dynamic cognitive trust on behaviour after the first performance feedback and separate between the human and algorithmic advisor. Cognitive trust after performance feedback influences behaviour towards both sources of advice. Although there was little social interaction within the JAS setting, trusting benevolence beliefs had a strong influence on behaviour towards an algorithmic advisor. In contrast, the initial influence of trusting benevolence beliefs on behaviour towards the human advisor was not observable anymore. Future research could examine the influence of cognitive trust with regard to long-term relationships as this study focusses on a short time with two forecasts.
6 Conclusion
To the best of our knowledge, this is the first study which investigates the influence of advice accuracy on trust within a forecasting task. Comparing human with algorithmic advice, the PAS suggests algorithm aversion when advice is perceived as bad. Yet, the threshold between near-perfect and bad advice in a forecasting task is not further specified.
We conclude that an advisor’s initial cognitive trust does not decrease when advice accuracy is higher than the initial forecasting accuracy of a decision maker – independent from the source of advice. Advice that is more accurate than the decision maker’s initial forecasting accuracy can even increase trusting intentions. However, advice that is as accurate as the decision maker’s initial forecasting accuracy decreases cognitive trust towards the human and algorithmic advisor. WOA then decreases significantly, however, only when the advice comes from a human advisor. Weight on algorithmic advice maintains at its initial level, resulting in behavioural algorithm appreciation. This finding contradicts the PAS as it would suggest relatively higher expectations towards the algorithmic advisor that are relatively more probable to be disconfirmed.
In sum, our results point to an important threshold between near-perfect and bad advice and extend the findings of Prahl and Van Swol (Citation2017). We find that this threshold between near-perfect and bad advice seems to be the decision maker’s initial forecasting accuracy. In situations where accuracy of the external advice equals the decision maker’s initial forecasting accuracy, algorithmic advice is used more rational.
Disclosure statement
No potential conflict of interest was reported by the author(s).
Notes
1. In this study, we consider an algorithm as a deterministic or non-deterministic rule for the sequence of steps to solve a problem.
2. Two participants in each advisor group were omitted due to unrealistically high error rate expectations, distorting the means of both groups.
References
- Armstrong, J.S. (1980). The Seer-Sucker Theory: The value of experts in forecasting. Technology Review, 82(7), 16–24.
- Bhattacherjee, & Premkumar. (2004). Understanding changes in belief and attitude toward information technology usage: A theoretical model and longitudinal test. MIS Quarterly, 28(2), 229–254. https://doi.org/10.2307/25148634
- Bigman, Y.E., & Gray, K. (2018). People are averse to machines making moral decisions. Cognition, 181(181), 21–34. https://doi.org/10.1016/j.cognition.2018.08.003
- Bonaccio, S., & Dalal, R.S. (2006). Advice taking and decision-making: An integrative literature review, and implications for the organizational sciences. Organizational Behavior and Human Decision Processes, 101(2), 127–151. https://doi.org/10.1016/j.obhdp.2006.07.001
- Byström, K., & Järvelin, K. (1995). Task complexity affects information seeking and use. Information Processing & Management, 31(2), 191–213. https://doi.org/10.1016/0306-4573(95)80035-R
- Castelo, N., Bos, M.W., & Lehman, D.R. (2019). Task-Dependent algorithm aversion. Journal of Marketing Research, 56(5), 809–825. https://doi.org/10.1177/0022243719851788
- Dawes, R.M., & Corrigan, B. (1974). Linear models in decision making. Psychological Bulletin, 81(2), 95–106. https://doi.org/10.1037/h0037613
- Deutsch, M. (1958). Trust and suspicion. Journal of Conflict Resolution, 2(4), 265–279. https://doi.org/10.1177/002200275800200401
- Dietvorst, B.J., & Bharti, S. (2020). People reject algorithms in uncertain decision domains because they have diminishing sensitivity to forecasting error. Psychological Science, 31(10), 1302–1314. https://doi.org/10.1177/0956797620948841
- Dietvorst, B.J., Simmons, J.P., & Massey, C. (2015). Algorithm aversion: People erroneously avoid algorithms after seeing them err. Journal of Experimental Psychology: General, 144(1), 114–126. https://doi.org/10.1037/xge0000033
- Dietvorst, B.J., Simmons, J.P., & Massey, C. (2018). Overcoming algorithm aversion: People will use imperfect algorithms if they can (even slightly) modify them. Management Science, 64(3), 1155–1170. https://doi.org/10.1287/mnsc.2016.2643
- Dijkstra, J.J. (1999). User agreement with incorrect expert system advice. Behavior & Information Technology, 18(6), 399–411. https://doi.org/10.1080/014492999118832
- Dzindolet, M.T., Peterson, S.A., Pomranky, R.A., Pierce, L.G., & Beck, H.P. (2003). The role of trust in automation reliance. International Journal of Human-computer Studies, 58(6), 697–718. https://doi.org/10.1016/S1071-5819(03)00038-7
- Dzindolet, M.T., Pierce, L.G., Beck, H.P., & Dawe, L.A. (2002). The perceived utility of human and automated aids in a visual detection task. Human Factors: The Journal of the Human Factors and Ergonomics Society, 44(1), 79–94. https://doi.org/10.1518/0018720024494856
- Einhorn, H.J. (1986). Accepting error to make less error. Journal of Personality Assessment, 50(3), 387–395. https://doi.org/10.1207/s15327752jpa5003_8
- Fildes, R., Goodwin, P., Lawrence, M., & Nikolopoulos, K. (2009). Effective forecasting and judgmental adjustments: An empirical evaluation and strategies for improvement in supply-chain planning. International Journal of Forecasting, 25(1), 3–23. https://doi.org/10.1016/j.ijforecast.2008.11.010
- Fishbein, M., & Ajzen, I. (1977). Belief, attitude, intention, and behavior: An introduction to theory and research. Philosophy and Rhetoric, 10(2), 177–188.
- Gefen, & Straub. (2003). Managing user trust in B2C e-Services. e-Service, 2(2), 7–24. https://doi.org/10.2979/esj.2003.2.2.7
- Goodyear, K., Parasuraman, R., Chernyak, S., Madhavan, P., Deshpande, G., & Krueger, F. (2016). Advice taking from humans and machines: An fMRI and Effective Connectivity Study. Frontiers in Human Neuroscience, 10(10), 542. https://doi.org/10.3389/fnhum.2016.00542
- Highhouse, S. (2008). Stubborn Reliance on Intuition and Subjectivity in Employee Selection. Industrial and Organizational Psychology, 1(3), 333–342. https://doi.org/10.1111/j.1754-9434.2008.00058.x
- Hoff, K.A., & Bashir, M. (2015). Trust in automation: Integrating empirical evidence on factors that influence trust. Human Factors: The Journal of the Human Factors and Ergonomics Society, 57(3), 407–434. https://doi.org/10.1177/0018720814547570
- Jian, J.-Y., Bisantz, A.M., Drury, C.G., & Llinas, J. (2000). Foundations for an empirically determined scale of trust in automated systems. International Journal of Cognitive Ergonomics, 4(1), 53–71. https://doi.org/10.1207/S15327566IJCE0401_04
- Jussupow, E., Benbasat, I., & Heinzl, A. (2020). Why are we averse towards algorithms? A comprehensive literature review on algorithm aversion. Proceedings of the 28th European Conference on Information Systems (ECIS), June 15-17, 2020, An Online AIS Conference (pp. 168). https://aisel.aisnet.org/ecis2020_rp/168
- Komiak, S.Y.X., & Benbasat, I. (2006). The effects of personalization and familiarity on trust and adoption of recommendation agents. MIS Quarterly, 30(4), 941–960. https://doi.org/10.2307/25148760
- Komiak, S., Wang, W., & Benbasat, I. (2005). Comparing customer trust in virtual salespersons with customer trust in human salespersons. Proceedings of the 38th Annual Hawaii International Conference on System Sciences, 6 Jan, 2005, Big Island, HI, USA (pp. 175a–175a). IEEE. https://doi.org/10.1109/HICSS.2005.154
- Kramer, R.M. (2010). 7 Trust barriers in cross-cultural negotiations: Psychological analysis. In M. N. K. Saunders, D. Skinner, G. Dietz, N. Gillespie, & R. J. Lewicki (Eds.), Organizational trust: A cultural perspective (pp. 182). Cambridge University Press.
- Lankton, N., McKnight, D.H., & Thatcher, J.B. (2014). Incorporating trust-in-technology into Expectation Disconfirmation Theory. The Journal of Strategic Information Systems, 23(2), 128–145. https://doi.org/10.1016/j.jsis.2013.09.001
- Lee, M.K.O., & Turban, E. (2001). A trust model for consumer internet shopping. International Journal of Electronic Commerce, 6(1), 75–91. https://doi.org/10.1080/10864415.2001.11044227
- Lewicki, R.J., & Bunker, B.B. (1995). Trust in relationships: A model of development and decline. Jossey-Bass/Wiley.
- Lewicki, R.J., Tomlinson, E.C., & Gillespie, N. (2006). Models of interpersonal trust development: Theoretical approaches, empirical evidence, and future directions. Journal of Management, 32(6), 991–1022. https://doi.org/10.1177/0149206306294405
- Li, X., Hess, T.J., & Valacich, J.S. (2008). Why do we trust new technology? A study of initial trust formation with organizational information systems. The Journal of Strategic Information Systems, 17(1), 39–71. https://doi.org/10.1016/j.jsis.2008.01.001
- Logg, J.M., Minson, J.A., & Moore, D.A. (2019). Algorithm appreciation: People prefer algorithmic to human judgment. Organizational Behavior and Human Decision Processes, 151(151), 90–103. https://doi.org/10.1016/j.obhdp.2018.12.005
- Longoni, C., Bonezzi, A., & Morewedge, C.K. (2019). Resistance to Medical Artificial Intelligence. Journal of Consumer Research, 46(4), 629–650. https://doi.org/10.1093/jcr/ucz013
- Madhavan, P., & Wiegmann, D.A. (2007). Effects of information source, pedigree, and reliability on operator interaction with decision support systems. Human Factors: The Journal of the Human Factors and Ergonomics Society, 49(5), 773–785. https://doi.org/10.1518/001872007X230154
- Madsen, M., & Gregor, S. (2000). Measuring human-computer trust. 11th australasian conference on information systems, Brisbane, Australia (pp. 6–8).
- Mayer, R.C., Davis, J.H., & Schoorman, F.D. (1995). An integrative model of organizational trust. Academy of Management Review, 20(3), 709–734. https://doi.org/10.2307/258792
- McKnight, D.H., & Chervany, N.L. (2001). Trust and distrust definitions: One bite at a time. Trust in Cyber-societies (pp. 27–54). Berlin, Heidelberg: Springer.
- McKnight, D.H., Choudhury, V., & Kacmar, C. (2002). Developing and validating trust measures for e-Commerce: An integrative typology. Information Systems Research, 13(3), 334–359. https://doi.org/10.1287/isre.13.3.334.81
- McKnight, D.H., Cummings, L.L., & Chervany, N.L. (1998). Initial Trust Formation in New Organizational Relationships. Academy of Management Review, 23(3), 473–490. https://doi.org/10.5465/amr.1998.926622
- Meehl, P.E. (1954). Clinical versus statistical forecast: A theoretical analysis and a review of the evidence. Minneapolis, MN: University ofMinnesota Press.
- Obermaier R and Müller F. (2008). Management accounting research in the lab – method and applications. Z Plan, 19(3), 325–351. https://doi.org/10.1007/s00187-008-0056-1
- Önkal, D., Goodwin, P., Thomson, M., Gönül, S., & Pollock, A. (2009). The relative influence of advice from human experts and statistical methods on forecast adjustments. Journal of Behavioral Decision Making, 22(4), 390–409. https://doi.org/10.1002/bdm.637
- Ord, K., Fildes, R.A., & Kourentzes, N. (2017). Principles of business forecasting. New York, NY: Wessex Press.
- Pavlou, P.A., & Gefen, D. (2004). Building effective online marketplaces with institution-based trust. Information Systems Research, 15(1), 37–59. https://doi.org/10.1287/isre.1040.0015
- Prahl, A., & Van Swol, L.M. (2017). Understanding algorithm aversion: When is advice from automation discounted? Journal of Forecasting, 36(6), 691–702. https://doi.org/10.1002/for.2464
- Renier, L.A., Schmid Mast, M., & Bekbergenova, A. (2021). To err is human, not algorithmic – Robust reactions to erring algorithms. Computers in Human Behavior, 124(124), 106879. https://doi.org/10.1016/j.chb.2021.106879
- Ring, P.S. (1996). Fragile and resilient trust and their roles in economic exchange. Business & Society, 35(2), 148–175. https://doi.org/10.1177/000765039603500202
- Rousseau, D.M., Sitkin, S.B., Burt, R.S., & Camerer, C. (1998). Not so different after all: A cross-discipline view of trust. Academy of Management Review, 23(3), 393–404. https://doi.org/10.5465/amr.1998.926617
- Schmidt, P., Biessmann, F., & Teubner, T. (2020). Transparency and trust in artificial intelligence systems. Journal of Decision Systems, 29(4), 260–278. https://doi.org/10.1080/12460125.2020.1819094
- Siau, K., & Wang, W. (2018). Building trust in artificial intelligence, machine learning, and robotics. Cutter Business Technology Journal, 31(2), 47–53.
- Soll, J.B., & Larrick, R.P. (2009). Strategies for revising judgment: How (and how well) people use others’ opinions. Journal of Experimental Psychology. Learning, Memory, and Cognition, 35(3), 780–805. https://doi.org/10.1037/a0015145
- Xie, H., David, A., Mamun, M.R.A., Prybutok, V.R., & Sidorova, A. (2022). The formation of initial trust by potential passengers of self-driving taxis. Journal of Decision Systems, 1–30. https://doi.org/10.1080/12460125.2021.2023258
- Yu, K., Berkovsky, S., Taib, R., Zhou, J., & Chen, F. (2019). Do I trust my machine teammate? An investigation from perception to decision. Proceedings of the 24th International Conference on Intelligent User Interfaces, March 2019, Marina del Rey, California (pp. 460–468). New York, NY: Association for Computing Machinery. https://doi.org/10.1145/3301275.3302277
Appendix A. Product demand prediction task in Country 1 and Country 2
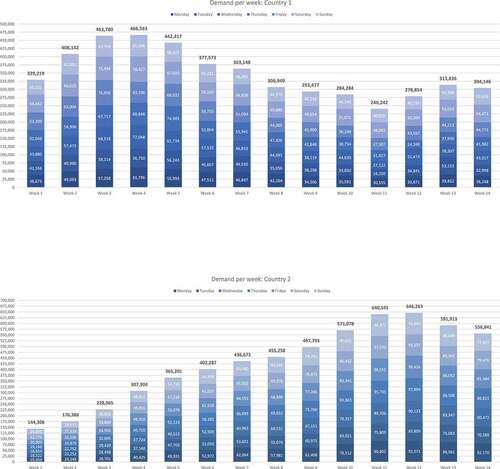
Appendix B. Questionnaire items
1. Measurement of Initial Cognitive Trust (adapted from McKnight et al., Citation2002; Lankton et al., Citation2014):
Initial Trusting Beliefs
I expect that my colleague/the algorithm …
Competence/Functionality
… will be able to make an accurate forecast.
Benevolence/Helpfulness
… will help me to improve my initial forecast.
Integrity/Reliability
… will analyse the available data error-free.
Initial Trusting Intentions
For an important forecasting task, I feel I can rely on my colleague/the algorithm.
2. Measurement of Dynamic Cognitive Trust (adapted from McKnight et al., Citation2002; Lankton et al., Citation2014):
Dynamic Trusting Beliefs
Based on the experience with my colleague/the algorithm, …
Competence/Functionality
… it was able to make an accurate forecast.
Benevolence/Helpfulness
… it helped me to improve my initial forecast.
Integrity/Reliability
… it analysed the available data error-free.
Dynamic Trusting Intentions
For an important forecasting task, I still feel I can rely on my colleague/the algorithm.