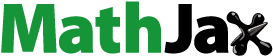
Abstract
Cyclodextrin glycosyltransferase (CGTase) is a bacterial glycosyltransferase which catalyses the conversion of starch to cyclodextrin. It can be produced industrially through a fermentation process. The optimal design of the fermentation medium and conditions is critical for metabolic production and microbial growth. Optimization of CGTase biosynthesis by recombinant Lactococcus lactis NZ:NSP:CGT was performed using an artificial neural network (ANN) as the main tool in order to improve the CGTase production in the fermentation process. Three parameters, including temperature, carbon source concentration and nitrogen source concentration, were determined as significant factors for improvement of CGTase production by L. lactis NZ:NSP:CGT in the fermentation process. Soluble potato starch and yeast extract were chosen as the best carbon and nitrogen sources for higher production of CGTase by L. lactis NZ:NSP:CGT. The optimum concentration of soluble starch and yeast extract were 3.82% and 5.67% (w/v), respectively, and the optimum temperature was 20 °C. The final CGTase activity under optimized conditions was 22.09 U/mL, which was close enough to the predicted value of 24.17 U/mL. The obtained results are of particular interest, as CGTase is an industrially important enzyme. In this study, its production through batch fermentation was optimized in order to maximize its production. In future studies, more investigations in various modes of fermentation should be conducted in order to increase the CGTase biosynthesis.
Abbreviations | ||
AAD: | = | absolute average deviation |
ANN: | = | artificial neural network |
CCD: | = | central composite design |
CD: | = | cyclodextrin |
CGTase: | = | cyclodextrin glycosyltransferase |
IBP: | = | incremental back propagation |
NICE: | = | Nisin controlled expression |
PBD: | = | Placket–Burman design |
RMSE: | = | root minimum square error |
Introduction
Cyclodextrin glycosyltransferase (CGTase, 1,4-α-D-glucan:1,4-α-D-glucopyranosyltransferase) is a key microbial amylolytic enzyme and a bacterial glycosyl transferase.[Citation1] This enzyme is classified as a member of the glycoside hydrolase family 13, which is recognized as α-amylase and is the largest family among glycoside hydrolases.[Citation2] CGTase catalytic activities range from intramolecular cyclization to intermoelcular reactions such as coupling and disproportionation as well as weak hydrolytic activity.[Citation3] Microorganisms synthesize CGTase in order to catalyse the conversion of starch present in their environment to cyclodextrin for the purpose of growth and survival.[Citation4] Several CGTase producers are Bacillus lehensis S8,[Citation5] Bacillus sp. TS1-1,[Citation6] B.megaterium,[Citation7] Paenibacillus pabuli US132 [Citation8] and Klebsiella pneumonia AS-22 .[Citation9] Bacillus species are well-known natural producers of CGTase.[Citation10]
CGTase converts starch and related compounds to cyclodextrin through cyclization activity.[Citation11] Cyclodextrin (CD), as the main and most important product of CGTase, is a non-reducing closed-ring maltooligosaccharide consisting of glucose monomers linked to each other by α1→4 glycosidic bonds.[Citation12,Citation13] The most common forms of CDs are comprised of six, seven or eight glucose residues and are known as α-, β- and γ-cyclodextrin, respectively.[Citation4] CD consists of a hydrophilic surface and an apolar cavity.[Citation12] The unique torus-shaped structure of CD with its non-polar interior leads to inclusion complex formation with other molecules.[Citation10] This capability of CDs leads to their wide use in different industries such as foods, cosmetics and toiletries, textiles, agrochemistry.[Citation14,Citation15]
Fermentation is employed for industrial enzyme production by microorganisms such as bacteria and fungi, which is performed under controlled conditions.[Citation16] In a fermentation process, the enhancement of enzyme yields is greatly influenced by physical and biological parameters.[Citation17] The optimal design of the culture medium and the environmental conditions are very critical aspects in the metabolic production and growth of microbial population and development of fermentation processes.[Citation18,Citation19] There are many studies by a number of researchers, e.g. Ravinder et al. [Citation19], Ahmed and El-Refai [Citation7], Kuo et al. [Citation20], Menocci and Goulart [Citation21] and Illias et al. [Citation10], conducted for the purpose of fermentation optimization, which reflects the importance of this issue. According to Khurana et al. [Citation22], the univariate technique is not suitable for use in all cases of optimized factor level determination in the enzyme production process. It is a time-consuming and difficult technique; it neglects the combinatorial effects and there is no guarantee that optimum results will be obtained.[Citation23]
Statistical optimization assists in accurate optimization of the process of interest while reducing the number of experiments as compared to conventional technique, therefore, immense saving in time and cost can be achieved.[Citation24] Recently, artificial neural network (ANN) has become an attractive tool for development of non-linear empirical models, especially in case of impracticality of conventional or phenomenological empirical models.[Citation25] ANN is a simulation of the human brain which is able to use the trained data in the range of an investigation to estimate the response.[Citation26]
The focus of this study was on optimization of CGTase biosynthesis by recombinant L. lactis NZ:NSP:CGT using ANN as the main tool. To the best of our knowledge, there is no literature available on either optimization of CGTase production using ANN or CGTase biosynthesis by L. lactis strain. Therefore, this study can be considered the first attempt at both.
Materials and methods
Strain and inoculum preparation
Recombinant Lactococcus lactis NZ:NSP:CGT, which carries the CGTase gene originally from Bacillus G1, was employed as the CGTase producer.[Citation1] Bacterial culture in the form of glycerol stock, maintained at −20 °C, was used for inoculum preparation. For this purpose, the stock culture was thawed and streaked on M17 agar (Merck, Germany) plate followed by incubation at 30 °C for 24 h. A single colony was inoculated in 10 mL of M17 broth and incubated overnight followed by a sub-culture in fresh M17 broth and incubation at the same conditions. A culture with optical density (OD) of 0.5 was used in order to inoculate the fermentation broth.
Experimental design
Plackett–Burman design
Initially, a screening step was performed using Placket–Burman Design (PBD) (Design Expert Software 6.06 (Stat Ease, USA)) in order to determine the significant factors out of all the parameters selected. The factors to be screened were initial pH, temperature, soluble starch concentration, yeast extract concentration, inoculum size and Na-β-glycerophosphate concentration based on published reports and M17 medium compositions. PBD suggested 12 experiments as presented in . The model of the system is expressed as follows:
(1)
(1) where Y represents the response variable and β0 is the interception coefficient; β1, β2, β3, β4, β5 and β6 are coefficients of the linear effects for the six independent variables (X1 = pH, X2 = temperature, X3 = soluble starch concentration, X4 = yeast extract concentration, X5 = inoculum size and X6 = Na-β-glycerophosphate concentration).
Table 1. Placket–Burman design matrix and corresponding results (CGTase activity and biomass concentrations).
Steepest ascent
The operating regions of optimization for significant parameters in the CGTase production process were determined using the steepest ascent method (). For this purpose, the first-order model generated by Plackett–Burman design (EquationEquation (1)(1)
(1) ) was employed. The experimental values were calculated using various coded values. Coded value determines the direction and scale of changes of each parameter.
Table 2. Steepest ascent/descent experiments and the corresponding results (CGTase activity and biomass concentrations).
Artificial neural network
The final optimization step was performed to determine the optimum level of key variables and their interaction effects on CGTase production as the main response. The experiments were designed using central composite design (CCD). According to CCD, a total of 19 experimental runs of fermentation (eight factorial points, six axial points (α = 1.682) and five centre points) were conducted as shown in .
Table 3. Experiments designed by CCD and the corresponding results (CGTase activity and biomass concentrations).
The experimental data were divided into training sets (14 items of data) and testing sets (5 items of data) with three input variables (temperature, soluble starch concentration and yeast extract concentration) and two output responses (CGTase activity and biomass concentration). The data were used to construct the regression-based networks by intelligent problem solver in Neural Power software (version 2.5 (CPS-X softwares, USA)). The training and testing processes were performed in order to determine the coefficient of correlation (R2), root minimum square error (RMSE) and absolute average deviation (AAD) values for the model:
(2)
(2) where X is the predicted CGTase activity, Y is the observed CGTase activity and
is the average observed CGTase activity;
(3)
(3) where yi,exp is the experimental response, yi,cal is the calculated response and n is the number of experiments;
(4)
(4) where yi,exp and yi,cal are the experimental and calculated responses, respectively, and P is the number of experiments.
A multilayer normal feed-forward network structure was used for model development, which was trained by incremental back propagation (IBP). The network structure consisted of one input layer (three nodes), one output layer (two nodes) and one hidden layer (nine nodes). The learning algorithms and number of neurons were involved in ANN parameters adjustments. Hyperbolic tangent transfer function was used, while other parameters for the network were set as default values.
Analytical procedures
The dry-cell-weight technique was used to measure the biomass concentration in the cultivation samples. Cell-free supernatant was used for substrate consumption determination. Starch-iodine assay was employed to measure the starch concentration in the samples.[Citation27] An appropriately diluted sample (1 mL) was mixed with 0.1 mL of iodine solution (0.2 g of I2 and 2.0 g of KI in 100 mL of distilled water). The mixture was then filled up to 10 mL using distilled water and the absorbance was measured at 590 nm.
Kaneko's method, with minor modifications, was employed for the CGTase assay.[Citation28] The reaction mixture containing 40 mg of soluble starch in 1.0 mL of 0.1 mol/L sodium phosphate buffer (pH 6.0) and 0.1 mL of crude enzyme was incubated at 60 °C for 10 min. The reaction was stopped by the addition of 3.5 mL of 30 mmol/L NaOH and 0.5 mL of 0.02% (w/v) phenolphthalein in 5 mmol/L Na2CO3 solution. The colour intensity was then measured at 550 nm using a spectrophotometer. One unit of the enzyme activity is defined as the amount of enzyme that forms 1 µmol of β-cyclodextrin per minute.
Results and discussion
Placket–Burman design
The PBD is considered a useful and powerful tool for quick selection of key factors in multivariable studies.[Citation29] Therefore, PBD was employed for screening in this study. The experimental matrix designed and the corresponding results are presented in .
The activity of the CGTase enzyme produced by L. lactis NZ:NSP:CGT varied from 0.00 U/mL to 9.18 U/mL in the 12 experiments due to the strong influence of some parameters on CGTase enzyme production. The low p-values (<0.05) of temperature, carbon source concentration and nitrogen source concentration show that these three parameters are significant factors for optimization of CGTase biosynthesis by L. lactis NZ:NSP:CGT. Based on the results, the lower limit of temperature (25 °C) and the upper limit of nitrogen source concentration (5 g/L) were more suitable for CGTase biosynthesis by L. lactis NZ:NSP:CGT, while the carbon source concentration was not found to influence the biosynthesis process. Further study is needed to determine the effects of these significant parameters on the responses with greater precision. A first-order model was fitted based on the results from 12 experiments and the equation was as follows:
(5)
(5) where Y is the CGTase activity and A, B, C, D, E and F are pH, temperature, soluble starch concentration, yeast extract concentration, inoculum size and Na-β-glycerophosphate concentration. The positive coefficients of soluble starch concentration (1.09) and yeast extract concentration (2.59) indicated the suitability of high concentrations of soluble starch and yeast extract for improvement of the CGTase production. As stated previously, soluble starch and yeast extract have induction effects on CGTase production.[Citation30,Citation31] In contrast, the negative coefficient of temperature (−1.14) suggested that low temperature is more favourable for CGTase biosynthesis.
Therefore, the three non-significant factors were eliminated from the formula and a new equation was formed as follows:
(6)
(6)
According to the results, there was a negative relationship between temperature and CGTase biosynthesis by L. lactis NZ:NSP:CGT, while carbon source and nitrogen source concentrations showed a direct positive relationship. These three parameters are normally among the ones used for CGTase production optimization. For example, carbon and nitrogen sources, as medium components, have been employed in CGTase optimization studies by Ibrahim et al.[Citation30], Ravinder et al. [Citation19], Sivakumar and Shakilabanu [Citation32] and Ayadi-Zouari et al. [Citation33]. On the other hand, temperature is considered a culture condition parameter, which has been studied by various researchers, e.g. Illias et al. [Citation10], Menocci and Goulart [Citation21] and Ravinder et al. [Citation19], in order to improve CGTase biosynthesis by various producers.
On the contrary, initial pH and inoculum size are among the parameters optimized by Ravinder et al. [Citation19], Illias et al. [Citation10], Menocci and Goulart [Citation21] and Sivakumar and Shakilabanu [Citation32] for CGTase production improvement, whereas these parameters did not show any significance in our study. These dissimilarities could be due to different characteristics of various CGTase producers in different environmental conditions and medium compositions.
The three significant factors (temperature, carbon source concentration and nitrogen source concentration) were subjected to further optimization to determine the optimum values for achieving maximum CGTase production.
Steepest ascent
In the steepest ascent technique, different values of three significant factors were tested to obtain the best range of optimization for each. For this purpose, Equation (6) was used. Based on the coefficients, the path was mostly moved along the direction in which soluble starch and yeast extract concentrations increased, while temperature decreased. The experiments performed and the corresponding results are presented in .
By increasing the coded value to more than one, no CGTase production was detected. This might be due to the very low temperature (13 °C), which can reduce the cell growth. The negative coded value resulted in a very low biomass concentration as well, which was most probably due to low concentrations of carbon and nitrogen sources. The highest CGTase enzyme activity of 24.27 U/mL was observed at 5.57% (w/v) soluble starch concentration, 5% (w/v) yeast extract concentration and temperature of 19 °C. According to the results, the aforementioned values are in the region of maximum CGTase production; therefore, they were used as centre points for further optimization. Thus, the centre point for temperature was chosen to be 19 °C and its range was set from 17 °C to 21 °C. The soluble starch concentration's centre point was 5.5% (w/v) and the upper and lower limits were 4.5% and 6.5% (w/v), respectively, while the yeast extract concentration was set between 4.5% and 5.5% (w/v). Since maximal CGTase activity can be possibly obtained in a specific range of carbon and nitrogen concentrations, the significant parameters and their corresponding spans determined so far were used for more accurate optimization.
Artificial neural network
CGTase biosynthesis by L. lactis NZ:NSP:CGT was further optimized using ANN. The experimental matrix designed by CCD and the corresponding responses are presented in .
The architecture of ANN is demonstrated by its topology.[Citation34] The network's topology comprised of three layers (3:9:2): an input layer of three fermentation variables; a middle, hidden layer, consisting of nine neurons and an output layer with two neurons for responses ().
Figure 1. Topology of the neural network for the estimation of CGTase production. Note: Black circles represent the inputs (neurons added for ANN processing): temperature, soluble starch concentration and yeast extract concentration. White circles represent the hidden and output layer (neurons generated during ANN processing). Red circles represent the bias.
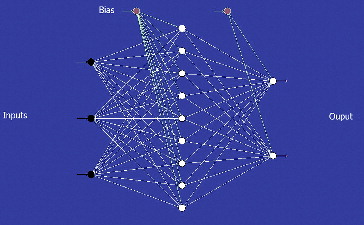
The network structure used for data was the multilayer full feed-forward IBP. Generally, feed-forward natural networks containing a hidden layer with a suitable number of neurons show capability of providing an accurate approximation.[Citation35] In the optimization of the neural network structure (number of hidden layers and neurons present, transfer function, learning rate, number of iterations and the momentum coefficient) in the learning and testing process, it is significant to obtain the best combinations and applications. The optimum number of hidden layers and their neurons are normally selected by the trial and error technique performed by the software itself. This optimization prevents the neural network from learning the database's noise. The number of iterations used in this study was between 49,000 and 57,000, with one hidden layer applied in the feed-forward network design. Increasing the numbers of iterations and hidden layers could lead to over-fitting.[Citation36]
The RMSE and determination coefficient for the testing set configuration was 0.234 and 0.967, respectively. ANN has the capability to rank the variables in terms of their importance based on responses ().
Figure 2. Importance of optimization parameters on CGTase production by Lactococcus lactis NZ:NSP:CGT. Note: A: temperature; B: soluble starch concentration; and C: yeast extract concentration.
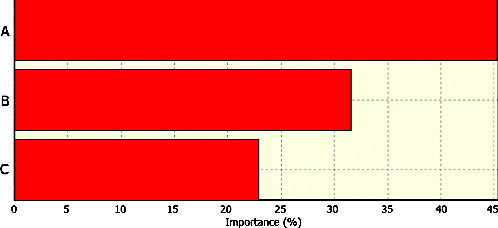
In this study, temperature showed the greatest importance (≈45.4%) for CGTase production optimization. Soluble starch concentration and yeast extract concentration represented the second (≈31.2%) and the third factor (≈23.4%) in terms of significant effects.
shows the three-dimensional plots of temperature, soluble starch concentration and yeast extract concentration on CGTase biosynthesis by L. lactis NZ:NSP:CGT. The response surface shown in (a) represents the interaction effect between temperature and soluble starch concentration. High CGTase production by L. lactis NZ:NSP:CGT was achieved at low concentrations of soluble starch and temperature between 19 °C and 20 °C. At higher concentrations of soluble starch, CGTase production was lowered. Even though from a concentration of 6.7% (w/v) onwards, CGTase production started to increase again, did not reach the maximum obtained at low concentrations, such as 3.6% (w/v). Temperatures other than the optimum point lead to a tremendous decrease in CGTase biosynthesis. (b) illustrates the maximum CGTase production as an interaction between temperature and yeast extract concentration. The trend of temperature effects on CGTase production is the same as in (a). Yeast extract concentration did not result in tangible differences but slightly higher CGTase biosynthesis was detected at higher yeast extract concentrations.
Figure 3. Three-dimensional plots of the ANN model for combined effects of temperature and soluble starch concentration (a) and temperature and yeast extract concentration (b) on CGTase production. Note: A: temperature; B: soluble starch concentration; and C: yeast extract concentration.
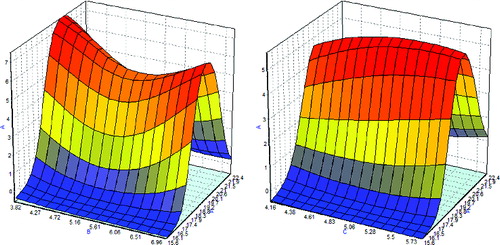
The final optimum values of the three variables were determined and the values were found to be: 20 °C for temperature and 3.82% and 5.67% (w/v) for the concentrations of soluble starch and yeast extract, respectively. A validation run using the optimum values was conducted to verify the obtained results. The obtained CGTase activity was 22.09 U/mL, while the predicted CGTase activity was determined as 24.17 U/mL. The predicted and the observed values differed by about two units. Generally, however, the exact same results were not obtained in any replicate of fermentations.
As mentioned earlier, the optimum concentration of soluble potato starch was determined as 3.82% (w/v). According to Burhan et al. [Citation37], strong substrate inhibition of microbial growth might occur at high starch concentrations. This is in agreement with the low concentration of biomass obtained for those high CGTase activities presented in . Moreover, high concentrations of starch increase the viscosity of the medium and lead to catabolite repression.[Citation6,Citation38] Therefore, the optimal concentration of carbon source obtained seems to be suitable in order to improve the CGTase production and at the same time to prevent the catabolite repression, high viscosity and extensive growth inhibition. Menocci and Goulart [Citation21] conducted a study on carbon source optimization for CGTase production by Bacillus sp. BACRP. The best carbon source was potato starch and the optimum concentration was determined as 4% (w/v). It is very similar with the results obtained in L. lactis.
The optimum yeast extract concentration was determined as 5.67% (w/v). As mentioned previously, yeast extract is a suitable nitrogen source for CGTase production by different species. Mahat et al. [Citation31] studied the optimum yeast extract concentration for CGTase production by Bacillus sp. TS1-1 and found the best concentration to be 1.89% (w/v). In another similar study, Ravinder et al. [Citation19] concluded that 0.5% (w/v) yeast extract concentration was the best for CGTase production by Bacillus sp. TPR71H. In contrast to these two studies, which employed Bacillus sp., as the enzyme producer, the present study used L. lactis as a CGTase producer and there is no information available about the optimum values for CGTase production by this strain.
There are similar studies on L. lactis for production of enzymes other than CGTase. Cheigh et al. [Citation39] employed L. lactis for bacteriocin production. In their study, the optimal value of yeast extract concentration as nitrogen source was determined as 3%. It can be suggested that various bacterial enzyme producers have different nitrogen requirements.
The expression system used in the present study is the nisin-controlled expression (NICE) system [Citation1,Citation40]. Mierau et al. [Citation40] conducted a study in order to optimize the L. lactis NICE system for industrial applications. They employed more than one nitrogen source (including yeast extract) in order to achieve the maximum expression of the NICE system and the total nitrogen sources concentration was 4.5% (w/v).
Recombinant CGTase-producing Escherichia E. coli was studied by Ravinder et al. [Citation19] in order to optimize the nitrogen source. In their study, combinational nitrogen sources were used and the total concentration was 7.5% (w/v). It seems that in the mentioned cases, recombinant CGTase production, specially using the NICE system, requires high nitrogen content to achieve its maximum expression capacity. The inducer-like activities of yeast extract toward CGTase production [Citation31] might be the reason for its high optimal concentration.
The optimum temperature for CGTase biosynthesis by L. lactis NZ:NSP:CGT was determined as 20 °C. As explained earlier, L. lactis NZ:NSP:CGT is a recombinant strain and the CGTase gene was originally cloned from Bacillus sp. G1 into E. coli and sub-cloned into L. lactis NZ:NSP:CGT. The optimum temperature for CGTase biosynthesis by Bacillus sp. G1 is 32 °C as reported by Illias et al. [Citation10]. Kim et al. [Citation41] studied the optimum culture conditions for the recombinant E. coli, carrying the CGTase gene from Bacillus sp. G1. They concluded that 20 °C is the best post-induction temperature to achieve the maximum CGTase activity. This is in good correspondence with the result obtained in this study. Normally, however, the optimum temperature for various producers of CGTase and for L. lactis producing different products is higher than 25 °C. For example, Low et al. [Citation42] reported 34.7 °C as the optimum temperature for CGTase biosynthesis by E. coli. Rosso et al. [Citation43], Mahat et al. [Citation31] and Higuti et al. [Citation44] concluded that 37 °C was the optimum temperature for maximum CGTase production by various Bacillus strains. In the case of L. lactis, Mall et al. [Citation45] and Sharma et al. [Citation46] determined 30 °C and 35 °C as the best temperature to achieve maximum nisin and bacteriocin production, respectively.
It has been observed that cold shock leads to induction of a few genes.[Citation47] Nisin induction is capable of acting in a vast range of temperatures, e.g. as low as 20 °C, but low temperatures might reduce the induction and heterologous protein production.[Citation40] Cultivating the recombinant bacteria at low temperatures enhances the desirable heterologous protein production.[Citation41] In this study, low temperature improved CGTase biosynthesis as well. Overall, CGTase biosynthesis by recombinant L. lactis NZ:NSP:CGT was successfully optimized and the enzyme activity was improved to 22.09 U/mL.
Conclusions
In this study, optimization of CGTase biosynthesis by recombinant L. lactis NZ:NSP:CGT was performed using ANN as the main tool. Initially, Placket–Burman Design (PBD) was used to determine the significant factors; followed by the steepest ascent method, which limited the operating region of optimization for significant parameters. The final optimization step was performed to determine the optimum level of key variables and their interaction effects on CGTase production as the main response. Temperature, carbon source concentration and nitrogen source concentration are three significant factors for optimization of CGTase biosynthesis by L. lactis NZ:NSP:CGT. At the end of this study, CGTase biosynthesis by recombinant L. lactis NZ:NSP:CGT was successfully optimized and the enzyme activity was improved to 22.09 U/mL.
Disclosure statement
No potential conflict of interest was reported by the authors.
Funding
This work was supported by the Ministry of Science, Technology and Innovation of Malaysia (MOSTI) [grant number 10-05-MGI-GMB001]; and the Research University Grant Scheme, Universiti Putra Malaysia.
References
- Subramaniam M, Baradaran A, Illias R, Rosfarizan M, Khatijah Y, Raha A. Effect of signal peptides on the secretion of β-cyclodextrin glucanotransferase in Lactococcus lactis NZ9000. J Mol Microbiol Biotechnol. 2012;22:361–372.
- Stam M.R, Danchin EGJ, Rancurel C, Coutinho PM, Henrissat B. Dividing the large glycoside hydrolase family 13 into subfamilies: towards improved functional annotations of alpha-amylase-related proteins. Protein Eng Des Selection. 2006;19(12):555–562.
- Ong RM, Goh KM, Mahadi NM, Hassan O, Rahman RNZRA, Illias RM. Cloning, extracellular expression and characterization of a predominant beta-CGTase from Bacillus sp. G1 in E. coli. J Ind Microbiol Biotechnol. 2008;35(12):1705–1714.
- Wang F, Du G, Li Y, Chen J. Optimization of cultivation conditions for the production of γ-cyclodextrin glucanotransferase by Bacillus macorous. Food Biotechnol. 2005;18(2):251–264.
- Yap P, Ariff A, Woo K, Hii S. Production of cyclodextrin glycosyltransferase (CGTase) by Bacillus lehensis S8 using sago starch as carbon source. J Biol Sci. 2010;10(7):676–681.
- Zain W, Illias R, Salleh M. Production of cyclodextrin glucanotransferase from alkalophilic Bacillus sp. TS1-1: optimization of carbon and nitrogen concentration in the feed medium using central composite design. Biochem Eng J. 2007;33(1):26–33.
- Ahmed E, El-Refai H. Cyclodextrin glucosyltransferase production by Bacillus megaterium NCR: evaluation and optimization of culture conditions using factorial design. Ind J Microbiol. 2007;50(3):303–308.
- Pinto F, Flôres S, Ayub M, Hertz P. Production of cyclodextrin glycosyltransferase by alkaliphilic Bacillus circulans in submerged and solid-state cultivation. Bioprocess Biosyst Eng. 2007;30(5):377–382.
- Gawande B, Patkar A. Purification and properties of a novel raw starch degrading-cyclodextrin glycosyltransferase from Klebsiella pneumoniae AS-22. Enzym Microb Technol. 2001;128(9–10):735–743.
- Illias R, Tien S, Rahman R, Rashid N, Yusoff W, Hamid A, Hassan O, Kamaruddin K. Application of factorial design to study the effects of temperature, initial ph and agitation on the production of cyclodextrin glucanotransferase from alkalophilic Bacillus sp. G1. Sci Asia. 2003;29:135–140.
- Matte CR, Nunes MR, Benvenutti EV, Schöffer JDN, Ayub MAZ, Hertz PF. Characterization of cyclodextrin glycosyltransferase immobilized on silica microspheres via aminopropyltrimethoxysilane as a “spacer arm”. J Mol Catalysis B: Enzym. 2012;78:51–56.
- Gastón JAR, Szerman N, Costa H, Krymkiewicz N, Ferrarotti SA. Cyclodextrin glycosyltransferase from Bacillus circulans DF 9R: activity and kinetic studies. Enzym Microb Technol. 2009;45(1):36–41.
- Sian H, Said M, Hassan O. Purification and characterization of cyclodextrin glucanotransferase from alkalophilic Bacillus sp. G1. Process Biochem. 2005;40(3–4):1101–1111.
- Dodziuk H. Molecules with holes–cyclodextrins. In: Dodziuk H, editor. Cyclodextrins and their complexes. Weinheim: WILEY-VCH Verlag GmbH &Co KGaA; 2006. p. 1–30.
- Uekama K, Hirayama F, Arima H. Pharmaceuticals applications of cyclodextrins and their derivatives. In: Dodziuk H, editor. Cyclodextrins and their complexes. Weinheim: WILEY-VCH Verlag GmbH &Co KGaA; 2006. p. 381–422.
- Sarrouh B, Santos TM, Miyoshi A, Dias R, Azevedo V. Up-to-date insight on industrial enzymes applications and global market. J Bioprocess Biotech. 2012;S4:002.
- Gangadharan D, Nampoothiri KM, Pandey A. α-Amylase production by Bacillus amyloliquefaciens using agro wastes as feed stock. Food Technol Biotechnol. 2011;49(3):336–340.
- Muthulakshmi C, Gomathi D, Kumar DG, Ravikumar G, Kalaiselvi M, Uma C. Production, purification and characterization of protease by Aspergillus flavus under solid state fermentation. Jordan J Biol Sci. 2011;4(3):137–148.
- Ravinder K, Prabhakar T, Bhavanidevi R. Optimization of process parameters for the production of cyclodextrin glycosyltransferase by newly isolated Bacillus sp. TPR71H by conventional method. Int J Adv Biol Res. 2012;3(2):578–584.
- Kuo CC, Lin CA, Chen JY, Lin MT, Duan KJ. Production of cyclodextrin glucanotransferase from an alkalophilic Bacillus sp. by pH stat fed-batch fermentation. Biotechnol Lett. 2009;31:1723–1727.
- Menocci V, Goulart A. Cyclodextrin glycosyltransferase production by new Bacillus sp. strains isolated from Brazilian soil. Braz J Microbiol. 2008;39:682–688.
- Khurana S, Kapoor M, Gupta S, Kuhad RC. Statistical optimization of alkaline xylanase production from Streptomyces violaceoruber under submerged fermentation using response surface methodology. Ind J Microb. 2007;47(2):144–52.
- Li Y, Liu Z, Zhao H, Xu Y, Kui F. Statistical optimizaion of xylanase production from new isolated Penicillium oxalicum ZH30 in submergerd fermentation. Biochem Eng J. 2007;34:82–86.
- Mander P, Choi YH, Seong JH, Na BH, Cho SS, Lee HJ, Yoo JC. Statistical optimization of a multivariate fermentation process for enhancing antibiotic activity of Streptomyces sp. CS392. Arch Pharm Res. 2013;36:973–980.
- Desai KM, Akolkar SK, Badhe YP, Tambe SS, Lele SS. Optimization of fermentation media for exopolysaccharide production from Lactobacillus plantarum using artificial intelligence-based techniques. Process Biochem. 2006;41(8):1842–1848.
- Baş D, Boyacı İH. Modeling and optimization II: comparison of estimation capabilities of response surface methodology with artificial neural networks in a biochemical reaction. J Food Eng. 2007;78(3):846–854.
- Nakamura LK. Lactobacillus amylovorus, a new starch-hydrolyzing species from cattle waste-corn fermentations. Int J Syst Bacteriol. 1981;31(1):56–63.
- Kaneko T, Kato T, Nakamura N, Horikoshi K. Spectrophotometric determination of cyclization activity of β-cyclodextrin-forming cyclomaltodextrin glucanotransferase. J Japanese Soc Starch Sci. 1987;34:45–48.
- Zhu LW, Wang CC, Liu RS, Li HM, Wan DJ, Tang YJ. Actinobacillus succinogenes ATCC 55618 fermentation medium optimization for the production of succinic acid by response surface methodology. J Biomed Biotechnol. 2012;626137.
- Ibrahim HM, Yusoff WMW, Hamid AA, Illias RM, Hassan O, Omar O. Optimization of medium for the production of β-cyclodextrin glucanotransferase using Central Composite Design (CCD). Process Biochem. 2005;40(2):753–758.
- Mahat M, Illias R, Rahman R. Production of cyclodextrin glucanotransferase (CGTase) from alkalophilic Bacillus sp. TS1-1: media optimization using experimental design. Enzym Microb Technol. 2004;35:467–473.
- Sivakumar N. Shakilabanu S. Original research article production of cyclodextrin glycosyl transferase by Bacillus megaterium. Int J Current Microbiol Appl Sci. 2013;2(7):44–55.
- Ayadi-Zouari D, Kammoun R, Jemli S, Chouayekh H, Bejar S. Secretion of cyclodextrin glucanotransferase in E. coli using Bacillus subtilis lipase signal peptide and optimization of culture medium. Ind J Exp Bio. 2012;50(1):72–79.
- Nelofer R, Ramanan RN, Rahman RNZRA, Basri M, Ariff AB. Comparison of the estimation capabilities of response surface methodology and artificial neural network for the optimization of recombinant lipase production by E. coli BL21. J Ind Microbiol Biotechnol. 2012;39(2):243–254.
- Nagata Y, Chu KH. Optimization of a fermentation medium using neural networks and genetic algorithms. Biotechnol Lett. 2005;25(21):1837–1842.
- Tan J, Ramanan R, Ling T, Shuhaimi M, Ariff A. Comparison of predictive capabilities of response surface methodology and artificial neural network for optimization of periplasmic interferon-α2b production by recombinant Escherichia coli. Minerva Biotecnol. 2011;23:1–2.
- Burhan N, Sapundzhiev T, Beschkov V. Mathematical modelling of cyclodextrin-glucanotransferase production by batch cultivation. Biochem Eng J. 2005;24(1):73–77.
- Gawande B, Sonawane A, Vogdand V, Patkar A. Optimization of cyclodextrin glycosyltransferase production from Klebsiella pneumoniae as-22 in batch, fed-batch, and continuous cultures. Biotechnol Prog. 2003;19(6):1679–1702.
- Cheigh CI, Choi HJ, Park H, Kim SB, Kook M-C, Kim TS, Jae-Kwan H, Pyun Y-R. Influence of growth conditions on the production of a nisin-like bacteriocin by Lactococcus lactis subsp. lactis A164 isolated from kimchi. J Biotechnol. 2002;95(3):225–235.
- Mierau I, Olieman K, Mond J, Smid EJ. Optimization of the Lactococcus lactis nisin-controlled gene expression system NICE for industrial applications. Microb Cell Fact. 2005;4(1):1–12.
- Kim P, Hassan O, Ahmad A, Muhammad N, Illias R. Excretory over-expression of Bacillus sp. G1 cyclodextrin glucanotransferase (CGTase) in Escherichia coli: optimization of the cultivation conditions by response surface methodology. Enzym Microb Technol. 2007;40:1256–1263.
- Low KO, Mahadi NM, Rahim RA, Rabu A, Bakar FDA, Murad AMA, Illias RM. An effective extracellular protein secretion by an ABC transporter system in Escherichia coli: statistical modeling and optimization of cyclodextrin glucanotransferase secretory production. J Ind Microbiol Biotechnol. 2011;38(9):1587–1597.
- Rosso AM, Ferrarotti SA, Krymkiewicz N, Nudel C. Optimization of batch culture conditions for cyclodextrin glucanotransferase production from Bacillus circulans DF 9R. Microb Cell Fact. 2002;9:1–9.
- Higuti IH, Anunciação P, José A. Studies on alkalophilic CGTase-producing bacteria and effect of starch on cyclodextrin-glycosyltransferase activity. Braz Arch Biol Technol. 2004;47:135–138.
- Mall P, Mohanty BK, Patankar DB. Physiochemical parameters optimization for enhanced nisin production by Lactococcus lactis (MTCC 440). Braz Arch Biol Technol. 2010;53(1):203–209.
- Sharma S, Garg A, Singh G. Optimization of fermentation conditions for bacteriocin production by Lactococcus lactis CCSULAC1 on modified MRS medium. Int J Dairy Sci. 2010;5(1):1–9.
- Wouters JA, Kamphuis HH, Kuipers OP, De Vos WM. Changes in glycolytic activity of Lactococcus lactis induced by low temperature. Appl Environ Microbiol. 2002;66(9):3686–3691.