Abstract
Jatropha curcas L. seeds possess high oil content. However, the low fruit yield is a bottleneck for its utilisation in biodiesel production. To date, the genes related to fruit yield of J. curcas are still poorly studied. Therefore, this study was conducted to compare the transcriptome profiles of two J. curcas clones with high and low fruit yield. The results showed that 52 381 unigenes were obtained and annotated to 55 orthologous terms. More than 9 653 single nucleotide polymorphisms (SNPs)/Indels and 15 971 SSR loci were identified. In addition, 76 differential expression genes (DEGs) were obtained and mainly down-regulated in a high fruit yield sample. Moreover, the DEGs were significantly enriched in phytohormone signalling pathways, including gibberellin, brassinolide and jasmonic acid. Furthermore, we suggested that some genes might inhibit the initiation of female floral differentiation to decrease the fruit yield in J. curcas. These data will create a reference transcriptome for the genomics database of J. curcas for future studies. We expect that the dataset will serve as a foundation to study the genes function, which help in engineering high-yielding varieties in J. curcas.
Introduction
The physic nut, Jatropha curcas L. (Euphorbiaceae), is a native species of Mexico and Central America, and is widely distributed in tropical and subtropical regions [Citation1]. The seeds of J. curcas contain a high percentage of lipids. However, its use in biodiesel production has been hindered by low fruit yield [Citation2]. Thus, an improvement of the plant to cultivate high yield Jatropha germplasm is desirable. Comparative transcriptome analysis is a better way to uncover the genetic mechanism responsible for fruit yield [Citation3]. The genes involved in fruit yield could be identified using a comparative transcriptome analysis on contrasting materials [Citation4]. In a previous study, 12084 ESTs have been reported in developing seeds of J. curcas [Citation5]. In another empirical study, a comparative transcriptome analysis was performed between gynoecious and monoecious Jatropha, and 70 genes were detected and enriched in phytohormone biosynthesis and signalling pathways [Citation4]. However, the information regarding transcriptome alterations associated with fruit yield in J. curcas remains scarce.
Physiologically, photosynthate is partitioned between vegetative and reproductive components during vegetative and reproductive growth stages [Citation6]. Fruit yield was maximized in kiwifruit and grapevine using a strategy consisting of multiple switches from vegetative to reproductive growth [Citation7]. The novel genes expressed during the transition from vegetative to reproductive growth represent an approach to understanding fruit yield at the molecular level [Citation8]. Therefore, the leaf buds might be a better sample to investigate these differentially expressed genes (DEGs) in different cultivars with significant variation in fruit yield. Moreover, phytohormones play important regulatory roles for fruit yield in plant reproductive growth stages. Auxin (IAA) and gibberellin (GA) are central plant hormones during growth and development [Citation9]. Auxin, a byproduct of tryptophan, has physiological effects on plant growth [Citation10]. GA has an important regulatory role during female flower differentiation, and its signal transductions are achieved by degrading DELLA proteins [Citation11]. Brassinolide (BR) and jasmonic acid (JA) were significantly involved in male floral differentiation in Arabidopsis [Citation12,Citation13]. Furthermore, it has been repeatedly indicated that spraying exogenous plant hormone could significantly increase the fruit yield in J. curcas [Citation14,Citation15]. To date, the genes related to fruit yield are still poorly understood in J. curcas.
Therefore, the present study was conducted to compare the transcriptomic profiles of two Jatropha samples using the Illumina Hiseq 2500 platform to identify DEGs related to fruit yield (FY). These data will provide some candidate genes potentially involved in fruit yield. We expect this study to improve our understanding towards increasing the fruit yield in J. curcas.
Materials and methods
Investigation of fruit and seed traits
In the present study, two clones of J. curcas, GC_19 and DC_45, were collected in Xinhai State-owned Protection Forest Farm (110.25°E, 19.76°N), Haikou, Hainan Province, China. Information about the geographic origin of these materials is provided in Supplementary Table S1. Forty individuals of each clone were planted in March 2010. The trial site is located in a subtropical maritime monsoon climate area, with an annual average sunshine >2000 h, mean annual temperature of 23.8 °C, and mean annual rainfall of 1664 mm. The plant density was 2500 plants per hectare at a distance of 2 m × 2 m. The fruit yield per plant (FY) of each clone was examined in November, 2014. All of the matured fruits (colour turned to black) for each clone were collected in December, 2014. Six seed traits: fruit weight (FW), seed rate (SR), seed volume (SV), 100-seed weight (SW), kernel rate (KR) and oil content (OC), were investigated with three replicates in each clone (Supplementary Table S2). Thereafter, transcriptome sequencing procedures were conducted to screen DEGs.
Sample collection and RNA extraction
The mixed leaf bud tissues of each clone (from 10 individuals) were collected in March 2015, and immediately frozen into liquid nitrogen until stored at –80 °C for RNA extraction. The total RNA of each sample was extracted separately using the TIANDZ plant RNA kit (Column Plant RNAout 2.0, TIANDZ, Beijing). The quantity and purity of total RNA was examined using agarose gel electrophoresis, Qubit® RNA Assay Kit (Life Technologies, CA, USA), and Nanodrop 2100 spectrophotometer (Agilent Technologies, USA).
Transcriptome sequencing and assembly
The two cDNA libraries in our study, including GC_19 and DC_45, were sequenced with Illumina HiSeq™ 2500 (Illumina, USA) at Novegene Bioinformatics Technology Co. Ltd (Beijing, China). Sequence adaptors, N’s more than 10% and low-quality reads (Qphred ≤20 for >50% read) were removed according to the method described by Liu et al. [Citation16]. The unigenes were assembled using Trinity software. De novo transcriptome data was used as a reference database to calculate the read count of each unigene among the samples and converted to expected number of reads per kilobases per million reads (RPKM) as described by Mortazavi et al. [Citation17].
Gene functional annotation and DEG analysis
Functional annotation and classification of all the unigenes were performed according to the Gene Ontology database (GO, http://www.geneontology.org/) and the Kyoto Encyclopedia of Genes and Genomes database (KEGG, https://www.kegg.jp/). Fold change (FC) is the gene expression difference between different samples. We used the threshold |log2 (FC)| > 1 and q < 0.005 as the criteria for identifying the DEGs. All of the up- or down-regulated genes in the present description were regulated in the first comparison component (GC_19). The Blastx alignments were conducted for the DEGs to TAIR 10 database (https://www.arabidopsis.org/). The volcano plot of DEGs in this study was drawn by R software (R-2.15.3-win) according to the procedure described by Silva et al. [Citation18].
Identification of SSRs, SNPs and Indels
Simple sequence repeats (SSR) were identified using MISA as described by Wang et al. [Citation19]. The samtools and picard tools were used to sort the clean data, and SNP calling and Indel calling were performed using GATK 2.0 according to the procedure described by Farrer et al. (2013) [Citation20]. Similar criteria for screening high-quality SNPs have been performed in previous studies [Citation21].
Quantitative real-time PCR (qRT-PCR) analysis
To validate the transcriptomic results, we isolated total RNA from the same plant materials, and a total of 23 DEGs were selected for qRT-PCR analysis. These genes were selected because of their high fold change based on DEGs analysis in the present study. The cDNA synthesis of the two samples (GC_19 and DC_45) was performed using PrimeScript® II first Strand cDNA Synthesis Kit (TaKaRa, Japan). The specific primers were designed by Primer Premier 5.0 (Supplementary Table S3). qRT–PCR was performed on Roche LightCyler480 system (Roche, Germany) with SYBR Premix Ex TaqTM II (TaKaRa, Japan). The cycling reaction was 94 °C for 2 min, followed by 40 cycles of 94 °C for 10 s, 55 °C for 10 s and 72 °C for 20 s. Three replicates were included for each gene, and β-actin (Jcactin) was used as the internal control according to the method described by Zhang et al. [Citation22]. The 2–ΔΔCt method was used to calculate the relative expression level of DEGs [Citation23]. The DEGs data have been deposited in GenBank Database under accession numbers from KY978611 to KY978627.
Data analysis
The data are mean values with standard deviation (±SD). Statistical analysis was performed using R software (R-2.15.3-win) for Duncan’s multiple range test and the correlation between qRT-PCR and RNA-seq data. The level of significance was set to p<0.05.
Results and discussion
Phenotypic variation of different clones
Forty individuals of each clone were evaluated to uncover the significant variation for all the investigated traits, except Kernel Rate (KR) (). These traits showed high values in GC_19, whereas they were lower in DC_45, especially FY (12.4).
Table 1. Statistical results of fruit & seed traits in J. curcas.
RNA-seq and assembly
Total RNA of GC_19 and DC_45 was isolated from J. curcas leaf bud tissues. RNA quality was determined using OD260/OD280 ratio (2.0 ± 0.05) and RIN (7.9–9.8) (Supplementary Table S4). A total of 99 219 542 raw reads was produced by transcriptome sequencing. After trimming adapters and low quality bases, 3.95G and 5.80 G high quality reads remained for GC_19 and DC_45, respectively (Supplementary Table S5). A total of 89 085 transcripts and 52 381 unigenes were obtained with a mean length of 1 411 bp (Supplementary Table S6; ). The length of the unigenes ranged from 201 to 17 124 bp with an average of 915 bp and more than half of the total assembly length >2 300 bp (N50 = 2 371) (Supplementary Table S6; ).
Gene functional annotation
All the unigenes were functionally characterized using BLAST on the Nr, Nt, KO, SwissProt, Pfam, GO, and KOG (). A total of 23 236 (44.35%) unigenes were annotated in at least one database. Moreover, 9 032 (17.24%) unigenes were assigned to orthologous groups of eukaryotic complete genomes (KOG), and the protein domains of 16 377 (31.26%) unigenes were annotated with Pfam. In addition, 18 093 (34.54%) unigenes were annotated with Gene Ontology (GO) terms (; ). The comparative analysis of the transcriptomes produced 55 orthologous terms, including 23 terms involved in biological processes, 19 terms involved in cellular components, and 13 terms involved in molecular functions.
Figure 2. Gene ontology distributions for the transcriptome of J. curcas. Main functional categories of the transcriptome related to plant physiology of the molecular function, cellular component, and biological process. The abscissas show the number of unigenes, and one unigene may be associated with different GO terms.
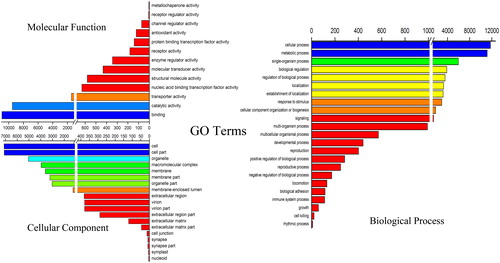
Table 2. Statistics about the gene functional annotation in different databases.
Furthermore, the unigenes were also classified into metabolic pathways using the Kyoto Encyclopedia of Genes and Genomes (KEGG). A total of 6 455 unigenes (12.32%) were annotated and divided into 31 categories of five clusters according to cellular processes, environmental information processing, genetic information processing, and metabolism and organismal systems (). In the KEGG pathway, 163 unigenes were related to plant hormone signal transduction, and 4 unigenes were related to steroid hormone biosynthesis. In addition, 75 unigenes were related to fatty acid biosynthesis and metabolism, with 14 unigenes involved in linoleic acid metabolism and 30 unigenes involved in α-linolenic acid metabolism.
Identification of SNPs, Indels and SSRs
More than 9 653 SNPs/Indels (5 582 SNPs and 4 071 Indels) were identified in the present study. Eighty-five percent (85%) of the transcripts contained only one or two SNPs/Indels (). Transitions (7 797; 53.31%) in SNPs were detected more frequently than transversions (6 830; 46.69%) (). Mononucleotide deletions were the most frequent mutation type (). Mono- to hexa-nucleotide SSRs were identified during the analysis of the unigenes (; Supplementary Table S7). A total of 15 971 SSR loci were detected and characterised, with mononucleotide repeats being the most abundant (10 646; 66.66%), followed by dinucleotides (2 964; 18.56%). These results could contribute to the development of a molecular marker database that can be used for genotype identification, genetic mapping and marker-assisted selection breeding. Moreover, at least 10 repeat mononucleotide SSRs were obtained, ranging from 10 to 24 bp. However, the di- to hexa-nucleotide SSRs mostly had from 5 to 12 repeat counts (Supplementary Table S7). Logically, the shorter repeat types would be expected to have more repeat counts and to be detected more generally. This result is consistent with previous studies in various plants [Citation24]; the types of SSR are influenced by the selective forces in different genes [Citation25].
Figure 4. Number of SNPs, Indels and SSRs in J. curcas. (a) Number of SNPs and Indels detected per unigene. (b) Frequencies of substitution types for different SNPs. (c) Frequencies of different indels. (d) Distribution of SSRs in J. curcas expressed sequence tags (EST) and the insert shows the distribution of the total number of SSRs in mono-, di-, tri-, tetra-, penta- and hexa-nucleotide repeats.
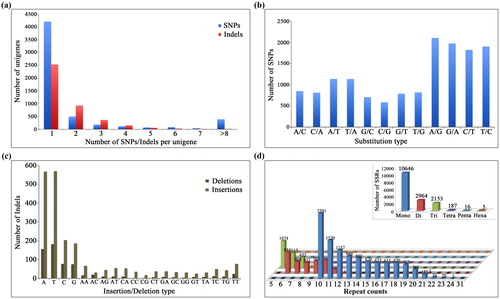
Differential expressed gene analysis
To investigate the DEGs associated with fruit yield, we collected leaf buds of two Jatropha clones with significant variation in fruit yield. In total, 76 DEGs were obtained: 9 upregulated and 67 downregulated in GC_19 vs. DC_45 (Supplementary Figure S1). In a previous study, the lower fruit yield was limited by poor female flowers in J. curcas, and the female floral differentiation could be initiated by inhibiting the expression of TS2, a gene for carpel abortion in J. curcas [Citation26]. Additionally, our previous study also found that the DEGs were mainly down-regulated in the female floral differentiation process [Citation27]. Thus, we suggested that some genes might inhibit the female floral differentiation, which resulted in the lower fruit yield in J. curcas. If these genes were downregulated to release the inhibition, the female floral differentiation would be induced to increase the fruit yield, and visa versa. This could reveal an underlying reason why a poor fruit yield was shown in J. curcas. In further studies, a strategic approach would be to knock down the key inhibitory genes to improve the female flowers and increase the seed yield of J. curcas.
Based on the KEGG enrichment analysis (), three DEGs, comp28501 (JcCLA1), comp25448 (JcFKF1) and comp25524 (JcGUN5), were significantly related to plant photosynthesis; two of them, comp10288 (JcDMR6) and comp24892 (JcTT4), were annotated for flavonoid biosynthesis; comp14170 (JcCPD) was associated with the brassinosteroid biosynthetic pathway; comp27080 (JcEBF1) was annotated as an EIN3-binding protein related to ethylene signal transduction pathways; comp12327 (JcGA2ox8) was annotated for gibberellin 20-oxidase activity; comp19008 (JcLOX3) and comp26531 (JcLOX2) were involved in jasmonic acid biosynthetic pathways; comp34410 (JcCYP79B2) was involved in tryptophan metabolism, a precursor of IAA; whereas the function of comp12317 was unknown, although it showed significant upregulation in GC_19 vs. DC_45. In addition, other DEGs were mainly related to the RNA transport, plant sugar metabolism and protein synthesis pathways. This suggested that the fruit yield was significantly associated with the phytohormone signalling pathway, and the JcGA2ox8 could positively influence the yield of J. curcas. It also uncovered a reason that the exogenous plant hormone was a feasible method to obtain a higher fruit yield in J. curcas [Citation28,Citation29].
Table 3. Significantly enriched DEGs associated with fruit yield in J. curcas.
Crop yields are often increased indirectly by phytohormones [Citation30], therefore understanding its internal mechanisms can lead to greater control of fruit yield [Citation26]. In the present study, JcGA2ox8 was upregulated, whereas the others (JcCLA1, JcFKF1, JcDMR6, JcTT4, JcCPD, JcEBF1, JcLOX3, JcLOX2 and JcCYP79B2) were mainly downregulated (). In a previous study, the exogenous application of GA could partially prevent pistil development in gynoecious Jatropha [Citation4]. GA2ox8 could inhibit bioactive gibberellins to reduce their content [Citation31]. In addition, the high level of BR could increase the seed yield in Arabidopsis [Citation12], and AtCPD is a negative regulator in the BR signalling transduction pathway [Citation32]. The DEGs associated with JA biosynthesis were lower in gynoecious than in monoecious Jatropha inflorescences [Citation4].
qRT-PCR validation
To validate the RNA-seq data, 30% of the total DEGs (23 DEGs) identified by RNA-seq were tested by qRT-PCR (Supplementary Table S3). These genes were selected because of their expression changed many fold in GC_19 vs. DC_45 based on RNA-seq, including 7 upregulated genes and 16 downregulated ones. All of them were consistent with the same trend of up- or downregulation between the two different expression analysis platforms (Supplementary Figure S2). The correlation of the two expression measurements was 0.89 between these 23 genes (R2 = 0.89), thereby confirming that both methods achieved consistent results. Taken together, we suggest that a high level of BR could improve the fruit yield, whereas the upregulation of GA and JA might decrease the fruit yield in J. curcas. Recently, we reported that exogenous GA could inhibit the endogenous GA biosynthesis and signaling pathways to improve the female flowers in J. curcas [Citation33]. However, the DEGs associated with JA signaling transduction pathway were up-regulated following GA3 application to contribute to the pollen development process. In further study, a strategic approach will be to investigate the concentration of endogenous phytohormone after exogenous GA3 treatment in J. curcas [Citation33].
Conclusions
This study is a comprehensive sequencing effort and gene function analysis using the transcriptome data of high and low fruit yield Jatropha germplasm. Seven phenotypic traits of fruit and seed were investigated, and the transcriptome profiles across two samples with significant variation in fruit yield were obtained. A total of 76 DEGs were detected; six of them were significantly related to phytohormone signalling pathways, and high level of BR could improve the fruit yield, whereas the upregulation of GA and JA might decrease the fruit yield in J. curcas. Furthermore, we suggested that some genes might inhibit the initiation of female floral differentiation to decrease the fruit yield. A large number of SSR and SNP/Indel loci were obtained, which will improve our understanding about the genetic variation of some important traits in J. curcas. The obtained dataset is a potentially invaluable scientific resource to study the gene functions, which would facilitate the breeding of high-yielding J. curcas varieties.
Abbreviations | ||
SNP | = | Single Nucleotide Polymorphisms |
DEGs | = | Differential Expression Genes |
EST | = | Expressed Sequence Tag |
IAA | = | Auxin |
GA | = | Gibberellin |
BR | = | Brassinolide |
JA | = | Jasmonic acid |
GO | = | Gene Ontology database |
KEGG | = | Kyoto Encyclopedia of Genes and Genomes database |
FC | = | Fold Change |
SSR | = | Simple Sequence Repeats |
cDNA | = | Complementary DNA |
RNA | = | Ribonucleic Acid |
RNA-seq | = | RNA Sequencing |
qRT-PCR | = | Quantitative Real-time PCR |
Acknowledgments
The authors greatly acknowledge Prof. Xin-Sheng Hu for useful discussions during our preparation of this article.
Disclosure statement
The authors declare no conflict of interest.
Additional information
Funding
References
- Shabanimofrad M, Rafii M, Wahab PEM, et al. Phenotypic, genotypic and genetic divergence found in 48 newly collected Malaysian accessions of Jatropha curcas L. Ind Crop Prod. 2013;42:543–551.
- Fairless D. Biofuel: the little shrub that could-maybe. Nature. 2007;449:652–655.
- Grover A, Kumari M, Singh S, et al. Analysis of Jatropha curcas transcriptome for oil enhancement and genic markers. Physiol Mol Biol Plants. 2014;20:139–142.
- Chen MS, Pan BZ, Fu Q, et al. Comparative transcriptome analysis between gynoecious and monoecious plants identifies regulatory networks controlling sex determination in Jatropha curcas. Front Plant Sci. 2017;7:1953. DOI: 10.3389/fpls.2016.01953
- Natarajan P, Kanagasabapathy D, Gunadayalan G, et al. Gene discovery from Jatropha curcas by sequencing of ESTs from normalized and full-length enriched cDNA library from developing seeds. BMC Genomics. 2010 [2017 Oct 27];11:606. [14 p.] DOI: 10.1186/1471-2164-11-606
- King D, Roughgarden J. Multiple switches between vegetative and reproductive growth in annual plants. Theor Popul Biol. 1982;21:194–204.
- Morgan DC, Stanley CJ, Warrington IJ. The effects of simulated daylight and shade-light on vegetative and reproductive growth in kiwifruit and grapevine. J Hortic Sci Biotech. 2015;4:473–484.
- Gangwar M, Sood H, Chauhan RS. Genomics and relative expression analysis identifies key genes associated with high female to male flower ratio in Jatropha curcas L. Mol Biol Rep. 2016;43:305–322.
- Depuydt S, Hardtke CS. Hormone signalling crosstalk in plant growth regulation. Curr Biol. 2011;21:R365–R373.
- Lau S, Shao N, Bock R, et al. Auxin signaling in algal lineages: fact or myth?. Trends Plant Sci. 2009;14:182–188.
- Daviere J, Achard P. Gibberellin signaling in plants. Development. 2013;140:1147–1151.
- Choe S, Fujioka S, Noguchi T, et al. Overexpression of DWARF4 in the brassinosteroid biosynthetic pathway results in increased vegetative growth and seed yield in Arabidopsis. Plant J. 2001;26:573–582.
- Ishiguro S, Kawai-Oda A, Ueda J, et al. The defective in anther dehiscence1 gene encodes a novel phospholipase A1 catalyzing the initial step of jasmonic acid biosynthesis, which synchronizes pollen maturation, anther dehiscence, and flower opening in Arabidopsis. Plant Cell. 2001;13:2191–2209.
- Makwana V, Shukla P, Robin P, GA application induces alteration in sex ratio and cell death in Jatropha curcas. Plant Growth Regul. 2010;61:121–125.
- Ghosh A, Chikara J, Chaudhary DR, et al. Paclobutrazol arrests vegetative growth and unveils unexpressed yield potential of Jatropha curcas. J Plant Growth Regul. 2010;29:307–315.
- Liu S, Chen J, Li S, et al. Comparative transcriptome analysis of genes involved in GA-GID1-DELLA regulatory module in symbiotic and asymbiotic seed germination of Anoectochilus roxburghii (Wall.) Lind1 (Orchidaceae). Int J Mol Sci. 2015;16:30190–30203.
- Mortazavi A, Williams BA, McCue K, et al. Mapping and quantifying mammalian transcriptomes by RNA-Seq. Nat Methods. 2008;5:621–628.
- Silva TS, Richard N. Methods in Molecular Biology. Vol 1362, Visualization and differential analysis of protein expression data using R. New York (NY): Humana Press; 2016.
- Wang X, Lu P, Luo Z. GMATo: A novel tool for the identification and analysis of microsatellites in large genomes. Bioinformation. 2013;9:541–544.
- Farrer RA, Henk DA, MacLean D, et al. Using false discovery rates to benchmark SNP-callers in next-generation sequencing projects. Sci Rep. 2013;3:1512.[6 p.] DOI: 10.1038/srep01512
- Barbazuk WB, Emrich SJ, Chen HD, et al. SNP discovery via 454 transcriptome sequencing. Plant J. 2007;51:910–918.
- Zhang L, He L, Fu Q, et al. Selection of reliable reference genes for gene expression studies in the biofuel plant Jatropha curcas using real-time quantitative PCR. Int J Mol Sci. 2013;14:24338–24354.
- Schmittgen TD, Livak KJ. Analyzing real-time PCR data by the comparative C(T) method. Nat Protoc. 2008;3:1101–1108.
- Rowland LJ, Alkharouf N, Darwish O, et al. Generation and analysis of blueberry transcriptome sequences from leaves, developing fruit, and flower buds from cold acclimation through deacclimation. BMC Plant Biol. 2012;12:46. DOI: 10.1186/1471-2229-12-46
- Bian Y, Ballington J, Raja A, et al. Patterns of simple sequence repeats in cultivated blueberries (Vaccinium section Cyanococcus spp.) and their use in revealing genetic diversity and population structure. Mol Breeding. 2014;34:675–689.
- Chen MS, Pan BZ, Wang GJ, et al. Analysis of the transcriptional responses in inflorescence buds of Jatropha curcas exposed to cytokinin treatment. BMC Plant Biol. 2014;14:318. DOI: 10.1186/s12870-014-0318-z
- Hui WK, Yang YT, Wu GJ, et al. Transcriptome profile analysis reveals the regulation mechanism of floral sex differentiation in Jatropha curcas L. Sci Rep. 2017;7:1642 DOI:10.1038/s41598-017-16545-5
- Abdelgadir HA, Jager AK, Johnson SD, et al. Influence of plant growth regulators on flowering, fruiting, seed oil content, and oil quality of Jatropha curcas. S Afr J Bot. 2010;76:440–446.
- Pan B, Luo Y, Song L, et al. Thidiazuron increases fruit number in the biofuel plant Jatropha curcas by promoting pistil development. Ind Crop Prod. 2016;81:202–210.
- Sakamoto T, Phytohormones and rice crop yield: strategies and opportunities for genetic improvement. Transgenic Res. 2006;15:399–404.
- Huang J, Tang D, Shen Y, et al. Activation of gibberellin 2-oxidase 6 decreases active gibberellin levels and creates a dominant semi-dwarf phenotype in rice (Oryza sativa L.). J Genet Genomics. 2010;37:23–36.
- Mathur J, Molnár G, Fujioka S, et al. Transcription of the Arabidopsis CPD gene, encoding a steroidogenic cytochrome P450, is negatively controlled by brassinosteroids. Plant J. 1998;14:593–602.
- Hui W, Wang Y, Chen X, et al. Analysis of transcriptional responses of the inflorescence meristems in Jatropha curcas following gibberellin treatment. Int J Mol Sci. 2018;19(2):432. DOI: 10.3390/ijms19020432