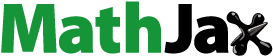
Abstract
Bioaerosol sampling is a critical step toward characterizing airborne pathogen exposure risks for many respiratory infectious diseases in human-to-human or human-to-animal outbreaks. The integrated performance-parameter design and sampling efficiency of aerosol-to-hydrosol samplers (cyclones) has been rarely published or studied. This review focuses on the determination of cyclone performance-parameter design and impact factors of the sampling efficiency, discusses their mechanisms of collection and describes their advantages and drawbacks. The crucial design parameters of cyclones in different applications are then discussed in detail. We also discuss how the aerosol-to-hydrosol sampling efficiency is intrinsically related to the performance parameters for bioaerosol cyclone design. In the final section of this review, scientific questions and challenges for future work are presented to encourage high collection efficiency design and high-quality cyclones.
Introduction
Bioaerosols are suspensions of airborne biological materials, including bacteria, viruses, proteins, spores, pollen, fungi, algae and cell fragments. They range in size from 0.01 to 100 µm [Citation1]. Pathogenic bioaerosols are a key reason that many emerging or re-emerging respiratory infectious diseases occur in human-to-human or human-to-animal outbreaks. For example, the viruses causing severe acute respiratory syndrome (SARS), H1N1 influenza, Middle East respiratory syndrome (MERS) and the novel coronavirus disease (COVID-19) can transmit through aerosols and cause thousands of deaths [Citation2–6]. Some transmissions through bioaerosols are fast and invisible, for example, there were at least 742 confirmed COVID-19 cases on the Diamond Princess cruise ship containing 3,700 people as the result of a superspreading event [Citation7]. Highly efficient bioaerosol samplers are necessary to capture these airborne biological particles with better viability to perform further biological analysis. Aerosol-to-hydrosol cyclones usually have high flow rates to collect large quantities of bioaerosols, after which they collect the bioaerosol as a concentrated liquid to create a cushioning effect and prevent violent impacts between the aerosol and collection media [Citation8,Citation9]. For monitoring a healthcare environment, an ideal cyclone should have the following characteristics: a high sampling efficiency, a fast and continuous sampling function, a high particle deposition efficiency, a proper particle size selection, a wide range of particle cut-off diameters, and a good microorganism viability [Citation1,Citation8,Citation10]. However, there are many performance parameters for cyclone design that affect the sampling efficiency of aerosol-to-hydrosol cyclones [Citation8]. The performance-parameter design and the sampling efficiency of aerosol-to-hydrosol cyclones have been rarely studied together. This review is focused on the performance-parameter design and the factors that affect the sampling efficiencies of bioaerosol cyclones. The collection mechanisms and the advantages and drawbacks of bioaerosol cyclones are described, and the crucial design parameters of cyclones in different applications are discussed in detail. Finally, this review addresses the design requirement for cyclones to achieve a high sampling efficiency.
Bioaerosol sampling
Category of current bioaerosol samplers
Bioaerosol sampling research has developed for over 200 years. Since their first introduction by Maddox in the 1800s [Citation11], bioaerosol samplers have advanced as the understanding of the aerodynamics and particle collection, and have improved and developed computational fluid dynamics methods [Citation1]. Macher [Citation12] stated that there are three key points related to the sampling performance: the inlet sampling efficiency for bioaerosol particles aspirated from the environment, the particle-capturing efficiency at the filter or laboratory culture, and the biological recovery efficiency for the viability of bioaerosol particles [Citation1]. From the 1900s to present, a large number of commercial bioaerosol sampling designs have been developed for different purposes, as summarized in .
Table 1. Categories of bioaerosol samplers.
Inertial samplers use gravity and external air flow to deposit biological particles onto a collection surface. These samplers can be either passive or active. Passive samplers have the advantage of not disturbing the surrounding air flow but losing smaller and lighter particles [Citation28,Citation29]. Active samplers include impactors, impingers and cyclones. For these samplers, smaller and lower-mass particles easily pass through a bent pipe and are collected by the medium, but larger and heavier particles hit the wall of the pipe and hence cannot traverse the bend due to inertial compaction. Furthermore, the impinger has a drawback in terms of collection losses from the evaporation of the collection liquid and the adhesion of the particles to the collection container wall [Citation26].
Sampling by filtration, in which the bioaerosol particles are trapped on fine fibres or porous membrane surfaces, is another way to collect particles. However, filters are less popular for bioaerosol particle collection because the bio-efficiency is low due to the desiccation of the pathogens and the adherence to the filter fibres [Citation28].
Cyclones
In recent applications, cyclones have been widely used as highly efficient samplers. King and McFarland [Citation15] showed that the culturability of Escherichia coli collected with a cyclone was about a factor of 100 higher than that collected with an impactor. They also found that real-time polymerase chain reaction (RT-PCR) showed E. coli identification was 100 times more concentrated by cyclone collection rather than impactor collection [Citation15]. Cyclones feature large air-flow rates, concentrated-liquid sampling processes, and better viabilities for biological particle collection [Citation17,Citation30].
In most cyclones, the bioaerosol enters the inlet and experiences a centrifugal force based on the shape of a spiral collection tube and the swirling air flow [Citation1,Citation8,Citation20,Citation31], as shown in . Within this airflow, the particles are acted upon by a centrifugal force proportional to their diameters, densities and speeds. McFarland et al. [Citation32] separated cyclones into two groups. They are wetted wall cyclones (WWCs) and batch wetted wall cyclones (BWWCs). WWCs use the centrifugal force to drive particles under inertia toward the liquid containers from an aerosol to a hydrosol [Citation28]. Cage et al. [Citation33] designed a WWC with a flow rate of 400 L/min and concentrated the sample in 12 mL of liquid. Another WWC, designed by Sigaev et al. [Citation34], had a flow rate of 60 L/min and concentrated the sample in 10 mL of hydrosol with a cut-off size of the collected particles of 0.5–1 µm (aerodynamic diameter, AD). Commercial WWCs include the SASS air collectors produced by Research International Inc. (Monroe, WA) with 265 L/min flow rates that concentrate the sample into 5 mL of liquid and the air samplers of Zaromb Research Corp (Burr Ridge, IL) with 300 L/min flow rates that concentrate the samples into 25 mL of liquid. Buchanan et al. [Citation35] developed an improved version of the WWC with a sampling flow rate of 950 L/min and a particle cut-off size of less than 1 µm (aerodynamic diameter, AD). Black & Shaw [Citation36] applied the liquid through a port at the air inlet slot and added a vortex finder at the cyclone body. The air flow rate was 900 L/min, and the pressure drop across the cyclone was 7 kPa. The collection efficiency was about 60% for bioaerosol particles in the AD size range of 1–7 µm. A phosphate buffered saline solution with Tween-20 surfactant was used as a collection buffer [Citation36]. Based on the design of Black and Shaw [Citation36], the WWC-1250 device was detailed by McFarland et al. [Citation32] with four modifications (). As reviewed in King et al. [Citation13], in addition to continuous WWCs, there are batch wetted wall cyclones (BWWC), where the particles are collected in liquid batches that are replaced on pre-set time intervals. Similar to the WWCs, BWWC systems are advantageous because they support long sampling periods, which allows for more concentrated samples and avoids the evaporation problems of impinging samplers [Citation18,Citation19].
Figure 1. Cyclone with swirling spiral pattern (Reused from Peng et al. [Citation31] with permission).
![Figure 1. Cyclone with swirling spiral pattern (Reused from Peng et al. [Citation31] with permission).](/cms/asset/b60772ad-7114-4a19-918b-6424c051051e/tbeq_a_1797529_f0001_c.jpg)
Figure 2. WWC-1250 device with four modifications by McFarland et al. [Citation32] [Reused with permission]. Red circled numbers: the aerosol inlet of the upgraded device forms a converging flow path into the cyclone,
the liquid is atomized before entering the inlet slot of this device,
the length of the cylindrically shaped cyclone body is shorter, and
the skimmer was changed to a straight shape rather than a divergent opening.
![Figure 2. WWC-1250 device with four modifications by McFarland et al. [Citation32] [Reused with permission]. Red circled numbers: 1 the aerosol inlet of the upgraded device forms a converging flow path into the cyclone, 2 the liquid is atomized before entering the inlet slot of this device, 3 the length of the cylindrically shaped cyclone body is shorter, and 4 the skimmer was changed to a straight shape rather than a divergent opening.](/cms/asset/7a610a63-dddf-49f6-9cae-ee9810511c05/tbeq_a_1797529_f0002_c.jpg)
Another type of cyclone is the virtual cyclone, which has a collecting probe operating with a major and a minor flow. Particles enter the cyclone and are carried by the airflow around a bend. By moving with the air, the smaller particles navigate the curve and are deposited on a collection filter. In contrast, the larger particles having greater inertia are carried into a different collection container ().
Figure 3. Virtual cyclone. Left: airflow in the virtual sampler (Reused from Haig et al. [Citation28] with permission). Right: schematic of a virtual cyclone (Reused from Song et al. [Citation20] with permission).
![Figure 3. Virtual cyclone. Left: airflow in the virtual sampler (Reused from Haig et al. [Citation28] with permission). Right: schematic of a virtual cyclone (Reused from Song et al. [Citation20] with permission).](/cms/asset/718a40bb-50e4-4ec2-977c-2439cbdd317b/tbeq_a_1797529_f0003_c.jpg)
Currently, a variety of conventional cyclones have been constructed and designed for different bioaerosol sampling purposes and working environments. The purpose of these designs is to achieve cyclones with high sampling efficiencies and to integrate them with detection systems for consistent sample delivery, automated operation and portability. Widely used commercial cyclones () include liquid-medium samplers such as the Coriolis®µ Biological Air Sampler with a 300 L/min flow rate (Bertin Technologies, Saint Quentin en Yvelines, France), the SASS 2300 with a 325 L/min flow rate (Research International, Inc., Monroe, WA, USA), the BioCapture650 with a 200 L/min flow rate (FLIR Systems, Inc. Wilsonville, OR, USA), the large-particle collecting cyclone S110 with a 10 L/min flow rate (Dekati, Kangasala, Finland), the Burkard cyclone sampler with a 16.6 L/min flow rate (Burkard manufacturing Co. Ltd, UK) and the BIO-Capturer-6 with an 80 L/min flow rate (Fuji Biotechnology, Inc., Hangzhou, China). Some cyclones perform particle-size selection before the air enters the inlet, and the particle-size selection head has five axial flow stages from 0.32 to 5.4 µm at a sampling flow rate of 28.3 L/min [Citation37]. Hsiao et al. [Citation38] developed a particle-size selection cyclone with a cut-off particle diameter from 0.04 to 11.3 µm. Tan et al. [Citation39] described the development of an automated electrostatic sampler that collected particles in a liquid reservoir and delivered them to sensing devices. Other reports have described a direct analysis system of airborne pathogens based on microfluidic enrichment [Citation40–42]. Another device, the MicroSampler, which is based on a two-phase fluid control in a microchip, provides real-time bioaerosol sensing [Citation43]. A recent study has focused on improvements in the real-time and automated operations of cyclones [Citation10]. However, few fully integrated bioaerosol sampling systems are capable of continuous real-time sampling. All of these cyclones have large particle cut-off diameters and low particle collection and aerosol-to-liquid efficiencies [Citation15, Citation44].
Figure 4. Recent commercial bioaerosol cyclones: Coriolis®µ (a), Research International SASS2300 (b), FLIR Systems BioCapture650 (c), Dekati S110 (d), Burkard C90M (e) and Fuji Biotechnology BIO-Capturer-6 (f).
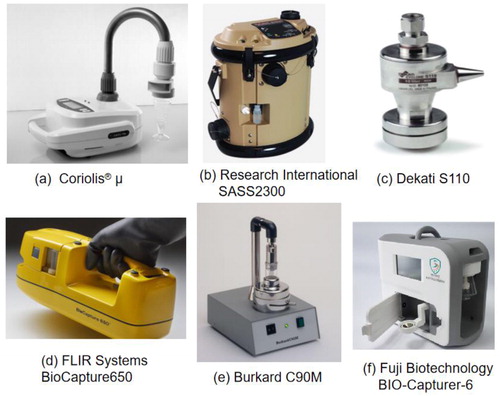
In most cyclones, larger particles are easier to collect than smaller particles. The air carries small particles at the bottom of the collection container, allowing them to escape out of the cyclone at the top of the outlet [Citation28]. Sampling losses may again arise from the evaporation of the collection liquid and the adherence to the wall of the collection container. The capturing efficiency of biological particles is affected by both the airflow rate and the cyclone geometry, including the size of the airflow inlet, the rate of the inlet airflow, and the geometric shape of the collection container. Apart from new mechanical geometric designs, the optimization of the cyclone’s sampling performance parameters are the most significant factors for achieving high-sampling-efficiency bioaerosol cyclones.
Crucial design and performance parameters of cyclones
Performance parameters
In the evaluation of the cyclone collection performance, the bioaerosol capturing efficiency is indispensable. There are no standards for evaluating the integrated performances for cyclones [Citation45]. However, there are three measurements used to describe the aerosol collection efficiency (). Most studies presumed that higher values of these three efficiencies can be used to evaluate the total sampling efficiency for cyclones [Citation1,Citation8]. First, the inlet efficiency (aerosol-to-aerosol efficiency, ηAA) is related to the ratio of the concentration of particles at the inlet entrance to that from the sampled aerosol. Second, the transport efficiency (aerosol-to-hydrosol efficiency, ηAH) is the ratio of the product of the particle concentration in a liquid or collection medium and the liquid flow rate to the product of the particle concentration and the air flow rate at the inlet. Finally, the deposition efficiency is the ratio of the concentration of particles in the liquid to the concentration of particles at the inlet entrance [Citation32]. In most studies on cyclone performance, improvements and developments were achieved through either simulations or experiments, but not through their integration [Citation46].
Figure 5. Venn diagram of the three cyclone performance parameters associated with the inlet efficiency, transport efficiency, and deposition efficiency. (1) Yellow: the common factors associated with all parameters. These are the sampled air-flow rate, particle concentration in the air stream, and particle size. (2) Blue: common factors between the inlet and deposition efficiencies. These are the particle concentrations in the air at the inlet. (3) Pink: common factors between the sampling and deposition efficiencies. These are particle concentrations in the liquid medium. (4) Green: common factors between the inlet and transport efficiencies. These are sampled with the air flow rate [Citation8].
![Figure 5. Venn diagram of the three cyclone performance parameters associated with the inlet efficiency, transport efficiency, and deposition efficiency. (1) Yellow: the common factors associated with all parameters. These are the sampled air-flow rate, particle concentration in the air stream, and particle size. (2) Blue: common factors between the inlet and deposition efficiencies. These are the particle concentrations in the air at the inlet. (3) Pink: common factors between the sampling and deposition efficiencies. These are particle concentrations in the liquid medium. (4) Green: common factors between the inlet and transport efficiencies. These are sampled with the air flow rate [Citation8].](/cms/asset/fac1b9b3-ae65-4be9-83a7-99f5cecfae7a/tbeq_a_1797529_f0005_c.jpg)
Experimental method
Generally, the air flow in a cyclone is too complex and turbulent to calibrate accurately in a laboratory set-up. Moreover, there are no international standards for testing the sampling efficiencies of cyclone bio-samplers or measuring the flow structures. Then, determining effective geometries of the devices is difficult. Many previous studies used wet-lab experimental data to evaluate the cyclone performance based on these three ratios [Citation17,Citation20,Citation47–50]. In some experiments, inactive microorganism simulants, such as polystyrene latex (PSL), were applied to test the aerosol-to-hydrosol collection efficiencies of cyclones [Citation15,Citation51,Citation52]. McFarland et al. [Citation32] defined the aerosol-to-hydrosol efficiency to be the intrinsic system of the WWC performance parameter with the detection of polystyrene latex spheres. They showed an aerodynamic diameter (AD) cut-off of 1.2 µm and an average collection efficiency of 90% over the AD size range of 2–10.2 µm. In addition, the cyclone performance evaluation with biological particles is a critical performance characterization. A previous study showed that the collection (aerosol-to-hydrosol) efficiency of a cyclonic swirling bioaerosol sampler for an aerosol cut-off size larger than 0.7 μm was 50%, and for that larger than 2 μm was 80% [Citation52]. In another case, the sampling efficiency was tested for two new cyclone-based bioaerosol samplers. PSL particles ranging from 0.5 to 10 μm were used as the test aerosols for acquiring sampling efficiencies. They found that the capture (aerosol-to-hydrosol) efficiency was 70%–80% for aerosol particles larger than 5 μm [Citation53]. In another study of a designed BWWC, the collection efficiency was 51% for 1 µm PSL and 55% for 3 µm PSL [Citation18].
Although the above results were not the same, the overall analytical method for determining the sampling efficiency was similar. Whether calling it sampling or collection or capturing efficiency, all of the previous studies examined the single aerosol-to-hydrosol efficiencies of cyclones based on one or two factors. No integrated evaluations for aerosol-to-aerosol, aerosol-to-hydrosol and deposition efficiencies have been performed. Time-consuming and expensive wet-lab experiments were performed using thousands of factor combinations, including the particle size, flow rate, pressure drop and movement dynamics, which were related to the sampling efficiency to determine the optimal combination by trial-and-error approaches. Additionally, the one-by-one testing of each parameter in a biological lab may be prone to evaluation biases, leading to a failure to obtain comprehensive results and to compare the sampling efficiencies between different bio-sampler designs. Hence, more comprehensive considerations using theoretical models are needed to evaluate many different influence factors.
Numerical design
Numerical simulations are useful means to study cyclones having a large number of influence factors and to optimize the integrated set of parameters to attain a high sampling efficiency and a better geometric design [Citation54]. Good predictive models, whether empirical, semi-empirical, or mathematical, can be used to design new cyclones for new applications or to improve existing designs [Citation30]. As reviewed by Zhao [Citation54], historically, Beekmans & Kim [Citation55] developed a quadratic response surface model (RSM) to evaluate the cyclone efficiency. In their model, the Reynolds number (Re) and Stokes number (Stk) were included, but the effects of the cyclone geometric parameters were not involved. In later studies, the Stokes and Reynolds numbers are two indicators that must be evaluated in the design of geometrically similar cyclones. They are related to the density of the liquid, aerodynamic particle diameter, viscosity, air flow rate at the inlet, air density, and inlet slot width, which are all related to sampling efficiency [Citation8,Citation54].
Computational fluid dynamics (CFD) has become a widely accepted design tool for research and development in fluid mechanics over the last decade. It can be used to optimize cyclone performance through modifications to the geometric design and different operational performance parameters [Citation56–59]. CFD models provide more details of the flow structures and particle behaviors related to the sampling efficiency, and they are crucial factors for guiding the geometric design parameters, including the sampling inlet, transport, and deposition of a cyclone. For example, Hu and McFarland [Citation16] redesigned the geometry and increased the flow rate of an earlier cyclone [Citation36] (). Previous CFD evaluations of the sampling efficiency were based on several single point measurements for each parameter using trial-and-error approaches [Citation60–62]. Many CFD models have been used and optimized to explore the parameter space of cyclones, which includes the flow rates, collection characteristics and pressure drop, liquid film thickness and width, liquid medium, and material for the liquid collector. All of these parameters were consistent with the experimental results [Citation63, Citation57,Citation64,Citation65]. For example, Wang et al. [Citation62] focused on reducing the pressure drop by adding a stick to the flow field of a cyclone. Another simulation model showed the strong effect of the geometric swirl number on the mean flow characteristics for cyclones [Citation61].
Figure 6. Modifications in aerosol-to-hydrosol cyclone based on CFD simulations (Reused from Hu & McFarland [Citation16] with permission): narrowing the rectangular entrance slot,
narrowing the shape of air-flow inlet,
adding water injection at the top of cyclone inlet,
changing to a rectangular cyclone body and
re-designing the shape of the cyclone skimmer in the rectangular region.
![Figure 6. Modifications in aerosol-to-hydrosol cyclone based on CFD simulations (Reused from Hu & McFarland [Citation16] with permission): 1 narrowing the rectangular entrance slot, 2 narrowing the shape of air-flow inlet, 3 adding water injection at the top of cyclone inlet, 4 changing to a rectangular cyclone body and 5 re-designing the shape of the cyclone skimmer in the rectangular region.](/cms/asset/97bd9991-7056-4090-8db0-5b68fdacb0ff/tbeq_a_1797529_f0006_c.jpg)
In the previous CFD simulation examples listed above, each point measurement corresponded to a single factor or parameter in a numerical model, and wet-lab experiments used an exhaustive method to evaluate the optimal parameter values of a cyclone. However, most geometric designs, aerosol flow structures, particle concentrations, and movement dynamics are correlated to each other. Many factors in the theoretical formulas must be considered and optimized in an integrated system under various restricted conditions. In some previous studies, empirical models were used based on the understanding of the flow structure and the experimental data in the cyclone to predict the particle dynamics and flow structure and to analyse the design parameters of the cyclone to develop cyclone modifications that promote higher collection efficiencies [Citation66–68]. These models based on finite-element methods are able to calculate the particle collection efficiency, cut-point particle size, pressure drop, and different geometries of cyclones.
Although several numerical simulations have been performed by CFD, they have some limitations, including the modeling reliability, the CPU processing ability, and mesh generation difficulties. The actual systems being simulated were usually complex, and the CFD simulations were often simplified, such as using simplified physical models, mathematical models, and boundary conditions, which may have affected the reliability of the simulations [Citation69]. The quality of the CFD numerical solutions strongly depends on the type of turbulence model applied to the continuous phase flow [Citation70]. This is another possible uncertainty affecting the calculating accuracy arising from the choice of the mathematical model. Currently, CFD can achieve many functions, and some software provides user-defined function assistance. However, there are still some complex cases that cannot be modeled, and programming is needed for simulation accuracy [Citation46]. Most CFD models include a large number of factors. Such complicated simulations require long CPU processing times and may result in data divergence [Citation71]. In addition, CFD simulations require more effort in the pre-processing for mesh generation. This is especially significant for simulating a complex cyclone model. Inadequate mesh generation, such as improperly selected mesh ratios and sizes, may result in uncertainties of the CFD modeling or divergent results [Citation72]. Recently, a meshless approach based on the LBM (Lattice‒Boltzmann Method) was employed for simulations. The concept of discrete grids was replaced by a lattice in the LBM. Furthermore, instead of solving Navier–Stokes equations like other CFD methods, this method calculates the streaming and collisions to simulate the motion of the whole fluid and predicts the hydrodynamics by tracking the probability distribution function to an equilibrium state. The greatest advantage of the LBM is its high efficiency for parallel computing [Citation73]. Hence, the LBM is considered to be more efficient, and highly recommended for the simulation design of complex geometric structures. In addition, CFD methods have been known to be macro-scale simulations, and thus, they have limitations for micro-scale calculations, such as small-size particles at the nano or micrometer scales, including bacteria, viruses, or fungi suspended in air. These small biological particles can become suspended in air by gravitational, Brownian and interaction forces, and they also have a penetration rate under air flow. Recently, the LBM has been shown to be a meso-scale simulation method with good parallelism, robustness, clear phase boundaries, and fast convergence for theoretical and computational approaches [Citation73]. However, the recent application of the LBM in fluid simulations only involved one or two-dimensional analysis, including indoor air or solid/liquid binary-phase systems [Citation39,Citation74-76]. Although CFD models have been widely used recently in the dynamic simulations of personal-inhalable bioaerosols and for risk analysis of infectious airborne diseases, improved three-dimensional-phase, accurate, and fast-caulculated LBM models with clear boundaries will be imperative to simulate the integrated parameters in triple-phase systems, including air, particles and liquid media, and to guide the optimization of bioaerosol cyclone design with a highest sampling efficient selection in the future study.
Determining design parameters for high bio-efficiency of cyclones
To reach the ultimate goal of an ideal cyclone, the bio-efficiency is always the most important aspect of bioaerosol sampling. The three formulas for bio-efficiency (ηAA, ηAH, and DE) described above are appropriate for comparing a family of geometrically similar cyclones and can be applied for the modification of specific units in cyclone re-design under the same restricted conditions. In addition, convergent variables that are more comprehensive and wider scope associated with these parameters are needed to provide performance and design evaluations.
In recent studies, the design of the aerosol and liquid sampling inlet device with a proper air flow rate is essential for airborne particle collection. Haig et al. [Citation28] suggested that the inlet of the cyclone is better positioned horizontally to prevent sampling bias. Once the air sample is taken-in by the device, the path of the airborne particle flow should be as straight and direct as possible to prevent losses in the bends of the conducting tube. Furthermore, the width of the inlet entrance was found to be a critical parameter for a WWC [Citation16]. McFarland et al. [Citation32] re-designed a WWC with four modifications that enabled a particle AD range from 1 µm to 10 µm collection. The modifications of the upgraded device were the flow path, inlet slot, cyclone body length and liquid flow rate [Citation10].
Ideally, for high aerosol collecting efficiency, sampling inlet, transport and deposition of a cyclone are three main aspects that should be focused on when designing a sampling system. Each can be assessed based on the specific components (). Correlations evaluated from numerical predictions (three-dimensional-phase LBM module), experimental measurements (inactive bacteria aerosol simulated house), and field studies (natural ventilation) provide results with a high degree of confidence to aid in the optimization of the design parameters (). Integrating the data from the numerical simulations, wet-lab calibrations, and microbiological aerosol demonstrations would provide a comprehensive analysis for the many complex designing parameters to obtain an ideal, high-sampling, efficient cyclone.
Table 2. Listing of various geometric design units for the cyclone sampling system.
Future design and trend of cyclones
Need for real-time bioaerosol sampling and detection
With advances in technology, real-time bioaerosol sampling and online detection have become increasingly important for a wide variety of research communities and scientific questions [Citation45]. There is a need for improved, affordable, real-time, high-sampling-efficiency detection instruments that measure particle types at sufficiently high resolution with an accurate specificity. The combination of high-efficiency cyclone sampling and real-time bioaerosol sensing techniques, including fluorescence spectroscopy, microfluidic techniques or quantitative polymerase chain reaction (qPCR), will provide a fast response with adequate counting and discriminative results.
Need for improved links between numerical modelling and experiments
Cyclone behavior is complex and difficult to model. In recent years, many researchers have developed high-sampling-efficiency predictive models for cyclone performance, which provide insight into how cyclone performance is affected by the geometric design [Citation30]. Detailed experiments of new numerical modelling prediction insights will provide confirmed data for improvements such as an LBM model. Continued and increased communication between the modeling and experiments is encouraged so that cyclone development and monitoring efforts are focused on the collection of solid datasets that can most efficiently reduce numerical model uncertainties.
Need for database development
Open databases of the performance parameters for different types of cyclones should be developed for the techniques listed in . These should include as many bioparticle sizes as possible, aerosolization parameters and clearly reported analysis conditions. This database should open a wide range of scientific objective and cross communities, including a cyclone’s geometric design based on high-collecting efficiency optimization by data mining or artificial intelligence. Openly shared results and big-data platforms for cross-disciplinary interactions will provide more opportunities for cyclone research.
Conclusions
The development of well-designed cyclones with high sampling efficiencies, fast and continuous sampling functions, a wide range of particle cut-off diameters, and good microorganism viability has progressed considerably in recent years, with a broad range of techniques being established or under development. As indicated by the overview provided in this review, the aerosol-to-hydrosol efficiency is intrinsically related to the cyclone performance parameters that are useful for design. However, the challenges for the optimization of cyclone performance parameters are still far from being integrated for real-time sampling and detection to link numerical modelling and experiments, and to develop a database. These challenges will be advantageous for the further development and refinement of cyclone sampling efficiency research and geometric design, and they will continue to open new opportunities for routine bioaerosol monitoring and research.
Disclosure statement
No potential conflict of interest is reported by the authors.
Additional information
Funding
References
- Vincent JH. Aerosol sampling: science, standards, instrumentation and applications. J Environ Qual. 2007;3(38):48.
- Devaux CA, Mediannikov O, Medkour H, et al. Infectious disease risk across the growing human-non human primate interface: a review of the evidence. Front Public Health. 2019;7:305–305.
- Judson SD, Munster VJ. Nosocomial transmission of emerging viruses via aerosol-generating medical procedures. Viruses. 2019;11(10):940. [cited 2020 Apr 28]
- Mackenzie JS, Smith DW. COVID-19: a novel zoonotic disease caused by a coronavirus from China: what we know and what we don't [Published online ahead of print, 2020 Mar 17]. Microbiol Aust. 2020;41 [cited 2020 Jun 25]; MA20013.
- Wu Y, Shen F, Yao M. Use of gelatin filter and BioSampler in detecting airborne H5N1 nucleotides, bacteria and allergens. J Aerosol Sci. 2010;41(9):869–879.
- Xu S, Li X, Yang J, et al. Comparative pathogenicity and transmissibility of pandemic H1N1, Avian H5N1, and human H7N9 influenza viruses in tree shrews. Front Microbiol. 2019;10:2955–2955.
- Yuen K-S, Ye Z-W, Fung S-Y, et al. SARS-CoV-2 and COVID-19: The most important research questions. Cell Biosci. 2020;10:40–40.
- Baron PA, Kulkarni P, Willeke K. 2011. Aerosol measurement: principles, techniques, and applications. Hoboken, N.J: Wiley.
- Dirgo J, Leith D. Cyclone collection efficiency: comparison of experimental results with theoretical predictions. Aerosol Sci Technol. 1985;4(4):401–415.
- Cho YS, Hong SC, Choi J, et al. Development of an automated wet-cyclone system for rapid, continuous and enriched bioaerosol sampling and its application to real-time detection. Sens Actuators B Chem. 2019;284:525–533.
- Gregory PH. 1973. The microbiology of the atmosphere. 2nd ed. London: L. Hill.
- Macher J. 1999. Bioaerosols: assessment and control. Macher J, A. C. o. G. I. Hygienists, editors. Cincinnati, Ohio: ACGIH.
- King MD, Lacey RE, Pak H, et al. Assays and enumeration of bioaerosols-traditional approaches to modern practices. Aerosol Sci Technol. 2020;54(5):611–633. ):
- McDonagh A, Noakes CJ. Sampling efficiency comparison of four bioaerosol samplers and two particle counters. In: UNSPECIFIED The 8th International Symposium on Modern Principles for Air Monitoring and Biomonitoring; 2014 June 15–19; Marseille, France; 2014 [cited 2020 March 5].
- King MD, McFarland AR. Bioaerosol sampling with a wetted wall cyclone: cell culturability and DNA integrity of Escherichia coli bacteria. Aerosol Sci Technol. 2012;46(1):82–93.
- Hu S, McFarland AR. Numerical Performance Simulation of a Wetted Wall Bioaerosol Sampling Cyclone. Aerosol Sci Technol. 2007;41(2):160–168.
- Kesavan J, Schepers D, McFarland AR. sampling and retention efficiencies of batch-type liquid-based bioaerosol samplers. Aerosol Sci Technol. 2010;44(10):817–829.
- King MD, Thien BF, Tiirikainen JS, et al. Collection characteristics of a batch-type wetted wall bioaerosol sampling cyclone. Aerobiologia. 2009;25(4):239–247.
- Yooseph S, Andrews-Pfannkoch C, Tenney A, et al. A metagenomic framework for the study of airborne microbial communities. PLoS One. 2013;8(12):e81862
- Song J, Zhang Y, Zhu D, et al. Performance evaluation of virtual cyclone with various inlet and outlet dimensions. J Aerosol Sci. 2019;128:114–124.
- Torczynski JR, Rader DJ. The virtual cyclone: a device for nonimpact particle separation. Aerosol Sci Technol. 1997;26(6):560–573.
- Wally K. Miniature bioaerosal concentrator: progress through fy2000. Albuquerque, NM: Sandia National Laboratories; 2001. (Sandia Report, SAND-94-2589).
- Cox CS, Wathes CM. Bioaerosols handbook. March 29, 1995. Boca Raton and London: CRC Press; 1995.
- Ghosh B, Lal H, Srivastava A. Review of bioaerosols in indoor environment with special reference to sampling, analysis and control mechanisms. Environ Int. 2015;85:254–272.
- Bond DR, Russell JB. Protonmotive force regulates the membrane conductance of Streptococcus bovis in a non-Ohmic fashion. Microbiology. 2000;146(3):687–694.
- Han T, Mainelis G. Investigation of inherent and latent internal losses in liquid-based bioaerosol samplers. J Aerosol Sci. 2012;45:58–68.
- Mainelis G, Grinshpun SA, Willeke K. Collection of airborne microorganisms by electrostatic precipitation. Aerosol Sci. Technol. 1999;30(2):127–144.
- Haig CW, Mackay WG, Walker JT, et al. Bioaerosol sampling: sampling mechanisms, bioefficiency and field studies. J Hosp Infect. 2016;93(3):242–255.
- Hinds WC. Aerosol Technology properties, behavior, and measurement of airborne particles. New York, NY: John Wiley & Sons; 2012.
- Kenny LC, Thorpe A, Stacey P. A collection of experimental data for aerosol monitoring cyclones. Aerosol Sci Technol. 2017;51(10):1190–1200.
- Peng W, Hoffmann AC, Dries H, et al. Reverse-flow centrifugal separators in parallel: Performance and flow pattern. AIChE J. 2007;53(3):589–597. [2020 Jun 26]
- McFarland AR, Haglund JS, King MD, et al. Wetted wall cyclones for bioaerosol sampling. Aerosol Sci Technol. 2010;44(4):241–252.
- Cage BR, Gorman PG, Going JE, et al. Continuous emission monitoring system. US Patent 5,861,316. January 19, 1999.
- Sigaev GI, Tolchinsky AD, Sigaev VI, et al. Development of a cyclone-based aerosol sampler with recirculating liquid film: theory and experiment. Aerosol Sci Technol. 2006;40(5):293–308.
- Buchanan LM, Harstad JB, Phillips JC, et al. Simple liquid scrubber for large-volume air sampling. Appl Microbiol. 1972;23(6):1140–1144.
- Black RS, Shaw MJ. Development of the wetted wall cyclone collector. Paper presented at the Scientific Conference on Obscuration and Aerosol Research. U.S. Army Research, Development and Engineering Command. Edgewood Chemical Biological Center; 2002 Jun 25 [cited 2020 Jan 06]; Edgewood, MD; 2002.
- Smith WB, Wilson J, Rufus R, et al. A five-stage cyclone system for in situ sampling. Environ Sci Technol. 1979;13(11):1387–1392.
- Hsiao T-C, Chen D-R, Li L, et al. Development of a multi-stage axial flow cyclone. Aerosol Sci Technol. 2010;44(4):253–261.
- Tan M, Shen F, Yao M, et al. Development of an automated electrostatic sampler (AES) for bioaerosol detection. Aerosol Sci Technol. 2011;45(9):1154–1160.
- Jing W, Zhao W, Liu S, et al. Microfluidic device for efficient airborne bacteria capture and enrichment. Anal Chem. 2013;85(10):5255–5262.
- Kang JS, Lee KS, Kim SS, et al. Real-time detection of an airborne microorganism using inertial impaction and mini-fluorescent microscopy. Lab Chip. 2014;14(1):244–251.
- Liu Q, Zhang Y, Jing W, et al. First airborne pathogen direct analysis system. Analyst. 2016;141(5):1637–1640.
- Choi J, Hong SC, Kim W, et al. Highly enriched, controllable, continuous aerosol sampling using inertial microfluidics and its application to real-time detection of airborne bacteria. ACS Sens. 2017;2(4):513–521.
- Lighthart B, Tong Y. Measurements of total and culturable bacteria in the alfresco atmosphere using a wet-cyclone sampler. Aerobiologia. 1998;14(4):325–332.
- Huffman JA, Perring AE, Savage NJ, et al. Real-time sensing of bioaerosols: review and current perspectives. Aerosol Sci Technol. 2020;54(5):465–495.
- Tian L, Ahmadi G. Particle deposition in turbulent duct flows-comparisons of different model predictions. J Aerosol Sci. 2007;38(4):377–397.
- Duquenne P, Coulais C, Bau S, et al. Performances of the BC-112 NIOSH cyclone for the measurement of endotoxins in bioaerosols: A study in laboratory conditions. J Aerosol Sci. 2018;116:92–105.
- Hubbard JA, Haglund JS, Tucker D, et al. Experimental study of heat and mass transfer in a family of wetted-wall bioaerosol sampling cyclones. Presentation at the U.S. Army Research, Development and Engineering Command Scientific Conference on Obscuration and Aerosol Research; June 23, 2009.
- Noh S-Y, Heo J-E, Woo S-H, et al. Performance improvement of a cyclone separator using multiple subsidiary cyclones. Powder Technol. 2018;338:145–152.
- Willeke K, Lin X, Grinshpun SA. Improved aerosol collection by combined impaction and centrifugal motion. Aerosol Sci Technol. 1998;28(5):439–456.
- Langer V, Hartmann G, Niessner R, et al. Rapid quantification of bioaerosols containing L. pneumophila by Coriolis® μ air sampler and chemiluminescence antibody microarrays. J Aerosol Sci. 2012;48:46–55.
- Wubulihairen M, Lu X, Lee PKH, et al. Development and laboratory evaluation of a compact swirling aerosol sampler (SAS) for collection of atmospheric bioaerosols. Atmos Pollut Res. 2015;6(4):556–561.
- Su W-C, Tolchinsky A D, Chen B T, et al. Evaluation of physical sampling efficiency for cyclone-based personal bioaerosol samplers in moving air environments. J Environ Monit. 2012;14(9):2430 doi:10.1039/c2em30299c.
- Zhao B. Development of a dimensionless logistic model for predicting cyclone separation efficiency. Aerosol Sci Technol. 2010;44(12):1105–1112.
- Beeckmans JM, Kim CJ. Analysis of the efficiency of reverse flow cyclones. Can J Chem Eng. 1977;55(6):640–643.
- Boysan F, Ayers WH, Swithenbank JA. A fundamental mathematical modelling approach for cyclone design. Trans. Inst. Chem. Engin. 1982;60:222–230.
- Griffiths WD, Boysan F. Computational Fluid Dynamics (CFD) and empirical modeling of a number of cyclone samplers. J Aerosol Sci. 1996;27(2):281–304.
- Shakeel Syed M, Rafeie M, Henderson R, et al. A 3D-printed mini-hydrocyclone for high throughput particle separation: application to primary harvesting of microalgae. Lab Chip. 2017;17(14):2459–2469.
- Zhou LX, Soo SL. Gas-solids flow and collection of solids in a cyclone separator. Powder Technol. 1990;63(1):45–53.
- Erdal FM, Shirazi SA. Local velocity measurements and computational fluid dynamics (CFD) Simulations of swirling flow in a cylindrical cyclone separator. Journal of Energy Resources Technology. 2004;126(4):326–333. [Jun 23 2020].
- Hoekstra AJ, Derksen JJ, Van Den Akker HEA. An experimental and numerical study of turbulent swirling flow in gas cyclones. Chem Eng Sci. 1999;54(13–14):2055–2065.
- Wang J-J, Wang L-Z, Liu C-W. Effect of a stick on the gas turbulence structure in a cyclone separator. Aerosol Sci Technol. 2005;39(8):713–721.
- Gimbun J, Chuah TG, Choong TSY, et al. Prediction of the effects of cone tip diameter on the cyclone performance. J Aerosol Sci. 2005;36(8):1056–1065.
- Ataki A, Bart H-J. The Use of the VOF-Model to Study the Wetting of Solid Surfaces. Chem Eng Technol. 2004;27(10):1109–1114.
- Gu F, Liu CJ, Yuan XG, et al. CFD simulation of liquid film flow on inclined plates. Chem Eng Technol. 2004;27(10):1099–1104.
- Kim CH, Lee JW. A new collection efficiency model for small cyclones considering the boundary-layer effect. J Aerosol Sci. 2001;32(2):251–269.
- Lapple CE. Processes use many collector types. Chem. Engr. 1955;58(5):144–151.
- Moore ME, McFarland AR. Performance modeling of single-inlet aerosol sampling cyclones. Environ Sci Technol. 1993;27(9):1842–1848.
- Wang X, Yang Z, Duan L. About some optimization design problems of ANSYS. Journal of Plasticity Engineering. 2007;14(6):181–184.
- Shalaby H, Wozniak K, Wozniak G. Numerical calculation of particle-laden cyclone separator flow using les. Eng Appl Comput Fluid Mech. 2008;2(4):382–392.
- Lee I-B, Bitog JPP, Hong S-W, et al. The past, present and future of CFD for agro-environmental applications. Computers & Electronics in Agriculture. 2013;93:168–183.
- Sajjadi H, Tavakoli B, Ahmadi G, et al. Computational fluid dynamics (CFD) simulation of a newly designed passive particle sampler. Environ Pollut. 2016;214:410–418.
- Yuan M, Qiao X. Comparison of LBM and CFD for hydrodynamic simulation of cross-flow tube bundle. Chinese J Process Eng. 2018;18(1):35–40.
- Ding L, Fung JLS, Seepana S, et al. Numerical study on particle dispersion and deposition in a scaled ventilated chamber using a lattice Boltzmann method. J Aerosol Sci. 2012;47:1–11.
- Jafari S, Salmanzadeh M, Rahnama M, et al. Investigation of particle dispersion and deposition in a channel with a square cylinder obstruction using the lattice Boltzmann method. J Aerosol Sci. 2010;41(2):198–206.
- Yeomans JM, Wagner AJ. Lattice Boltzmann simulations of complex fluids. IMA J Manage Math. 2000;11(4):257–267. [October 01 2000].