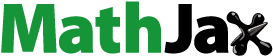
Abstract
The 16S rRNA amplicon sequencing technique is a key aspect of studies of microbial communities but does not provide direct evidence of a community’s functional capabilities. This work aimed to assess the structure of the uncultured bacterial communities from two locations in Lake Tuz in Turkey to provide information on their roles in the lake ecosystem. The most abundant phyla in the lake water were Firmicutes (84%) for lake sample site 1 (TG1), 70% for lake sample site 2 (TG2), Fusobacteria (9% for TG1, 22% for TG2) and Proteobacteria (6% for TG1, 7% for TG2). The most abundant genera were Romboutsia (45% for TG1, 35% for TG2), Clostridium sensu stricto 1 (8% for TG1, 8% for TG2), Cetobacterium (9% for TG1, 22% for TG2) and Photobacterium (2% for TG1, 3% for TG2). PICRUSt constitutes a novel bioinformatics tool to establish profiles for bacterial protein functions based on metagenomic 16S rRNA data for a community of unculturable bacteria. PICRUSt also provides information on whole-community metabolic functions related to adaptation, bioremediation potential and the ability of various groups of microorganisms to survive in highly saline water. The overall results provide an effective strategy for assessing the metabolic capacities of microbes in situ in a high-salt aquatic environment such as Lake Tuz and the potential of these microbes to serve as bioremediation agents. This approach provides useful insights into predictive metagenomics of an unculturable microbial community for which only marker-gene surveys are currently available.
Introduction
Natural hypersaline environments such as inland seas, lakes, salt marshes and marine salterns as well as man-made evaporation ponds are found on all continents. Thalassohaline brine lakes are formed by evaporation of seawater and have a near neutral pH and ionic composition like that of seawater, with NaCl as the main component. In contrast, athalassohaline brine lakes form as a consequence of local geology, and Mg2+ and Ca2+ are the predominant divalent cations. The defining characteristic of hypersaline habitats is their large diversity of salt-loving microbes. The concentrations of salts in hypersaline habitats vary from above 15% to saturation, and pH ranges from 6 to 11 [Citation1].
Lake Tuz (in Turkish, Tuz Gölü) is a thalassohaline brine lake (salinity, 32.4%) located in the arid central plateau of Turkey, 105 km northeast of Konya and 150 km south/southeast of Ankara (). It is the second largest lake in Turkey. To date, little effort has been made to characterize the community of salt-tolerant microbes of Lake Tuz toward the goal of predicting the contribution of individual bacterial species to the ecosystem based on 16S rRNA gene sequences. Several studies have used both in vitro and classical microbe-identification approaches as well as molecular methods to determine the microbial diversity of aquatic environments [Citation2–6]. Our current study complements the work of Mutlu et al. [Citation4], who found that Lake Tuz is dominated by Archaea and Bacteria. However, our findings focus only on bacteria that predominate in Lake Tuz with respect to their molecular features that allow salt tolerance and their potential use for bioremediation of xenobiotics. Although a large breadth of bacterial diversity can be determined by analysing 16S rRNA gene sequences, this does not provide compelling information concerning the contributions made by the various bacterial species to the overall aquatic ecosystem. Regardless of this shortcoming, 16S rRNA gene amplicon sequencing, compared with conventional approaches, is more efficient at determining all the various aspects of microbial community structure and functions. Moreover, the use of primer pairs targeting the hypervariable region of the 16S rRNA gene may bias the estimates of bacterial abundance toward certain phyla [Citation7]. This can be mitigated by using primer pairs that target the V3–V4 hypervariable regions of the 16S rRNA gene. Such primer pairs have been reported to capture the true range of bacterial phyla and thus are effective for Illumina MiSeq analyses of microbial communities that inhabit complex aquatic environments [Citation8,Citation9]. The advent of innovative molecular methods such as metagenomics using high-throughput Illumina technology and pyrosequencing has enabled the routine, comprehensive characterization of microbial communities using culture-independent methods. These techniques have reduced the cost and time required for sequencing and generate numerous sequences for each sample [Citation10]. Moreover, these new methods can identify the predominant bacteria in a sample and permit the discovery of minor taxa in the bacterial community; importantly, minor taxa may play substantive roles in any given bacterial community or, if not, the minor taxa may be relics of an ancient ecosystem that underwent a shift in environmental conditions [Citation11]. Analysis of the 16S rRNA gene amplicon remains the standard method for culture-independent studies of microbial diversity, and this approach has made it clear that there exist enormous numbers of unculturable (and therefore uncharacterized) microbes whose metabolic capacities, life cycles and relative ecosystem impact remain largely undefined.
Figure 1. Map showing Lake Tuz, where water samples were collected for the study (https://www.worldatlas.com/maps/turkey).
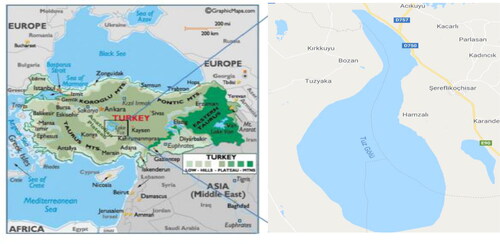
Recently, several 16S rRNA gene studies have extended our ability to infer the functional contribution of individual bacterial community members by mapping a subset of abundant 16S rRNA sequences to their nearest sequenced reference genome [Citation12–14]. Currently, predicting microbial functions from 16S rRNA gene sequencing data is a common alternative to shotgun metagenomic approaches. The computational tool PICRUSt (Phylogenetic Investigation of Communities by Reconstruction of Unobserved States) predicts functional metagenomic content based on the frequency of detected 16S rRNA gene sequences corresponding to genomes in regularly updated, functionally annotated genome databases [Citation15]. Therefore, the clustering of 16S rRNA sequences into operational taxonomic units (OTUs) or amplicon sequence variants enables direct comparison with 16S rRNA gene sequences from reference genomes in a database [Citation12,Citation14]. PICRUSt uses reference phylogenetic trees of 16S rRNA gene amplicons to infer metabolic information in genomes included in databases such as the Kyoto Encyclopedia of Genes and Genomes (KEGG) and thereby allow prediction of metagenomic function.
Herein, we report the physicochemical properties of lake water and bacterial species composition of the bacterial community at two sites in Lake Tuz and predict the contributions of bacteria to the lake ecosystem using a 16S rRNA amplicon sequencing approach. The bacterial communities from Lake Tuz were directly isolated from each sample without cultivation. The most abundant phyla were Firmicutes, Fusobacteria and Proteobacteria, and we predicted functional genes related to evolutionary adaptability and the potential for bioremediation. Our results will support future research on bioremediation carried out by potential resident microbes that produce previously unknown enzymes—for instance, new types of dehalogenases [Citation16–19] We note that detailed information on microbial communities is necessary for judging the biotechnological potential of individual bacterial species.
Materials and methods
Sample collection and analysis of physicochemical properties of bacterial species
Water samples were collected from two locations, termed TG1 and TG2, of the hypersaline Lake Tuz, at latitude 38°44′26 N and longitude 33°23′04 E and latitude 38°47′48 N and longitude 33°23′26 E, respectively, during the peak of the dry season in August 2018. Each sample was taken aseptically and transferred to a sterile plastic container, brought to the laboratory within 4 h and immediately stored at 4 °C for further analysis. The 16S rRNA amplicon analysis of all water samples was performed by First Base Laboratories-Apical Scientific (Malaysia). The physicochemical properties of the water samples were measured onsite using calibrated YSI equipment (YSI Inc., Yellow Springs, OH, USA). Aqueous Na+, K+, Ca2+, and Cl– were analysed according to the standard methods described by [Citation20].
DNA extraction
Water samples were filtered through a 0.45-µm-pore-size sterile mixed cellulose ester membrane (Fisher Scientific, Canada). The filters were cut into fragments (1 cm2) using sterilized scissors and were aseptically transferred into PowerBead tubes (MoBio, Carlsbad, CA, USA) for DNA extraction using the PowerSoil isolation kit (MoBio). The concentrated and purified DNA samples were assessed using a NanoDrop 8000 spectrophotometer (Thermo Scientific). The purified DNA was subjected to agarose gel electrophoresis and visualized with ethidium bromide. DNA was stored at –20 °C until further analysis.
PCR amplification, library preparation and illumina MiSeq sequencing
Bacterial composition was investigated by targeting the V3–V4 hypervariable regions of the 16S rRNA gene. Amplicons were generated using the bacteria-specific oligonucleotide primers 27 F (5′AGAGTTTGATCCTGGCTCAG3′) and 1492 R (3′GGTTACCTTGTTACGACTT5′) [Citation21] and amplification was carried out in a 50-µL reaction volume that contained purified genomic DNA (gDNA), 0.3 pmol of each primer, 1 µL DNA Taq polymerase (1 U/µL) (Sigma-Aldrich) and PCR buffer containing dNTPs and pure water (PCR/Amplification kit, Sigma-Aldrich). PCR was performed according to the following conditions: 1 cycle of 94 °C for 2 min (initial denaturation), 20 cycles of 98 °C for 10 s (annealing) and 51 °C 30 s and 68 °C for 1 min (extension) of the amplified DNA. Then, the amplicons were extracted from a 1.7% Tris-acetate-EDTA agarose gel and purified using a DNA Gel Extraction kit (Axygen Bioscience, Union City, USA) and quantified using QuantiFluor ST (Promega, Madison, USA). The eluted products were then used for library preparation using a 16S rRNA metagenomic sequencing library preparation protocol [Citation8]. Finally, purified library products were pooled in equimolar amounts and sequenced (paired-end, 2 × 300 bp) on an Illumina MiSeq platform by Apical Scientific (Malaysia). The quality and quantity of PCR products and libraries were assessed using the TapeStation 4200 Picogreen DNA quantification reagent and spectrophotometry. Neighboring taxa were revealed using BLASTn and analysed by pairwise sequence alignment to calculate nucleotide sequence identity using the EzBioCloude server (www.ezbiocloud.net) [Citation22]. Sequences with ≥97% identity were considered to belong to the same OTU.
Bioinformatics analyses
The raw reads were quantified using the sequence analysis program Trimmomatic (http://www.usadellab.org/cms/?page=trimmomatic) [Citation23]. All raw reads were quality filtered and checked using a minimum quality score of 25 over at least 75% of the sequence read, and low-quality sequences containing >10 consecutive low-quality base pairs, ambiguous bases, errors in barcode sequences or >2 nt mismatches from the primer sequences were discarded. Paired-end reads were merged using USEARCH software (version 11.0.667, http://www.drive5.com/usearch/). All sequences of <150 bp or >600 bp (sequenced on the MiSeq platform) were disregarded. Reads were then aligned with the SILVA 16S rRNA database (Release 132) and inspected for chimeric errors using VSEARCH v2.6.2. Chimeric sequences were identified and removed using UCHIME [Citation24]. Sequences were clustered into OTUs (i.e. ≥97% sequence identity) using UPARSE (version 11.0.667 http://www.drive5.com/uparse/) [Citation25], and the RDP Naïve Bayesian Classifier in the SLIVA database (http://wwwarbsilva.de) [Citation26] was applied to perform sequence-level taxonomic classification and identify OTUs. Spurious OTUs with only one read (singleton) or doubletons were detected upon further analysis using the clustering algorithm QIIME 1.9.1 (Quantitative Insights Into Microbial Ecology). To further explore patterns in alpha diversity, each of species richness (Chao estimator), species coverage (coverage) and species diversity (Shannon diversity index and Simpson index) was calculated and rarefaction analyses were performed using mothur software at the 97% identity level [Citation27].
Predictive metagenome analysis
The PICRUSt tool was used to predict the metagenome based on 16S rRNA amplicon datasets [Citation15]. The metagenomic functional potential of the Lake Tuz overall microbial community was investigated using 16S rRNA abundance data via PICRUSt v.2.1.3-b (https://github.com/picrust/picrust2/wiki) with default parameters [Citation28]. The 16S rRNA–based metagenome was functionally annotated using KEGG pathway functions (i.e. EC and KO [KEGG orthology] accessions) using hidden state prediction [Citation29]. Based on the abundances of 16S rRNA amplicon sequence variants and phylogenetic proximity to reference taxa with available genomes, amplicon sequence variants having a nearest sequences taxon index score of ≤2 were discarded from subsequent analyses.
Results and discussion
Physicochemical properties of Lake Tuz water samples
Lake Tuz is categorized as thalassohaline with ionic constituents Na+ and Cl– being the major ions followed by and the pH is >7. The physicochemical analyses revealed that Na+ and Cl– were the dominant ions in the water of Lake Tuz. presents results about the other physicochemical parameters of the water. Therefore, our physicochemical analyses of samples collected from Lake Tuz revealed that the water contains sufficient amounts of important ions to sustain the growth of halophilic bacteria.
Table 1. Physicochemical properties of water samples.
Bacterial diversity of Lake Tuz water samples
High-throughput sequencing with an Illumina MiSeq platform was used to explore the composition and structure of the bacterial community inhabiting Lake Tuz. A total of 302,308 reads, representing 133 OTUs, were produced by sequencing the V3–V4 region of 16S rRNA gene-based amplicons from the two sample locations, TG1 and TG2. All reads were ascribed to bacteria, and 155,242 reads were assigned to TG1 (67 OTUs) and 147,066 to TG2 (66 OTUs). The reads were clustered and distributed as follows: 67 OTUs for TG1 and 66 OTUs for TG2. The single OTU that was unique in TG1 belonged to class Coriobacteria.
The rarefaction curve in reached a plateau stage, implying that the sequencing depth was appropriate for a detailed description of the overall bacterial community. The sequence reads were used to examine whether alpha diversity differed between any two water samples and hence whether the bacterial composition differed between the samples (). Rarefaction curves and alpha-diversity indices were based on 97% (nucleic acid) sequence identity to provide information about bacterial richness and homogeneity in the lake.
Bacterial composition
A metagenomic analysis of Lake Tuz water was carried out by amplicon sequencing using next-generation technology to explore the diversity of microbial communities. Amplicon sequences of TG1 and TG2 were categorized into eight distinct phyla, namely Firmicutes, Fusobacteria, Proteobacteria, Acidobacteria, Cyanobacteria, Actinobacteria, Deinococcus-Thermus and Bacteroidetes, and one unclassified phylum (). Firmicutes was the major phylum of the bacterial communities, accounting for 84% of all bacterial phyla in TG1 and 70% in TG2. Fusobacteria and Proteobacteria were the second and third most represented phyla among the bacterial communities, accounting for 9% and 6% of TG1 and 22% and 7% of TG2, respectively ().
Figure 3. Bacterial phyla represented among the species identified at each of the sites TG1 and TG2.
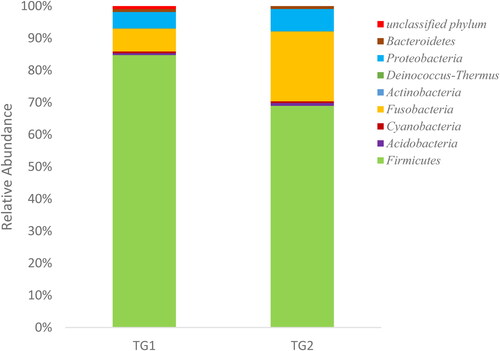
The phyla could be subdivided into classes () and genera (). Only 11 and 12 classes of bacteria were found in TG1 and TG2, respectively. Based on the average relative abundance, Clostridia (83% for TG1, 68% for TG2), Fusobacteria (9% for TG1, 22% for TG2) and Gammaproteobacteria (6% for TG1, 7% for TG2) were the three major classes of bacteria in the two samples (). For TG1 and TG2, 45% and 35%, respectively, of the sequences were members of genus Romboutsia, and 8% of the sequences belonged to the genus Clostridium sensu stricto 1 in each sample. The major proportion of OTUs belonged to unclassified bacteria, which comprised 39.5% of genera for TG1 and 36.7% for TG2. These were followed by genera Cetobacterium and Photobacterium, which accounted for 9% and 1%, respectively, of TG1, and for 22% and 1% of TG2 ().
Surprisingly, the dominant domain was Bacteria and not Archaea. A previous fluorescence in situ hybridization analysis revealed that Lake Tuz is dominated by Archaea (58%) and Bacteria (21.7%) [Citation4], and Jacob et al. [Citation30] found that Archaea is a major domain (50%) of the entire Dead Sea microbial community. Both Lake Tuz and the Dead Sea have high salinity (34% salt) and are typical of thalassohaline brine. The present study revealed that Firmicutes (70–84%), Fusobacteria (9–22%) and Proteobacteria (6–7%) are the three major bacterial phyla, whereas Mutlu et al. [Citation4] indicated that Lake Tuz is dominated by members of Bacteroidetes and represented by Haloquadratum spp. and Salinibacter spp. [Citation3]. Our current study also revealed that Bacteroidetes (0.6%) is less dominant in the water samples. Jacob et al. [Citation30] reported that the Dead Sea is dominated by two important phyla, namely Proteobacteria (55.9%) and Firmicutes (41.7%).
We found that Romboutsia () is the most highly represented bacterial genus in Lake Tuz (35–45% of species). According to Wang et al. [Citation31], Romboutsia is gram-positive and was commonly found in an alkaline-saline lake of an oilfield in China. There were varieties of bacteria present in hypersaline environments from previous and current studies. These results were attributed to the selection of sampling locations of the lake or sea and the time of the month of sampling, each of which helps determine the microbial diversity that changes owing to continuous fluctuations in the physicochemical properties of the water and also possibly to the method used for analyses [Citation32–34]. Since the bacterial composition was determined in samples collected only at specific sites, all studies have suggested that the results may not necessarily represent the entire lake but could serve as a baseline for future work owing to the fact that potential biases may be introduced. Concerning the present study, it is interesting to note that there are still unclassified phyla and that the unclassified bacteria constitute 36–39% of the total population. The inability to identify most of the bacterial species in Lake Tuz may be attributable to limitations on OTU sampling depth. Thus, a more robust OTU sampling depth may address this potential issue.
Prediction of functional profiles using 16S rRNA data
A functional profile was generated using PICRUSt to predict gene families based on bacterial metagenomes by modelling genes from 16S rRNA data derived from the generated OTUs and its reference genome database [Citation15]. To gain insight into the metabolic contribution of bacteria to the lake ecosystem, the prediction tool PICRUSt was used to determine the functional characteristics of the bacterial communities in the lake. The principal metabolic pathways were common to samples from both TG1 and TG2. and present the number of sequence reads of the predicted genes involved in pollutant degradation and adaptation to a high-salt environment, respectively. Bioremediation-related enzymes and other proteins that had evolved to facilitate microbial adaptation to a high-salt environment were found to be highly represented in both TG1 and TG2. The proportions of enzyme types were similar among samples from both sites, although this result must be confirmed by complementary studies involving isolation and characterization of bioremediation-competent bacteria.
Table 2. Enzyme classes for which cellular abundance was found to be related to their bioremediation potential.
Table 3. Metabolic enzymes for which cellular abundance was related to adaptation to high-salt conditions.
Genes predicted from Lake Tuz were found to encode haloacid, haloacetate and haloalkane dehalogenases, alkane 1-monooxygenase and other genes related to the metabolism of hydrocarbons, halogenated organic compounds and heavy metals, such as arsenic and mercury, suggesting the presence of naturally occurring halogenated compounds and/or possible contaminants or pollutants in the lake (). On the other hand, most of the bacterial species in the microbial community of Lake Tuz had genes that were predicted to be involved in the synthesis of halo-adaptation compounds such as ectoine, glycine-betaine, glutamate, trehalose and choline (). The unique cellular enzymatic machinery of halophilic microbes allows them to thrive in extreme saline environments, and this is related to the elevated content of acidic amino acids in bacterial proteins, which increase the net negative charge of a protein’s surface. Therefore, the bacteria effectively use hydrocarbons as their sole source of both carbon and energy, and thus such bacteria may be valuable bioremediation agents for the treatment of saline effluents and hypersaline waters contaminated with toxic compounds that are resistant to degradation [Citation17,Citation35].
Conclusions
Most of the bacterial phyla in Lake Tuz highlight the importance of these bacteria for degrading pollutants and thus these groups may be the focus of future studies. Very few reports have been published concerning pollutant degradation in saline/hypersaline environments with respect to microbial diversity and the prediction of metabolic and enzymatic systems using metagenomic amplicon sequencing [Citation5,Citation36–40]. This finding suggests that the bacteria inhabiting Lake Tuz could have potential applications in biotechnology as well. However, this study could serve as a foundation for future studies of the bioremediation of pollutants and heavy metals in extreme or polluted environments. A different approach such as shotgun metagenomic sequencing and/or quantitative PCR could be employed to identify pollutant-degrading bacteria at the species level.
Author contributions
HAO performed the investigation, analysed the data and wrote the original draft. MFE, MANM and AAAH provided resources and input on the experimental design, methodology and data analyses. FH and YK conceived and designed the study and acquired research funding. RAW revised and edited the manuscript and carried out formal data analysis. All authors read and approved the final manuscript.
Ethics statement
Samples were collected under a permit given by the Turkish Authority (ORMAN VE SU ĠġLERĠ BAKANLIĞI, reference no. 72784983-488.04-26581) dated February 3, 2016.
Disclosure of interest
No potential conflict of interest was reported by the authors.
Additional information
Funding
References
- Edbeib MF, Wahab RA, Huyop F. Halophiles: biology, adaptation, and their role in decontamination of hypersaline environments. World J Microbiol Biotechnol. 2016;32(8):1–23.
- Birbir M, Ogan A, Calli B, et al. Enzyme characteristics of extremely halophilic archaeal community in Tuzkoy Salt Mine, Turkey. World J Microbiol Biotechnol. 2004;20(6):613–621.
- Birbir M, Calli B, Mertoglu B, et al. Extremely halophilic Archaea from Tuz Lake, Turkey, and the adjacent Kaldirim and Kayacik salterns. World J Microbiol Biotechnol. 2007;23(3):309–316.
- Mutlu MB, Martínez-García M, Santos F, et al. Prokaryotic diversity in Tuz Lake, a hypersaline environment in Inland Turkey. FEMS Microbiol Ecol. 2008;65(3):474–483.
- Edbeib MF, Wahab RA, Kaya Y, et al. In silico characterization of a novel dehalogenase (DehHX) from the halophile Pseudomonas halophila HX isolated from Tuz Gölü Lake, Turkey: insights into a hypersaline-adapted dehalogenase. Ann Microbiol. 2017;67(5):371–382.
- Edbeib MF, Aksoy HM, Kaya Y, et al. Haloadaptation: insights from comparative modeling studies between halotolerant and non-halotolerant dehalogenases. J Biomol Struct Dyn. 2020;38(12):3452–3410.
- Tremblay J, Singh K, Fern A, et al. Primer and platform effects on 16S rRNA tag sequencing. Front Microbiol. 2015;6:771.
- Klindworth A, Pruesse E, Schweer T, et al. Evaluation of general 16S ribosomal RNA gene PCR primers for classical and next-generation sequencing-based diversity studies. Nucleic Acids Res. 2013;41(1):e1.
- Vargas-Albores F, Martínez-Córdova LR, Martínez-Porchas M, et al. Functional metagenomics: a tool to gain knowledge for agronomic and veterinary sciences. Biotechnol Genet Eng Rev. 2019;35(1):69–91.
- Huse SM, Dethlefsen L, Huber JA, et al. Exploring microbial diversity and taxonomy using SSU rRNA hypervariable tag sequencing. PLoS Genet. 2008;4(11):e1000255.
- Zaikova E, Goerlitz DS, Tighe SW, et al. Antarctic relic microbial mat community revealed by metagenomics and metatranscriptomics. Front Ecol Evol. 2019;7:1.
- Narayan NR, Weinmaier T, Laserna-Mendieta EJ, et al. Piphillin predicts metagenomic composition and dynamics from DADA2-corrected 16S rDNA sequences. BMC Genomics. 2020;21(1):56.
- Mise K, Fujita K, Kunito T, et al. Phosphorus-mineralizing communities reflect nutrient-rich characteristics in Japanese arable Andisols. Microbes Environ. 2018;33(3):282–289.
- Mallick H, Franzosa EA, McLver LJ, et al. Predictive metabolomic profiling of microbial communities using amplicon or metagenomic sequences. Nat Commun. 2019;10(1):3136.
- Langille MGI, Zaneveld J, Caporaso JG, et al. Predictive functional profiling of microbial communities using 16S rRNA marker gene sequences. Nat Biotechnol. 2013;31(9):814–821.
- Muslem WH, Edbeib MF, Aksoy HM, et al. Biodegradation of 3-chloropropionic acid (3-CP) by Bacillus cereus WH2 and its in silico enzyme-substrate docking analysis. J Biomol Struct Dyn. 2020;38(11):3432–3441.
- Oyewusi HA, Wahab RA, Kaya Y, et al. Alternative bioremediation agents against haloacids, haloacetates and chlorpyrifos using novel halogen-degrading bacterial isolates from the hypersaline lake Tuz. Catalysts. 2020;10(6):651.
- Heidarrezaei M, Shokravi H, Huyop F, et al. Isolation and characterization of a novel bacterium from the marine environment for trichloroacetic acid bioremediation. Appl Sci. 2020;10(13):4593.
- Muslem WH, Edbeib MF, Wahab RA, et al. The potential of a novel β-specific dehalogenase from Bacillus cereus WH2 as a bioremediation agent for the removal of β-haloalkanoic acids. Malays J Microbiol. 2017;13(4):298–307.
- Association APH, Association AWW, Federation WPC, et al. Standard methods for the examination of water and wastewater. Vol. 2. USA: American Public Health Association; University of Illinois at Urbana-Champaign, 1915.
- Lane DJ. 16S/23S rRNA sequencing. In: Stackebrandt E, Goodfellow M, editors. Nucleic acid techniques in bacterial systematics. New York: Wiley; 1991. p. 115–175.
- Yoon S-H, Ha S-M, Kwon S, et al. Introducing EzBioCloud: a taxonomically united database of 16S rRNA gene sequences and whole-genome assemblies. Int J Syst Evol Microbiol. 2017;67(5):1613–1617.
- Bolger AM, Lohse M, Usadel B. Trimmomatic: a flexible trimmer for Illumina sequence data. Bioinformatics. 2014;30(15):2114–2120.
- Edgar RC, Haas BJ, Clemente JC, et al. UCHIME improves sensitivity and speed of chimera detection. Bioinformatics. 2011;27(16):2194–2200.
- Edgar RC. UPARSE: highly accurate OTU sequences from microbial amplicon reads. Nat Methods. 2013;10(10):996–998.
- Quast C, Pruesse E, Yilmaz P, et al. The SILVA ribosomal RNA gene database project: improved data processing and web-based tools. Nucleic Acids Res. 2013;41(Database issue):D590–D596.
- Schloss PD, Westcott SL, Ryabin T, et al. Introducing mothur: open-source, platform-independent, community-supported software for describing and comparing microbial communities. Appl Environ Microbiol. 2009;75(23):7537–7541.
- Douglas GM, Maffei VJ, Zaneveld J, et al. PICRUSt2: an improved and extensible approach for metagenome inference. bioRxiv. 2019;10:672295.
- Louca S, Doebeli M. Efficient comparative phylogenetics on large trees. Bioinformatics. 2018;34(6):1053–1055.
- Jacob JH, Hussein EI, Shakhatreh MAK, et al. Microbial community analysis of the hypersaline water of the Dead Sea using high‐throughput amplicon sequencing. MicrobiologyOpen. 2017;6(5):e00500.
- Wang X, Wang X, Liu M, et al. Bioremediation of marine oil pollution by Brevundimonas diminuta: effect of salinity and nutrients. Desalin Water Treat. 2016;57(42):19768–19768.
- Tipayno SC, Truu J, Samaddar S, et al. The bacterial community structure and functional profile in the heavy metal contaminated paddy soils, surrounding a nonferrous smelter in South Korea. Ecol Evol. 2018;8(12):6157–6168.
- Hao B, Wu H, Li W, et al. Periphytic algae mediate interactions between neighbor and target submerged macrophytes along a nutrient gradient. Ecol Indic. 2020;110:105898.
- Jing Y, Zhang F, He Y, et al. Assessment of spatial and temporal variation of ecological environment quality in Ebinur Lake Wetland National Nature Reserve, Xinjiang, China. Ecol Indic. 2020;110:105874.
- Edbeib MF, Wahab RA, Huyop F. Characterization of an α-haloalkanoic acid–degrading Pseudomonas aeruginosa MX1 isolated from contaminated seawater. Biorem J. 2016;20(2):89–97.
- Khosrowabadi E, Huyop F. Screening and characterization of several 2,2-dicholoropropionic acid–degrading bacteria isolated from marine sediment of Danga bay and east coast of Singapore island. Biorem J. 2014;18(1):20–27.
- Mohanta TK, Mohanta YK, Mohanta N. Role of biotechnology in bioremediation. In: Handbook of Research on Uncovering New Methods for Ecosystem Management Through Bioremediation. Eds. Singh SO, Srivastava K. IGI Global, Pennsylvania, USA. 2015; 399–432.
- Parte SG, Mohekar AD, Kharat AS. Microbial degradation of pesticide: a review. Afr J Microbiol Res. 2017;11(24):992–1012.
- Akcay K, Kaya Y. Isolation, characterization and molecular identification of a halotolerant Bacillus megaterium CTBmeg1 able to grow on halogenated compounds. Biotechnol Biotechnol Equip. 2019;33(1):945–953.
- Nemati M, Edbeib MF, Hamid A, et al. Identification of putative Cof-like hydrolase associated with dehalogenase in Enterobacter cloacae MN1 isolated from the contaminated sea-side area of the Philippines. Malays J Microbiol. 2013;9(3):253–259.