Abstract
Cannabis sativa L. (cannabis) is a medicinal plant and has been used for many years for the treatment of epilepsy (EP), which is a common neurological disease. This study aimed to investigate the mechanism of cannabis action in EP, with emphasis on the leading compounds, targets and pathways. In this study, systematic pharmacology and bioinformatics approaches were employed to identify the active ingredients and potential targets of cannabis for treating EP. Furthermore, network construction, Gene Ontology (GO) and Kyoto Encyclopedia of Genes and Genomes (KEGG) enrichment analysis, and molecular docking were used to elucidate the mechanism of cannabis against EP. A total of 360 compounds were collected in this work. Among them, 226 active compounds and 116 predicted targets were obtained based on absorption, distribution, metabolism and excretion (ADME) screening and databases, respectively. Among the 226 active compounds, most were cannabinoids. The topological analysis showed that cannabinoid receptor 1, albumin and glycogen synthase kinase-3 beta (CNR1, ALB and GSK3B) were the key targets with intense interaction. The GO and KEGG enrichment analysis suggested cannabis might produce the antiepileptic effects by regulating many pathways, including calcium signalling pathway, MAPK signalling pathway, GABAergic synapse, etc. Additionally, cannabinol methyl ether (M54) might be the leading compound based on molecular docking. Consequently, this study holistically illuminates the active constituents and mechanism of cannabis based on network pharmacology, which contributes to searching for leading compounds and development of new drugs in the treatment of EP.
Supplemental data for this article is available online at https://doi.org/10.1080/13102818.2021.1942208 .
Introduction
Epilepsy (EP) is a nervous system disease with transient, stereotyped, recurrent and repetitive characteristics, which is caused by sudden abnormal discharge of brain neurons. According to the meta-analysis of international studies, the prevalence of EP is 6.4 per 1,000 people, and the annual incidence is 67.8 per 100,000 people [Citation1]. There are more than 50 million EP patients in the world, and the mortality rate is 3 times that of the general population [Citation2]. Due to the causes above, EP has increasingly attracted people’s attention recently. The pathogenesis of EP has been intensively studied, as relating signalling pathways include AK signalling pathway, BDNF signalling pathway, mTOR signalling pathway, TGF signalling pathway, calcium signalling pathway and MAPK signalling pathway [Citation3].
Cannabis sativa L. (cannabis), an annual dioecious herb of the genus Cannabis of the Cannabaceae family, has been extensively used as a traditional herbal medicine for the treatment of EP and neuropathic pain. It mainly contains various chemical components such as cannabinoids, flavonoids, terpenoids and so on, leading to a mass of pharmacological effects with a wide range. Most importantly, the cannabidiol (CBD) component of cannabis has been reported to treat EP [Citation4, Citation5]. However, other active components and mechanisms of cannabis therapy for EP have not been fully elucidated.
Network pharmacology, an attractive concept put forward by Hopkins [Citation6], integrates the ideas of systems biology and multi-directional pharmacology. It analyzes the action mechanism of drugs by constructing a complex network relationship of compound–target–disease. Recently, pharmacological research has shifted from the traditional search for a single target to multiple targets multi-level comprehensive network research [Citation7, Citation8]. It promotes further research on Traditional Chinese Medicine (TCM) and expands the development of modern drugs. In addition, molecular docking is a theoretical simulation method based on receptor characteristics and molecular interaction [Citation9]. It can predict the binding sites and affinity between drug molecules and target proteins, so as to verify the experimental results of network pharmacology [Citation10].
To explore the pharmacological and molecular mechanism of antiepileptic activity of cannabis at the overall level, the network pharmacology analysis was employed (). Firstly, the chemical database of cannabis was constructed, and the active compounds were screened according to SwissADME. Second, the targets of compounds and EP were predicted from five databases, involving Swiss TargetPrediction, PharmMapper, GeneCards, Therapeutic Target Database (TTD) and Online Mendelian Inheritance in Man (OMIM). The common targets were considered as the candidate antiepileptic targets of cannabis. Furthermore, networks were constructed by Cytoscape, and Gene Ontology (GO) and Kyoto Encyclopedia of Genes and Genomes (KEGG) were performed to clarify the mechanism of the cannabis compounds against EP. Finally, the interaction between key compounds and targets was analyzed using molecular docking.
Materials and methods
Chemical ingredients database building
All chemical ingredients from cannabis were collected from Traditional Chinese Medicines for Systems Pharmacology database and analysis platform (TCMSP, available online: http://lsp.nwsuaf.edu.cn/) [Citation11] and related literatures.
Active ingredients screening
The molecular properties of compounds were determined using SwissADME database (available online: http://www.swissadme.ch/index.php) [Citation12]. The selection for candidate compounds involved gastrointestinal absorption (GI absorption) and drug likeness rules (Lipinski, Ghose, Veber, Egan and Muegge). For the screening indicator, the GI absorption was considered as high, and two or more indices of drug likeness rules had to be Yes.
Target prediction and validation
Target prediction is helpful to clarify the underlying mechanism of action of cannabis. Swiss TargetPrediction (available online: http://www.swisstargetprediction.ch/) and PharmMapper (available online: http://www.lilab-ecust.cn/pharmmapper/) databases were used to retrieve and validate related targets of compounds. Then, the target name–gene name standard conversion was performed using the UniProt database (available online: https://www.uniprot.org/), so that the names of each target were corrected to the official names. After that, the candidate targets for the treatment of EP were screened by searching the GeneCards (available online: https://www.genecards.org/), TTD (available online: http://db.idrblab.net/ttd/) and OMIM (available online: https://omim.org/) databases [Citation13]. The Venny 2.1 platform was used to draw a Veen diagram showing the overlapping of cannabis targets and EP targets. The overlapping was considered for potential therapeutic targets of cannabis against EP.
Compound-target (C-T) network construction
For a scientific explanation of the pharmacological mechanism of cannabis, two networks were established as follows: (1) the C–T network was established by linking active ingredients with their targets that were related to EP; (2) the C-T network of cannabinoids and their related targets was constructed.
GO and KEGG enrichment
In organisms, genes cannot perform their functions independently. Different genes coordinate with each other to finish a series of biochemical reactions to perform their biological functions. Therefore, GO and KEGG pathway enrichment analysis were used to provide a more systematic and comprehensive understanding of the mechanism of action. The Metascape platform [Citation14] was applied to thoroughly analyze enrichment information. GO/KEGG terms with p < 0.05 were considered significantly enriched. The top 20 pathways containing the number of targets were selected. The Target–Pathway (T–P) network was constructed to link compounds and pathways with related targets by Cytoscape [Citation15], and the network topological properties were analyzed by Network Analyzer. In addition, the KEGG Mapper tool (available online: https://www.kegg.jp/kegg/mapper.html) was used for the construction of the pathway map.
Molecular docking
Active ingredients that were in the top ten and the top five targets in terms of degree value were screened based on the C–T network. The structures of the target proteins and the compounds were obtained from the PDB (available online: http://www.rcsb.org/) and PubChem (available online: http://www.ncbi.nlm.gov/pccompond) databases, respectively. Both of them were introduced into Schrodinger for molecular docking, and the docking score was analyzed to predict and evaluate the interaction between compounds and targets (the lower the docking score, the more negative number, and the better the binding affinity).
Results
Classification of active compounds and predicted targets
In this research, a total of 360 compounds were found in cannabis from the literatures and TCMSP database. Among them, 235 active compounds were selected through SwissADME database. The screening criteria were GI absorption shown as ‘high’, and at least two rules being ‘yes’ in the drug likeness rules (). In addition, a total of 1117 targets were predicted through Swiss TargetPrediction and PharmMapper databases using these active compounds, and 1403 EP-related targets were retrieved from the GeneCards, TTD and OMIM databases. By comparing these targets with the predicted cannabis targets, 116 common targets were filtered as the key targets for researching on the antiepileptic activity of the cannabis compounds (). Nine ingredients that did not have any relevant targets were removed. The detailed information of the 116 candidate targets is displayed in .
Table 1. 235 potential active ingredients and ADME parameters of Cannabis sativa L.
Table 2. Information on 116 potential targets of Cannabis sativa L.
C–T Network construction and analysis
To understand the interaction between compounds and targets, the C–T network () was constructed by mapping 226 candidate ingredients and their related 116 targets, which consisted of 342 nodes and 1668 edges. The possible interactions between natural products and target proteins were assessed by degree, an important topological parameter. The results showed that cannabinoids displayed more intimate association to most targets, when docking with Cannabinoid receptor 1 (CNR1), Albumin (ALB) and Glutamate receptor ionotropic, NMDA 2 A (GRIN2A), etc. To further identify the active ingredients of cannabis for the treatment of EP, the C-T network () of cannabinoids was also constructed, which embodied 199 nodes (106 cannabinoid-compounds and their related 93 targets) and 996 edges. A network analysis showed that cannabicitran (M117, degree = 20), cannabichromanone D (M85, degree = 20), (±)-6,7-trans-epoxycannabigerolic (M100, degree = 19) and cannabinol methyl ether (M54, degree = 18) had the highest number of connections to different, which might play a key role in the treatment of EP. What is more, it could be observed that CNR1 (degree = 113), Androgen receptor (AR, degree = 97), Glycogen synthase kinase-3 beta (GSK3B, degree = 59), ALB (degree = 53) and Mitogen-activated protein kinase 10 (MAPK10, degree = 51), which corresponded to multiple compounds, might be the key targets of the network.
GO and KEGG enrichment analysis
The Metascape database was used to analyze the 116 potential targets of cannabis for EP by using GO and KEGG enrichment analysis. The threshold was set at p < 0.05, and the previous GO annotation results and KEGG pathway results were filtered out, as shown in . GO enrichment results showed that the main biological processes involved in the active ingredients of cannabis were chemical synaptic transmission, anterograde trans-synaptic signalling and so on. The main cellular components of cannabis were synaptic membrane, post-synapse, postsynaptic membrane and so on. The major molecular functions were neurotransmitter receptor activity, postsynaptic neurotransmitter receptor activity and so on. KEGG pathway analysis displayed that cannabis could play an overall regulatory role through multiple pathways, including neuroactive ligand-receptor interaction, nicotine addiction and so on.
Pathway construction and analysis
Based on the prediction results of KEGG enrichment, the pathways and targets were shown in . The results showed an average degree of 9.71 per target and 5.57 per pathway, and several target proteins (82 out of 98) mapped to multiple pathways. The T–P network of the top 20 pathways with 73 targets mapped to 20 pathways was constructed as shown in . The results displayed that the candidate ingredients were intensively associated with the pathways as follows: neuroactive ligand-receptor interaction, MAPK signalling pathway, taste transduction, GABAergic synapse, retrograde endocannabinoid signalling and cAMP signalling pathway. An integrated ‘EP pathway’ was constructed using 15 EP-related signalling pathways extracted from KEGG pathway as shown in . The targets of the integrated ‘EP pathway’ exhibited a close functional relationship with those related to EP. The calcium signalling pathway, MAPK signalling pathway and synaptic connections were the main antiepileptic pathways in cannabis, which were displayed in , respectively.
Molecular docking analysis
In order to further explore the active mechanism, the interactions between the potential active compounds (M10, M11, M30, M34, M46, M54, M85, M100, M116 and M117) and the targets proteins were elucidated using Schrodinger, the docking scores of targets with the active compounds are listed in . The interaction between the active site residues and the target protein is shown in . The results indicated that M10 and M46 produced hydrogen bonds with GSK3B (LYS-85, ASP-200 and GLC-185) and MAPK10 (H2O), respectively (). In addition, M54 showed a PI–PI stacking interaction with CNR1 (PHE-379 residue) and AR (TYR–210 and TRP–21), respectively (). According to the docking result of ALB, there was not only conventional PI-PI stacking interaction (TYR-138) but also a Pi–anion interaction with ABG-117 residue ().
Figure 7. Schematic 3 D representation that the molecular docking model. (A) Binding mode of M10 to GSK3B. (B) Binding mode of M46 to MAPK10. (C) Binding mode of M54 to CNR1. (D) Binding mode of M54 to AR. (E) Binding mode of M34 to ALB.
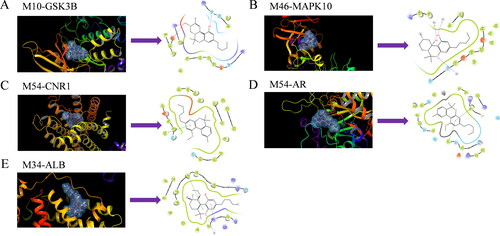
Table 3. Molecule docking scores for potential active ingredients with targets.
Discussion
Network pharmacology has caught more and more attention and becomes an effective tool in identifying alternative targets for traditional medicines and developing new drugs. In the present study, the action mechanism of cannabis against EP was analyzed at the overall level based on the network pharmacology. As a result, a total of 116 potential targets and 226 active compounds in cannabis were obtained, suggesting a potential comprehensive treatment strategy based on TCM featured by multiple compounds, targets and pathways by applying a variety of methods, including C-T and T-P network construction, GO and KEGG pathway enrichment analysis and molecule docking.
Firstly, we predicted the active compounds in cannabis which might be the leading compounds for further drug development, indicating that cannabinoids have better performance corresponding to the potential targets, such as CNR1, GSK3B and MAPK10. As shown in , a total of 106 active cannabinoids and 93 potential targets of cannabinoids against EP were obtained. It is interesting to note that in addition to CBD and tetrahydrocannabinol (THC) [Citation16], other cannabinoids might have the antiepileptic effect that has not been confirmed, such as M117, M85, M100 and M54. In these cannabinoids, the oxygen of the hydroxyl and keto groups prefer to interact with the targets by forming hydrogen bonds with the active site residues, while the benzene rings and hexatomic rings prefer to form PI–PI stacking interaction or Pi–anion interaction with the active site residues, which might have better performance in the treatment of EP. CNR1, AR, GSK3B, ALB and MAPK10 acted on most cannabinoids, which might be the key targets for the treatment of EP. Already accumulated evidence indicated that CNR1 is abundant in the hippocampus of the central nervous system, and activation of CNR1 inhibits nerve transmission, reduces nerve excitability and regulates the intrinsic excitability of neurons, and most cannabinoids act on CNR1 [Citation17]. GSK3B plays a key role in maintaining intracellular homeostasis. When GSK3B is activated, the expression of Potassium/sodium hyperpolarization-activated cyclic nucleotide-gated channel 4 (HCN4) increases and the phosphorylation of AMPA receptor subunit GluA1 at Serine 831 is inhibited, which protects the central nervous system and achieves antiepileptic effect [Citation18]. Therefore, active cannabinoids might play a key role in the process of EP treatment.
Secondly, GO, the main bioinformatics approach, consists of biological process, cellular component and molecular function. KEGG, a database about pathways, was accessed to obtain not only a gene set but also to define the complex relationship between genes. Based on the results of GO and KEGG pathway enrichment analysis, the targets were associated with various biological processes and pathways, including calcium signalling pathway, MAPK signalling pathway, Synaptic connections, nicotine addiction and morphine addiction, which were interacting.
Calcium signalling pathway
The calcium signalling pathway () plays an important role in epileptic seizures, and Voltage-dependent N-type calcium channel subunit alpha-1B (CACNA1B), Voltage-dependent L-type calcium channel subunit alpha-1C (CACNA1C), Voltage-dependent T-type calcium channel subunit alpha-1G (CACNA1G) and Neuronal acetylcholine receptor subunit alpha-7 (CHRNA7) in the pathway are all related to EP. These targets change the concentration of Ca2+ in and out of cells, which in turn affects the cell activities such as transmitter release and cell excitation, and prevents the occurrence and seizure of EP. The mutation of CACNA1B affected the calcium ion transmission and leads to the damage of the synaptic nerve, thus causing EP-related diseases [Citation19]. Clinical studies had shown that the mutation of CACNA1C encoding L-type calcium channel was associated with Timothy and Brugada syndrome [Citation20]. At the same time, CACNA1G mutation was closely related to the onset of cerebellar atrophy in childhood [Citation21]. These provide evidence for the active compounds in cannabis to inhibit EP.
MAPK signalling pathway
The MAPK signalling pathway (), which is involved in signal transduction after activation of various growth factors, cytokines, mitogens and hormone receptors [Citation22, Citation23], is closely related to the pathogenesis of EP. Growth factor receptor-bound protein 2 (GRB2), which binds Fibroblast growth factor receptor 1 (FGFR1) and Fibroblast growth factor receptor 3 (FGFR3) to Son of sevenless (SOS), Transduced rat sarcoma (Ras), which in turn activate Mitogen-activated protein kinase (Mek) and Mitogen-activated protein kinase kinase kinase 1 (MEKK1) and phosphorylate MAPK10. P38 MAPK is activated and its phosphorylation level is increased in the model of epileptic seizure in rats. Inhibition of P38 MAPK reduces neuronal damage and thus reduces the epileptic seizures [Citation24]. This underscores the importance of the MAPK signalling pathway in preventing seizures.
Synaptic connections
During seizures, the synaptic connections () in the brain are abnormal, which in turn increases brain excitability. Cannabis might be able to treat EP through GABAergic Synapse, glutamatergic synapse, dopaminergic synapse, cholinergic synapse, and so on. Studies have shown that GABAA receptor mutation causes GABAergic synapses to be impaired, the function of GABAA receptor to be reduced and the binding force of GABA to be reduced; and GABRA5, the subunit of GABAA receptor, has been proposed as the pathogenic gene of epileptic encephalopathy with early onset [Citation25, Citation26]. When the content of GABA is increased, excitatory neurotransmitter is inhibited, to control seizures [Citation27]. Glutamatergic Synapse is directly related to an abnormal discharge of neurons and has always been thought to be closely related to the occurrence of EP. Hyperactivity of GLS and other targets in this pathway leads to an increase in glutamate content, which acts on ion channels and increases excitability [Citation28–30]. Similarly, dopaminergic synapse and cholinergic synapse also facilitate epileptic seizures [Citation31–33].
Other signalling pathways
The study also predicted that nicotine addiction and morphine addiction, which was also effective in EP, reduced the duration and frequency of epileptic seizures by stimulating neurocytokines and suppressing neurotransmission [Citation34, Citation35]. Retrograde endocannabinoid could promote seizures by inhibiting the release of GABA at inhibitory synapses [Citation36].
In summary, these pathways play a significant role in the treatment of EP and also provide a piece of powerful evidence for multi-target treatment. Besides, molecular docking was used as the target and molecular interaction widely. The good molecular docking results showed that M54 (cannabinol methyl ether) had a high binding score with its corresponding targets, which could possibly become the lead compound for further research.
Conclusions
To clarify the mechanism of action of cannabis in the EP treatment systematically, the network pharmacology and molecular docking were employed to explore the active compounds, the targets of cannabis against EP, and the related signalling pathways. A total of 226 potentially active compounds in cannabis and 1117 of their targets were predicted by multiple databases. At the same time, 1403 EP-related targets were screened by multiple databases. A total of 116 common targets were obtained by statistical intersection. The GO and KEGG enrichment analysis indicated that there were multiple interactions. The results showed that compounds in cannabis, especially cannabinoids, interact with the targets (CNR1, ALB, GSK3BA, AR and MAPK10) to ameliorate EP, and the effect is due to the joint action of the calcium signalling pathway, MAPK signalling pathway, synaptic connections and so on. Moreover, molecular docking analysis confirmed the antiepileptic effect of cannabis. However, in vitro and in vivo experiments should be carried out based on this study. In sum, the mechanism of cannabis in the EP treatment was analyzed systematically based on network pharmacology. This study provides a practicable strategy for the development of new cannabis drugs for EP.
Abbreviations | ||
EP | = | epilepsy |
Cannabis | = | Cannabis sativa L. |
CBD | = | cannabidiol |
C-T | = | compound-target |
T-P | = | target-pathway |
TCM | = | traditional Chinese medicine |
ADME | = | absorption, distribution, metabolism and excretion |
OMIM | = | online Mendelian inheritance in man |
TTD | = | therapeutic target database |
GO | = | gene ontology |
KEGG | = | Kyoto encyclopedia of genes and genomes |
TCMSP | = | traditional Chinese medicines for systems pharmacology database and analysis platform |
GI | = | gastrointestinal |
TCM | = | traditional Chinese medicine |
THC | = | tetrahydrocannabinol |
HCN4 | = | potassium/sodium hyperpolarization-activated cyclic nucleotide-gated channel 4 |
CACNA1B | = | voltage-dependent N-type calcium channel subunit alpha-1B |
CACNA1C | = | voltage-dependent L-type calcium channel subunit alpha-1C |
CACNA1G | = | voltage-dependent T-type calcium channel subunit alpha-1G |
CHRNA7 | = | neuronal acetylcholine receptor subunit alpha-7 |
GRB2 | = | growth factor receptor-bound protein 2 |
FGFR1 | = | fibroblast growth factor receptor 1 |
FGFR3 | = | fibroblast growth factor receptor 3 |
SOS | = | son of sevenless |
Mek | = | mitogen-activated protein kinase |
MEKK1 | = | mitogen-activated protein kinase kinase kinase 1. |
Data availability
The data supporting the findings reported in this study are available from the corresponding author upon reasonable request.
Disclosure statement
W.X. is affiliated with Kanion Pharmaceutical Co. Ltd. There is no conflict of interest to declare regarding the content of interest of this article.
Additional information
Funding
References
- Fiest K, Sauro K, Wiebe S, et al. Prevalence and incidence of epilepsy: a systematic review and meta-analysis of international studies. Neurology. 2017;88(3):296–303.
- Kissani N, Lengané YTM, Patterson V, et al. Telemedicine in epilepsy: how can we improve care, teaching, and awareness?Epilepsy Behav. 2020;103(Pt A):106854–106860.
- Devinsky O, Vezzani A, O’Brien TJ, et al. Epilepsy. Nat Rev Dis Primers. 2018;4(1):1–24.
- Prospéro-García O, Contreras AER, Gómez AO, Grupo de Neurociencias de la Universidad Nacional Autónoma de México, et al. Endocannabinoids as therapeutic targets. Arch Med Res. 2019;50(8):518–526.
- Szaflarski JP, Bebin EM. Cannabis, cannabidiol, and epilepsy-from receptors to clinical response. Epilepsy Behav. 2014;41:277–282.
- Wang Z, Liu J, Yu Y, et al. Modular pharmacology: the next paradigm in drug discovery. Expert Opin Drug Discov. 2012;7(8):667–677.
- Wang Y, Yang L. Systems pharmacology based research framework of traditional Chinese medicine. World Chinese Med. 2013;8(7):103–110.
- Huang C, Zheng C, Li Y, et al. Systems pharmacology in drug discovery and therapeutic insight for herbal medicines. Brief Bioinform. 2014;15(5):710–733. doi:.
- Ferreira LLG, Santos RN, Oliva G, et al. Molecular docking and structure-based drug design strategies. Molecules. 2015;20(7):13384–13421.
- Hirayama N. Docking simulations between drugs and HLA molecules associated with idiosyncratic drug toxicity. Drug Metab Pharmacokinet. 2017;32(1):31–39.
- Ru J, Li P, Jinan W, et al. TCMSP: a database of systems pharmacology for drug discovery from herbal medicines. J Cheminf. 2014;6(1):13–18.
- Daina A, Michielin O, Zoete V. SwissADME: a free web tool to evaluate pharmacokinetics, drug-likeness and medicinal chemistry friendliness of small molecules. Sci Rep. 2017;7(1):42713–42717.
- Wang Y, Zhang S, Li F, et al. Therapeutic target database 2020: enriched resource for facilitating research and early development of targeted therapeutics. Nucleic Acids Res. 2020;48(D1):D1031–D1041.
- Zhou Y, Zhou B, Pache L, et al. Metascape provides a biologist-oriented resource for the analysis of systems-level datasets. Nat Commun. 2019;10(1):1–10.
- Shannon P, Markiel A, Ozier O, et al. Cytoscape: a software environment for integrated models of biomolecular interaction networks. Genome Res. 2003;13(11):2498–2504.
- Porter BE, Jacobson C. Report of a parent survey of cannabidiol-enriched cannabis use in pediatric treatment-resistant epilepsy. Epilepsy Behav. 2013;29(3):574–577.
- Wallace M, Wiley JL, Martin BR, et al. Assessment of the role of CB1 receptors in cannabinoid anticonvulsant effects. Eur J Pharmacol. 2001;428(1):51–57.
- Urbanska M, Kazmierska-Grebowska P, Kowalczyk T, et al. GSK3β activity alleviates epileptogenesis and limits GluA1 phosphorylation. EBioMed. 2019;39:377–387.
- Gorman KM, Meyer E, Grozeva D, NIHR BioResource, et al. Bi-allelic loss-of-function CACNA1B mutations in progressive epilepsy-dyskinesia. Am J Hum Genet. 2019;104(5):948–956.
- Bozarth X, Dines JN, Cong Q, et al. Expanding clinical phenotype in CACNA1C related disorders: From neonatal onset severe epileptic encephalopathy to late-onset epilepsy. Am J Med Genet A. 2018;176(12):2733–2739.
- Chemin J, Siquier-Pernet K, Nicouleau M, et al. De novo mutation screening in childhood-onset cerebellar atrophy identifies gain-of-function mutations in the CACNA1G calcium channel gene. Brain. 2018;141(7):1916–1998.
- Anjum R, Blenis J. The RSK family of kinases: emerging roles in cellular signalling. Nat Rev Mol Cell Biol. 2008;9(10):747–758.
- Kim EK, Choi E-J. Pathological roles of MAPK signalling pathways in human diseases. Biochim Biophys Acta. 2010;1802(4):396–405.
- Kim S-W, Yu Y-M, Piao CS, et al. Inhibition of delayed induction of p38 mitogen-activated protein kinase attenuates kainic acid-induced neuronal loss in the hippocampus. Brain Res. 2004;1007(1-2):188–191.
- Hernandez CC, Xiangwei W, Hu N, et al. Altered inhibitory synapses in de novo GABRA5 and GABRA1 mutations associated with early onset epileptic encephalopathies. Brain. 2019;142(7):1938–1954.
- Li R, Wu B, He M, et al. HAP1 modulates epileptic seizures by regulating GABAAR function in patients with temporal lobe epilepsy and in the PTZ-Induced epileptic model. Neurochem Res. 2020;45(9):1997–2008.
- Treiman DM. GABAergic mechanisms in epilepsy. Epilepsia. 2001;42(s3):8–12.
- Rumping L, Tessadori F, Pouwels PJ, et al. GLS hyperactivity causes glutamate excess, infantile cataract and profound developmental delay. Hum Mol Genet. 2019;28(1):96–104. doi:.
- O’Shea RD. Roles and regulation of glutamate transporters in the central nervous system. Clin Exp Pharmacol Physiol. 2002;29(11):1018–1023.
- Aragón C, López-Corcuera B. Structure, function and regulation of glycine neurotransporters. Eur J Pharmacol. 2003;479(1–3):249–262.
- Rezaei M, Sadeghian A, Roohi N, et al. Epilepsy and dopaminergic system. Physiol Pharmacol. 2017;21(1):1–14.
- Starr MS. The role of dopamine in epilepsy. Synapse. 1996;22(2):159–194.
- Friedman A, Behrens CJ, Heinemann U. Cholinergic dysfunction in temporal lobe epilepsy. Epilepsia. 2007;48(s5):126–130.
- Lippert T, Gelineau L, Napoli E, et al. Harnessing neural stem cells for treating psychiatric symptoms associated with fetal alcohol spectrum disorder and epilepsy. Prog Neuro-Psychopharmacol Biol Psych. 2018;80(5):10–264.
- Gholami M, Saboory E, Ahmadi AA, et al. Long-time effects of prenatal morphine, tramadol, methadone, and buprenorphine exposure on seizure and anxiety in immature rats. Int J Neurosci. 2019;130(9):1–16.
- Sugaya Y, Kano M. Control of excessive neural circuit excitability and prevention of epileptic seizures by endocannabinoid signalling. Cell Mol Life Sci. 2018;75(15):2793–2811.