Abstract
Bupleurum chinense DC. is a perennial herb plant belonging to the Umbelliferae family, genus Bupleurum, that is used as a traditional Chinese medicine. Coumarins are characteristic secondary metabolites of the Apiaceous family, which possess anti-inflammatory, anti-cancer and antiviral activities, among others. Few studies on the coumarin biosynthesis pathways in B. Chinese have been reported. In this study, we identified 89 differentially expressed genes belonging to 7 gene families involved in the synthesis of coumarins in B. chinense, using transcriptome and metabolomic data obtained by high-throughput RNA-sequencing data analysis, and liquid chromatography tandem mass spectrometry. Five coumarin compounds: esculetin (2), fraxetin (4), psoralen (5), parmesan (6) and angelicin (7) were identified through metabolomic analysis. A total of 39 unigenes belonging to five gene families: shikimate O-hydroxycinnamoyltransferase (HCT), caffeic acid ο-methyltransferase (COMT), 4-coumarate: coenzyme A ligase (4CL), feruloyl-CoA 6’-hydroxylase (F6’H) and psoralen synthase (PS), were identified by correlating coumarin content with transcriptional changes. According to correlation network analysis, PS genes (Bc09210, Bc12711), COMT genes (Bc14830, Bc17539, Bc28792, Bc32856) and HCT genes (Bc32814, Bc10245) may be important factors affecting coumarin biosynthesis.
Introduction
Bupleurum chinense DC. is a perennial herb plant of the Bupleurum genus, Apiaceous family [Citation1], that is used as a traditional Chinese medicine [Citation2]. Its major bioactive constituents include saikosaponin, volatile oils, lignans, flavonoids, coumarins, polysaccharides, sterols and other compounds [Citation3–5]. These secondary metabolites showed various pharmacological properties, including anti-inflammatory, anti-tumor, antiviral, antioxidant, anti-ulcer, immunomodulatory and hepatoprotective activities [Citation6–9]. Coumarins are the characteristic chemical constituents of the Apiaceous family, and the coumarins isolated from Bupleurum are generally simple coumarins [Citation1,Citation10–13] ( and ).
Coumarins, also known as 1,2-benzopyrones, are natural phenylpropanoid compounds with high stability and solubility, and low toxicity. Previous studies have revealed a variety of physiological and pharmacological activities of coumarins, including antioxidant, insecticidal, phytoalexin, growth inhibition and other effects on plants. In those studies, coumarins acted as non-enzymatic antioxidants and phytoprotectants that improved glyoxalase enzyme activity under saline conditions [Citation14,Citation15], and that activated plants’ "Priming" mode via phytoalexin activity to defend against pathogen infection [Citation16]. High coumarin concentrations will also inhibit seed germination [Citation17]. Simultaneously, coumarins also display anti-viral, anti-fungal, anti-tumor, anti-inflammatory, anti-osteoporosis, anti-cancer and other activities [Citation18], and variants including osthole, umbelliferone, esculin and 4-hydroxycoumarin primarily initiate apoptosis in T98G and MOGGCCM cells, and display anti-glioma potential [Citation19]. Coumarins can act as anti-hepatitis drugs by interfering with signalling pathways, and can inhibit influenza, enterovirus 71 and coxsackievirus A16 infections [Citation20]. Coumarins are biosynthesized via phenylpropanoid metabolism [Citation21], which involves enzymes including phenylalanine ammonia lyase (PAL), cinnamate 4-hydroxylase (C4H), 4-coumarate: coenzyme A ligase (4CL), caffeic acid 3-O-methyltransferase (COMT), psoralen synthase (PS), angelicin synthase (AS), feruloyl-CoA ortho-hydroxylase (F6’H) and shikimate O-hydroxy cinnamoyl transferase (HCT) [Citation22]. Phenylalanine is used as an initial substrate in biosynthesis [Citation23]. The initial reaction forms cinnamic acid, catalyzed by PAL, which is then converted to ρ-coumaric acid by C4H. Afterwards, ρ-coumaric acid undergoes further reactions to produce various coumarins [Citation24], including umbelliferone, esculetin and scopoletin, which can be produced from their respective o-hydroxycinnamoyl-CoA thioesters using recombinant coumarin synthase (COSY) by trans-cis isomerization followed by lactonization [Citation25].
Currently, high-throughput integrated analysis technology is an essential means of obtaining valuable biological information. Transcriptomics, metabolomics and their combination are important aspects of systems biology [Citation26], which have been applied to studies of Bupleurum. Illumina HiSeqTM 2500 high-throughput sequencing technology was used for Bupleurum transcriptome sequencing, obtaining 6.52 GB of clean data, and 59,288 unigenes [Citation27]. Chloroplast genome sequences from B. chinense DC. and B. boissieuanum H. Wolff were sequenced on an Illumina HiSeq X Ten platform, and comparative genomics and phylogenetic analysis were carried out based on sequencing data [Citation28]. The coumarin biosynthetic pathways of other plants have also been studied. One hundred and twenty-nine up-regulated differentially expressed genes (DEGs) (encoding BGA (99 DEGs), 4CL (2 DEGs), HCT (13 DEGs), COMT (7 DEGs) and F60H1 (8 DEGs)) involved in coumarin biosynthesis and accumulation were identified by analyzing the transcriptome data of flower, leaf, root and stem tissues of Cnidium monnieri L. Cuss [Citation24]. A large amount of transcriptomic data for Peucedanum praeruptorum Dunn was assembled to identify putative CYP450 and multidrug resistance (MDR) genes involved in coumarin compound biosynthesis and transport [Citation21]. To date, coumarins have been under-studied in B. chinense. Thus the biosynthetic pathway remains to be studied, and key regulatory genes remain unclear.
To identify the functional genes regulating coumarin biosynthesis in B. chinense, this study performed metabolome analysis on B. chinense root, stem, leaf and flower tissues at flowering stage. Transcriptome analyses were also performed. We screened to identify key genes involved in regulatory networks impacting coumarin biosynthesis by correlating transcriptome and metabolome analysis results.
Materials and methods
Plant materials
The commercial B. chinense variety Chuanbeichai No. 1 (CBC1), bred by Dr. Jianhe Wei from the Institute of Medicinal Plant Development (IMPLAD), Chinese Academy of Medical Sciences and Peking Union Medical College (Beijing, China), and Dr. Da-bin Hou from Southwest University of Science and Technology (Sichuan, China), was used as our experimental material. CBC1 materials were planted in a 150 cm × 400 cm plot in a greenhouse (25 °C, 14 h light/10 h dark) in Mianyang, Sichuan province (31°32′ N, 104°42′ E), with both plant spacing and row spacing of 25 cm. Root, stem, leaf and flower tissues were collected at the flowering stage in June 2018. After cleaning with ultrapure water, three biological replicates of each sample type were immediately frozen in liquid nitrogen and stored in an ultra-low freezer at −80 °C.
Metabolite extraction and HPLC-MS/MS analysis
One hundred milligrams each of 12 tissue samples (four organs × three biological replicates) were placed into EP tubes, snap frozen in liquid nitrogen, ground, then suspended in 500 μL 80% methanol (Thermo, United States) aqueous solution containing 0.1% formic acid (Thermo, United States), and vortexed. All samples were placed in an ice bath for 5 min, then centrifuged at 15000 rpm (Thermo ST16R centrifuge, United States), 4 °C for 10 min. A portion of each supernatant was diluted to a final concentration containing 53% methanol, using 1/2 volume LC-MS grade water. Samples were centrifuged at 15000 rpm, 4 °C for 20 min in a fresh Eppendorf tube, and supernatants were collected for analyses by high-performance liquid chromatography-tandem mass spectrometry (HPLC-MS/MS). Equal volumes were taken from each sample and blended for a quality control (QC) reference sample.
An ExionLC™ AD system (SCIEX, United States) coupled with a QTRAP® 6500+ mass spectrometer (SCIEX) [Citation29,Citation30] were used for HPLC-MS/MS analysis. A BEH C8 Column (100 mm ×2.1 mm, 1.9 μm, Waters, United States) was used with a 30-min linear gradient at a flow rate of 0.35 mL/min in positive polarity mode (ESI+). An aHSS T3 Column (100 mm ×2.1 mm) was used with a 25-min linear gradient at a flow rate of 0.35 mL/min in negative polarity mode (ESI–). In both modes, the mobile phase consists of solvent A (0.1% formic acid–water) and solvent B (0.1% formic acid–acetonitrile). The solvent gradient was as follows: 5% B (2% in ESI–), 1 min; 5%–100% B (2%–100% in ESI–), 24.0 min (18 min in ESI–); 100% B, 28.0 min (22 min in ESI–); 100%–5% B, 28.1 min (22.1 min in ESI–); 5% B, 30 min (25 min in ESI–). A QTRAP® 6500+ mass spectrometer was operated in ESI + and ESI– modes with Curtain Gas at 35 psi, Collision Gas at Medium, an IonSpray Voltage of 5500 V (–4500 V), Temperature of 500 °C, Ion Source Gas of 1:55. Data files were integrated and peaks corrected with SCIEX OS Version 1.4 software.
Metabolite data analyses
Identified metabolites were annotated to understand the functional characteristics and classification of the different metabolites using KEGG (http://www.genome.jp/kegg/), HMDB (http://www.hmdb.ca/) and LIPID MAPS (http://www.lipidmaps.org/) databases. Principal component analysis (PCA) and Partial least squares discriminant analysis (PLS-DA) were performed using MetaX software [Citation31–33]. Differentially expressed metabolites were found by the Variable Importance in the Projection (VIP) of the PLS-DA model combined with the statistical significance (P-value) of univariate analysis (t-test), with a threshold setting of VIP > 1.0, FC > 2.0 or FC < 0.5 and p-value < 0.05 [Citation34–36]. Clustering heat maps were produced with heatmap package [Citation37], and correlations between different metabolites were analyzed by cor (x, y) in R language (method = Pearson) [Citation38].
Iso-seq and transcriptome analyses
RNA was extracted from four different organs, and transcriptome sequencing was carried out according to the Isoform Sequencing protocol (Iso-Seq) using a Clontech SMARTer PCR cDNA Synthesis Kit (Clontech, United States) and the BluePippin Size Selection System protocol described by Pacific Biosciences (Pacific Biosciences, California, United States). Sequencing data was processed using SMRTlink 5.1 software. Additional nucleotide errors were corrected using Illumina RNAseq data with LoRDEC software [Citation39], and CD-HIT [Citation40] was used to remove redundancies in corrected consensus reads. We used high confidence protein sequences derived from this species or closely related species for ANGEL training, then ran ANGEL to predict and analyze coding sequences (CDS) [Citation41]. CNCI [Citation42], CPC [Citation43], Pfam [Citation44] and PLEK [Citation45] were used to predict the coding potential of transcripts. Plant transcription factors (TFs) were predicted using iTAK software [Citation46]. Iso-seq and transcriptome analyses were both completed by the Novogene Bioinformatics Institute (Novogene, Beijing, China).
Gene functional annotation and analyses
After redundancies were removed with CD-HIT software, gene function was annotated using the following databases: NCBI non-redundant protein sequences (NR) [Citation47], NCBI non-redundant nucleotide sequences (NT), Gene Ontology (GO) [Citation48], Cluster of Orthologous Groups of proteins (COG) [Citation49], Kyoto Encyclopedia of Genes and Genomes (KEGG) [Citation50], Protein family (Pfam), followed by the manually annotated and reviewed protein sequence database (Swiss-Prot) [Citation51]. BLAST was used for alignments, with an e-value of ‘1e−10′ in NT database analysis, and Diamond BLASTX was used with an e-value of ‘1e−10′ in NR, KOG, Swiss-Prot and KEGG database analyses. Hmmscan was used for Pfam database analysis. The expected number of Fragments Per Kilobase of transcript sequence per Millions base pairs sequenced (FPKM) transformation was determined using the readcount data of each sample compared to each gene, as counted by bowtie2 of RSEM [Citation52] software in end-to-end, sensitive mode. Other default parameters were used for comparison results, after correction of consensus sequence redundancy. The average Fragments Per Kilobase Million (FPKM) values of genes were converted to log2 values, and the Heatmap package in R (3.6.2) was used for visualization. Differential expression analysis was performed using the DESeq2 R package (3.6.2) [Citation53]. We used a model based on the negative binomial distribution by DESeq2 to determine differential expression from digital gene expression data. The Benjamin and Hochberg approaches were used to control the false discovery rate and adjust the obtained P values. Genes with adjusted P-values < 0.05 found by DESeq2 were designated as differentially expressed. GO enrichment analysis of differentially expressed genes was carried out with the GOseq R package (3.6.2), and KOBAS software was used to test the statistical enrichment of differentially expressed genes (DEGs) in KEGG pathways.
Integrative metabolome and transcriptome analyses
A regulatory factor network was constructed using the Pearson correlation algorithm on TF related genes and metabolites. In four different organs, the average content of metabolomic data and the average value of differentially expressed genes in transcriptomic data were used for correlation analyses, and the fold changes in metabolomic and transcriptomic data in each organ were compared. The log2 (fold change) of each metabolite, and log2 (fold change) of each transcript were calculated using EXCEL, and a correlation coefficient of R2 > 0.9 (P-value < 0.05) was selected. Cytoscape (version 3.6.1) [Citation54] software was used to visualize metabolites and transcription factors.
Results
Coumarins compounds of metabolites
In this study, 5 metabolites involved in coumarin biosynthesis pathways were found. These were fraxetin, angelicin, psoralen, esculetin and marmesin (). Significant differences in fraxetin and angelicin were observed between root and stem, and between root and leaf, and differences in psoralen were observed between root and flower (VIP >1.0, P-value <0.05). The fraxetin and angelicin contents in roots were higher than those in leaf, stem and flower samples. The esculetin and marmesin contents were higher in leaves than in other tissues. The psoralen content was highest in flowers.
Table 1. Summary of Fraxetin, Angelicin, Psoralen, Esculetin, marmesin.
Unigenes involved in coumarins biosynthesis
Coumarin biosynthesis mainly occurs through the phenylalanine pathway. FPKM values in various organs were used to indicate levels of differential expression. Eighty-nine differentially expressed genes related to enzymes in coumarin biosynthesis pathways were identified, including members of seven gene families: PAL (2 unigenes), C4H (1 unigenes), HCT (13 unigenes), COMT (40 unigenes), 4CL (5 unigenes), F6’H (14 unigenes) and PS (14 unigenes) (, ). We produced a heatmap to show the relative expression of different genes in root, stem, leaf and flower tissues. A portion of these genes are obviously highly expressed in roots, especially the C4H and F6’H families. Some other gene families are highly expressed in flowers, including two HCT genes, sixteen COMT genes, one 4CL gene and three PS genes. The remaining genes were highly expressed in stems or leaves.
Integrated analysis of gene expression and metabolome data
Pearson correlation analysis between 5 coumarins and 89 unigenes was carried out, yielding strong correlation coefficient values (P-value <0.05) between 39 unigenes and 3 metabolites. An interaction network between metabolites and unigenes associated with coumarin biosynthesis pathways was established based on the results in root, stem, leaf and flower tissues in B. chinense, as described above (). Differences in unigene and metabolite distribution in different organs, and their relationships to each other can be found in the network. Among these, the contents of fraxetin and angelicin were obviously highest in roots, while the psoralen content was highest in flowers. Bc12711 (encoding PS), Bc14830 (encoding COMT), Bc32814 (encoding HCT) displayed their strongest correlations with fraxetin, Bc10245 (encoding HCT) displayed its strongest correlation with angelicin. The strongest correlations with psoralen in flowers were seen with Bc09210 (encoding PS), Bc17539 (encoding COMT), Bc28792 (encoding COMT) and Bc32856 (encoding COMT), as these are most abundantly expressed in flowers.
Figure 3. Putative biosynthetic pathways of coumarins in Bupleurum. PAL, phenylalanine ammonia lyase; C4H, cinnamate 4-hydroxylase; C3H, ρ-coumarate 3-hydroxylase; HCT, shikimate O-hydroxycinnamoyltransferase; COMT, caffeic acid ο-methyltransferase; 4CL, 4-coumarate: coenzyme A ligase; F6’H, feruloyl-CoA 6’-hydroxylase; PS,psoralen synthase.; C2’H, ρ-coumaroyl CoA 2’-hydroxylase; U-8-P, umbelliferone 8-prenyltransferase; CS, columbianetin synthase; AS, angelicin synthase; CA2H, caffeic acid 2-hydroxylase; OMT, ο-methyl-transferase; S8H, scopoletin 8-hydroxylase; *, no corresponding gene was found.
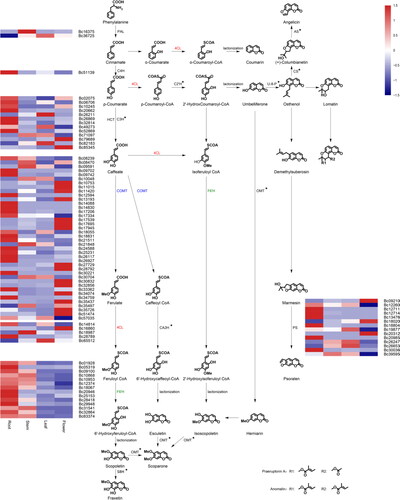
Figure 4. Connection network between regulatory genes and coumarins. The shapes represent roots, stems, leaves and flowers from the inside out; the expression levels of genes (green, ellipse) and metabolites (red, diamond) are indicated by shades of colour; the thickness of the line indicates the size of the correlation.
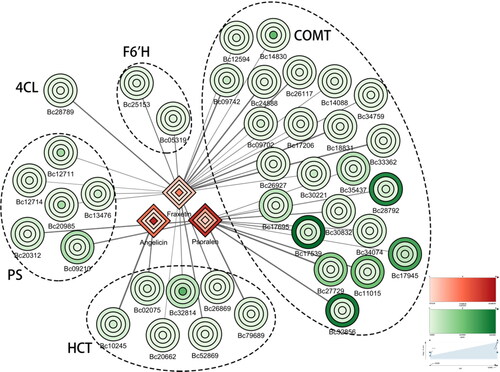
Discussion
As a traditional Chinese herbal medicine, Bupleurum possesses important medicinal properties. Most studies of Bupleurum to date have focussed on its active components, including saikosaponin and volatile oils. There is currently a lack of research into coumarin biosynthesis. In the present study, 12 specimens were comprehensively analyzed for metabolome and transcriptome data for root, stem, leaf and flower tissues. Correlation analyses between 89 genes and 5 coumarins identified 39 genes that were significantly correlated with 3 coumarins (fraxetin, angelicin and psoralen).
We used HPLC and high-resolution MS, solid-phase extraction (SPE) with LC-MS, ultraviolet spectrophotometry, and other biophysical techniques for quantitative coumarin studies [Citation55–57]. Metabolites, especially the coumarins fraxetin, psoralen, angelicin, esculetin and marmesin in root, stem, leaf and flower of Bupleurum were detected with HPLC-MS/MS (). In CBC1, each of these metabolites produced a different performance result. Fraxetin is most abundant in roots, as it is in Arabidopsis. Other studies have shown that fraxetin chelates and mobilizes Fe most efficiently [Citation58]. Our results are thus consistent with the general finding of large amounts of iron in plant roots [Citation59]. The relative content of angelicin, which is formed by angelicin synthase catalyzed conversion of (+) – Columbianetin [Citation60,Citation61] in different organs of CBC1 displayed the same pattern as that of fraxetin (root > flower > stem > leaf). The psoralen contents in root, stem, leaf and seed were comparable to results from a study of Psoralea corylifolia, which found that the psoralen content was highest in fruits, and lowest in roots [Citation62]. In this study, the psoralen content was assayed in root, stem, leaf and flower tissues, and was found to be highest in flowers and lowest in roots. Esculetin and marmesin were also detected in our samples, displaying their highest content in leaves, but the esculetin content was lowest in roots, and the marmesin content was lowest in stems.
The five metabolites in the present study can all be synthesized through the phenylalanine metabolic pathway (), and can be regulated by PAL, C4H, HCT, COMT, 4CL, F6’H, PS gene family enzymes. One gene (Bc16375) in the PAL gene family displayed significantly upregulated expression in stems alone. The Bc36725 gene displayed significantly downregulated expression in roots. In addition, one C4H gene, eight HCT genes, nineteen COMT genes, one 4CL gene, eight PS genes, and fourteen F6’H genes displayed significantly upregulated expression in roots. Four HCT genes, seventeen COMT genes, one 4CL gene, and three PS genes displayed elevated expression in flowers.
According to transcriptome and correlation analyses based on FPKM values, 39 unigenes related to coumarin biosynthesis from 5 gene families (HCT, COMT, 4CL, F6’H and PS) were identified (). These genes have different correlations with coumarin metabolites in the four examined tissues. Bc10245 and Bc02075, from the HCT family, correlated significantly (P-value <0.05) with angelicin. Thirteen unigenes (Bc79689 from the HCT family, Bc09210 and Bc20312 from the PS family, and Bc17539, Bc32856, c28792, Bc17945, Bc11015, Bc27729, Bc35437, Bc17695, Bc34074 and Bc30832 from the COMT family) correlated significantly with psoralen. The remaining 24 unigenes correlated significantly with fraxetin. In previous studies, PAL, 4CL, HTC and F6′H were identified as important enzymes for scopoletin biosynthesis [Citation63]. Scopoletin is synthesized through the phenylpropanoid pathway, which is the largest biosynthetic pathway among all pathways involved in secondary metabolite biosynthesis. Most of these transcripts play an important role in coumarin synthesis via this pathway [Citation64]. In the phenylalanine metabolic pathway, C4H and 4CL can convert cinnamate into p-coumaroyl-CoA via hydroxylation, methylation and dehydration [Citation65], and their activity levels can change in coordinated fashion in different development stages [Citation66]. The results of this study show that both Bc51139 (encoding C4H) and Bc28789 (encoding 4CL) were highly expressed in the root, and Bc28789 correlated significantly with fraxetin (p-value =0.01). Most research into phenylpropanoid metabolism has focussed on COMT, which is the first plant small molecule OMT to be described [Citation67], or have focussed on an important protein that participates in lignin synthesis [Citation68], but is also important for coumarin biosynthesis. In total, 13 and 10 unigenes are significantly associated with fraxetin and psoralen, respectively. Among these, expression levels of Bc14830, which correlates with fraxetin, are highest in the roots. The expression levels of Bc17539, Bc28792 and Bc32856 are higher in the flowers, and are all significantly correlated with psoralen. This suggests that COMT may regulate psoralen biosynthesis. PS (a cytochrome P450) can directly convert marmesin into psoralen [Citation69], consistent with the results of the present study, which indicate that genes PS are highly expressed in flowers and significantly correlate with psoralen. The coumarin content generally correlates positively with expression levels of related enzymes, and various enzymes may interact with each other and ultimately affect coumarin synthesis. The F6′H gene could be over-expressed to increase the cellular scopoletin content [Citation64], consistent with our results, that characterized most of the F6 ‘H genes as having their highest expression in roots, corresponding with highest fraxetin content occurring in roots.
In brief, transcriptomic and metabonomic analyses were performed on root, stem, leaf and flower tissues of adult CBC1 plants in this study. Enzymes involved in coumarin biosynthesis pathways were analyzed based on transcriptomic and metabonomic analysis results. Comparing the metabolite levels with related unigene expression levels indicated 8 unigenes that may play important roles in regulating coumarin biosynthesis. These results can be used to inform cultivation and breeding of new B. chinense cultivars in the future and can also be helpful for other research and related studies of other plant species.
Conclusions
In conclusion, we explored the regulatory network associated with coumarin biosynthesis using integrated analysis involving the metabolome and transcriptome of four tissues from CBC1. We also found that genes in COMT, HCT, PS, F6’H and 4CL families played an important role in regulating coumarin biosynthesis in B. chinense. In addition, Bc09210 (encoding PS), BC12711 (encoding PS), BC14830 (encoding COMT), BC32814 (encoding HCT), BC17539 (encoding COMT), BC28792 (encoding COMT), BC32856 (encoding COMT) and BC10245 (encoding HCT) may be key regulatory factors, making them of interest for future studies of coumarins in B. chinense.
Author contributions
Bin Yang and Shi-Hang Liu wrote the manuscript. Hua Chen and Meng Zhang performed data analyses. Jun Zhao and Yiguan Zhang performed transcriptome analyses. Bin Yang, Yilian He, and Yuchan Li performed metabolome analyses. Xia Yu performed the integrated analysis. Liang Feng seeded Chuanbeichai No.1. Dabin Hou bred out the commercial varieties of Chuanbeichai No.1. Junning Zhao and Ma Yu funded the project and helped Bin Yang complete the manuscript.
Supplemental Material
Download MS Excel (13 KB)Disclosure statement
The authors declare that the research was conducted in the absence of any commercial or financial relationships that could be construed as a potential conflict of interest.
Data availability statement
All data generated or analyzed during this study are included in this published article and its supplementary information files, further inquiries can be directed to the corresponding author.
Additional information
Funding
References
- Ashour ML, Wink M. Genus Bupleurum: a review of its phytochemistry, pharmacology and modes of action. J Pharm Pharmacol. 2011;63(3):305–321.
- Luo S. Studies on the chemical constituents of the aerial parts of Bupleurum chinense DC. China J Chinese Materia Medica. 1988;13(1):36–38.
- Wei X, Li CZ, Yong B, et al. Saikosaponins inhibit increased glutamate and GABA expressions in the hippocampus of pentetrazole-induced slow kindling rats. J Southern Medical Univ. 2006;26:1132–1135.
- Xiao GS, Yang Y, Sun WY, et al. Study on extraction technology of active ingredients from Bupleurum chinense DC. Huangshi: Lishizhen Medicine and Materia Medica Research; 2008.
- Li R. Analysis of effective components and pharmacological action of bupleurum chinense. Shaanxi J Traditional Chinese Med. 2013;34:750–751.
- Tong H, Tian D, He Z, et al. Polysaccharides from Bupleurum chinense impact the recruitment and migration of neutrophils by blocking fMLP chemoattractant receptor-mediated functions. Carbohydr Polym. 2013;92(2):1071–1077.
- Huan-Le F, Bei-Bei Y, Rong-Li, Yan-Bin C. Research on the mechanism of Chaihu Huangqin medicine based on integrated pharmacology to improve the clinical symptoms of COVID-19. J Hainan Med Univ. 2020;26(12):15–19.
- Li XQ, Song YN, Wang SJ, et al. Saikosaponins: a review of pharmacological effects. J Asian Nat Prod Res. 2018;20(1):1–13.
- Gevrenova R, Zheleva-Dimitrova D, Ruseva S, et al. Cytotoxic and hepatoprotective effects of Bupleurum flavum flavonoids on hepatocellular carcinoma HEP-G2 cells. J Pharmaceut Res Int. 2016;11:1–8.
- Shan Y, Feng X, Dong Y, et al. The advance on the research of chemical constituents and pharmacological activities of bupleurum. Chinese Wild Plant Resour. 2004;4:5–7.
- Dridi S, Laraoui H, Gasmi AI. Phytochemical study of Algerian Bupleurum atlanticum murb (apiaceae). Der Pharmacia Lettre. 2016;8:246–248.
- Lu M, Meng Q, Liu X. Traditional Chinese medicine radix Bupleuri research were reviewed. For alI Health. 2017;11:24.
- Yan M, Yang L, Hou A, et al. Research progress on chemical composition and pharmacological effect of Bupleurum chinense. Formation Traditional Chinese Med. 2018;035:103–109.
- Parvin K, Hasanuzzaman M, Mohsin SM, et al. Coumarin improves tomato plant tolerance to salinity by enhancing antioxidant defense, glyoxalase system and ion homeostasis. Plant Biol J. 2021;23(S1):181–192.
- Bian X, Zhao Q, Bu F, et al. Effect and evaluation of different drying methods on phenylpropanoid compounds in cinnamomi ramulus. Modern Chinese Med. 2020;22:71–75.
- Wang F, Chen H, Ai Y, et al. The effect of coumarin on plant priming. Genom Appl Biol. 2017;036:2094–2096.
- Tan G. Research progress on influencing factors of bupleuri seed germination. Shaanxi J Agric Sci. 2018;064:87–90.
- Dai Y, Zhao Y, Zhang M, et al. The physiological and pharmacological activities of coumarin compounds. Shandong Chem Ind. 2021;50:30–31.
- Sumorek-Wiadro J, Zając A, Bądziul D, et al. Coumarins modulate the anti-glioma properties of temozolomide. Eur J Pharmacol. 2020;881:173207.
- Shruti M, Achyut P, Siddharth M. Coumarin: an emerging antiviral agent. Heliyon. 2020;6:e03217.
- Zhao Y, Liu T, Luo J, et al. Integration of a decrescent transcriptome and metabolomics dataset of Peucedanum praeruptorum to investigate the CYP450 and MDR genes involved in coumarins biosynthesis and transport. Front Plant Sci. 2015;6:996.
- Liu T, Yao R, Zhao Y, et al. Cloning, functional characterization and site-directed mutagenesis of 4-coumarate: coenzyme a ligase (4CL) involved in coumarin biosynthesis in Peucedanum praeruptorum dunn. Front Plant Sci. 2017;8:4.
- Yadav V, Wang Z, Wei C, et al. Phenylpropanoid pathway engineering: an emerging approach towards plant defense. Pathogens. 2020;9(4):312.
- Shi Y, Zhang S, Peng D, et al. De novo transcriptome analysis of Cnidium monnieri (L.) cuss and detection of genes related to coumarin biosynthesis. PeerJ. 2020;8:e10157.
- Ruben V, Lisa S, Carol SK, et al. COSY catalyses trans-cis isomerization and lactonization in the biosynthesis of coumarins. Nat Plants. 2019;5(10):1066–1075.
- Jin Y, Li H, Feng C. Transcriptome-metabolomics analysis and its application in studying drug action mechanism. Biotechnol Bull. 2018;34:68–76.
- Du J. Analysis on the transcriptome of Bupleuri radix through RNA-Seq [master’s thesis]. Xian (China): Shanxi Agricultural University; 2015.
- Zhang M-y, Zhang Y-Q, Li Y-M, et al. Complete plastid genomesof Bupleurum chinense DC. and B. boissieuanum H. Wolff, with a comparative analysis and phylogenetic study of medicinal Bupleurum species. Acta Pharmaceutica Sin. 2021;56:618–629.
- Wilson ID, Gika H, Theodoridis G, et al. Global metabolic profiling procedures for urine using UPLC-MS. Nat Protoc. 2010;5(6):1005–1018.
- Dunn WB, Broadhurst D, Begley P, et al. Procedures for large-scale metabolic profiling of serum and plasma using gas chromatography and liquid chromatography coupled to mass spectrometry. Nat Protoc. 2011;6(7):1060–1083.
- Wen B, Mei Z, Zeng C, et al. metaX: a flexible and comprehensive software for processing metabolomics data. BMC Bioinf. 2017;18(1):14.
- Anne-Laure B, Korbinian S. Partial least squares: a versatile tool for the analysis of high-dimensional genomic data. Briefings Bioinf. 2007;8:32–44.
- Wang J-B, Pu S-B, Sun Y, et al. Metabolomic profiling of autoimmune hepatitis: the diagnostic utility of nuclear magnetic resonance spectroscopy. J Proteome Res. 2014;13(8):3792–3801.
- Haspel JA, Chettimada S, Shaik RS, et al. Circadian rhythm reprogramming during lung inflammation. Nat Commun. 2014;5:4753.
- Heischmann S, Quinn K, Cruickshank-Quinn C, et al. Exploratory metabolomics profiling in the kainic acid rat model reveals depletion of 25-hydroxyvitamin D3 during epileptogenesis. Sci Rep. 2016;6:31424.
- Sreekumar A, Poisson LM, Rajendiran TM, et al. Metabolomic profiles delineate potential role for sarcosine in prostate cancer progression. Nature. 2009;457(7231):910–914.
- Xi C, Xie C, Sun L, et al. Longitudinal metabolomics profiling of Parkinson’s disease-related α-synuclein A53T transgenic mice. PLoS One. 2015;10(8):e0136612.
- Rao G, Sui J, Zhang J. Metabolomics reveals significant variations in metabolites and correlations regarding the maturation of walnuts (Juglans regia L.). Biol Open. 2016;5(6):829–836.
- Salmela L, Rivals E. LoRDEC: accurate and efficient long read error correction. Bioinformatics. 2014;30(24):3506–3514.
- Fu L, Niu B, Zhu Z, et al. CD-HIT: accelerated for clustering the next-generation sequencing data. Bioinformatics. 2012;28(23):3150–3152.
- Shimizu K, Adachi J, Muraoka Y. ANGLE: a sequencing errors resistant program for predicting protein coding regions in unfinished cDNA. J Bioinform Comput Biol. 2006;04(03):649–664.
- Sun L, Luo H, Bu D, et al. Utilizing sequence intrinsic composition to classify protein-coding and long non-coding transcripts. Nucleic Acids Res. 2013;41(17):e166.
- Kong L, Zhang Y, Ye Z, et al. CPC: assess the protein-coding potential of transcripts using sequence features and support vector machine. Nucleic Acids Res. 2007;35(Web Server Issue):W345–W349.
- Finn RD, Coggill P, Eberhardt RY, et al. The pfam protein families database: towards a more sustainable future. Nucleic Acids Res. 2016;44(D1):D279–D285.
- Li A, Zhang J, Zhou Z. PLEK: a tool for predicting long non-coding RNAs and messenger RNAs based on an improved k-mer scheme. BMC Bioinformatics. 2014;15:311.
- Zheng Y, Jiao C, Sun H, et al . iTAK: a program for genome-wide prediction and classification of plant transcription factors, transcriptional regulators, and protein kinases. Mol Plant. 2016;9(12):1667–1670.
- Li W, Jaroszewski L, Godzik A. Tolerating some redundancy significantly speeds up clustering of large protein databases. Bioinformatics. 2002;18(1):77–82.
- Ashburner M, Ball CA, Blake JA, et al. Gene ontology: tool for the unification of biology. The gene ontology consortium. Nat Genet. 2000;25(1):25–29.
- Tatusov RL, Fedorova ND, Jackson JD, et al. The COG database: an updated version includes eukaryotes. BMC Bioinformatics. 2003;4:41–14.
- Kanehisa M, Goto S, Kawashima S, et al. The KEGG resource for deciphering the genome. Nucleic Acids Res. 2004;32(Database Issue):D277–D280.
- Amos B, Rolf A. The SWISS-PROT protein sequence database and its supplement TrEMBL in 2000. Nucleic Acids Res. 2000;28(1):45–48.
- Li B, Dewey CN. RSEM: accurate transcript quantification from RNA-Seq data with or without a reference genome. BMC Bioinform. 2011;12:323.
- Anders S. Analysing RNA-Seq data with the DESeq package. Mol Biol. 2010;43:1–17.
- Shannon P, Markiel A, Ozier O, et al. Cytoscape: a software environment for integrated models of biomolecular interaction networks. Genome Res. 2003;13(11):2498–2504.
- Su K, Wen A, Liu J. Determination of total coumarin in Glehnia littoralis. J North Pharmacy. 2019;16:1–3.
- Tian F, He X, Sun J, et al. Simultaneous quantitative analysis of nine constituents in six Chinese medicinal materials from Citrus genus by high-performance liquid chromatography and high-resolution mass spectrometry combined with chemometric methods. J Sep Sci. 2020;43(4):736–747.
- Kreidl M, Rainer M, Jakschitz T, et al. Determination of phototoxic furanocoumarins in natural cosmetics using SPE with LC-MS. Anal Chim Acta. 2020;1101:211–221.
- Ziegler J, Schmidt S, Strehmel N, et al. Arabidopsis transporter ABCG37/PDR9 contributes primarily highly oxygenated coumarins to root exudation. Sci Rep. 2017;7(1):3704.
- Wu Q, Zhu X, Shen R. Mechanism of boron facilitate root cell wall iron reutilization in iron deficient. J Plant Nutr Fertil. 2019;025:264–273.
- Jian X, Zhao Y, Wang Z, et al. Two CYP71AJ enzymes function as psoralen synthase and angelicin synthase in the biosynthesis of furanocoumarins in Peucedanum praeruptorum dunn. Plant Mol Biol. 2020;104(3):327–337.
- Larbat R, Hehn A, Hans J, et al. Isolation and functional characterization of CYP71AJ4 encoding for the first P450 monooxygenase of angular furanocoumarin biosynthesis. J Biol Chem. 2009;284(8):4776–4785.
- Qin L, Liu WZ. Accumulation and histological localizations of furanocoumarins in Psoralea corylifolia. J Wuhan Botanical Res. 2007;25:360–365.
- Rajniak J, Giehl RFH, Chang E, et al. Biosynthesis of redox-active metabolites in response to iron deficiency in plants. Nat Chem Biol. 2018;14(5):442–450.
- Rafaliya RV, Sakure AA, Parekh MJ, et al . Study of dynamics of genes involved in biosynthesis and accumulation of scopoletin at different growth stages of Convolvulus prostratus Forssk. Phytochemistry. 2021;182:112594.
- Pang L, Wu Y, Pan Y, et al. Insights into exogenous melatonin associated with phenylalanine metabolism in postharvest strawberry. Postharvest Biol Technol. 2020;168:111244.
- Miao L, Zhang Y, Yang X, et al . Colored light-quality selective plastic films affect anthocyanin content, enzyme activities, and the expression of flavonoid genes in strawberry (Fragaria × Ananassa) fruit. Food Chem. 2016;207:93–100.
- Kota P, Guo D, Zubieta C, et al. O-Methylation of benzaldehyde derivatives by "lignin specific" caffeic acid 3-O-methyltransferase. Phytochemistry. 2004;65(7):837–846.
- Lu N, Ma W, Han D, et al. Genome-wide analysis of the catalpa bungei caffeic acid O-methyltransferase (COMT) gene family: identification and expression profiles in normal, tension, and opposite wood. PeerJ. 2019;7:e6520.
- Stanjek V, Miksch M, Lueer P, et al. Biosynthesis of psoralen: mechanism of a cytochrome P450 catalyzed oxidative bond cleavage. Angew Chem Int Ed. 1999;38(3):400–402.