Abstract
The Sugarcane yellow leaf virus (SCYLV) is associated with sugarcane yellow leaf disease (SCYLD) and is considered to be the most economically deleterious emerging pathogen that represents a potential threat and danger to sugarcane cultivation in China. Over the last two decades, high genetic diversity in the SCYLV genotypes was observed worldwide, with a greater chance of YLD incidence for sugarcane injury. SCYLV infection has significantly damaged its economic traits and is responsible for substantial losses in biomass production in sugarcane cultivars. This study aims to identify and analyse sugarcane microRNAs (miRNAs) as therapeutic targets against SCYLV using plant miRNA prediction tools. Mature sugarcane miRNAs are retrieved and are used for hybridisation of the SCYLV. A total of seven common sugarcane miRNAs were selected based on consensus genomic positions. The biologically significant, top ranked ssp-miR528 was consensually predicted to have a potentially unique hybridisation site at nucleotide position 4162 for targeting the ORF5 of the SCYLV genome; this was predicted by all the algorithms used in this study. Then, the miRNA–mRNA regulatory network was generated using the Circos algorithm, which was used to predict novel targets. There are no acceptable commercial SCYLV-resistant sugarcane varieties available at present. Therefore, the predicted biological data offer valuable evidence for the generation of SCYLV-resistant sugarcane plants.
Introduction
Sugarcane yellow leaf virus (SCYLV) is an emerging Polerovirus in the Luteoviridae family. SCYLV is composed of a positive-sense single-stranded RNA (ssRNA) molecule of 5847-5892 nucleotides [Citation1]. SCYLV RNA genome symmetry is organised into six recognised AUG-initiated open reading frames. ORF0 encodes an RNA-silencing suppressor P0 protein that induces cell death. The transcriptions of ORF1 and ORF2 are started simultaneously in a precise manner. They encode genome-linked peptide (VPg) and RNA-dependent RNA polymerase (RdRp), respectively. The viral capsid is composed of predominant coat protein (CP) encoded by the ORF3. ORF4 encodes movement protein (MP), while ORF5 encodes a read-through protein (RT) [Citation2]. SCYLV-infected sugarcane plants were first noticed in Hawaii in 1988 on variety H65-0782, exhibiting yellow leaf syndrome (YLS) [Citation3]. Sugarcane yellow leaf disease (SCYLD) outbreaks caused by SCYLV significantly constrain sugarcane production all over the world. SCYLD-infected commercial sugarcane cultivars yield losses of up to 25% worldwide [Citation4, Citation5]. SCYLV is known to be spread by infected stalk cuttings and by four aphid species. M. sacchari is known as a common sugarcane aphid and works as an efficient SCYLV vector worldwide [Citation6]. SCYLV has been identified and purified from the infected tissues of sugarcane cultivars using a reverse-transcription loop-mediated isothermal amplification (RT-LAMP) assay [Citation7], double antibody sandwich enzyme-linked immunosorbent assay (DAS-ELISA)[Citation8], reverse transcription (RT)-PCR [Citation9], a tissue blot immunoassay (TBIA), serology [Citation10] and microscopy [Citation11].
Plant microRNAs (miRNAs) are endogenous, noncoding, regulatory molecular bigwigs of 19-24 nucleotides that control post-transcriptional level gene regulatory networks [Citation12]. Their precursor RNA (pre-miRNAs) molecules have unique stem-loop hair-pin secondary structures. The miRNA precursors (pre-miRNAs) are processed by the enzyme RNA-polymerase III (Dicer) to generate mature miRNA (mat-miRNA). The mat-miRNAs are incorporated into the effector complex RNA-induced silencing complex (RISC). They identify their target sequence to suppress it at post-transcriptional level [Citation13]. miRNAs and siRNAs (small interfering RNA) are integral parts of plant small RNA (sRNA), which plays a key role in the cytoplasmic pathways of RNA silencing [Citation14].miRNA-mediated RNA silencing is a major source of plant innate immunity [Citation15]. Gene silencing at post transcriptional level is highly implicated in triggering a host plant’s defense against foreign invading viruses. Sugarcane plants have a multilayered innate immune system in the form of miRNAs to combat pathogens. miRNAs play a key role in cell proliferation to target the cell cycle, as well as to regulate multiple signaling pathways. Transgenic plants containing artificial microRNA (amiRNAs) constructs have been utilised to successfully impart resistance against viral infection [Citation16].amiRNA-technology has been demonstrated successfully in crop plants against Potyvirus [Citation17], Tymovirus [Citation18], Cucumovirus [Citation17], Begomovirus [Citation19], Orthotospovirus [Citation17], Potexvirus and Tobamovirus [Citation20]. In the sugarcane genome, 28 mature miRNAs and their precursor hair-pin sequences have been reported, and a subset of these mat-miRNAs in sugarcane should have targets in the SCYLV genome.
This current synergistic computational approach was designed to identify the most effective sugarcane miRNAs against SCYLV infection. In this study, we implemented computational algorithms for the prediction of host-derived miRNA targets against the SCYLV genome as precedents for developing SCYLV resistance in sugarcane cultivars using amiRNA-based technology. amiRNA is an effective technique to generate virus-resistant sugarcane varieties. amiRNAs can provide an efficient mechanism with advantages such as stability, environmental safety and high specificity. amiRNA-based effective resistance was observed in transgenic plants against Cucumber mosaic virus (CMV) infection, which revealed that the amiRNA approach is a more effective strategy than short hairpin RNA-based silencing methodology [Citation17]. Host delivered plant miRNAs repressed the translation mechanism by degrading their mRNA targets to silence gene expression levels [Citation19]. Potential sugarcane miRNAs were also screened to understand complex host–polerovirus interactions. In the present study, siRISC sensitive cleavage sites were identified in the SCYLV genome for the design of valid amiRNAs. The 21-nucleotide (nt) amiRNA sequence should not have mismatches at nucleotides 10 or 11, as these regions are binding sites of miRNA with its target. amiRNAs have the ability to silence a specific viral sequence. amiRNA constructs are highly specific to the silencing of the target gene which gives minimum off-targets effects. As a result silencing expression was stably transferred to future generations [Citation18]. The predicted miRNA can be utilised to transform sugarcane and develop SCYLV-resistant plants.
Materials and methods
Biological data retrieval and viral genome analysis
A total of 28 mature sugarcane (commonly called Saccharum officinarum-microRNA (sof-miRNA) and Saccharum sp.microRNA (ssp-miRNA) and precursor miRNAs were downloaded from the miRBase version 22 (http://mirbase.org/) biological database (Accession numbers MI0001754-MI0001769 and MI0018180-MI0018197) (Table S1 (Supplementary Materials) [Citation21]. We generated the genomic sequence of a SCYLV isolate (SCYLV-CHN-HN1; accession number HQ342888) from the NCBI GenBank. pDRAW32 DNA analysis (AcaClone 1.1.147) was downloaded and used for annotation, editing, analysis and production of graphical output of SCYLV ORFs.
Miranda
miRanda is used to predict genomic miRNA targets and is considered the most commonly implemented standard miRNA-target predictor-scanning computational algorithm. Various algorithmic features are involved for predicting host–virus interactions. These properties include seed-based interaction, RNA-RNA duplex dimerisation, cross-species target conservation, minimum free energy (MFE) and sequence complementarity [Citation22]. An miRanda algorithm (written in C programming language) was obtained from the source website. The miRanda algorithm was run under well-defined standard settings.
RNA22
RNA22 is a novel pattern-recognition algorithm and is considered a friendly user, diverse web server that predicts statistically significant target patterns [Citation23]. The highly sensitive algorithmic features include non-seed-based interactions, MFE, site complementarity and pattern recognition. It does not consider cross-species conservation filters. The RNA22 algorithm was run after setting standard parameters at the following: sensitivity (63%), specificity (61%) and MFE 12.5 Kcal/mol.
RNAhybrid
RNAhybrid is a new flexible online available tool for the easy and rapid prediction miRNA targets. MFE-based hybridisation of miRNA and mRNA is a key feature. Other features include the following: site complementarity, free energy, helix constraints, seed match and target-site abundance [Citation24]. MFE value was selected at a threshold of −20 Kcal/mol for a single target.
psRNATarget
The psRNATarget algorithm is a highly sensitive plant miRNA prediction tool and is accessed using a web server. The psRNATarget algorithm uses reverse complementarity between target viral mRNA region and host miRNAs and can be accessed at http://plantgrn.noble.org/psRNATarget/ [Citation25]. Target-site accessibility is evaluated by calculating the unpaired energy (UPE) in the psRNATarget algorithm. The miRNA–mRNA interaction was computed using user-defined settings, with an expectation cut-off value 7.5.
RNAfold
The RNAfold web server is a major secondary structure prediction tool. Analysis was performed with user-defined settings (minimum free energy and partition function, avoid isolated base pairs and interactive RNA secondary structure plot) [Citation26]. It has been used to calculate MFE around target sites.
Free energy (ΔG) computation
In order to understand the miRNA–mRNA interaction, the free energy (ΔG) of duplex binding was computed. RNAcofold is employed to estimate the ΔG of miRNA–mRNA duplex hetero-dimer binding [Citation27]. Consensus sugarcane miRNAs and corresponding SCYLV target genomic region sequences were processed in the RNAcofold web server under default parameters.
Mapping of miRNA–target interaction
A Circos plot was generated between predicted host-derived miRNAs and SCYLV genes using the Circos algorithm [Citation28].
Statistical analysis
Sugarcane miRNAs that predicted biological data obtained from the computational algorithmic tools were processed into a graphical representation using scripts written on R statistical software [Citation29].
Results
Sugarcane miRNAs’ target loci on SCYLV genome
Using the miRBase (version 22) biological miRNA database, plant miRNA target prediction tools and an in silico-based framework (), we aimed to identify miRNA with a potential to target the SCYLV- CHN-HN1 RNA genome. We accessed the SCYLV- CHN-HN1 genome from GenBank, and computational annotation of protein coding genes was performed (). As miRNA binding to target RNA genome is highly promiscuous, we predicted the binding strength and significance of the 28 sugarcane candidate miRNAs to the SCYLV genome using the four algorithmic tools: miRanda, RNA22 (v2), RNAhybrid, and psRNATarget. In total, 14 sugarcane miRNAs targeting 29 loci were predicted by the miRanda algorithm. RNA22: 14 sugarcane miRNAs and 27 loci. RNAhybrid predicted that 28 sugarcane miRNAs targeted 28 loci. psRNATarget: 12 sugarcane miRNAs and 16 loci (, File S1 and Table S2).
Figure 1. A computational framework of host-delivered miRNA prediction from the SCYLV-CHN-HN1 genome. Flow chart summarising different prediction steps for scrutinising sugarcane-encoded miRNA targets from the SCYLV genome. The biological data represents retrieved sugarcane miRNAs and SCYLV genome. The algorithmic framework composed of four different types of computational tools. The R language was employed to create plots. The biological data were selected for the refinement of in-house scripts/codes.
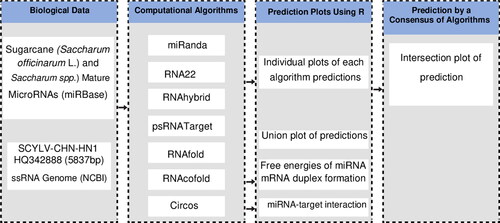
Figure 2. Schematic representation of sugarcane yellow leaf virus genome organisation. Coordinates are based on SCYLV-CHN-HN1 accession number HQ342888. The SCYLV-CHN-HN1 genome consists of 5837 nucleotides and harbours 6 ORFs (0-5).

Figure 3. Venn diagram plot of SCYLV-CHN-HN1 depicting common sugarcane-encoded miRNAs concluded by all the algorithms. In total, 28 loci are targeted in the SCYLV genome by sugarcane-encoded miRNAs. Furthermore, seven sugarcane miRNAs (sof-miR159e, sof-miR167 (a, b), sof-miR168b, ssp-miR169, ssp-miR528 and ssp-miR444b) are predicted by all the computational tools used in this study.
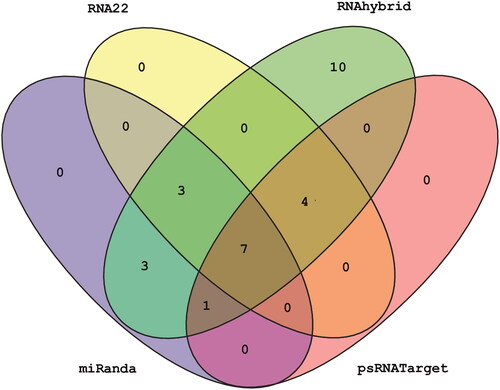
ORF0 encoding RNA silencing suppressor (RSS)
ORF0 encodes the P0 protein, also represented as an RNA silencing suppressor (RSS) of the SCYLV genome. ORF0 (24–784 bp) is composed of 770 bp encoding an RSS protein with 256 amino acids (AA), and it controls the development of viral symptoms. It was observed to be targeted at three positions by the miRanda algorithm: sof-miR168 (a, b) (locus 374) and ssp-miR169 at locus 682 (). The ORF0 sequence is targeted by ssp-miR528 at a unique position (locus 262) by the RNA22 and psRNATarget algorithms ().
ORF1 encoding multifunctional protein
ORF1 (199-2151 bp) is composed of 1952 bp encoding a multifunctional protein (651 AA). The ORF1 sequence is targeted by nine consensual sugarcane miRNAs: sof-miR159 (a, b, c, d and e), sof-miR167 (a, b), sof-miR168b and ssp-miR528, at unique consensus positions (1143, 1646, 2145 and 262, respectively), and by at least two prediction algorithms. The miRanda algorithm predicted that sugarcane miRNAs would target the ORF1: sof-miR168 family at certain nucleotide positions (2140, 374 and 2117), sof-miR408 (e) (locus 888), sof-miR166 (locus 834) and ssp-miR169 (loci 682, 1118) (). RNA22 predicted the following miRNA targets: sof-miR168a (locus 1045), ssp-miR528 (locus 1137) and ssp-miR444 family at locus 1850 (). RNAhybrid predicted four miRNAs: sof-miR408 family at loci positions (965 and 1949) and sof-miR166 (locus 1709) (). The sof-miR396 and ssp-miR444b targeted the ORF1 at nt position 1666, as predicted by psRNATarget ().
ORF2 encoding RNA-dependent RNA polymerase (RdRp)
The ORF2 (1692-3410 bp) is composed of 1781 bp and encodes an RNA-dependent RNA polymerase (RdRp) protein that works as a catalyst for the viral genome replication mechanism. From the consensus of three prediction algorithms, only three miRNAs, sof-miR167 (a, b) and sof-miR169, were hybridised to ORF2 at SCYLV genome locus 2279.miRanda predicted the following miRNAs: sof-miR396 (locus 2661) and ssp-miR166 (locus 2288) (). The RNA22 algorithm predicted the following miRNAs to target the ORF2 sequence: sof-miR159e (locus 2513), ssp-miR169 (locus 2775) and ssp-miR528 (locus 2282). Multiple loci interactions were predicted for the ssp-miR444 family at nucleotide positions (1850, 2507 and 2792) by RNA22 (). Potential miRNAs for targeting ORF2 were predicted to be from the sof-miR159 family at locus 2681, sof-miR167 (a, b) (locus 2923), and sof-miR396 (locus 2321) by RNAhybrid (). Only one miRNA was identified by psRNATarget: ssp-miR444b (locus 2317) ().
ORF3 encoding capsid protein (CP)
Capsid protein (CP, 196 AA) is encoded by the ORF3 (3605-4195 bp). Four sugarcane miRNAs, sof-miR399 (locus 3732), ssp-miR166 (locus 3672) and ssp-miR528 (locus 4162, 4048), were observed to have hybridisation binding sites in ORF3 at four different loci by miRanda (). RNA22 predicted binding of only one miRNA: ssp-miR528 (locus 4162) (). Two important targeting miRNAs were predicted: ssp-miR528 (loci 4162 and 4702) by the psRNATarget ().
ORF4 encoding movement protein (MP)
ORF4 (3636-4088 bp) encodes a movement protein (MP, 150AA) of the SCYLV genome. ORF4 was targeted by three miRNAs: ssp-miR396 (locus 3732), ssp-miR166 (locus 3672) and ssp-miR528 (locus 4048), as indicated by miRanda ().
ORF5 encoding putative aphid transmission factor (PATF)
ORF5 (3605-5635 bp) encodes a read-through protein (RT) of 676 AA size in the SCYLV genome. miRanda predicted seven miRNAs: sof-miR159e (locus 4545), sof-miR396 (locus 5115), ssp-miR528 (locus 5268), ssp-miR444 family (loci 4381) and ssp-miR1432 (locus 4627) ().
Three potential miRNAs were predicted for silencing the SCYLV genome by RNA22: sof-miR168b (locus 5087), ssp-miR528 (locus 5169) and ssp-miR444c-3p (locus 4890) (). Eleven miRNAs: sof-miR156 (locus 5375), sof-miR168a (locus 4961), sof-miR168b (locus 5191), ssp-miR437b (locus 4798), ssp-miR437c, (locus 4597), ssp-miR827 (locus 4638), ssp-miR444 family at loci 4511, ssp-miR1128 (locus 4993) and ssp-miR1432 (locus 5482), were detected by RNAhybrid (). psRNATarget identified two miRNAs: sof-miR168b (locus 5620) and ssp-miR528 (locus 4702) ().
Visualization of miRNA target
For the miRNA–host gene interaction analysis, we constructed Circos plots to combine the biologically credible information in a precise manner. The mapped sugarcane miRNAs are depicted in the SCYLV genome (). In order to ensure the highest level of visual clarity for improved readability, we analysed sugarcane miRNAs and their SCYLV targets, as predicted by all the algorithms used in this study.
Figure 5. A schematic interaction diagram of sugarcane–target interaction in the SCYLV genome is depicted. Circos plot summarising the sugarcane miRNAs predicted by the algorithm miRanda in SCYLV genome. The red, green, purple, brown and blue colored lines show SCYLV genomic components (ORF0-5). Predicted sugarcane miRNAs containing target ORFs of the SCYLV genome by each algorithm are interconnected with colored lines.
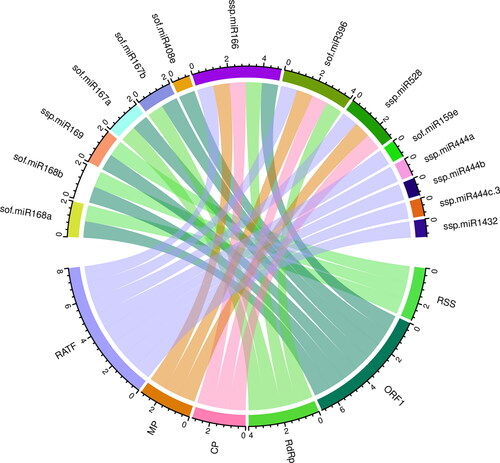
Prediction of consensual sugarcane miRNAs
Of the 28 targeting mature sugarcane miRNAs, only 7 sugarcane miRNAs (sof-miR159e, sof-miR167 (a, b), sof-miR168b, ssp-miR169, ssp-miR528 and ssp-miR444b) were detected by union of consensus between the multiple algorithms (). Nine consensual sugarcane miRNAs showed consensus hybridisation binding sites at the common locus; these were confirmed by two algorithms (). Interestingly, four consensus miRNAs (sof-miR167 (a, b), ssp-miR169 and ssp-miR528, at unique positions (2279, 2277 and 4437, respectively), were predicted to have potential hybridisation biding sites at the common locus; this was confirmed by three algorithms ().ssp-miR528 was the only targeting sugarcane miRNA that had potential hybridisation sites at common locus 4162, which was predicted by all four of the algorithms as shown in (). The ssp-miR528 was encoded at three different common genomic loci (262, 4436 and 4162); this was confirmed by at least two of the algorithms ().
Figure 6. Intersection plot representing consensus sugarcane miRNAs predicted by at least two algorithms at common loci. Color codes are given within the figure. Minimum free energy (miRanda, RNA22 and RNAhybrid) and expectation cut-off score (psRNATarget) are indicated.
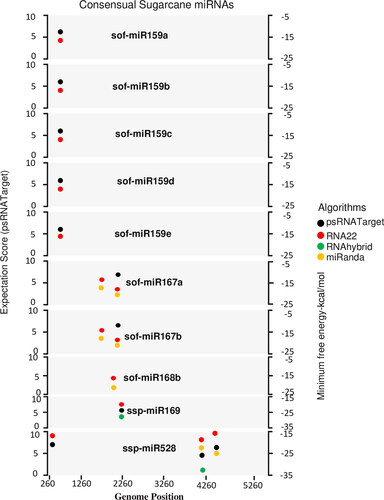
The ssp-miR528 had multiple inferred target interactions in the gene regulatory network and had hybridisation sites at different genomic loci: 4048, 5268, 5169, 2282, 1137 and 4702. These findings were confirmed by at least one algorithm (Table S1, supplementary material). The CP and RT gene sequence was targeted by ssp-miR528 at consensus position 4162.
We generated a Circos plot to integrate biological data from consensus sugarcane miRNAs and their predicted SCYLV genomic target genes ().
Secondary structure prediction and validation
Consensus sugarcane miRNAs were used for the determination of stable secondary structures of the precursor sequences. MFE (minimum free energy) was the most crucial characteristic for structure determination. The predicted secondary structures of seven precursors were finalised (). In the current study, MFE ranged from −48.5 to 107.5 (−kcal/mol) for the seven consensus miRNAs (Table S3, supplementary material). Due to high variability in the sequence of precursor miRNAs, the precursor miRNAs were characterised and validated by the minimum free energy index (MFEI). The value of MFEI ranged from −0.83 to −1.262 for the consensus miRNAs (Table S3, supplementary material).
Prediction of miRNA–mRNA interaction
Free energy (ΔG) is a key component for estimating the miRNA–mRNA interaction. Four consensual sugarcane miRNAs were used to estimate the (ΔG). These were sof-miR167 (a, b) (ΔG: −18.20 kcal/mol) and ssp-miR528 (ΔG: −21.10 kcal/mol).
Discussion
Over the last two decades, an emerging virus, SCYLV, has affected the yield and quality of sugarcane production in Pakistan and China. Gene silencing of sugarcane-infecting virus genome was triggered using host-derived miRNAs through amiRNA-based technology [Citation18]. Recently, miRNA has emerged as a novel endogenous target for gene expression and regulation, and it has been utilised for the genetic improvement of crops in order to combat plant viruses. amiRNA-based silencing of the target RNA or DNA viral genome is an effective and novel approach that has been implemented successfully to boost viral resistance in crops [Citation20]. In the current study, mature sugarcane miRNAs (sof-miR159e, sof-miR167 (a, b), sof-miR168b, ssp-miR169, ssp-miR528 and ssp-miR444b) were selected to develop an SCYLV-resistant sugarcane cultivar, and their interactions with the ORF0, ORF3, ORF4 and ORF5 of SCYLV were observed. This study indicated that ssp-miR528 was selectively employed by SCYLV.
Three computational algorithms (miRanda, RNA22 and psRNATarget) identified the consensus hybridisation site of ssp-miR528 at locus (4162), while RNAhybrid predicted a binding site in the same region at locus 4154. Intriguingly, the MFEs of the consensus target pair were calculated to be −22.93 kcal/mol (miRanda), 19.6 kcal/mol (RNA22) and 32.3 Kcal/mol (RNAhybrid). These are all high and the expectation cut-off is 5.0 (). A lower expectation value indicates a high correlation between miRNA and its target candidate [Citation25]. Experimental studies have revealed a crucial correlation of MFE between the translation repression and the target-binding site of the seed sequence [Citation30]. In order to assess the thermodynamic stability of the miRNA–mRNA duplex, MFE is a key factor to monitor site accessibility for the accurate prediction of a secondary duplex structure [Citation31]. High stability of the RNA duplex is observed due to the strong hybridisation binding of miRNA to mRNA ().
In this study, we designed three approaches at individual, union and intersection levels to control false-positive results. The union approach depends upon the combination of several computational algorithms when predicting true or false targets. Using this approach, the sensitivity level of predicted targets increased by decreasing specificity. In contrast to this approach, the intersectional level of study depends entirely upon the combination of two or more tools that result in the high specificity of predicted targets due to a decrease in sensitivity. Our results showed we have achieved the best results with high performance for predicting and estimating novel targets using both computational approaches, as shown in and Citation6.
Several studies have suggested the gene silencing of target viruses using host-delivered miRNAs using computational algorithms. Genome-wide identification and comprehensive analysis of highly potential candidate miRNA targets against plant viruses have therefore been discovered [Citation32–34]. The current study was designed by an equal novel computational approach for predicting novel targets against SCYLV in order to combat Polereovirus infection in sugarcane cultivars.
The development of varietal-resistant sugarcane to combat viral infection is the preferred way to control yield and quality losses. However, gene pyramiding of desirable agronomic traits with SCYLV resistance is challenging due to the complex sugarcane genome. The high regeneration efficiency of sugarcane callus, the predicted miRNA (ssp-miR528), can be utilised to develop sugarcane cultivars that are resistant to SCYLV. RNAi technology has been widely used to screen host-delivered factors against viruses, as well as to discover novel cellular functions. Here, we retrieved experimentally validated mature sugarcane miRNAs with annotated targets of the SCYLV genome. An amiRNA-based construct was designed to combat SCYLV in sugarcane cultivars that harbour a modified miRNA/miRNA* sequence in a duplex of the precursor (ssp-MIR528), as shown in .
Figure 9. Schematic representation of amiRNA-mediated gene-silencing strategy and determinants for experimental workflow was designed to develop transgenic sugarcane cultivars. The candidate amiRNA is the consensus ssp-MIR528 precursor, and it is designed after miRNA/miRNA duplex replacement. More pre-amiRNA is processed to develop a mature amiRNA/amiRNA* duplex.
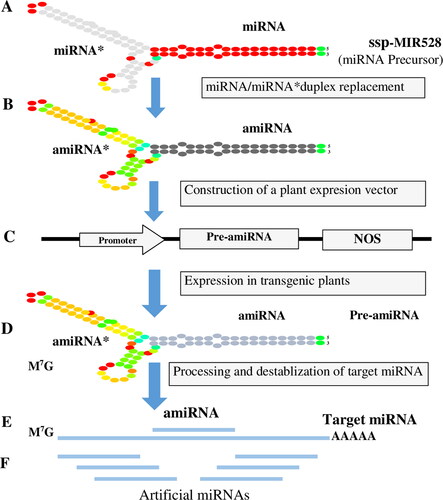
In conclusion, our computationally designed framework for the silencing of the SCYLV genome could offer a novel method for the development of current antiviral agents. The ssp-miR528 has high drought tolerance in the sugarcane hybrid RB867515 cultivar [Citation35]. It is involved in the regulation of SsCBP1 factor and has a monocot lineage during miRNA-based posttranscriptional regulation [Citation36]. ssp-miR528 is involved in depressing the transcriptional activities of target transcripts (pectin acetyl esterase and endopolygalacturonase). ssp-miR528 is a kind of copper miRNA, and it was up-regulated during A. avenae infection in plants. The expression profile was validated with by qRT-PCR analysis [Citation37].
Only a few host-derived miRNA predictions against crop viruses are currently available in the literature. Thus, the current study expands the body of preexisting scholarship. Furthermore, our two earlier studies provide computational support for the control of Badnaviruses in sugarcane cultivars using sof-miR396 [Citation32] and sof-miR159 [Citation38]. The expression of ssp-miR528 in transgenic sugarcane cultivars to silence target genes of the SCYLV genome can further enhance our understanding of important host–virus-related interactions.
Conclusions and recommendations
Since the discovery of RNAi-based gene silencing technology, many laboratories around the world have demonstrated the expression of host-delivered miRNAs against viruses in crop plants. In the current study, ssp-miR528 was identified as the most effective sugarcane miRNA to interact with the SCYLV-CHN-HN1 genome. Based on our reported findings, ssp-miR528 may constitute a potential and effective therapeutic approach to cure SCYLV-CHN-HN1 infection in sugarcane cultivars. Pathological consequences are required to further validate large transgenic sugarcane cultivar development. Therefore, a future challenge will be to identify the critical targets of the ssp-miR528 involved in silencing the SCYLV-CHN-HN1 genome, as well as to establish their contribution to a genome-editing-based transformation system. Predicted novel targets can be engineered for the development of SCYLV-resistant sugarcane cultivars using sugarcane transformation techniques.
Author contributions
M.A.A. and S.Z. conceived the study and wrote the manuscript. F.A., M.A.A., X.H., X.F, J.K and L.S performed the bioinformatics analysis. M.A.A., X.F and L.S analysed the data. All authors read and approved the final manuscript.
Supplemental Material
Download Zip (290.1 KB)Acknowledgments
M.A.A acknowledges the fellowship from TYSP, Ministry of Science and Technology, Government of China. The authors acknowledge Haikou Experimental Station, Chinese Academy of Tropical Agricultural Sciences, Haikou, China.
Disclosure statement
No potential conflict of interest was reported by the authors.
Additional information
Funding
References
- D’Arcy C, Domier L. Family Luteoviridae. In: Fauquet CM, Mayo MA, Maniloff J, Desselberger U, Ball LA, editors. Virus Taxonomy. VIIIth Report of International Committee on Taxonomy of Viruses; 2005. London: Elsevier/Academic Press.
- Mangwende T, Wang M-L, Borth W, et al. The P0 gene of sugarcane yellow leaf virus encodes an RNA silencing suppressor with unique activities. Virology. 2009;384(1):38–50.
- Schenck S. Yellow leaf syndrome–a new disease of sugarcane. Report of HSPA Experiment Station, 1990. p. 98.
- Rott P, Mirkov TE, Schenck S, et al. Recent advances in research on sugarcane yellow leaf virus, the causal agent of sugarcane yellow leaf. Tunbridge Wells, UK: Sugar Cane International; 2008;26:18–27.
- Holkar SK, Balasubramaniam P, Kumar A, et al. Present status and future management strategies for sugarcane yellow leaf virus: a major constraint to the global sugarcane production. Plant Pathol J. 2020;36(6):536–557.
- Guohui Z, Junguang L, Donglin X, et al. Occurrence of sugarcane yellow leaf virus in South China and its transmission by the sugarcane–colonizing aphid, ceratovacuna lanigera. Zhongguo Nong ye ke xue = Zhongguo Nongye Kexue. 2006; 39(10):2023–2027.
- Amata RL, Fernandez E, Filloux D, et al. Prevalence of sugarcane yellow leaf virus in sugarcane-producing regions in Kenya revealed by reverse-transcription loop-mediated isothermal amplification method. Plant Dis. 2016;100(2):260–268.
- Comstock J, Irey M, Lockhart B, et al. Incidence of yellow leaf syndrome in CP cultivars based on polymerase chain reaction and serological techniques. Sugar Cane. 1998;4:21–24.
- Xie Y, Wang M, Xu D, et al. Simultaneous detection and identification of four sugarcane viruses by one-step RT-PCR. J Virol Methods. 2009;162(1-2):64–68.
- Schenck S, Hu J, Lockhart B. Use of a tissue blot immunoassay to determine the distribution of sugarcane yellowleaf virus in Hawaii. Sugar Cane (United Kingdom), 1997.
- Madugula S, Gali U. Detection of sugarcane yellow leaf virus (SCYLV) causing yellow leaf disease (YLD) of sugarcane using serological and molecular tools. ICPJL. 2018;6(2):58–62.
- Brennecke J, Stark A, Russell RB, et al . Principles of microRNA-target recognition. PLoS Biol. 2005;3(3):e85.
- Brodersen P, Sakvarelidze-Achard L, Bruun-Rasmussen M, et al. Widespread translational inhibition by plant miRNAs and siRNAs. Science. 2008;320(5880):1185–1190.
- Baulcombe D. RNA silencing in plants. Nature. 2004; 431(7006) :356–363.
- Li F, Pignatta D, Bendix D, et al. MicroRNA regulation of plant innate immune receptors. Proc Natl Acad Sci U S A. 2012; 109(5):1790–1795.
- Carbonell A, Kobayashi K, Nishiguchi M, et al.et al. Design, synthesis, and functional analysis of highly specific artificial small RNAs with antiviral activity in plants. in Antiviral resistance in plants. 2019. Humana, New York, NY: Springer; p. 231–246.
- Duan C-G, Wang C-H, Fang R-X, et al. Artificial microRNAs highly accessible to targets confer efficient virus resistance in plants. J Virol. 2008;82(22):11084–11095.
- Niu Q-W, Lin S-S, Reyes JL, et al. Expression of artificial microRNAs in transgenic Arabidopsis thaliana confers virus resistance. Nat Biotechnol. 2006;24(11):1420–1428.
- Ali I, Amin I, Briddon RW, et al. Artificial microRNA-mediated resistance against the monopartite begomovirus cotton leaf curl burewala virus. Virol J. 2013;10(1):231–238.
- Petchthai U, Le Yee CS, Wong S-M. Resistance to CymMV and ORSV in artificial microRNA transgenic Nicotiana benthamiana plants. Sci Rep. 2018; 8(1) :1–8.
- Kozomara A, Birgaoanu M, Griffiths-Jones S. miRBase: from microRNA sequences to function. Nucleic Acids Res. 2019;47(D1):D155–D162.
- John B, Enright AJ, Aravin A, et al. Human microRNA targets. PLoS Biol. 2004;2(11):e363.
- Miranda KC, Huynh T, Tay Y, et al. A pattern-based method for the identification of MicroRNA binding sites and their corresponding heteroduplexes. Cell. 2006;126(6):1203–1217.
- Krüger J, Rehmsmeier M. RNAhybrid: microRNA target prediction easy, fast and flexible. Nucleic Acids Res. 2006;34(Web Server issue):W451–W454.
- Dai X, Zhuang Z, Zhao PX. psRNATarget: a plant small RNA target analysis server (2017 release). Nucleic Acids Res. 2018;46(W1):W49–W54.
- Hofacker IL. Vienna RNA secondary structure server. Nucleic Acids Res. 2003;31(13):3429–3431.
- Bernhart SH, Tafer H, Mückstein U, et al. Partition function and base pairing probabilities of RNA heterodimers. Algorithms Mol Biol. 2006;1(1):3–10.
- Krzywinski M, Schein J, Birol I, et al. Circos: an information aesthetic for comparative genomics. Genome Res. 2009;19(9):1639–1645.
- Gandrud C. Reproducible research with R and R studio. 2013. CRC Press.
- Doench JG, Sharp PA. Specificity of microRNA target selection in translational repression. Genes Dev. 2004;18(5):504–511.
- Peterson SM, Thompson JA, Ufkin ML, et al. Common features of microRNA target prediction tools. Front Genet. 2014;5:23.
- Ashraf F, Ashraf MA, Hu X, et al. A novel computational approach to the silencing of sugarcane bacilliform Guadeloupe a virus determines potential host-derived MicroRNAs in sugarcane (saccharum officinarum L.). PeerJ. 2020;8:e8359.
- Gaafar YZA, Ziebell H. Novel targets for engineering physostegia chlorotic mottle and tomato brown rugose fruit virus-resistant tomatoes: in silico prediction of tomato microRNA targets. PeerJ. 2020;8:e10096.
- Iqbal MS, Jabbar B, Sharif MN, et al. In silico MCMV silencing concludes potential host-derived miRNAs in maize. Front Plant Sci. 2017;8:372.
- Gentile A, Dias LI, Mattos RS, et al. MicroRNAs and drought responses in sugarcane. Front Plant Sci. 2015; 6:58.
- Zanca AS, Vicentini R, Ortiz-Morea FA, et al. Identification and expression analysis of microRNAs and targets in the biofuel crop sugarcane. BMC Plant Biol. 2010;10(1) :260–213.
- Tavares EQ, De Souza AP, Romim GH, et al. The control of endopolygalacturonase expression by the sugarcane RAV transcription factor during aerenchyma formation. J Exp Bot. 2019;70(2):497–506.
- Ashraf MA, Feng X, Hu X, et al. In silico identification of sugarcane (Saccharum officinarum L.) genome encoded microRNAs targeting sugarcane bacilliform virus. PLoS ONE 2022;17(1):e0261807.