ABSTRACT
In the context of global research in snow-affected regions, research in the Australian Alps has been steadily catching up to the more established research environments in other countries. One area that holds immense potential for growth is hydrological modelling. Future hydrological modelling could be used to support a range of management and planning issues, such as to better characterise the contribution of the Australian Alps to flows in the agriculturally important Murray-Darling Basin despite its seemingly small footprint. The lack of recent hydrological modelling work in the Australian Alps has catalysed this review, with the aim to summarise the current state and to provide future directions for hydrological modelling, based on advances in knowledge of the Australian Alps from adjacent disciplines and global developments in the field of hydrologic modelling. Future directions proffered here include moving beyond the previously applied conceptual models to more physically based models, supported by an increase in data collection in the region, and modelling efforts that consider non-stationarity of hydrological response, especially that resulting from climate change.
1. Introduction
Snow-affected mountainous areas globally are colloquially termed ‘water towers’, owing to their role in the storage of water in the form of snow during winter before its release as snowmelt come spring (Simpkins Citation2018; Viviroli and Weingartner Citation2004). On continental Australia, an area of approximately 5,000 km2 lies above the snowline (Bilish, McGowan, and Callow Citation2018; Pepler, Trewin, and Ganter Citation2015), known as the Australian Alps, with 1,675 km2 experiencing snow cover lasting 2 months or more (Sanecki et al. Citation2006). Only about 5% of the area is above the treeline and qualified as ‘alpine’, with the rest being ‘subalpine’ for being below the treeline (Bilish, McGowan, and Callow Citation2018; Costin et al. Citation2000). This unique environment is a water resource to the agriculturally important Murray-Darling Basin (Brown and Millner Citation1989; S. Y. U. Schreider, Whetton, et al. Citation1997; Worboys, Good, and Spate Citation2011) as well as hydro-power generation (Domicelj Citation1980; Hardman Citation1968), the mecca of snow sports and snow tourism in Australia (Bicknell and Mcmanus Citation2006; K. J. Hennessy et al. Citation2008; König Citation1999; Morrison and Pickering Citation2013; Pickering and Buckley Citation2010), and home to many distinctive flora and fauna (Costin et al. Citation2000; Happold Citation1998). It also holds great Indigenous cultural significance (Lennon Citation2003). For example, Indigenous groups from areas adjacent to the high country would move into the mountains in pursuit of Bogong moth (Agrotis infusa) during the moths migration, a tradition that has recently been revived (Searby Citation2008; Stephenson et al. Citation2020). Despite its wide-reaching importance, research in hydrological modelling of the Australian Alps lags that of countries and continents where snow is more prominent.
Runoff from the majority of the Australian Alps region flows into the Murray-Darling Basin (MDB) (), providing water resources to Australia’s food bowl. It has been estimated that between 20% and 29% of water in the MDB is sourced from the Australian Alps (Brown and Millner Citation1989; Reinfelds et al. Citation2014; Worboys, Good, and Spate Citation2011), which makes up less than 1% of the total area of the MDB (1,059,000 km2). For example, the Kiewa Basin () in the Victorian portion of the Australian Alps contributes the highest runoff per unit area of all the Murray tributaries (mean annual discharge of 705 GL – equal to 223 mms over a year – from 1,985 km2), driven primarily by snowmelt from the basin’s headwaters between August and October (S. Y. U. Schreider, Whetton, et al. Citation1997). The percentage contribution increases in times of drought across the MDB (Brown and Millner Citation1989; S. Fiddes and Timbal Citation2016; Reinfelds et al. Citation2014). This is a result of these headwater catchments receiving larger volumes of precipitation (Grose et al. Citation2019; Landvogt, Bye, and Lane Citation2008; Sarmadi et al. Citation2019), that is more reliable than precipitation received in the adjacent lowlands (Dey et al. Citation2019; Murphy and Timbal Citation2008). Additionally, these catchments are commonly energy-limited on an annual scale, rather than precipitation-limited like areas downstream (Reinfelds et al. Citation2014), meaning that annual precipitation totals are larger than evaporative demand, resulting in a system where groundwater recharge and runoff is common. There is a seasonal dynamic where these energy-limited catchments can become water-limited (Bilish, Callow, and McGowan Citation2020).
Figure 1. The Murray-Darling Basin with the Australian Alps region highlighted. Major watercourses as defined by the Geoscience Australia are shown. The Murray and Murrumbidgee Rivers are highlighted, as these are the two major snow affected rivers in the basin.
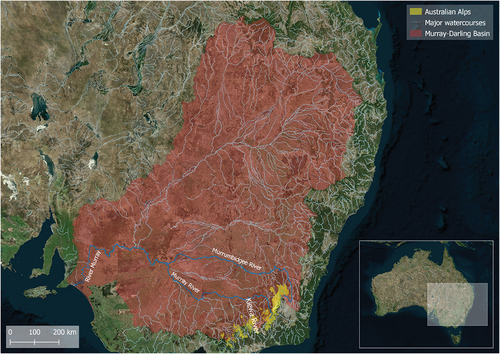
Despite the importance of runoff from the Australian Alps to the MDB, there has been limited research investigating the contribution of snowmelt to water resources in the MDB. This science gap has been recognised for decades (see e.g. Brown and Millner Citation1989). Water sourced from snowmelt in the Australian Alps is used primarily for irrigation and hydropower generation as well as contributing to environmental flows in the Basin (Bilish, McGowan, and Callow Citation2018). The Snowy Hydro Scheme, consisting of nine power stations and 16 major dams, estimates on average 50% of inflows from snowmelt and rain during spring (Snowy Hydro Limited Citation2020).
In this review paper, we aim to summarise the current state of hydrological modelling that explicitly considers snowmelt in the Australian Alps and suggest future directions to guide the field. We juxtapose the state of hydrological modelling in the Australian Alps against other research disciplines (e.g. ecology, climatology) which conduct work in the region, and snow hydrology efforts in other parts of the world. To the best of the authors knowledge, this is the first such review.
2. Snow hydrology and data
Snow hydrology is the study of the water cycle as pertaining to snow, including its formation, accumulation, melting, and impact on water resources and the environment. With an improved understanding of snow hydrology, we can improve our streamflow forecast skills to optimise water reservoir and hydroelectric operations in light of other demands for water downstream (Asadzadeh et al. Citation2014; Zhao, Cai, and Li Citation2019). As precipitation that falls as snow is stored temporally in a snowpack, the processes that drive the hydrological cycle in snow-affected area differ in terms magnitude and timing compared to rain-dominated systems (Dozier Citation1987; Male and Granger Citation1981).
Energy fluxes are important drivers of snowmelt, particularly radiation (short- and long-wave) and turbulent (sensible and latent) energy fluxes (Male and Granger Citation1981). Latent heat flux involves the exchange of energy between the surface, here the snowpack, and the atmosphere where a phase change is involved (e.g. sublimation, condensation) (Ledley Citation2003), while sensible heat flux is the transfer of heat resulting from a difference in temperature between the surface and the atmosphere without a phase change (Schmid and Read Citation2022).
Data available to inform snow hydrology research are varied in type, being broadly classed as in-situ observations, modelling outputs and remotely sensed data (Dong Citation2018). In-situ data are collected in the environment being studied, as opposed to being collected remotely or through model simulations. In-situ data collection has a long history in snow hydrology, including measurements of snowfall amounts starting as early as the 1500s (Dong Citation2018). Although the collection of remotely sensed snow data does not have the long-term history of in-situ measurements, the breadth of data collection is possible at a much wider scale.
Measuring snowfall is notoriously difficult, with undercatch being a significant source of error (Groisman and Legates Citation1994; Rasmussen et al. Citation2012). Measurement error can account for upwards of 50% of solid precipitation, with windy conditions being a large contributor to undercatch (Groisman and Legates Citation1994; Rasmussen et al. Citation2012). Gauge types not suited for capturing solid precipitation also contribute to the issue (Rasmussen et al. Citation2012). Chubb et al. (Citation2015) found undercatch in the Snowy Mountains in the New South Wales portion of the Australian Alps could be as great as 52% of precipitation when a precipitation gauge was not adequately shielded. Average seasonal undercatch in that region varied between 6% and 15% of precipitation (T. Chubb et al. Citation2015). As precipitation is the prime input variable to hydrological models, it follows that additional error would be introduced if poor quality precipitation data are used to force the model. Input errors can be reduced by using gauges that are double fenced as this configuration has been shown to minimise undercatch (Rasmussen et al. Citation2012), or by applying a transfer function to precipitation measurements (Kochendorfer et al. Citation2022; D. Yang et al. Citation2005). Gridded rainfall products are available for Australia (Jones, Wang, and Fawcett Citation2009), but large precipitation underestimates have been emphasised from such products over the Australian Alps (T. H. Chubb et al. Citation2016; Pepler, Trewin, and Ganter Citation2015). In past modelling efforts of snow-affected catchments in the Australian Alps, precipitation data errors were not explicitly addressed (G. Burns, Fowler, and Horne Citation2023; Duggan and Garr Citation2006; S. Y. Schreider and Jakeman Citation2000, Citation2001; S. Y. U. Schreider, Young, and Jakeman Citation1997a, Citation1997, Citation1997c, Citation2001; Tan et al. Citation2005; Trim et al. Citation2023), something also deficient in hydrological modelling in general.
In-situ measurements of snow on the ground can be undertaken manually or by automated instruments. Snow courses are sets of points at which manual measurements of snow depth and snow water equivalent (SWE) are made periodically and were first introduced in the United States in the early twentieth century. With the construction of the Snowy Mountains Scheme beginning in 1949, a similar measurement approach was adopted in New South Wales. More than 50 snow courses were operated during the initial investigations phase though these were consolidated over the following decades. In more recent years, many Australian studies have utilised data from three index snow courses (Spencers Creek, Deep Creek and Three Mile Dam) that are surveyed approximately weekly throughout the snow season and have become the primary long-term record of the Australian snowpack (e.g. Davis Citation2013; Duus Citation1992; Nicholls Citation2005; Pepler, Trewin, and Ganter Citation2015). Additionally, a limited number of the original snow courses have continued to be surveyed less frequently (K. J. Bormann, Evans, and McCabe Citation2014). In the present context, it is important to note that snow courses are typically chosen to inform forecasts of seasonal inflows and are not specifically ‘designed as measures of the absolute amount of SWE in a basin, or to yield SWE estimates across a basin’ (Dressler, Fassnacht, and Bales Citation2006, 706). This means that assumptions of representativeness must be carefully considered when using these data for hydrological modelling (Bilish et al. Citation2019).
The invention of computing dramatically enhanced the capacity for modelling of hydrological systems, allowing collected data to be applied to a larger area (Silberstein Citation2006). However, models are simplified representations of real-world systems (Sorooshian et al. Citation2008) and should be acknowledged as such. Model development should be driven by the research goals, which may include prediction, scenario analysis and diagnostic learning (Razavi et al. Citation2022). In this way, models have been classified based on resultant model properties, namely data-driven compared to process-based () (Beven Citation2005; Devi, Ganasri, and Dwarakish Citation2015; Silberstein Citation2006; Wheater, Jakeman, and Beven Citation1993).
Figure 2. Classification scheme of hydrological models, based on model structure and spatial representation of the area (i.e. catchment) to be modelled, and snowmelt models that are included in hydrological models.
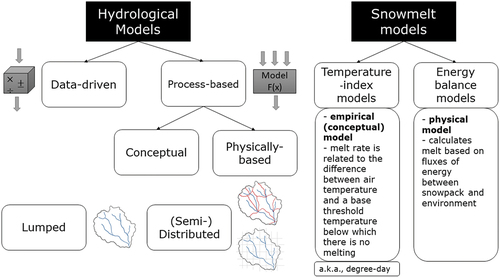
Data-driven models, which relate input and output data mathematically without consideration of physical processes (Corzo et al. Citation2009; Elshorbagy et al. Citation2010; Wheater, Jakeman, and Beven Citation1993), are juxtaposed against process-based models (Beven Citation2005; Wheater, Jakeman, and Beven Citation1993). Process-based models can be further divided into conceptual, where effective parameters describe a conglomerate of processes, or physically-based, where parameters have physical meaning and can be measured in theory () (Devi, Ganasri, and Dwarakish Citation2015; Silberstein Citation2006). On a spatial scale, models can be further divided based on how the catchment to be modelled is represented. Lumped models treat the catchment area as a single homogenous unit, where a single runoff output at the catchment outlet is calculated () (Beven Citation2005; Devi, Ganasri, and Dwarakish Citation2015; Silberstein Citation2006). Semi-distributed and distributed models divide a catchment into smaller sub-catchments or via a grid to be summed later () (Beven Citation2005; Devi, Ganasri, and Dwarakish Citation2015; Silberstein Citation2006).
When a catchment is snow-affected, a hydrological model is modified to account for the storing of precipitation as a snowpack. Subsequent snowmelt can be modelled in two main ways – using a melt rate within a temperature-index model or by evaluating energy fluxes () (K. J. Bormann, Evans, and McCabe Citation2014; S. Y. U. Schreider, Whetton, et al. Citation1997). The melt rate is a calibratable (i.e. adjustable) parameter that relates snowmelt to air temperature, whereas physical models weigh up the energy inputs and loss to the snowpack with residual energy assumed to be used for melting (Beven Citation2005; K. J. Bormann, Evans, and McCabe Citation2014; S. Y. U. Schreider, Whetton, et al. Citation1997). These classifications are not prescriptive, with there being many models that straddle categories.
Remotely sensed data is collected at a distance, often using the sensors that collect information in the electromagnetic spectrum (Fussell, Rundquist, and Harrington Citation1986). Remotely sensed data has been highly useful in hydrological modelling (Ali et al. Citation2023; Kite and Pietroniro Citation1996; X. Xu, Li, and Tolson Citation2014) and snow studies (Andreadis and Lettenmaier Citation2006; Dong Citation2018). Remote-sensing imagery can provide information on several snow properties including snow covered area, snow depth and snow water equivalent (Andreadis and Lettenmaier Citation2006; Dong Citation2018; Kite and Pietroniro Citation1996).
Nevertheless, remotely sensed data collection can struggle in complex mountainous terrain, where clouds can cause confusion for optical sensors (Dong Citation2018). An example can be seen in Bormann et al. (Citation2012), where there were only 15 days over an 11-year period where the Landsat satellite passing over the Australian Alps coincided with clear sky conditions during the winter months. Microwave remote-sensing and LiDAR offers support to overcome this, but is not without drawbacks (Dong Citation2018).
3. Hydrology of the Australian Alps
The hydrology of the Australian Alps received significant attention in 1950s and 60s primarily by prominent Australian ecologist, Alec Baillie Costin, and his colleagues (Costin and Wimbush Citation1961; Costin, Wimbush, and Cromer Citation1959, Citation1960, Citation1961, Citation1964). Their studies linked soils, vegetation and water over multiple landscape types in the Australian Alps, at both alpine and subalpine, and lower montane, elevations. Their research methods included field studies at many sites in different environments to represent the complexity observed in the region. Many future studies expanded the work of Costin and colleagues (Brookhouse, Farquhar, and Roderick Citation2013; Brown Citation1972; A. J. Schwartz Citation2021; A. J. Schwartz, McGowan, and Callow Citation2020).
Australia’s snowpack is prone to high variability, both between seasons and within a single season (Bilish et al. Citation2018, Citation2019; T. H. Chubb, Siems, and Manton Citation2011; Davis Citation2013; K. Hennessy et al. Citation2003; Nicholls Citation2005; Pepler, Trewin, and Ganter Citation2015). The winter snowpack that blankets the Australian Alps differs from snowpacks elsewhere, straddling the maritime and ephemeral classes of the popular Sturm et al. (Citation1995) snow cover classification system. The maritime class is characterised by warm, deep snow cover, whereas the snow cover of the ephemeral class is thin and short-lasting, frequently melting completely in the time between snowfall events (Sturm, Holmgren, and Liston Citation1995). Higher-elevation snow cover in Australia most resembles the maritime class, but experiences higher winter air temperatures than those defined by Sturm et al. (Citation1995) for the maritime class (Sanecki et al. Citation2006). Snow at lower elevations in the Australian Alps is more aligned with the ephemeral class (Sanecki et al. Citation2006).
The distinctive snow cover experienced by the Australian Alps and the spatial variability of the snowpack and its characteristics (Bilish et al. Citation2019; K. J. Bormann et al. Citation2013) make the Australian Alps an interesting study region. While only parts of the snowpack can be classed as ephemeral, the instability of phase (accumulation vs. ablation) throughout the Australian snow season means that melt is commonly generated mid-season and the conventional representation of a snow season as two distinct phases does not fit; the term ‘marginal snow environment’ has been proposed to describe this behaviour (Bilish et al. Citation2019). As the dynamics of snowpacks change globally with the warming climate (López-Moreno et al. Citation2020; Stewart Citation2009), the characteristic qualities of the Australian snowpack may become more widespread across the world in the next few decades, and therefore, lessons learned through more research in the Australian Alps could be leveraged to uncover possible future dynamics of snowpacks elsewhere.
Snowpack dynamics in the Australian Alps have continued to be studied further (e.g. Bormann et al. (Citation2013), Bormann et al. (Citation2014), Bilish et al. (Citation2018), Bilish et al. (Citation2019)), highlighting the variability in snowpack characteristics (i.e. SWE, snow depth, snow density) over small spatial scales (Bilish et al. Citation2019) and between years (K. J. Bormann et al. Citation2013). For example, Bormann et al. (Citation2012) characterised snow cover in Australia spatially and temporally using satellite data, Bormann et al. (Citation2013) improved understanding of snow density in Australia, and Bormann et al. (Citation2014) improved the applicability of Temperature-Index Models (TIMS) in an Australian setting. Bilish et al. (Citation2018) first characterised the energy fluxes controlling snowmelt in an Australian setting and Bilish et al. (Citation2020) investigated the seasonality, interannual variability, and contribution of the snowpack to streamflow in a small subalpine catchment. However, improved runoff modelling of the Australian Alps that incorporates these advances is yet to be implemented.
Snow accumulation dynamics in an Australian setting are influenced by vegetation, with deep snow found in small clearings as a result of snow drifting (Costin et al. Citation1961). Less snow accumulation is observed under canopies, with shallowest snow depths observed in open areas (Costin et al. Citation1961; A. J. Schwartz Citation2021; A. J. Schwartz, McGowan, and Callow Citation2020). Canopies also play an important role during periods of snowmelt (Costin et al. Citation1961), providing for a reduction in shortwave radiation to the snowpack, an increase in longwave radiation, and a varying impact on the turbulent fluxes (Bilish et al. Citation2019; A. J. Schwartz, McGowan, and Callow Citation2020). Fire disturbances impact snow accumulation dynamics, enhancing ablation (i.e. loss) rates and reducing snowpack longevity post winter (A. J. Schwartz Citation2021; A. J. Schwartz, McGowan, and Callow Citation2020).
Advances have been made in modelling snow-affected areas, but these advances tend to be geographically limited, focused predominantly on the Northern Hemisphere (de Jong, Lawler, and Essery Citation2009; Knoben et al. Citation2020; Parajka, Merz, and Blöschl Citation2007). Alpine research in Australia has focused on mountain climatology (T. Chubb et al. Citation2011, Citation2015, Citation2016; Davis Citation2013; Duus Citation1992; Pepler, Trewin, and Ganter Citation2015; Ruddell et al. Citation1990; Slatyer, Cochrance, and Galloway Citation1984) and ecology (e.g. response of alpine vegetation to disturbance, impact of introduced species) (Green and Osborne Citation1981; Hughes Citation2003; Sanecki et al. Citation2006; Scherrer and Pickering Citation2005). Precipitation-focused research across the Australian Alps has featured predominantly, including the analysis of precipitation historical frequencies and trends (T. H. Chubb, Siems, and Manton Citation2011), evaluation of precipitation gauge undercatch (T. Chubb et al. Citation2015), comparison of precipitation datasets (T. H. Chubb et al. Citation2016), and the development of long-term precipitation and related datasets (Davis Citation2013).
Investigations into snow season length and snow cover have occurred, with Slatyer et al. (Citation1984) modelling snow season length, Ruddell et al. (Citation1990) determining relationships between meteorological drivers and snow cover, Duus (Citation1992) modelling snow cover, and Pepler et al. (Citation2015) assessing the impact of climate drivers on snow cover. Recently the soils of the Australian Alps have garnered research interest (Wilson et al. Citation2022). High elevation soils in the Australian Alps are of ecological and hydrological importance, however, the understanding of how these soils form and function is limited (Wilson et al. Citation2022). Threats to alpine soils in Australia are varied (e.g. climate change, fire, feral animals) even though the long-term impacts of these threats have been identified as research priorities (Wilson et al. Citation2022).
Climate change has understandably also been an important focus area for research in the Australian Alps (K. Hennessy et al. Citation2003; McGowan et al. Citation2018; Nicholls Citation2005; Ruddell et al. Citation1990; Slatyer, Cochrance, and Galloway Citation1984), with climate change projected to significantly impact areas with average winter air temperatures close to 0°C, as occurs in the Australian Alpine region (Adam, Hamlet, and Lettenmaier Citation2009; Di Luca, Evans, and Ji Citation2018; McGowan et al. Citation2021; Nolin and Daly Citation2006). Within alpine Australia, climate change has manifested in decadal decreasing trends in maximum snow depth, snow season duration, and snow-covered area (K. J. Bormann, McCabe, and Evans Citation2012; Davis Citation2013; S. L. Fiddes, Pezza, and Barras Citation2015; Green and Pickering Citation2009; K. Hennessy et al. Citation2003; Sánchez-Bayo and Green Citation2013; Whetton, Haylock, and Galloway Citation1996), with spring snow depth particularly affected (Nicholls Citation2005; Pepler, Trewin, and Ganter Citation2015). Warming, rather than reductions in precipitation, are the suggested cause of declining snow depths come spring (Nicholls Citation2005; Pepler, Trewin, and Ganter Citation2015). However, a reduction in autumn and spring precipitation over the period 1958–2012 has also been reported (Theobald, McGowan, and Speirs Citation2016).
Large amounts of long-term hydrologic data have been collected and continue to be collected in the Australian Alps at select sites, mostly to inform hydropower generation (Brown and Millner Citation1989). Research in the Australian Alps has largely relied on a few long-term measurement sites (e.g. Davis Citation2013; Duus Citation1992; Nicholls Citation2005) or small headwater catchments (e.g. Bilish, Callow, and McGowan Citation2018, Citation2019, Citation2020) with findings extrapolated to larger areas (K. Hennessy et al. Citation2003). The scarcity of ground-based data has been overcome to some extent with the use of remotely sensed data (K. J. Bormann, Evans, and McCabe Citation2014; Rasouli et al. Citation2022). But remotely sensed data are yet to be incorporated into hydrological modelling in the Australian Alps as has happened elsewhere (Andreadis and Lettenmaier Citation2006; Dong Citation2018; Kite and Pietroniro Citation1996; Molotch and Margulis Citation2008). Additionally, no study has yet estimated the runoff from the entirety of Australian Alps (Brown and Millner Citation1989; S. Y. Schreider Citation1996), except in general terms (Worboys, Good, and Spate Citation2011), or tried to quantify the contribution of snowmelt to runoff in the snow-affected catchments, with the exception of estimation by Bormann (Citation2013, 224–225). However, it has been suggested that the existing marginality of the snowpack in the Australian Alps reduces the direct control it has on runoff at present, and limits the impact on runoff of future shifts towards proportionally less snowfall, when compared to regions with more persistent snow cover (Bilish, Callow, and McGowan Citation2020).
4. Current state of hydrological modelling in the Australian Alps
Only a handful of local studies considered snow when modelling runoff from snow-affected catchments on mainland Australia (G. Burns, Fowler, and Horne Citation2023; DeWalle and Rango Citation2008, 308; Duggan and Garr Citation2006; S. Y. Schreider and Jakeman Citation2000; Citation2001; S. Y. Schreider et al. Citation1997a, Citation1997, Citation1997c, Citation1996; Tan et al. Citation2005; Trim et al. Citation2023)Footnote1 (Supplementary Material). These studies use one of the six different conceptual rainfall-runoff models () – IHACRES (Identification of unit Hydrographs And Component flows from Rainfall, Evaporation and Streamflow; Jakeman, Littlewood, and Whitehead Citation1990), AWBM (Australian Water Balance Model; Boughton Citation1993), SIMHYD (Simple Hydrology; Chiew, Peel, and Western Citation2002; Li et al. Citation2010), SRM (Snowmelt-Runoff Model; Martinec Citation1975), Wapaba (water partition and balance; Q. J. Wang et al. Citation2011) and GR4J (Perrin, Michel, and Andréassian Citation2003).
Table 1. Past hydrological modelling of snow-affected catchments on mainland Australia (excluding Tasmania).
IHACRES, AWBM, SIMHYD and Wapaba were modified to account for snowmelt with the addition of an empirical, degree-day approach within a temperature-index model (TIM) (). It was noted that the GR4J model used in Trim et al. (Citation2023) was also modified, presumably to GR4JSG (Nepal et al. Citation2017) where a degree-day approach is used. SRM was developed specifically to predict snowmelt runoff and also includes a degree-day approach (DeWalle and Rango Citation2008, chap. 11). Both of the two most recent studies (G. Burns, Fowler, and Horne Citation2023; Trim et al. Citation2023) run at a monthly timestep, differing from the daily or sub-daily timestep of earlier modelling efforts (DeWalle and Rango Citation2008 −308;, pp. 306; Duggan and Garr Citation2006; S. Y. Schreider and Jakeman Citation2000, Citation2001; S. Y. U. Schreider, Young, and Jakeman Citation2001, Citation1997a, Citation1997, Citation1997c; Tan et al. Citation2005).
TIMs are frequently used to model runoff from snowpacks, including ‘marginal’ snow environments characterised by winter air temperatures close to freezing, short snow seasons, and high intra- and inter-season variability (Hock Citation2003). Their popularity owes much to their limited data requirements of only daily temperature and precipitation (S. Y. U. Schreider, Jakeman, Dyer, et al. Citation1997). TIMs are most effective when modelling over longer periods (e.g. months) (DeWalle and Rango Citation2008). Under marginal snow conditions, the effectiveness of TIMs has been questioned (K. J. Bormann, Evans, and McCabe Citation2014). This is because TIMs assume the contribution from energy balance components is constant throughout the season, which is an oversimplification in the Australian Alps and elsewhere (Bilish, McGowan, and Callow Citation2018).
In addition to journal articles, conference papers and textbooks, two theses that modelled runoff from the Australian Alps were found (K. Bormann Citation2013; S. Y. Schreider Citation1996) (see Supplementary Material). Modelling of snow-affected catchments in Schreider (Citation1996) was published in Schreider et al. (Citation1997a), Schreider et al. (Citation1997), and Schreider et al. (Citation1997c). Bormann (Citation2013) applied multiple-snowmelt schemes, including that of Schreider et al. (Citation1997c), within a TIM to estimate snowmelt contributions from the snowpack to the Murray-Darling Basin. Mean annual snowmelt contributions were reported as 7,514 ML/day (K. Bormann Citation2013, 225). In Bormann (Citation2013), only snowmelt was assessed independent of direct rainfall-runoff processes. The peer-reviewed published work to come out of Bormann (Citation2013) (i.e. K. J. Bormann, Evans, and McCabe Citation2014, Citation2012, Citation2013) did not report snowmelt beyond the point-scale (K. J. Bormann, Evans, and McCabe Citation2014).
Beyond published research on hydrological modelling in the Australian Alps, Snowy Hydro Ltd. uses two models to provide hydrological guidance for the operation of the Snowy Mountains Scheme: a semi-distributed daily forecast model with a temperature-index snowmelt component and a tuned artificial neural network model that provides insight on sub-daily variability. Snowy Hydro Ltd. also produces estimates of water volumes stored in the snowpack and probabilistic monthly forecasts of winter-spring inflows using regression models based on measurements at the index snow courses.
On an Australian national scale, several models operate to simulate runoff. The Bureau of Meteorology’s and CSIRO Australian Water Resources Assessment’s AWRA-L and AWRA-R models (Dutta et al. Citation2017; Frost and Wright Citation2018) are widely used (e.g. Wallace et al. (Citation2013), Kunnath-Poovakka et al. (Citation2016), Khan et al. (Citation2020)), but do not consider snow (Dutta et al. Citation2017; Frost and Shokri Citation2021). eWater’s Source model (Dutta et al. Citation2013; Welsh et al. Citation2013) is the Australian National Hydrological Modelling Platform (Carr and Podger Citation2012) and has been tested and used extensively (e.g. Dutta et al. (Citation2012), Rassam et al. (Citation2013), Yang et al. (Citation2017), Zafari et al. (Citation2022)). As Source is a modelling platform with the potential to embed different modelling modules within it, it has the ability to handle snow processes (Dolk, Penton, and Ahmad Citation2020, 202; Iqbal et al. Citation2018; Nepal et al. Citation2017; Rawat, Lahari, and Gosain Citation2018). Although the Source model has been applied to snow-affected catchments in Australia, the necessary modifications were not made to account for snow (Welsh et al. Citation2013).
Another potential modelling framework for snow hydrology in Australia is based on land-surface models (LSMs). LSMs simulate and predict exchanges of energy, water and carbon between the surface and the atmosphere (Blyth et al. Citation2021). In this way, LSMs are viewed as physically based (Figure 3) (Overgaard, Rosbjerg, and Butts Citation2006). LSMs are influenced by, and link, several scientific disciplines including atmospheric sciences and hydrology (Overgaard, Rosbjerg, and Butts Citation2006). Simulation of the water cycle (e.g. precipitation, evaporation, transpiration, infiltration, and runoff) is common in LSMs to predict streamflow, estimate water resources, and assess land-use impacts (Blyth et al. Citation2021). Remotely sensed data can be incorporated into LSMs (Overgaard, Rosbjerg, and Butts Citation2006; X. Xu, Li, and Tolson Citation2014). Examples of LSMs that include snow dynamics are the Community Land Model (CLM) (Lawrence et al. Citation2019), Joint UK Land Environment Simulator (JULES) (Best et al. Citation2011), and the Noah-Multiparameterization Land Surface Model (Noah-MP) (Niu et al. Citation2011; Z.-L. Yang et al. Citation2011). All these models have been applied within an Australian setting (Dumedah and Walker Citation2014; Hostache et al. Citation2020; Kala, Valmassoi, and Hirsch Citation2023; Kumar et al. Citation2021; X. Yang et al. Citation2020), but the Australian Alps have not been the focus region. LSMs applied to snow-affected parts of Australia have intentionally neglected the impact of snow (Lievens et al. Citation2015). The rationale for this omission was an assumption that snow has a limited influence on streamflow in the entirety of the MDB due to the limited spatial and temporal extent (Lievens et al. Citation2015). However, as detailed earlier (Section 1), this has been shown not to be the case.
5. Future of snow-runoff modelling in Australia
There are several research directions to extend the current emerging state of hydrological modelling of snow-affected catchments in Australia, leveraging both recent research in hydrology and related fields in the region and practices employed in the hydrological modelling community generally.
5.1. Model intercomparison and uncertainty analysis
It is common practice to compare model performance of multiple models on a single catchment (or set of catchments) using a common performance metric or metrics (Chiew, Stewardson, and McMahon Citation1993; Coron et al. Citation2012; Donnelly-Makowecki and Moore Citation1999; Franchini and Pacciani Citation1991; Knoben et al. Citation2020) – a practice called model intercomparison (Clark et al. Citation2008). To date, model comparisons have not been undertaken for catchments in the Australian Alps. This is unsurprising given that only six models have been applied to the region (). It would be beneficial to directly compare the models already applied as well as additional hydrological models that include snow dynamics to catchments in the Australian Alps to assess performance differences between models. Identifying the cause of differences in appropriate performance metrics between models could inform future model selection and development for runoff modelling from snow-affected catchments in marginal snow environments.
Although model intercomparison studies are useful by providing a range of possible simulation results typically for the same input data, the reasons behind model performance differences may be harder to understand (Clark et al. Citation2008; Pappas et al. Citation2013). Sensitivity analysis is another type of model diagnostics that is able to give further model insights, including identifying the critical sources of output uncertainty (Do and Razavi Citation2020; Koo et al. Citation2020; Razavi et al. Citation2021; Shin et al. Citation2013). As there are multiple ways uncertainty can be introduced to a modelling exercise (e.g. observed data, model structure, model assumptions, parameter estimation, calibration or a combination of these (Sitterson et al. Citation2018)), it is important to choose a sensitivity analysis method that is holistic (e.g. Koo et al. (Citation2020)). Although a useful form of model assessment, sensitivity analysis is not often undertaken as part of the modelling process (Saltelli et al. Citation2020), and if it is, the analysis may not account for all or most uncertainty sources (Koo et al. Citation2020). Future hydrological modelling in the Australian Alps, and globally, should endeavour to analyse and report on uncertainties (Jakeman, Letcher, and Norton Citation2006; Saltelli et al. Citation2020).
5.2. Non-stationarity and climate change assessment
When the hydrological response of an area changes, it is characterised as hydrologic non-stationary (Ajami et al. Citation2017; Chiew et al. Citation2014; Razavi et al. Citation2015). Hydrologic non-stationarity has already been detected (Ajami et al. Citation2017; Chiew et al. Citation2014; Saft et al. Citation2015). Climate change is likely to alter hydrologic processes, introducing non-stationarity (Chiew et al. Citation2014). Physically based models have been suggested to cope well with non-stationarity, as a result of being comprised of equations that describe theoretically observable state and flux variables that adhere to the laws of physics (e.g. conservation of mass, energy, and momentum) (Fatichi et al. Citation2016). As the parameters of conceptual models often require calibration, the results of which depend on the period calibrated to, and there is potential for non-stationarity to render the current generation of models unable to capture future responses (Duethmann, Blöschl, and Parajka Citation2020). However, Vaze et al. (Citation2010), Chiew et al. (Citation2014) and Fowler et al. (Citation2016) suggest that this can be overcome with well-thoughout calibration periods and techniques (i.e. searching for periods in the historic record that are extreme or similar to climate projections and critically assessing common calibration strategies).
Climate change has impacted the Australian Alps and will continue to do so (e.g. K. Hennessy et al. Citation2003; McGowan et al. Citation2018; Nicholls Citation2005; Whetton, Haylock, and Galloway Citation1996). It is common to use data created under climate scenarios to force models and assess climate change impacts (e.g. Chiew et al. (Citation2009)). Climate change projections have been incorporated into hydrological models in the past (e.g. Coron et al. (Citation2012), Rasmussen et al. (Citation2011), Livneh and Badger (Citation2020), Jung et al. (Citation2012)). Past modelling work that compared uncertainty in climate change impacts, as assessed using a hydrological model, between rain-dominated and snow-dominated catchments found increased uncertainty in the latter catchment (Jung, Moradkhani, and Chang Citation2012). The increased uncertainty in future response to climate change impacts was attributed to increased difficulty in modelling snow processes compared to rain processes (Jung, Moradkhani, and Chang Citation2012). This sentiment is not unique; snow-affected catchments are consistently more difficult to model generally (Knoben et al. Citation2020). In an Australian setting, Schreider et al. (Citation1997), Burns et al. (Citation2023) and Trim et al. (Citation2023) have tested in hydrological implications of climate change on catchments in the Australian Alps using models. Under dry scenarios streamflow from selected Australian alpine catchments was modelled to reduce by over 50% by 2070 (S. Y. U. Schreider, Jakeman, Whetton, et al. Citation1997), with low streamflow years projected to occur more commonly into the future (G. Burns, Fowler, and Horne Citation2023). There is still scope to improve our understanding about the possible impacts of climate change on runoff from the Australian Alps using multiple models of different types forced by climate projections.
5.3. Data collection and modelling
TIMs type models are the only snow representation that has been applied to snow-affected areas of Australia (). Due to the drawbacks of TIMs, physically based models have been proposed as potentially offering more accurate representations of snowpacks and resulting runoff over smaller timescales (Bilish, McGowan, and Callow Citation2018). Models that simulate snowmelt and subsequent runoff based on energy balance components have a long history, proving useful in other snow-dominated areas (Gao et al. Citation2015; Lawrence et al. Citation2019; Niu et al. Citation2011; Pomeroy et al. Citation2007; Z. Wang, Zeng, and Decker Citation2010). Physically based models allow for detailed examination of how different factors influence snowmelt and do not require calibration (however, may still benefit from calibration (Essery et al. Citation2013; Mendoza et al. Citation2015)). As of yet, such models have not been applied in the Australian Alps due to data availability. Only Bilish et al. (Citation2018), Schwartz et al. (Citation2020b) and Schwartz et al. (Citation2020a) have measured energy fluxes to the snowpack in the Australian Alps, and only for relatively short periods (one winter season, two winter seasons, and two winter seasons, respectively). Only Schwartz et al. (Citation2020a) collected flux data at multiple sites, with the other studies focusing on a single site. The single-site flux data collected by Schwartz et al. (Citation2020b) are available for download (McGowan, Schwartz, and Callow Citation2019). The flux data collected were leveraged by several follow-on studies: Schwartz et al. (Citation2020b), Schwartz et al. (Citation2021a), Schwartz et al. (Citation2021b). In other snow-affected environments, there is a long history of flux measurements, for example, at Niwot Ridge and Forest in Colorado (Blanken et al. Citation2009; S. P. Burns et al. Citation2016; Knowles et al. Citation2015; Turnipseed et al. Citation2002). Fortunately, the newly established Australian Mountain Research Facility will instal long-term flux towers in the Australian Alps (Mountain Research Facility Citation2023). The development of physically based models requires detailed additional data (S. Y. U. Schreider, Whetton, et al. Citation1997). Combining a TIM with physical elements is a practical step to improve model performance using available data and recent advancements in the understanding of snow processes (K. J. Bormann, Evans, and McCabe Citation2014) while detailed flux data acquisition is beginning in the Australian Alps. Once these datasets are available, careful consideration will need to be given to their application at the catchment scale given the variability and frequent patchiness of the snow cover (Bilish, McGowan, and Callow Citation2018).
Although efforts have begun to increase instrumentation throughout the Australian Alps, there is scope for models to inform where and what type of in-situ data would be most valuable in reducing model uncertainty. This could be undertaken in two steps: 1) sensitivity analysis to identify the dominate sources of uncertainty (e.g. Abdelhamed et al. (Citation2023), Sheikholeslami et al., (Citation2017), Haghnegahdar et al. (Citation2017) and Koo et al. (Citation2020)) and 2) targeted data collection to reduce the identified uncertainties. Using models to inform optimal design of monitoring networks has proved useful, for example, in groundwater systems (e.g. Fienen et al (Citation2011, Citation2010) and Janardhanan et al. (Citation2020).
Supplementary_V3_Submitted.docx
Download MS Word (48.2 KB)Supplementary_details_V2_Submitted.xlsx
Download MS Excel (44.5 KB)Acknowledgements
Natasha would like to acknowledge Dr Joseph Guillaume for assisting in the setup of the literature review. The authors would like to acknowledge the Traditional Owners of the land on which this research was completed (Canberra and the Snowy Mountains), Ngunawal, Ngunnawal, Ngambri, Ngarigu and Walgal Country, and pay our respects to Elders past, present and emerging. Many thanks to the two anonymous reviewers for their constructive feedback on the manuscript.
Disclosure statement
No potential conflict of interest was reported by the author(s).
Supplementary material
Supplemental data for this article can be accessed online at https://doi.org/10.1080/13241583.2024.2343453
Additional information
Funding
Notes on contributors
Natasha Harvey
Natasha Harvey is a PhD student at The Fenner School of Environment & Society, ANU, having previously obtained a Bachelor of Environmental Systems (Hons) from The University of Sydney and a Master’s degree from The University of Colorado Boulder. Natasha’s research interests include hydrology and water modelling.
Saman Razavi
Dr Saman Razavi is an Associate Professor at the University of Saskatchewan. He holds a PhD in Civil Engineering with a focus on Hydrology and Water Resources from the University of Waterloo, and a Master’s degree in Hydraulic Engineering from Amirkabir University of Technology. His Bachelor’s degree in Civil Engineering is from Iran University of Science and Technology. His research interests include hydrology, water management, and methods to address uncertainty and sensitivity in environmental modeling.
Shane Bilish
Dr Shane Bilish is a Hydrologist at Snowy Hydro Limited. He completed a Bachelor’s and Master’s in Geophysics at The University of Auckland, followed by a PhD in Physical Geography and Hydrology at The University of Western Australia, focusing on snowpack dynamics in the Australian Alps.
Notes
1. Schreider (Citation1996) can be considered a preprint of Schreider et al. (Citation1997c) (Sergei Schreider, pers comms., 6 July 2023).
References
- Abdelhamed, M. S., M. E. Elshamy, S. Razavi, and H. S. Wheater. 2023. “Challenges in Hydrologic-Land Surface Modeling of Permafrost Signatures—A Canadian Perspective.” Journal of Advances in Modeling Earth Systems 15 (3): e2022MS003013. https://doi.org/10.1029/2022MS003013.
- Adam, J. C., A. F. Hamlet, and D. P. Lettenmaier. 2009. “Implications of Global Climate Change for Snowmelt Hydrology in the Twenty-First Century.” Hydrological Processes 23 (7): 962–972. https://doi.org/10.1002/hyp.7201.
- Ajami, H., A. Sharma, L. E. Band, J. P. Evans, N. K. Tuteja, G. E. Amirthanathan, and M. A. Bari. 2017. “On the Non-Stationarity of Hydrological Response in Anthropogenically Unaffected Catchments: An Australian Perspective.” Hydrology and Earth System Sciences 21 (1): 281–294. https://doi.org/10.5194/hess-21-281-2017.
- Ali, M. H., I. Popescu, A. Jonoski, and D. P. Solomatine. 2023. “Remote Sensed And/Or Global Datasets for Distributed Hydrological Modelling: A Review.” Remote Sensing 15 (6): 1642. https://doi.org/10.3390/rs15061642.
- Andreadis, K. M., and D. P. Lettenmaier. 2006. “Assimilating Remotely Sensed Snow Observations into a Macroscale Hydrology Model.” Advances in Water Resources 29 (6): 872–886. https://doi.org/10.1016/j.advwatres.2005.08.004.
- Asadzadeh, M., S. Razavi, B. A. Tolson, and D. Fay. 2014. “Pre-Emption Strategies for Efficient Multi-Objective Optimization: Application to the Development of Lake Superior Regulation Plan.” Environmental Modelling & Software 54:128–141. https://doi.org/10.1016/j.envsoft.2014.01.005.
- Best, M. J., M. Pryor, D. B. Clark, G. G. Rooney, R. L. H. Essery, C. B. Ménard, J. M. Edwards, et al. 2011. “The Joint UK Land Environment Simulator (JULES), Model Description – Part 1: Energy and Water Fluxes.” Geoscientific Model Development 4 (3): 677–699. https://doi.org/10.5194/gmd-4-677-2011.
- Beven, K. 2005. Rainfall-Runoff Modelling: The Primer. 2nd ed. UK: Wiley-Blackwell, Lancaster University.
- Bicknell, S., and P. Mcmanus. 2006. “The Canary in the Coalmine: Australian Ski Resorts and Their Response to Climate Change.” Geographical Research 44 (4): 386–400. https://doi.org/10.1111/j.1745-5871.2006.00409.x.
- Bilish, S. P., J. N. Callow, and H. A. McGowan. 2020. “Streamflow Variability and the Role of Snowmelt in a Marginal Snow Environment.” Arctic, Antarctic, and Alpine Research 52 (1): 161–176. https://doi.org/10.1080/15230430.2020.1746517.
- Bilish, S. P., J. N. Callow, G. S. McGrath, and H. A. McGowan. 2019. “Spatial Controls on the Distribution and Dynamics of a Marginal Snowpack in the Australian Alps.” Hydrological Processes 33 (12): 1739–1755. https://doi.org/10.1002/hyp.13435.
- Bilish, S. P., H. A. McGowan, and J. N. Callow. 2018. “Energy Balance and Snowmelt Drivers of a Marginal Subalpine Snowpack.” Hydrological Processes 32 (26): 3837–3851. https://doi.org/10.1002/hyp.13293.
- Blanken, P. D., M. W. Williams, S. P. Burns, R. K. Monson, J. Knowles, K. Chowanski, and T. Ackerman. 2009. “A Comparison of Water and Carbon Dioxide Exchange at a Windy Alpine Tundra and Subalpine Forest Site Near Niwot Ridge, Colorado.” Biogeochemistry 95 (1): 61–76. https://doi.org/10.1007/s10533-009-9325-9.
- Blyth, E. M., V. K. Arora, D. B. Clark, S. J. Dadson, M. G. De Kauwe, D. M. Lawrence, J. R. Melton, et al. 2021. “Advances in Land Surface Modelling.” Current Climate Change Reports 7 (2): 45–71. https://doi.org/10.1007/s40641-021-00171-5.
- Bormann, K., 2013. “Snowpack Characteristics and Modelling in the Marginal Snowfields of Southeast Australia.” PhD Thesis, UNSW Sydney. https://doi.org/10.26190/unsworks/16741.
- Bormann, K. J., J. P. Evans, and M. F. McCabe. 2014. “Constraining Snowmelt in a Temperature-Index Model Using Simulated Snow Densities.” Journal of Hydrology 517:652–667. https://doi.org/10.1016/j.jhydrol.2014.05.073.
- Bormann, K. J., M. F. McCabe, and J. P. Evans. 2012. “Satellite Based Observations for Seasonal Snow Cover Detection and Characterisation in Australia.” Remote Sensing of Environment 123:57–71. https://doi.org/10.1016/j.rse.2012.03.003.
- Bormann, K. J., S. Westra, J. P. Evans, and M. F. McCabe. 2013. “Spatial and Temporal Variability in Seasonal Snow Density.” Journal of Hydrology 484:63–73. https://doi.org/10.1016/j.jhydrol.2013.01.032.
- Boughton, W. C., 1993. “A Hydrograph-Based Model for Estimating the Water Yield of Ungauged Catchments.” In Hydrology and Water Resources Conference. 317–324. Australia, Canberra: Institute of Engineers.
- Brookhouse, M. T., G. D. Farquhar, and M. L. Roderick. 2013. “The Impact of Bushfires on Water Yield from South-East Australia’s Ash Forests.” Water Resources Research 49 (7): 4493–4505. https://doi.org/10.1002/wrcr.20351.
- Brown, J. A. H. 1972. “Hydrologic Effects of a Bushfire in a Catchment in South-Eastern New South Wales.” Journal of Hydrology 15 (1): 77–96. https://doi.org/10.1016/0022-1694(72)90077-7.
- Brown, J. A. H., and F. C. Millner, 1989. “Aspects of the Meteorology and Hydrology of the Australian Alps.” In Proceedings of the First Fenner Conference, Australian Alps Liaison Committee. Presented at the The Scientific Significance of the Australian Alps. 297–329. Canberra. ( Chapter 17).
- Burns, G., K. Fowler, and A. Horne, 2023. “A Practical Approach to Assessing Climate Change Impacts on Snow Cover and Streamflow in Southeast Australia.” In Presented at the MODSIM2023, 25th Interncational Congress on Modelling and Simulation, Modelling and Simulation Society of Australia and New Zealand. Darwin, NT. https://doi.org/10.36334/modsim.2023.burns.
- Burns, S. P., G. D. Maclean, P. D. Blanken, S. P. Oncley, S. R. Semmer, and R. K. Monson. 2016. “The Niwot Ridge Subalpine Forest US-NR1 AmeriFlux Site – Part 1: Data Acquisition and Site Record-Keeping.” Geoscientific Instrumentation, Methods and Data Systems 5 (2): 451–471. https://doi.org/10.5194/gi-5-451-2016.
- Carr, R., and G. Podger, 2012. “eWater Source - Australia’s Next Generation IWRM Modelling Platform.” Presented at the Hydrology and Water Resources Symposium 2012. 742–749. https://doi.org/10.3316/informit.907715010983417.
- Chiew, F. H. S., M. C. Peel, and A. W. Western. 2002. Application and testing of the simple rainfall-runoff model SIMHYD. In Mathematical models of small watershed hydrology and applications, edited byV. P. Singh and D. Frevert, 335–367. Colorado USA: Water Resources Publications.
- Chiew, F. H. S., N. J. Potter, J. Vaze, C. Petheram, L. Zhang, J. Teng, and D. A. Post. 2014. “Observed Hydrologic Non-Stationarity in Far South-Eastern Australia: Implications for Modelling and Prediction.” Stochastic Environmental Research and Risk Assessment 28 (1): 3–15. https://doi.org/10.1007/s00477-013-0755-5.
- Chiew, F. H. S., M. J. Stewardson, and T. A. McMahon. 1993. “Comparison of Six Rainfall-Runoff Modelling Approaches.” Journal of Hydrology 147 (1–4): 1–36. https://doi.org/10.1016/0022-1694(93)90073-I.
- Chiew, F. H. S., J. Teng, J. Vaze, D. A. Post, J. M. Perraud, D. G. C. Kirono, and N. R. Viney. 2009. “Estimating Climate Change Impact on Runoff Across Southeast Australia: Method, Results, and Implications of the Modeling Method.” Water Resources Research 45 (10). https://doi.org/10.1029/2008WR007338.
- Chubb, T. H., M. J. Manton, S. T. Siems, and A. D. Peace. 2016. “Evaluation of the AWAP Daily Precipitation Spatial Analysis with an Independent Gauge Network in the Snowy Mountains.” Journal of Southern Hemisphere Earth Systems Science 66 (1): 55–67. https://doi.org/10.1071/es16006.
- Chubb, T., M. J. Manton, S. T. Siems, A. D. Peace, and S. P. Bilish. 2015. “Estimation of Wind-Induced Losses from a Precipitation Gauge Network in the Australian Snowy Mountains.” Journal of Hydrometeorology 16 (6): 2619–2638. https://doi.org/10.1175/JHM-D-14-0216.1.
- Chubb, T. H., S. T. Siems, and M. J. Manton. 2011. “On the Decline of Wintertime Precipitation in the Snowy Mountains of Southeastern Australia.” Journal of Hydrometeorology 12 (6): 1483–1497. https://doi.org/10.1175/JHM-D-10-05021.1.
- Clark, M. P., A. G. Slater, D. E. Rupp, R. A. Woods, J. A. Vrugt, H. V. Gupta, T. Wagener, and L. E. Hay. 2008. “Framework for Understanding Structural Errors (FUSE): A Modular Framework to Diagnose Differences Between Hydrological Models.” Water Resources Research 44 (12): 44. https://doi.org/10.1029/2007WR006735.
- Coron, L., V. Andréassian, C. Perrin, J. Lerat, J. Vaze, M. Bourqui, and F. Hendrickx. 2012. “Crash Testing Hydrological Models in Contrasted Climate Conditions: An Experiment on 216 Australian Catchments.” Water Resources Research 48 (5): 48. https://doi.org/10.1029/2011WR011721.
- Corzo, G. A., D. P. Solomatine, M. Hidayat de Wit, M. Werner, S. Uhlenbrook, and R. K. Price. 2009. “Combining Semi-Distributed Process-Based and Data-Driven Models in Flow Simulation: A Case Study of the Meuse River Basin.” Hydrology and Earth System Sciences 13 (9): 1619–1634. https://doi.org/10.5194/hess-13-1619-2009.
- Costin, A. B., L. W. Gay, D. J. Wimbush, and D. Kerr, 1961. “Studies in Catchment Hydrology in the Australian Alps. III. Preliminary Snow Investigations.” Division of Plant Industry Technical Paper No. 15. Melbourne, Australia: Commonwealth Scientific and Industrial Research Organization.
- Costin, A. B., M. Gray, C. Totterdell, and D. Wimbush. 2000. Kosciuszko Alpine Flora. Clayton, Victoria, Australia: CSIRO Publishing. https://doi.org/10.1071/9780643101142.
- Costin, A. B., and D. J. Wimbush, 1961. “Studies in Catchment Hydrology in the Australian Alps. IV. Interception by Trees of Rain, Cloud and Fog.” (Division of Plant Industry Technical Paper No. 16). Commonwealth Scientific and Industrial Research Organization, Melbourne, Australia.
- Costin, A. B., D. J. Wimbush, and R. N. Cromer, 1964. “Studies in Catchment Hydrology in the Australian Alps.” V. Soil Moisture Characteristics and Evapotranspiration (Division of Plant Industry Technical Paper No. 20). Commonwealth Scientific and Industrial Research Organization, Melbourne, Australia.
- Costin, A. B., D. J. Wimbush, and D. Kerr, 1960. Studies in Catchment Hydrology in the Australian Alps. II. Surface Run-Off and Soil Loss (Division of Plant Industry Technical Paper No. 14). Commonwealth Scientific and Industrial Research Organization, Melbourne, Australia.
- Costin, A. B., D. J. Wimbush, D. Kerr, and L. W. Gay, 1959. Studies in Catchment Hydrology in the Australian Alps. I. Trends in Soils and Vegetation (Division of Plant Industry Technical Paper No. 13). Commonwealth Scientific and Industrial Research Organization, Melbourne, Australia.
- Davis, C. J. 2013. “Towards the Development of Long-Term Winter Records for the Snowy Mountains.” Australian Meteorological and Oceanographic Journal 63 (2): 303–313. https://doi.org/10.22499/2.6302.003.
- de Jong, C., D. Lawler, and R. Essery. 2009. “Mountain Hydroclimatology and Snow Seasonality—Perspectives on Climate Impacts, Snow Seasonality and Hydrological Change in Mountain Environments.” Hydrological Processes 23 (7): 955–961. https://doi.org/10.1002/hyp.7193.
- Devi, G. K., B. P. Ganasri, and G. S. Dwarakish, 2015. “A Review on Hydrological Models.” Aquatic Procedia, International Conference on Water Resources, Coastal and Ocean Engineering (ICWRCOE’15). 4: 1001–1007. https://doi.org/10.1016/j.aqpro.2015.02.126.
- DeWalle, D. R., and A. Rango. 2008. Principles of Snow Hydrology. Cambridge, UK: Cambridge University Press.
- Dey, R., S. C. Lewis, J. M. Arblaster, and N. J. Abram. 2019. “A Review of Past and Projected Changes in Australia’s Rainfall.” WIREs Climate Change 10 (3): e577. https://doi.org/10.1002/wcc.577.
- Di Luca, A., J. P. Evans, and F. Ji. 2018. “Australian Snowpack in the NARCliM Ensemble: Evaluation, Bias Correction and Future Projections.” Climate Dynamics 51 (1–2): 639–666. https://doi.org/10.1007/s00382-017-3946-9.
- Dolk, M., D. J. Penton, and M. D. Ahmad. 2020. “Amplification of Hydrological Model Uncertainties in Projected Climate Simulations of the Upper Indus Basin: Does it Matter Where the Water Is Coming From?” Hydrological Processes 34 (10): 2200–2218. https://doi.org/10.1002/hyp.13718.
- Domicelj, S. 1980. “The Australian Snowy Mountains Scheme: National Growth and Regional Development.” Habitat International 5 (5–6): 601–616. https://doi.org/10.1016/0197-3975(80)90004-1.
- Dong, C. 2018. “Remote Sensing, Hydrological Modeling and in situ Observations in Snow Cover Research: A Review.” Journal of Hydrology 561:573–583. https://doi.org/10.1016/j.jhydrol.2018.04.027.
- Donnelly-Makowecki, L. M., and R. D. Moore. 1999. “Hierarchical Testing of Three Rainfall–Runoff Models in Small Forested Catchments.” Journal of Hydrology 219 (3–4): 136–152. https://doi.org/10.1016/S0022-1694(99)00056-6.
- Do, N. C., and S. Razavi. 2020. “Correlation Effects? A Major but Often Neglected Component in Sensitivity and Uncertainty Analysis.” Water Resources Research 56 (3): e2019WR025436. https://doi.org/10.1029/2019WR025436.
- Dozier, J. 1987. “Recent Research in Snow Hydrology.” Reviews of Geophysics 25 (2): 153–161. https://doi.org/10.1029/RG025i002p00153.
- Dressler, K. A., S. R. Fassnacht, and R. C. Bales. 2006. “A Comparison of Snow Telemetry and Snow Course Measurements in the Colorado River Basin.” Journal of Hydrometeorology 7 (4): 705–712. https://doi.org/10.1175/JHM506.1.
- Duethmann, D., G. Blöschl, and J. Parajka. 2020. “Why Does a Conceptual Hydrological Model Fail to Correctly Predict Discharge Changes in Response to Climate Change?” Hydrology and Earth System Sciences 24 (7): 3493–3511. https://doi.org/10.5194/hess-24-3493-2020.
- Duggan, S. J., and A. M. Garr. 2006. Runoff Modelling for Snow-Affected Catchments in the Snowy Mountains, In: 30th Hydrology & Water Resources Symposium: Past, Present & Future, 539–544. Sandy Bay, Tasmania, Australia: Conference Design.
- Dumedah, G., and J. P. Walker. 2014. “Intercomparison of the JULES and CABLE Land Surface Models Through Assimilation of Remotely Sensed Soil Moisture in Southeast Australia.” Advances in Water Resources 74:231–244. https://doi.org/10.1016/j.advwatres.2014.09.011.
- Dutta, D., J. Vaze, S. Kim, J. Hughes, A. Yang, J. Teng, and J. Lerat. 2017. “Development and Application of a Large Scale River System Model for National Water Accounting in Australia.” Journal of Hydrology 547:124–142. https://doi.org/10.1016/j.jhydrol.2017.01.040.
- Dutta, D., W. D. Welsh, J. Vaze, S. S. H. Kim, and D. Nicholls. 2012. “A Comparative Evaluation of Short-Term Streamflow Forecasting Using Time Series Analysis and Rainfall-Runoff Models in eWater Source.” Water Resources Management 26 (15): 4397–4415. https://doi.org/10.1007/s11269-012-0151-9.
- Dutta, D., K. Wilson, W. D. Welsh, D. Nicholls, S. Kim, and L. Cetin. 2013. “A New River System Modelling Tool for Sustainable Operational Management of Water Resources.” Journal of Environmental Management 121:13–28. https://doi.org/10.1016/j.jenvman.2013.02.028.
- Duus, A. L. 1992. “Estimation and Analysis of Snow Cover in the Snowy Mountains Between 1910 and 1991.” Australian Meteorological Magazine 40 (4): 195–204. http://www.bom.gov.au/jshess/papers.php?year=1992.
- Elshorbagy, A., G. Corzo, S. Srinivasulu, and D. P. Solomatine. 2010. “Experimental Investigation of the Predictive Capabilities of Data Driven Modeling Techniques in Hydrology - Part 1: Concepts and Methodology.” Hydrology and Earth System Sciences 14 (10): 1931–1941. https://doi.org/10.5194/hess-14-1931-2010.
- Essery, R., S. Morin, Y. B. Lejeune, and C. Ménard. 2013. “A Comparison of 1701 Snow Models Using Observations from an Alpine Site.” Advances in Water Resources, Snow–Atmosphere Interactions and Hydrological Consequences 55:131–148. https://doi.org/10.1016/j.advwatres.2012.07.013.
- Fatichi, S., E. R. Vivoni, F. L. Ogden, V. Y. Ivanov, B. Mirus, D. Gochis, C. W. Downer, et al. 2016. “An Overview of Current Applications, Challenges, and Future Trends in Distributed Process-Based Models in Hydrology.” Journal of Hydrology 537:45–60. https://doi.org/10.1016/j.jhydrol.2016.03.026.
- Fiddes, S. L., A. B. Pezza, and V. Barras. 2015. “A New Perspective on Australian Snow.” Atmospheric Science Letters 16 (3): 246–252. https://doi.org/10.1002/asl2.549.
- Fiddes, S., and B. Timbal. 2016. “Assessment and Reconstruction of Catchment Streamflow Trends and Variability in Response to Rainfall Across Victoria, Australia.” Climate Research 67 (1): 43–60. https://doi.org/10.3354/cr01355.
- Fienen, M. N., J. E. Doherty, R. J. Hunt, and H. W. Reeves, 2010. “Using Prediction Uncertainty Analysis to Design Hydrologic Monitoring Networks: Example Applications from the Great Lakes Water Availability Pilot Project: U.S. Geological Survey Scientific Investigations.” Report 2010–5159.
- Fienen, M. N., R. J. Hunt, J. E. Doherty, and H. W. Reeves. 2011. Using models for the optimization of hydrologic monitoring. U.S. Geological Survey. https://doi.org/10.3133/fs20113014.
- Fowler, K. J. A., M. C. Peel, A. W. Western, L. Zhang, and T. J. Peterson. 2016. “Simulating Runoff Under Changing Climatic Conditions: Revisiting an Apparent Deficiency of Conceptual Rainfall-Runoff Models.” Water Resources Research 52 (3): 1820–1846. https://doi.org/10.1002/2015WR018068.
- Franchini, M., and M. Pacciani. 1991. “Comparative Analysis of Several Conceptual Rainfall-Runoff Models.” Journal of Hydrology 122 (1–4): 161–219. https://doi.org/10.1016/0022-1694(91)90178-K.
- Frost, A. J., and A. Shokri, 2021. The Australian Landscape Water Balance Model (AWRA-L V7). Technical Description of the Australian Water Resources Assessment Landscape Model Version 7. Bureau of Meteorology Technical Report.
- Frost, A. J., and D. P. Wright, 2018. “The Australian Water Resource Assessment Landscape Model - AWRA-L: Improved Performance and Regional Calibration.” In Water and Communities. Presented at the Hydrology and Water Resources Symposium, Engineers. 933–949. Australia, Melbourne, Australia. https://doi.org/10.3316/informit.127604291992740.
- Fussell, J., D. Rundquist, and J. A. Harrington. 1986. “On defining remote sensing.” Photogrammetric Engineering and Remote Sensing 52 (9): 1507–1511.
- Gao, Y., K. Li, F. Chen, Y. Jiang, and C. Lu. 2015. “Assessing and Improving Noah-MP Land Model Simulations for the Central Tibetan Plateau.” Journal of Geophysical Research: Atmospheres 120 (18): 9258–9278. https://doi.org/10.1002/2015JD023404.
- Green, K., and W. S. Osborne. 1981. “The Diet of Foxes, Vulpes Vulpes (L.), In Relation to Abundance of Prey Above the Winter Snowline in New South Wales.” Wildlife Research 8 (2): 349–360. https://doi.org/10.1071/wr9810349.
- Green, K., and C. M. Pickering. 2009. “The Decline of Snowpatches in the Snowy Mountains of Australia: Importance of Climate Warming, Variable Snow, and Wind.” Arctic, Antarctic, and Alpine Research 41 (2): 212–218. https://doi.org/10.1657/1938-4246-41.2.212.
- Groisman, P. Y., and D. R. Legates. 1994. “The Accuracy of United States Precipitation Data.” Bulletin of the American Meteorological Society 75:215–228. https://doi.org/10.1175/1520-04771994075<0215:TAOUSP>2.0.CO;2.
- Grose, M. R., J. Syktus, M. Thatcher, J. P. Evans, F. Ji, T. Rafter, and T. Remenyi. 2019. “The Role of Topography on Projected Rainfall Change in Mid-Latitude Mountain Regions.” Climate Dynamics 53 (5–6): 3675–3690. https://doi.org/10.1007/s00382-019-04736-x.
- Haghnegahdar, A., S. Razavi, F. Yassin, and H. Wheater. 2017. “Multicriteria Sensitivity Analysis as a Diagnostic Tool for Understanding Model Behaviour and Characterizing Model Uncertainty.” Hydrological Processes 31 (25): 4462–4476. https://doi.org/10.1002/hyp.11358.
- Happold, D. C. D. 1998. “The Subalpine Climate at Smiggin Holes, Kosciuszko National Park, Australia, and Its Influence on the Biology of Small Mammals.” Arctic and Alpine Research 30 (3): 241–251. https://doi.org/10.2307/1551971.
- Hardman, D. J. 1968. “The Snowy Mountains Hydro-Electric Authority: Origins and Antecedents.” Australian Journal of Public Administration 27 (3): 205–236. https://doi.org/10.1111/j.1467-8500.1968.tb00714.x.
- Hennessy, K., P. Whetton, I. Smith, J. Bathols, M. Hutchinson, and J. Sharples. 2003. In New South Wales National Parks and Wildlife Service, New South Wales Department of Infrastructure, Planning and Natural Resources. Aspendale, Victoria, Australia: CSIRO Atmospheric Research.
- Hennessy, K. J., P. H. Whetton, K. Walsh, I. N. Smith, J. M. Bathols, M. Hutchinson, and J. Sharples. 2008. “Climate Change Effects on Snow Conditions in Mainland Australia and Adaptation at Ski Resorts Through Snowmaking.” Climate Research 35:255–270. https://doi.org/10.3354/cr00706.
- Hock, R. 2003. “Temperature Index Melt Modelling in Mountain Areas.” Journal of Hydrology, Mountain Hydrology and Water Resources 282 (1–4): 104–115. https://doi.org/10.1016/S0022-1694(03)00257-9.
- Hostache, R., D. Rains, K. Mallick, M. Chini, R. Pelich, H. Lievens, F. Fenicia, G. Corato, N. E. C. Verhoest, and P. Matgen. 2020. “Assimilation of Soil Moisture and Ocean Salinity (SMOS) Brightness Temperature into a Large-Scale Distributed Conceptual Hydrological Model to Improve Soil Moisture Predictions: The Murray–Darling Basin in Australia As a Test Case.” Hydrology and Earth System Sciences 24 (10): 4793–4812. https://doi.org/10.5194/hess-24-4793-2020.
- Hughes, L. 2003. “Climate Change and Australia: Trends, Projections and Impacts.” Austral Ecology 28 (4): 423–443. https://doi.org/10.1046/j.1442-9993.2003.01300.x.
- Iqbal, M., G. Akhter, A. Ashraf, and S. Ayub. 2018. “Snowmelt Runoff Assessment and Prediction Under Variable Climate and Glacier Cover Scenarios in Astore River Basin, Western Himalayas.” Arabian Journal Geosciences 11 (18): 568. https://doi.org/10.1007/s12517-018-3923-6.
- Jakeman, A. J., R. A. Letcher, and J. P. Norton. 2006. “Ten Iterative Steps in Development and Evaluation of Environmental Models.” Environmental Modelling & Software 21 (5): 602–614. https://doi.org/10.1016/j.envsoft.2006.01.004.
- Jakeman, A. J., I. G. Littlewood, and P. G. Whitehead. 1990. “Computation of the Instantaneous Unit Hydrograph and Identifiable Component Flows with Application to Two Small Upland Catchments.” Journal of Hydrology 117 (1–4): 275–300. https://doi.org/10.1016/0022-1694(90)90097-H.
- Janardhanan, S., D. Gladish, D. Gonzalez, D. Pagendam, T. Pickett, and T. Cui. 2020. “Optimal Design and Prediction-Independent Verification of Groundwater Monitoring Network.” Water 12 (1): 123. https://doi.org/10.3390/w12010123.
- Jones, D. A., W. Wang, and R. Fawcett. 2009. “High-Quality Spatial Climate Data-Sets for Australia.” Australian Meteorological and Oceanographic Journal 58 (4): 233. https://doi.org/10.22499/2.5804.003.
- Jung, I.-W., H. Moradkhani, and H. Chang. 2012. “Uncertainty Assessment of Climate Change Impacts for Hydrologically Distinct River Basins.” Journal of Hydrology 466:73–87. https://doi.org/10.1016/j.jhydrol.2012.08.002.
- Kala, J., A. Valmassoi, and A. L. Hirsch. 2023. “Assessing the Maximum Potential Cooling Benefits of Irrigation in Australia During the “Angry Summer” of 2012/2013.” Weather and Climate Extremes 39:100538. https://doi.org/10.1016/j.wace.2022.100538.
- Khan, M. S., J. Baik, and M. Choi. 2020. “Inter-Comparison of Evapotranspiration Datasets Over Heterogeneous Landscapes Across Australia.” Advances in Space Research 66 (3): 533–545. https://doi.org/10.1016/j.asr.2020.04.037.
- Kite, G. W., and A. Pietroniro. 1996. “Remote Sensing Applications in Hydrological Modelling.” Hydrological Sciences Journal 41 (4): 563–591. https://doi.org/10.1080/02626669609491526.
- Knoben, W. J. M., J. E. Freer, M. C. Peel, K. J. A. Fowler, and R. A. Woods. 2020. “A Brief Analysis of Conceptual Model Structure Uncertainty Using 36 Models and 559 Catchments.” Water Resources Research E2019wr025975 56 (9). https://doi.org/10.1029/2019WR025975.
- Knowles, J. F., S. P. Burns, P. D. Blanken, and R. K. Monson. 2015. “Fluxes of Energy, Water, and Carbon Dioxide from Mountain Ecosystems at Niwot Ridge, Colorado.” Plant Ecology & Diversity 8 (5–6): 663–676. https://doi.org/10.1080/17550874.2014.904950.
- Kochendorfer, J., M. Earle, R. Rasmussen, C. Smith, D. Yang, S. Morin, E. Mekis, et al. 2022. “How Well Are We Measuring Snow Post-SPICE?” Bulletin of the American Meteorological Society 103 (2): E370–E388. https://doi.org/10.1175/BAMS-D-20-0228.1.
- König, U. 1999. “Climate Change and Snow Tourism in Australia.” Geographica Helvetica 54 (3): 147–157. https://doi.org/10.5194/gh-54-147-1999.
- Koo, H., M. Chen, A. J. Jakeman, and F. Zhang. 2020. “A Global Sensitivity Analysis Approach for Identifying Critical Sources of Uncertainty in Non-Identifiable, Spatially Distributed Environmental Models: A Holistic Analysis Applied to SWAT for Input Datasets and Model Parameters.” Environmental Modelling & Software 127:104676. https://doi.org/10.1016/j.envsoft.2020.104676.
- Kumar, S. V., T. Holmes, N. Andela, I. Dharssi, C. Vinodkumar Hain, C. Peters-Lidard, S. P. Mahanama, K. R. Arsenault, W. Getirana, and A. Nie. 2021. “The 2019–2020 Australian Drought and Bushfires Altered the Partitioning of Hydrological Fluxes.” Geophysical Research Letters 48 (1): e2020GL091411. https://doi.org/10.1029/2020GL091411.
- Kunnath-Poovakka, A., D. Ryu, L. J. Renzullo, and B. George. 2016. “The Efficacy of Calibrating Hydrologic Model Using Remotely Sensed Evapotranspiration and Soil Moisture for Streamflow Prediction.” Journal of Hydrology 535:509–524. https://doi.org/10.1016/j.jhydrol.2016.02.018.
- Landvogt, P. K., J. A. Bye, and T. P. Lane. 2008. “An Investigation of Recent Orographic Precipitation Events in Northeast Victoria.” Australian Meteorological Magazine 57 (3): 235–247. http://www.bom.gov.au/jshess/papers.php?year=2008.
- Lawrence, D. M., R. A. Fisher, C. D. Koven, K. W. Oleson, S. C. Swenson, G. Bonan, N. Collier, B. Ghimire, L. van Kampenhout, and D. Kennedy. 2019. “The Community Land Model Version 5: Description of New Features, Benchmarking, and Impact of Forcing Uncertainty.” Journal of Advances in Modeling Earth Systems 11 (12): 4245–4287. https://doi.org/10.1029/2018MS001583.
- Ledley, T. S. 2003. “Energy Balance Model, Surface.” In Encyclopedia of Atmospheric Sciences, edited by J.R. Holton, 747–754. Oxford: Academic Press. https://doi.org/10.1016/B0-12-227090-8/00150-0.
- Lennon, J. L. 2003. “The Cultural Significance of Australian Alpine Areas.” Historic Environment 17 (2): 14–17. https://australia.icomos.org/publications/historic-environment/he-vol-17-no-2-2003-celebrating-mountains/.
- Lievens, H., S. K. Tomer, A. Al Bitar, G. J. M. De Lannoy, M. Drusch, G. Dumedah, H.-J. Hendricks Franssen, et al. 2015. “SMOS Soil Moisture Assimilation for Improved Hydrologic Simulation in the Murray Darling Basin, Australia.” Remote Sensing of Environment 168:146–162. https://doi.org/10.1016/j.rse.2015.06.025.
- Livneh, B., and A. M. Badger. 2020. “Drought Less Predictable Under Declining Future Snowpack.” Nature Climate Change 10 (5): 452–458. https://doi.org/10.1038/s41558-020-0754-8.
- Li, C., H. Wang, J. Liu, D. Yan, F. Yu, and L. Zhang. 2010. “Effect of Calibration Data Series Length on Performance and Optimal Parameters of Hydrological Model.” Water Science and Engineering 3:378–393. https://doi.org/10.3882/j.issn.1674-2370.2010.04.002.
- López-Moreno, J. I., J. W. Pomeroy, E. Alonso-González, E. Morán-Tejeda, and J. Revuelto. 2020. “Decoupling of Warming Mountain Snowpacks from Hydrological Regimes.” Environmental Research Letters 15 (11): 114006. https://doi.org/10.1088/1748-9326/abb55f.
- Male, D. H., and R. J. Granger. 1981. “Snow Surface Energy Exchange.” Water Resources Research 17 (3): 609–627. https://doi.org/10.1029/WR017i003p00609.
- Martinec, J. 1975. “Snowmelt-Runoff Model for Stream Flow Forecasts.” Nordic Hydrology 6 (3): 145–154. https://doi.org/10.2166/nh.1975.0010.
- McGowan, H., K. Borthwick, A. Schwartz, J. N. Callow, S. Bilish, and S. Browning. 2021. “Atmospheric Rivers: An Overlooked Threat to the Marginal Snowpack of the Australian Alps.” Journal of Hydrometeorology 22 (10): 2521–2532. https://doi.org/10.1175/JHM-D-20-0293.1.
- McGowan, H., J. N. Callow, J. Soderholm, G. McGrath, M. Campbell, and J. Zhao. 2018. “Global Warming in the Context of 2000 Years of Australian Alpine Temperature and Snow Cover.” Scientific Reports 8 (1): 4394. https://doi.org/10.1038/s41598-018-22766-z.
- McGowan, H., A. Schwartz, and N. Callow. 2019. “Synoptic Weather Energy Balance in the Australia’s Snowy Mountains.” https://doi.org/10.14264/uql.2019.691.
- Mendoza, P. A., M. P. Clark, M. Barlage, B. Rajagopalan, L. Samaniego, G. Abramowitz, and H. Gupta. 2015. “Are We Unnecessarily Constraining the Agility of Complex Process-Based Models?” Water Resources Research 51 (1): 716–728. https://doi.org/10.1002/2014WR015820.
- Molotch, N. P., and S. A. Margulis. 2008. “Estimating the Distribution of Snow Water Equivalent Using Remotely Sensed Snow Cover Data and a Spatially Distributed Snowmelt Model: A Multi-Resolution, Multi-Sensor Comparison.” Advances in Water Resources, Hydrologic Remote Sensing 31 (11): 1503–1514. https://doi.org/10.1016/j.advwatres.2008.07.017.
- Morrison, C., and C. M. Pickering. 2013. “Perceptions of Climate Change Impacts, Adaptation and Limits to Adaption in the Australian Alps: The Ski-Tourism Industry and Key Stakeholders.” Journal of Sustainable Tourism 21 (2): 173–191. https://doi.org/10.1080/09669582.2012.681789.
- Mountain Research Facility, Australian, 2023. Sites & Data [WWW Document]. Accessed August 24, 2023. https://www.amrf.org.au/amrf-sites/.
- Murphy, B. F., and B. Timbal. 2008. “A Review of Recent Climate Variability and Climate Change in Southeastern Australia.” International Journal of Climatology 28 (7): 859–879. https://doi.org/10.1002/joc.1627.
- Nepal, S., J. Chen, D. J. Penton, L. E. Neumann, H. Zheng, and S. Wahid. 2017. “Spatial GR4J Conceptualization of the Tamor Glaciated Alpine Catchment in Eastern Nepal: Evaluation of GR4JSG Against Streamflow and MODIS Snow Extent.” Hydrological Processes 31 (1): 51–68. https://doi.org/10.1002/hyp.10962.
- Nicholls, N. 2005. “Climate Variability, Climate Change and the Australian Snow Season.” Australian Meteorological Magazine 54 (3): 177–185 http://www.bom.gov.au/jshess/papers.php?year=2005.
- Niu, G.-Y., Z.-L. Yang, K. E. Mitchell, F. Chen, M. B. Ek, M. Barlage, A. Kumar, et al. 2011. “The Community Noah Land Surface Model with Multiparameterization Options (Noah-MP): 1. Model Description and Evaluation with Local-Scale Measurements.” Journal of Geophysical Research: Atmospheres 116 (D12). https://doi.org/10.1029/2010JD015139.
- Nolin, A. W., and C. Daly. 2006. “Mapping “At Risk” Snow in the Pacific Northwest.” Journal of Hydrometeorology 7 (5): 1164–1171. https://doi.org/10.1175/JHM543.1.
- Overgaard, J., D. Rosbjerg, and M. B. Butts. 2006. “Land-Surface Modelling in Hydrological Perspective – a Review.” Biogeosciences 3 (2): 229–241. https://doi.org/10.5194/bg-3-229-2006.
- Pappas, C., S. Fatichi, S. Leuzinger, A. Wolf, and P. Burlando. 2013. “Sensitivity Analysis of a Process-Based Ecosystem Model: Pinpointing Parameterization and Structural Issues.” Journal of Geophysical Research: Biogeosciences 118 (2): 505–528. https://doi.org/10.1002/jgrg.20035.
- Parajka, J., R. Merz, and G. Blöschl. 2007. “Uncertainty and Multiple Objective Calibration in Regional Water Balance Modelling: Case Study in 320 Austrian Catchments.” Hydrological Processes 21 (4): 435–446. https://doi.org/10.1002/hyp.6253.
- Pepler, A., B. Trewin, and C. Ganter. 2015. “The Influence of Climate Drivers on the Australian Snow Season.” Australian Meteorological and Oceanographic Journal 65 (2): 195–205. https://doi.org/10.22499/2.6502.002.
- Perrin, C., C. Michel, and V. Andréassian. 2003. “Improvement of a Parsimonious Model for Streamflow Simulation.” Journal of Hydrology 279 (1–4): 275–289. https://doi.org/10.1016/S0022-1694(03)00225-7.
- Pickering, C. M., and R. C. Buckley. 2010. “Climate Response by the Ski Industry: The Shortcomings of Snowmaking for Australian Resorts.” AMBIO: A Journal of the Human Environment 39 (5–6): 430–438. https://doi.org/10.1007/s13280-010-0039-y.
- Pomeroy, J. W., D. M. Gray, T. Brown, N. R. Hedstrom, W. L. Quinton, R. J. Granger, and S. K. Carey. 2007. “The Cold Regions Hydrological Model: A Platform for Basing Process Representation and Model Structure on Physical Evidence.” Hydrological Processes 21 (19): 2650–2667. https://doi.org/10.1002/hyp.6787.
- Rasmussen, R., B. Baker, J. Kochendorfer, T. Meyers, S. Landolt, A. P. Fischer, J. Black, J. M. Thériault, P. Kucera, and D. Gochis. 2012. “How Well Are We Measuring Snow: The NOAA/FAA/NCAR Winter Precipitation Test Bed.” Bulletin of the American Meteorological Society 93 (6): 811–829. https://doi.org/10.1175/BAMS-D-11-00052.1.
- Rasmussen, R., C. Liu, K. Ikeda, D. Gochis, D. Yates, F. Chen, M. Tewari, M. Barlage, J. Dudhia, and W. Yu. 2011. “High-Resolution Coupled Climate Runoff Simulations of Seasonal Snowfall Over Colorado: A Process Study of Current and Warmer Climate.” Journal of Climate 24 (12): 3015–3048. https://doi.org/10.1175/2010JCLI3985.1.
- Rasouli, A. A., K. K. W. Cheung, K. Mohammadzadeh Alajujeh, and F. Ji. 2022. “On the Detection of Snow Cover Changes Over the Australian Snowy Mountains Using a Dynamic OBIA Approach.” Atmosphere 13 (5): 826. https://doi.org/10.3390/atmos13050826.
- Rassam, D. W., L. Peeters, T. Pickett, I. Jolly, and L. Holz. 2013. “Accounting for Surface–Groundwater Interactions and Their Uncertainty in River and Groundwater Models: A Case Study in the Namoi River, Australia.” Environmental Modelling & Software 50:108–119. https://doi.org/10.1016/j.envsoft.2013.09.004.
- Rawat, S. S., S. Lahari, and A. K. Gosain. 2018. “Integrated Water Resource Assessment of Irrigation System of Haryana.” Agricultural Sciences 9 (04): 489. https://doi.org/10.4236/as.2018.94034.
- Razavi, S., A. Elshorbagy, H. Wheater, and D. Sauchyn. 2015. “Toward Understanding Nonstationarity in Climate and Hydrology Through Tree Ring Proxy Records.” Water Resources Research 51 (3): 1813–1830. https://doi.org/10.1002/2014WR015696.
- Razavi, S., D. M. Hannah, A. Elshorbagy, S. Kumar, L. Marshall, D. P. Solomatine, A. Dezfuli, M. Sadegh, and J. Famiglietti. 2022. “Coevolution of Machine Learning and Process-Based Modelling to Revolutionize Earth and Environmental Sciences: A Perspective.” Hydrological Processes 36 (6): e14596. https://doi.org/10.1002/hyp.14596.
- Razavi, S., A. Jakeman, A. Saltelli, C. Prieur, B. Iooss, E. Borgonovo, E. Plischke, et al. 2021. “The Future of Sensitivity Analysis: An Essential Discipline for Systems Modeling and Policy Support.” Environmental Modelling & Software 137:104954. https://doi.org/10.1016/j.envsoft.2020.104954.
- Reinfelds, I., E. Swanson, T. Cohen, J. Larsen, and A. Nolan. 2014. “Hydrospatial Assessment of Streamflow Yields and Effects of Climate Change: Snowy Mountains, Australia.” Journal of Hydrology 512:206–220. https://doi.org/10.1016/j.jhydrol.2014.02.038.
- Ruddell, A. R., W. F. Budd, I. N. Smith, P. L. Keage, and R. N. Jones. 1990. The South-East Australian Alpine Climate Study. Parkville, Victoria: Department of Meteorology, University of Melbourne.
- Saft, M., A. W. Western, L. Zhang, M. C. Peel, and N. J. Potter. 2015. “The Influence of Multiyear Drought on the Annual Rainfall-Runoff Relationship: An Australian Perspective.” Water Resources Research 51 (4): 2444–2463. https://doi.org/10.1002/2014WR015348.
- Saltelli, A., G. Bammer, I. Bruno, E. Charters, M. Di Fiore, E. Didier, W. Nelson Espeland, et al. 2020. “Five Ways to Ensure That Models Serve Society: A Manifesto.” Nature 582 (7813): 482–484. https://doi.org/10.1038/d41586-020-01812-9.
- Sánchez-Bayo, F., and K. Green. 2013. “Australian Snowpack Disappearing Under the Influence of Global Warming and Solar Activity.” Arctic, Antarctic, and Alpine Research 45 (1): 107–118. https://doi.org/10.1657/1938-4246-45.1.107.
- Sanecki, G. M., K. Green, H. Wood, and D. Lindenmayer. 2006. “The Characteristics and Classification of Australian Snow Cover: An Ecological Perspective.” Arctic, Antarctic, and Alpine Research 38 (3): 429–435. https://doi.org/10.1657/1523-0430(2006)38[429:TCACOA]2.0.CO;2.
- Sarmadi, F., Y. Huang, G. Thompson, S. T. Siems, and M. J. Manton. 2019. “Simulations of Orographic Precipitation in the Snowy Mountains of Southeastern Australia.” Atmospheric Research 219:183–199. https://doi.org/10.1016/j.atmosres.2019.01.002.
- Scherrer, P., and C. M. Pickering. 2005. “Recovery of Alpine Vegetation from Grazing and Drought: Data from Long-Term Photoquadrats in Kosciuszko National Park, Australia.” Arctic, Antarctic, and Alpine Research 37 (4): 574–584. https://doi.org/10.1657/1523-0430(2005)037[0574:ROAVFG]2.0.CO;2.
- Schmid, M., and J. Read. 2022. “Heat Budget of Lakes.” In Encyclopedia of Inland Waters, edited by T. Mehner and K. Tockner, 467–473. 2nd edn. Oxford:Elsevier. https://doi.org/10.1016/B978-0-12-819166-8.00011-6.
- Schreider, S. Y., 1996. Large Scale Modelling of Hydrologic Response for Climate Impact Assessment and Flood Forecasting ( PhD Thesis). The Australian National University, Canberra.
- Schreider, S. Y., and A. J. Jakeman, 2000. Restoration of the Island Bend Streamflow Regime for the Period Prior to the Construction of the Snowy Mountains Hydroelectric Scheme, in: Hydro 2000: Interactive Hydrology; Proceedings. Barton, A.C.T. Australia, 1030–1035. https://doi.org/10.3316/informit.584809731333751.
- Schreider, S.Yu., and A. J. Jakeman. 2001. “Streamflow Modelling on a Subdaily Time Step in the Upper Murray Basin.” Mathematical and Computer Modelling 33 (6–7): 659–668. https://doi.org/10.1016/S0895-7177(00)00270-3.
- Schreider, S.Yu., A. J. Jakeman, B. G. Dyer, and R. I. Francis. 1997. “A Combined Deterministic and Self-Adaptive Stochastic Algorithm for Streamflow Forecasting with Application to Catchments of the Upper Murray Basin, Australia.” Environmental Modelling & Software 12 (1): 93–104. https://doi.org/10.1016/S1364-8152(96)00050-3.
- Schreider, S.Yu., A. J. Jakeman, P. H. Whetton, and A. B. Pittock. 1997. “Estimation of Climate Impact on Water Availability and Extreme Events for Snow-Free and Snow-Affected Catchments of the Murray-Darling Basin.” Australian Journal of Water Resources 2 (1): 35–46. http://hdl.handle.net/102.100.100/224605?index=1.
- Schreider, S.Yu., P. H. Whetton, A. J. Jakeman, and A. B. Pittock. 1997. “Runoff Modelling for Snow-Affected Catchments in the Australian Alpine Region, Eastern Victoria.” Journal of Hydrology 200 (1–4): 1–23. https://doi.org/10.1016/S0022-1694(97)00006-1.
- Schreider, S. Y., P. H. Whetton, A. J. Jakeman, A. B. Pittock, and J. Li, 1996. Runoff Modelling for Snow-Affected Catchments in the Australian Alpine Region, in: Working Paper. Australian National University, Centre for Resource and Environmental Studies, Canberra, 26.
- Schreider, S.Yu., P. C. Young, and A. J. Jakeman. 2001. “An Application of the Kalman Filtering Technique for Streamflow Forecasting in the Upper Murray Basin.” Mathematical and Computer Modelling 33 (6–7): 733–743. https://doi.org/10.1016/S0895-7177(00)00276-4.
- Schwartz, A. J., 2021. Influence of Bushfire on Australian Snowpack Hydrometeorology within Snow Gum Forests ( PhD).
- Schwartz, A., H. McGowan, and N. Callow. 2021a. “Influence of Bushfire on Accumulation and Ablation of a Marginal Montane Snowpack in Snow Gum Forests.” Journal of Hydrology 603:126795. https://doi.org/10.1016/j.jhydrol.2021.126795.
- Schwartz, A., H. McGowan, and N. Callow. 2021b. “Snowpack Thermal Patterns in Pre- and Post-Bushfire Snow Gum Forests.” Journal of Hydrology 602:126789. https://doi.org/10.1016/j.jhydrol.2021.126789.
- Schwartz, A. J., H. McGowan, and N. Callow. 2020. “Impact of Fire on Montane Snowpack Energy Balance in Snow Gum Forest Stands.” Agricultural and Forest Meteorology 294:108164. https://doi.org/10.1016/j.agrformet.2020.108164.
- Schwartz, A. J., H. A. McGowan, A. Theobald, and N. Callow. 2020. “Quantifying the Impact of Synoptic Weather Types and Patterns on Energy Fluxes of a Marginal Snowpack.” The Cryosphere 14 (8): 2755–2774. https://doi.org/10.5194/tc-14-2755-2020.
- Searby, R. 2008. “Red Dog, Horses and Bogong Boths: The Memorialisation of Animals in Australia.” Public History Review 15:117–134. https://doi.org/10.5130/phrj.v15i0.728.
- Sheikholeslami, R., F. Yassin, K.-E. Lindenschmidt, and S. Razavi. 2017. “Improved Understanding of River Ice Processes Using Global Sensitivity Analysis Approaches.” Journal of Hydrologic Engineering 22 (11): 04017048. https://doi.org/10.1061/(ASCE)HE.1943-5584.0001574.
- Shin, M.-J., J. H. A. Guillaume, B. F. W. Croke, and A. J. Jakeman. 2013. “Addressing ten Questions About Conceptual Rainfall–Runoff Models with Global Sensitivity Analyses in R.” Journal of Hydrology 503:135–152. https://doi.org/10.1016/j.jhydrol.2013.08.047.
- Silberstein, R. P. 2006. “Hydrological Models Are so Good, Do We Still Need Data? Terrestrial and Fluvial Systems, Integrative Modelling of Climatic.” Environmental Modelling & Software 21 (9): 1340–1352. https://doi.org/10.1016/j.envsoft.2005.04.019.
- Simpkins, G. 2018. “Snow-related water woes.” Nature Climate Change 8 (11): 945–945. https://doi.org/10.1038/s41558-018-0330-7.
- Sitterson, J., C. Knightes, R. Parmar, K. Wolfe, B. Avant, and M. Muche. 2018. “An Overview of Rainfall-Runoff Model Types.” In Proceedings of the 9th International Congress on Environmental Modelling and Software, Fort Collins, Colorado, USA. Switzerland: International Environmental Modelling and Software Society. https://scholarsarchive.byu.edu/iemssconference/2018/Stream-C/41/.
- Slatyer, R. O., P. M. Cochrance, and R. W. Galloway. 1984. “Duration and Extent of Snow Cover in the Snowy Mountains: And a Comparison with Switzerland.” Search (Sydney, NSW) 15:327–331. https://doi.org/10.3316/ielapa.850404098.
- Snowy Hydro Limited, 2020. Snowy Live Data [WWW Document]. Snowy Hydro. Accessed January 30, 2023. https://www.snowyhydro.com.au/generation/live-data/.
- Sorooshian, S., K. Hsu, E. Coppola, B. Tomassetti, M. Verdecchia, and G. Visconti. 2008. Hydrological Modelling and the Water Cycle: Coupling the Atmospheric and Hydrological Models. Berlin, Heidelberg: Springer Science & Business Media. https://doi.org/10.1007/978-3-540-77843-1.
- Stephenson, B., B. David, J. Fresløv, L. J. Arnold, J.-J. Delannoy, F. Petchey, C. Urwin, et al. 2020. “2000 Year-Old Bogong Moth (Agrotis Infusa) Aboriginal Food Remains, Australia.” Scientific Reports 10 (1): 22151. https://doi.org/10.1038/s41598-020-79307-w.
- Stewart, I. T. 2009. “Changes in Snowpack and Snowmelt Runoff for Key Mountain Regions.” Hydrological Processes 23 (1): 78–94. https://doi.org/10.1002/hyp.7128.
- Sturm, M., J. Holmgren, and G. E. Liston. 1995. “A Seasonal Snow Cover Classification System for Local to Global Applications.” Journal of Climate 8 (5): 1261–1283. https://doi.org/10.1175/1520-0442(1995)008<1261:ASSCCS>2.0.CO;2.
- Tan, K. S., F. H. S. Chiew, R. B. Grayson, P. J. Scanlon, and L. Siriwardena. 2005. “Calibration of a Daily Rainfall-Runoff Model to Estimate High Daily Flows.” In MODSIM 2005 International Congress on Modelling and Simulation, Melbourne, Australia, 2960–2966. Melbourne, Australia: Modelling and Simulation Society of Australia and New Zealand.
- Theobald, A., H. McGowan, and J. Speirs. 2016. “Trends in Synoptic Circulation and Precipitation in the Snowy Mountains Region, Australia, in the Period 1958–2012.” Atmospheric Research 169:434–448. https://doi.org/10.1016/j.atmosres.2015.05.007.
- Trim, A., G. M. Podger, D. Dutta, and J. M. Puertas, 2023. Modelling the Murray–Darling Basin Southern Connected System. Presented at the MODSIM2023, 25th International Congress on Modelling and Simulation, Modelling and Simulation Society of Australia and New Zealand, Darwin, NT. https://doi.org/10.36334/modsim.2023.trim.
- Turnipseed, A. A., P. D. Blanken, D. E. Anderson, and R. K. Monson. 2002. “Energy Budget Above a High-Elevation Subalpine Forest in Complex Topography.” Agricultural and Forest Meteorology 110 (3): 177–201. https://doi.org/10.1016/S0168-1923(01)00290-8.
- Vaze, J., D. A. Post, F. H. S. Chiew, J.-M. Perraud, N. R. Viney, and J. Teng. 2010. “Climate Non-Stationarity – Validity of Calibrated Rainfall–Runoff Models for Use in Climate Change Studies.” Journal of Hydrology 394 (3–4): 447–457. https://doi.org/10.1016/j.jhydrol.2010.09.018.
- Viviroli, D., and R. Weingartner. 2004. “The Hydrological Significance of Mountains: From Regional to Global Scale.” Hydrology and Earth System Sciences 8 (6): 1017–1030. https://doi.org/10.5194/hess-8-1017-2004.
- Wallace, J., C. Macfarlane, D. McJannet, T. Ellis, A. Grigg, and A. van Dijk. 2013. “Evaluation of Forest Interception Estimation in the Continental Scale Australian Water Resources Assessment – Landscape (AWRA-L) Model.” Journal of Hydrology 499:210–223. https://doi.org/10.1016/j.jhydrol.2013.06.036.
- Wang, Q. J., T. C. Pagano, S. L. Zhou, H. A. P. Hapuarachchi, L. Zhang, and D. E. Robertson. 2011. “Monthly versus Daily Water Balance Models in Simulating Monthly Runoff.” Journal of Hydrology 404 (3–4): 166–175. https://doi.org/10.1016/j.jhydrol.2011.04.027.
- Wang, Z., X. Zeng, and M. Decker. 2010. “Improving Snow Processes in the Noah Land Model.” Journal of Geophysical Research: Atmospheres 115 (D20): 115. https://doi.org/10.1029/2009JD013761.
- Welsh, W. D., J. Vaze, D. Dutta, D. Rassam, J. M. Rahman, I. D. Jolly, P. Wallbrink, et al. 2013. “An integrated modelling framework for regulated river systems.” Environmental Modelling & Software, Thematic Issue on the Future of Integrated Modeling Science and Technology 39:81–102. https://doi.org/10.1016/j.envsoft.2012.02.022.
- Wheater, H. S., A. J. Jakeman, and K. J. Beven. 1993. “Progress and Directions in Rainfall-Runoff Modelling.” In Modelling Change in Environmental Systems, edited by A.J. Jakeman, M.B. Beck, and M.J. McAleer, 101–132. Chichester: Wiley.
- Whetton, P. H., M. R. Haylock, and R. Galloway. 1996. “Climate Change and Snow-Cover Duration in the Australian Alps.” Climatic Change 32 (4): 447–479. https://doi.org/10.1007/BF00140356.
- Wilson, B. R., M. Tulau, L. Kuginis, S. McInnes-Clarke, S. Grover, H. Milford, and B. R. Jenkins. 2022. “Distribution, Nature and Threats to Soils of the Australian Alps: A Review.” Austral Ecology 47 (2): 166–188. https://doi.org/10.1111/aec.13115.
- Worboys, G. L., R. B. Good, and A. Spate. 2011. Caring for Our Australian Alps Catchments: A Climate Change Action Strategy for the Australian Alps to Conserve the Natural Condition of the Catchments and to Help Minimise Threats to High Quality Water Yields. Canberra: Australian Alps Liaison Committee; Department of Climate Change and Energy Efficiency.
- Xu, X., J. Li, and B. A. Tolson. 2014. “Progress in Integrating Remote Sensing Data and Hydrologic Modeling.” Progress in Physical Geography: Earth and Environment 38 (4): 464–498. https://doi.org/10.1177/0309133314536583.
- Xu, C.-Y., J. Seibert, and S. Halldin. 1996. “Regional Water Balance Modelling in the NOPEX Area: Development and Application of Monthly Water Balance Models.” Journal of Hydrology 180 (1–4): 211–236. https://doi.org/10.1016/0022-1694(95)02888-9.
- Yaclng, A., D. Dutta, J. Vaze, S. Kim, and G. Podger. 2017. “An Integrated Modelling Framework for Building a Daily River System Model for the Murray–Darling Basin, Australia.” International Journal of River Basin Management 15 (3): 373–384. https://doi.org/10.1080/15715124.2017.1315814.
- Yang, D., D. Kane, Z. Zhang, D. Legates, and B. Goodison. 2005. “Bias Corrections of Long-Term (1973–2004) Daily Precipitation Data Over the Northern Regions.” Geophysical Research Letters 32 (19): 32. https://doi.org/10.1029/2005GL024057.
- Yang, Z.-L., G.-Y. Niu, K. E. Mitchell, F. Chen, M. B. Ek, M. Barlage, L. Longuevergne, et al. 2011. “The Community Noah Land Surface Model with Multiparameterization Options (Noah-MP): 2. Evaluation Over Global River Basins.” Journal of Geophysical Research: Atmospheres 116 (D12). https://doi.org/10.1029/2010JD015140.
- Yang, X., S. Tian, W. Feng, J. Ran, W. You, Z. Jiang, and X. Gong. 2020. “Spatio-Temporal Evaluation of Water Storage Trends from Hydrological Models Over Australia Using GRACE Mascon Solutions.” Remote Sensing 12 (21): 3578. https://doi.org/10.3390/rs12213578.
- Zafari, N., A. Sharma, D. Navaratna, V. M. Jayasooriya, C. McTaggart, and S. Muthukumaran. 2022. “A Comparative Evaluation of Conceptual Rainfall–Runoff Models for a Catchment in Victoria Australia Using eWater Source.” Water 14 (16): 2523. https://doi.org/10.3390/w14162523.
- Zhao, Q., X. Cai, and Y. Li. 2019. “Determining Inflow Forecast Horizon for Reservoir Operation.” Water Resources Research 55 (5): 4066–4081. https://doi.org/10.1029/2019WR025226.