Abstract
We discuss the dynamic impact of industrial cluster on regional innovation capacity from the view of life cycle. To identify an industrial cluster, we propose the location quotient composite indexes with technological innovation as the major consideration. Meanwhile, the entire life cycle evolution of industrial clusters is divided into emerging, growth, maturity and decline stages with the graphical index theory and the agglomeration degree measurement. Referring to Cobb-Douglas production function model and conduction process of ‘industrial cluster, innovation network, regional innovation’, we develop a mathematical model to measure the dynamic impact. The model is quantitatively verified using the general equipment manufacturing industrial cluster of Yangtze River Delta area as empirical study and is deemed to have been validated.
1. Introduction and literature review
The interaction between industrial cluster development and regional economic growth can be manifested as follows: industrial cluster development will impel economic growth and urge the formation of a regional innovation system. While the participants of the cluster establish closer relationships, their innovation and improved productivity will promote the local economy. The prosperous regional economy will bring huge profits, which will further attract external enterprises to gather around the cluster. This interaction is well known due to much research (Audretsch, Citation1998; Costa & Iezzi, Citation2004; Gordon & McCann, Citation2005; Varga, Citation2006), and we take this interaction as a basis to discuss the regional innovation impact from the view of cluster life cycle.
Industrial clusters emerged in Northern America and Western Europe in the 1970s when small- and medium-sized enterprises in some regions gathered for prompt development. The clusters in the Third Italy and Silicon Valley have become some of the world-class typical industrial clusters today. This economic phenomenon arouses the controversy about the innovation capacity between large- and medium-sized enterprise, and no convincing result has come from this controversy (Durlauf & Blume, Citation2012). Meanwhile, the argument has promoted academic research on the impact of industrial clusters on regional innovation capacity.
An increasing number of studies have found that there is no inevitable relationship between the innovation process and factors such as the concentration of intelligence, venture capital, infrastructure, and information services (Castells & Hall, Citation1994; Ma, Citation2013; Ye, Citation2009). Innovation is a process in which the behavioural agents develop technology with each other through mutual and synergistic collaboration. Thereby, the innovation activity of industrial cluster behavioural agents is an essential factor in the promotion of regional innovation capacity. In general, the behavioural agents participating in innovation activities can be divided into three categories (Asheim & Isaksen, Citation2002; Isaksen, Citation1997; Porter, Citation1998). The first type is composed of the leading enterprise of the industrial cluster and the supportive industries closely related in the industrial chain. The second type is innovative institutions directing the exchange of innovative information, innovative transformation, and innovative personnel training, such as technology research and development institutions, higher education institutions, technology and skills training institutions. This category has continually improved during the development of industrial clusters and has made significant contributions to the promotion and development of regional innovation capacity. Finally, chambers of commerce and other organisations in the cluster compose the third category. Within the industrial clusters, improvement of innovation capability relies on the sustainable supply and input of technology development resources from both internal and external. For instance, the improvement of innovation capacity relies on the continuous supply of internal and external technical resources to the industrial cluster, namely talents and academic research, enterprise research and development, and other technological innovation information transfer networks (Broersma & Oosterhaven, Citation2009; Freeman, Citation1991; Galarraga, Paluzie, Pons, & Fabregat, Citation2008). In conclusion, scientific research personnel resource input and capital investment are the key variables of regional innovation capacity that influence the model.
Do the characters of industrial clusters have a direct effect on regional innovative capacity? Mothe and Paquet (Citation1998) take 60 industrial clusters in knowledge-based regions, large cities, technopolises and marginal areas as empirical objects and summarise three key characters of industrial cluster. Firstly, the strong relationship between the supportive technology of enterprises and the infrastructure of industrial clusters benefits the innovation process and the industrial cluster’s improvement. Secondly, the geographical aggregation of enterprise, education and research institutions, and financial and other commercial institutions in the industrial cluster enhance the efficiency of the innovation process. Third, the larger the scale of the industrial displays, the higher the self-satisfaction standard reaches, which leads to less input needed from sources external, and the smaller the industrial cluster would benefit external parts. The importance of knowledge spillovers has been debated for a long time in the research of the spatial agglomeration of economic activities, especially regarding whether the knowledge spillover would cross geographic boundaries. It is generally recognised that it is much easier for major breakthroughs and highly uncertain knowledge to move across buildings or streets in the same region than across larger stretches of land and oceans. Studies reveal the significance of tacit knowledge in the innovation process and that the marginal transfer cost rises rapidly with distance. Hence, spatial geographical proximity and face-to-face interaction is vitally important (Arvanitis, Lokshin, Mohnen, & Wörter, Citation2013; Boix, Hervás, & De Miguel, Citation2014; Boutellier, Gassmann, & Zedtwitz, Citation2008; Carlino, Chatterjee, & Hunt, Citation2007; Cooke, Citation2002a; Cooke, Citation2002b; Fritsch & Graf, Citation2010; Gersbach & Schmutzler, Citation1999; Lawson & Lorenz, Citation1999; Lissoni, Citation2001; Storper & Venables, Citation2004; Wallsten, Citation2001). These studies show that the agglomeration degree of industrial clusters is an important variable influences regional innovation capacity.
The interaction relationship between industrial clusters and regional innovation capacity is an important driving force of permanent regional economic development (Brenner, Cantner, Fornahl, Fromhold, & Werker, Citation2011; Breschi & Lissoni, Citation2009; Hassink & Klaerding, Citation2012). Therefore, it is important to analyse the influence paths of industrial clusters and regional innovation capacity. After defining the three key factors of regional innovation capacity, we provide the research issues on the influence effect. Wang and Hou (Citation2007) distinguish the concentration ratio of industrial clusters and observe the technological development expenditure per capita. They find that highly concentrated industry has larger research and development expenditures. According to Hu and Jiao (Citation2008), who take the typical industry such as the oil and gas resources industry as empirical objects and analyse the relationship between industrial clusters and regional innovation capacity, industries with regional concentration production capacity also show regional concentration in their own innovation activities. Although the existing studies have focused on the relationship between industrial clusters and regional innovation capacity (Tavassoli & Tsagdis, Citation2014), these empirical studies are lack of specific industrial data and sufficiently dynamic description of the influence effect.
Due to the stages and periodicities in the evolution cycle of industrial clusters, Brusco (Citation1990) divides the stages into two segments including spontaneous generation and external intervention. Tichy (Citation1998) proposes a four-stage industrial evolution method (emerging, growth, maturity and decline) based on Vernon’s life cycle theory. The four-stage life cycle of industrial cluster evolution is now widely accepted and applied. However, the qualitative description research of industrial cluster evolution is still a difficult problem, which drives the promotion of quantitative judgment methods (Carbonara, Giannoccaro, & Pontrandolfo, Citation2002; Menzel & Fornahl, Citation2010; Martin & Sunley, Citation2011). These abovementioned dividing methods can depict a sketch of the evolution process but are insufficient for further refining the demarcation point of each stage. Thus it is impossible to capture the degree of the influence effect during the various stages. As reported by Fritsch and Slavtchev (Citation2010) who estimate the Marshall external economic effect between industry specialisation and innovation input, a U curve is found in the impact of industry specialisation, which acts on regional innovation efficiency. Broekel and Boschma (Citation2012) argue that proximity may be a crucial driver for agents to connect and exchange knowledge, but too much proximity between agents on any of the dimensions might harm their innovative performance at the same time. In addition, they note that research expenditures, common knowledge innovation and regional specialisation also affect regional innovation efficiency.
The majority of the common research mentioned above focus on either innovation activities or spatial adjacency. However, the relationship between the two factors is not fully discussed with empirical study and results.
The conclusions provided by many researchers go to two opposite directions that one is weak correlation and the other is negative correlation (Gilbert, McDougall, & Audretsch, Citation2008; Molina, Boix, & Oliver, Citation2012; Tichy, Citation1998; Watts, Wood, & Wardle, Citation2003). We will explain why this happens from the following three aspects. Firstly, the lack of recognition of industry cluster makes no clear judgment whether research object has properties of industrial cluster. Secondly, from the theoretical point of view, without the recognition of industrial cluster life cycle stages, the effects of industrial clusters on regional innovation capacity in different life cycle stages could not be distinguished. Thirdly, the analysis methods used do not have general applicability.
During the reformation of China’s economic market system, economy is closely bound up to the development of industrial clusters. Thus industrial clusters have played an increasingly important role in the regional economic development. Taking advantage of natural resources, labour and government policy, some representative industrial clusters have grown rapidly, such as the textile industry in Zhejiang, semiconductor fabrication in Dongguan, motorcycle manufacturing in Chongqing, and the steel industry in Tangshan. Among these regions, the Yangtze River Delta area is best recognised for its scale and development speed. The industrial cluster in the Yangtze River Delta area forms in the 1990s following the development of Shanghai. Capital, advanced technologies, labour and other elemental factors gathered and turned into the early industrial cluster during the emerging stage. The Wenzhou and Southern Jiangsu patterns accelerate the development in the Yangtze River Delta area and serve as a boon for the national economy. In addition, the life cycle evolution of the industrial cluster in the Yangtze River Delta area has made huge impacts on other industrial clusters, which also makes research on innovation capacity of significance.
The aim of this article is to construct the impact model of industrial cluster on regional innovation capacity in different stages through quantitative identification of industrial clusters and classification of life cycle stages. On this basis, we select general equipment industry in Yangtze River Delta to do empirical analysis. We hope to enrich the theory about effects of industrial cluster evolution and provide important reference to promoting sustainable development of industrial cluster and regional economic through this research. Therefore, we will analyse and improve these defects and the remainder of this article is as follows. In section 2, the impact mechanism of the industrial cluster on regional innovation capacity is displayed. The impact of industrial clusters on regional innovation capacity in different stages of life cycle will be evaluated from innovation network perspective. Section 3 focuses on the identification of industrial clusters. Unlike most of the present research, which has paid less attention to the effect of technological innovation, we utilise location quotient composite indexes to identify the industrial cluster. Together with other traditional factors, we consider the technological innovation in location quotient composite indexes to be a major factor in identifying industrial clusters. Section 4 provides the partition results of the industrial cluster life cycle. To avoid the limitation of data availability and method feasibility, we combine a graphical index methodology and an agglomeration degree measurement methodology to observe how clusters change during their entire life cycles. After the previous preparation, we calculate the impact of the industrial cluster on regional innovation capacity in various stages of its life cycle in Section 5. Industrial clusters have various impacts on regional innovation capacity during different stages of their life cycles due to their development level and the innovation network condition. Furthermore, existing work focuses mainly on case analyses of industrial clusters from macro view rather than considering the internal mechanisms of clustering. To supplement mathematical measurement on the micro mechanisms of industrial clusters, we present a model to describe the impact of industry clusters on regional innovation capacity in various life cycle stages by referring to the Cobb-Douglas production function and the conduction process of ‘industrial cluster, innovation network, and regional innovation.’ Finally the conclusion section and further research implications is also presented.
2. Impact mechanism of various stages of the industrial cluster life cycle on regional innovation capacity
The impact mechanism of industrial clusters on regional innovation capacity can be described with the ‘industrial cluster, innovation network, regional innovation’ model (Balland, Vaan, & Boschma, Citation2013). From the structural and functional perspective, a change of the innovation network structure will alter the way innovators utilise their resources and the technology transfer mode. Consequently, it will affect the utilisation of innovation resources and propagation effect of the cluster and finally make an impact on the regional innovation capacity. The agglomeration degree and innovation structure of the industrial cluster vary at various stages of the cluster life cycle, which leads to various impacts on the regional innovation capacity (Fri, Pehrsson, & Søilen, Citation2013).
2.1 Emerging stage
At the beginning of this embryo period when there are few enterprises with slow progress, the level of the industrial cluster is low. Because the highly divided production and socialisation networks are yet to be completed, the connections among the network are very weak. Due to an insufficient environment for innovation activities, innovation is not profitable and is not the main business of enterprise. With rare interactions between the members of the cluster, the structure of the innovation network is loose, and thus, no obvious advantages have been shown in the cluster’s core competency or technological innovation capacity. Enterprises tend to follow each other in the innovation scheme when there is a lack of communication and information sharing. Therefore, compared to other stages, the weak impact of the industrial cluster on the regional innovation capacity during this period will lead to fewer patents.
2.2 Growth stage
The industrial cluster starts to attract enterprises outside the cluster in this stage, and large quantities of supporting enterprises, agencies and service organisations start to join the cluster. The innovation network shows significant advantages in helping enterprises perform innovation activities (Chiu, Citation2009; Lu & Beamish, Citation2006; Palmatier, Dant, & Crewel, Citation2007; Sullivan & Weerawardena, Citation2006; Walter, Auer, & Ritter, Citation2006). The follower enterprises benefit from the cluster network and redevelop the technology, which pressures the leading enterprises and pushes them to start a new round of innovation to avoid obsolescence. Meanwhile, the information platforms and intermediary agencies function and provide the needed knowledge and services. As a result, the connections among the enterprises strengthen. In this stage, both the density and clustering coefficient of the net increase, and communication channels for exchanging information and knowledge in the cluster are formed. The confidential relationships among the enterprises enhance the stability of the network during communication and cooperation. In summary, the innovative enterprises improve technology development and the amount of imitative enterprises rises during this period. Together, these factors serve the most remarkable function and lead to a positive promotion of the industrial cluster network, and the number of patents increases rapidly.
2.3 Maturity stage
A higher industrial cluster level provides a better innovation environment for the enterprises. Collective learning is the main characteristic of this innovation environment (Caniëls & Romijn, Citation2005; Keeble & Wilkinson, Citation1999; Morosini, Citation2004). Driven by collective learning, each enterprise continuously carries out innovation activities, which leads to the emergence of an innovation network in the environment and completes the functions of the entire innovative cluster system. During this period, the innovation network of the cluster is maturing, and the internal structure shows adjustment and strength among the enterprises. The number of industrial chains increases and the cooperation among the enterprises goes from single strand to cross-unit, which forms the value chain of the network. As the cluster matures, the innovation network structure reaches its optimum state of equilibrium. Simultaneously, the development speed of enterprise slows, and the circulation of knowledge, information and resource is fairly complete within the cluster. Universities and research institutes become the technological innovation centres. Following this, the enterprises in the cluster share innovation results through a knowledge spillover effect, and the new innovation spread is carried out by the cluster innovation network. Technological innovation still follows the path in its growth stage, that is, most innovations occur gradually, and the patents created reach the limit. With this process, the mutual learning capacity and promotion momentum are insufficient and cause technology duplication and a lack of innovative vigour. As a result, the coverage area of the cluster innovation network suspends its expansion and retracts gradually, which leads to a recession of the impact of the industrial cluster on the regional innovation capacity.
2.4 Decline stage
This is a changing stage for the industrial cluster where its structure borders start to break and reconnect, which leads to a differentiation of the innovation network. As the entire industry declines, profit gliding is inevitable. There will be two paths after the full development of the innovation network: the decaying of the disadvantaged cluster, and the replacement of the original clusters by advantaged clusters who followed the emerging market trend and became the key members of a new cluster innovation network, which ultimately results in an upgrade substitution. During the decay process of the existing structure, certain subjects retreat from the cluster and new enterprises enter. The relationships among the members will go through ‘breakage-reconnection-breakage’ stages, and the process will be repeated until the new structure is satisfied. After reconstruction, the cluster structure is loose again and sensitive to external conditions. On the other hand, uncompetitive elements will be eliminated by the market. Exhaustion of the innovation environment and endogenous forces indicate the collapse of innovation diffusion and activities, which also weaken the promotion of the regional innovation capacity. The decreased number of patents is an important characteristic of this stage.
3. Identification of industrial clusters
3.1 Identification model for industrial clusters
From the essential research paradigm, the first question of industrial cluster research is how to identify clusters, via which the empirical object can be shown to be consistent with the characteristics of industrial clusters. Industrial clusters are intermediate organisations located in a specific geographical region that are officially or unofficially formed by enterprises with shared goals and unified rules. Through competition and cooperation, members in the cluster complete the human resource distribution and establish economic and technological relationships based on input–output analyses. Both the vertical relationship of the industrial value chain in the cluster layer and the complement relationship among enterprises and supportive service agencies drive regional economic development and engender advantages of their own. Thus, the identification model we propose considers traditional elements and emphasises technical innovation. Specifically, we take location as the basis and fully regard the space concentration of the industrial cluster as embodying the degree of specialisation for regional industry. Furthermore, we evaluate location quotient composite indexes by applying the location quotient and the characters of the industrial cluster under the present economic development environment including the location quotient of industrial production (LQ1), location quotient of the quantity of enterprise (LQ2), location quotient of the concentration of employees (LQ3) and location quotient of the technical innovative capacity (LQ4). The location quotient of the technical innovative capacity index is also called comparative labour productivity or relative national income and represents the technological level, the new orientation of the regional economy and the new economic growth point. The functions of the four location quotients are displayed in Table .
Table 1. Functions of location quotient composite index.
The weight of the four indexes mentioned above has been extensively studied in the existing literature and is generally considered to vary with small differences (Chen & Yan, Citation2012; Liu, Chen, & Wang, Citation2007). To simplify the quantification of the cluster identification, we assume the four indexes have equal significance, that is(1)
where .
3.2 Identification of the industrial cluster in the Yangtze River Delta area
Following the literature on industrial clusters and considering the relative research material, we chose the Yangtze River Delta area as the empirical object for this rticle. In the economics field, the Yangtze River Delta area is generally taken to be the coastal regions containing the Shanghai, Jiangsu and Zhejiang provinces, which is also the leading national economic region of China both in scale and speed. There are three primary reasons to choose the Yangtze River Delta area: the development history, development pattern and concentration effect of the regional industrial cluster.
3.2.1 Long development history of the industrial cluster
Benefitting from the geographic advantages of the coastal region, Shanghai became the most important city for China’s international communications in culture and economy long ago. Thus, the international and national private capital and the best professional talents from Zhejiang and Jiangsu flow into Shanghai continuously, which has enhanced the development of Shanghai. Shanghai is the most economically developed city of China. In the 1990s, following the development of Shanghai, capital, technology and labour elements gathered in the Yangtze River Delta area and promoted the appearance of the earlier industrial cluster region.
3.2.2 Advanced development pattern
Since the 1980s, the ‘Wenzhou pattern’ and the ‘Suzhou pattern’ appeared in the Yangtze River Delta area, which led to township and rural enterprise development. These two patterns led to improvement and new trails in China’s industrialisation and marketisation revolution, which greatly promoted the development of the regional economy and led to the emergence of an industrial cluster of some productive departments. Taking advantage of the advanced patterns, the industrial cluster of the Yangtze River Delta area developed vigorously. This has also been particularly important for the continuous development of the regional economy.
3.2.3. Significant concentration effect
The area of the Yangtze River Delta area forms only 2% of the country’s territory but produces a quarter of the gross domestic product. An important factor behind this is the economic form of the industrial cluster. The speed of the industrial cluster development is accelerating in the Yangtze River Delta area since the reform and opening-up policy. The Yangtze River Delta area agglomeration economic circle together with Southern China and the Circum-Bohai Sea Economic Zone constitute the primary supporting points of China’s industrial cluster and have become an international industrial cluster. The Yangtze River Delta area is an important manufacturing base in China, and it mainly focuses on equipment manufacturing. The data collected through our research suggests that the Yangtze River Delta area has significant advantages in the quantity of enterprises, the number of employees, production value and profitability over its competitors. Additionally, its indicators are also much higher than those of other manufacturing industries. Along with the development of this industry, the innovation of enterprises is enhanced and has become the most active industry in technical innovation. Take the Zhejiang province as an example; the technical R&D and the purchase of IPs for this industry accounted for 54.1% of the gross province expenditure and 48.9% of the gross province expense in 2010. The increment reached 29.9%, which is 3.9% higher than the province average.
Considering the research situations from the data availability, significance, and applicability, we investigated the time series of the general equipment industry in the Yangtze River Delta area for 10 years. The raw time series comes from the statistical yearbooks of the Jiangsu, Zhejiang and Shanghai provinces, the China industrial economic statistical yearbook, and the China statistical yearbook on science and technology. The calculation results of the industrial cluster identification with the location quotient composite index are shown in Table below.
Table 2. Industrial cluster identification results for general equipment industry in the Yangtze River Delta area.
Based on the fundamental judging principle of the location quotient, the results of Jiangsu, Zhejiang and Shanghai are all beyond 1.2, which indicates the existence of an industrial cluster. Thus, the empirical study is of value. We also calculated the location quotients of industrial production, enterprise quantity, employee density and technical innovation capacity. Based on our results, the location quotients of the technical innovation capacity in the three provinces are approximately 0.9 (below 1.0), which indicates space for improvement even though the industrial cluster of general equipment in the Yangtze River Delta area has a positive impact on the regional innovation capacity. To simplify the quantification process, we assumed the weight of each indicator to be 0.25, which should be different in practical production according to industry properties. Additionally, the value of the industrial output and number of practitioners in the Yangtze River Delta region’s general equipment industry is increasing gradually, but the LQ indicators of the three regions are decreasing gradually. This situation illustrates that the influence of the traditional input, including human resources and capital, is declining during the industrial cluster’s development.
4. Partition of the industrial cluster life cycle
4.1 Partition model of the industrial cluster life cycle
Depending on the identification results above, the general equipment industry in the Yangtze River Delta area is assumed to be a typical industrial cluster that displays periodic characteristic of its own. Thus, the next issue is the partitioning of the life cycle stages of the industrial cluster (Brenner, Citation2006; Duque, Rey, & Gomez, Citation2010; Feser & Bergman, Citation2000; Holmen & Jacobson, Citation2003; Kelton, Margaret, & Robert, Citation2007; Titze, Brachert, & Kubis, Citation2011). There are no unified methods to classify the stages of industrial cluster life cycles. There are also no specific definitions of each stage. Nevertheless, we can identify and classify the stages quantitatively and qualitatively with the characters of their outward manifestation and the main indicators of the industrial cluster. Below are the details of the general quantitative methodology.
4.1.1. Graphic index method
This method classifies the life cycle stages with the change of scale indicators relevant to the industrial cluster including the number of enterprises, employees, total industrial output value, total income, total profit, etc. The method is based on the fluctuation and trend of the scale indicators for industrial clusters from the emerging and growth stages to the maturity and decline stages, as shown in Figure (Gong, Jin, & Wang, Citation2008; Sun & Gong, Citation2010).
Figure 1. Periodical change of the scale indicators of industrial clusters.
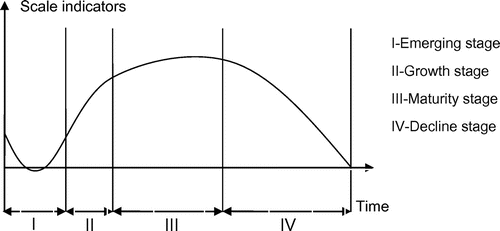
Part I of Figure above shows the emerging stage of industrial clusters during which the development of the cluster is slow and the promotion of the indicators may fluctuate. Along with the cluster’s rapid development in the growth stage, the indicators experience a fast increment in Part II of the figure. The increment of the cluster indicators slows down in Part III. As the industrial cluster enters the maturity stage, an optimisation will be reached. The scale of the cluster will tend to be steady with the enterprises entering and exiting the cluster forming equilibrium. After the cluster reaches the peak of the maturity stage, internal development problems will act as a centrifugal force, which makes the cluster less attractive to external enterprises and expels the internal enterprises. Finally, bankruptcy and emigration will appear at the end of this stage with the indicators decreasing gradually as shown in Part IV of the figure. By calculating and comparing the relevant scale indicators of the cluster, observing the change of the development process on the time dimension, and plotting the corresponding life cycle graph, we can gain clear understanding on the life cycle of the industrial cluster.
4.1.2. Agglomeration degree measurement method
By measuring the change of cluster agglomeration degree and observing its regular pattern, we can identify the various stages of the life cycle quantitatively. The intrinsic property of the industrial cluster can be considered to be the space aggregation of specific industry or business in the specific area. Although some industrial clusters may have cross-industry development, the nature of the cluster evolution is led by dominant industrial enterprises and organisations, and the most prominent character of the evolution is the continuously changing cluster agglomeration degree. As a result, we can depict the evolution of industrial cluster life cycle by following and capturing the changing process.
The commonality of the two methods introduced above is the identification of the stages of the cluster by quantitatively measuring the degree of development; the differences exist in the range of feasibility. For the agglomeration degree measurement method, the result closely relates to the structure of the cluster and the organisation structure of enterprises. If the cluster is dominated by monopoly enterprises, this method will exaggerate the results; on the other hand, it may underestimate the agglomeration degree when the majority of the enterprises are small-sized corporations. Although the graphical index method does not have this limitation, it is not comprehensive enough to reflect the relevant changes on the structure. We combined both of the methodologies in the empirical study to yield a comprehensive result.
4.2 Partition of the industrial cluster life cycle of the Yangtze River Delta area
We summarise the economy development history as below to find the starting point of the industrial cluster life cycle of the Yangtze River Delta area. At the beginning of China’s reforming and opening, the scale of the state-owned economy in the Yangtze River Delta area was large and represented a large proportion of the total economy. Due to the central government’s stringent policy and complicated input–output relationships, the reform work was difficult. During this period, the area was under an endogenous increase in development at a very slow speed. The development of Shanghai Pudong in 1993 marks the new era of the Yangtze River Delta area from where rapid development started and it soon became the leader of national economic growth. Great changes took place in Jiangsu and Zhejiang, with township enterprises leading property relations reform and the innovation of private enterprises in Zhejiang accelerating the development of this area. As a result, we conduct our empirical study based on the data from the general equipment industrial cluster of the Yangtze River Delta area with graphical index and agglomeration degree measurement methodologies stated in 4.1.
The raw data is derived from the 1994–2011 statistical yearbooks of Jiangsu, Zhejiang and Shanghai and includes the amount of enterprises, employees, total industrial output value, main business income and total profit (Liu & Chen, Citation2004). During the selection of indicators, the following two aspects should be considered.
The first are the amounts of enterprises and employees. From the evolution process of industrial cluster, it is obvious that the amounts of enterprises and employees have presented the scale of industrial clusters. During the evolution process from junior to senior, more and more enterprises are attracted into the cluster as the result of the enhancing gather ability. Meanwhile, the number of employees needed also grows with the number and scale of enterprise. Conversely, if the cluster develops to decline stage, the amount will reduce respectively. Considering the growth rate, the scale of industrial cluster and cardinality is small during the emerging stage which will make the growth rate larger In Emerging stage, the growth rates of the indicators will continue increase due to the accumulative effect of industrial cluster which attracts a large number of enterprises and talents gathering in the cluster until the maturity stage. These indicators will show steady growth trend when the cluster enters maturity stage. Thus, the two indicators are the main content that reflects industrial cluster’s scale effect.
The second are the total industrial output value, main business income and total profit. The three indicators mainly reflect the economic strength of industrial cluster during the life cycle. In emerging stage of the industrial cluster, because of the unformed competitive advantage, these indicators are much smaller. Along with the scale’s expansion, stronger competitive advantage and more enterprises joining, the total industrial output value will increase. It is the proof of development of the cluster. On the other side, these indicators will decline in the declining stage. Also from the aspect of growth rate, during the emerging stage, as the limitation of economic strength, the total industrial output value and main business income are much smaller while the net profit may be larger relatively. However, in the following stage, after the entire economic scale of the cluster showed up, total industrial output value and main business income will increase rapidly until they reach a stable level. Thus these three indicators are important to represent the economic effects of the industrial cluster.
We plotted the development process of the general equipment manufacturing industrial cluster in the Yangtze River Delta area as shown in Figure . Figure includes five small maps (a, b, c, d and e), which respectively correspond to the change of the five indicators which are the amount of enterprise, the amount of employees, total industrial output, main business income and total profit. The horizontal axis represents time and the vertical axis represents the value of corresponding indexes.
Figure 2. Development process of the general equipment manufacturing industrial cluster in the Yangtze River Delta area.
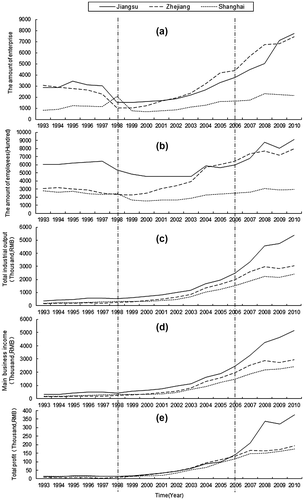
In Figure , we can see the similar tendencies during the process, but the situations of the general equipment industrial cluster’s specific indicators differ in various regions. First, there is similar tendency during the development process of the industrial cluster from the specific indicators of enterprise amount and professional employee amount. The trend fluctuates obviously in 1993–1998 and shows increasing tendency from 1999 to 2006. The high degree of the tendency appears at 2008, and then the surging slightly declines in the following years, especially in the Zhejiang and Shanghai provinces.
Second, the total output, main business and total profit of industry in these areas also demonstrate growth trends in three stages during the sample period. In the first stage, from 1993 to 1998, there is no obvious rising trend of the indicators but asmooth and steady growth. Over the period of 1999–2006, all the indicators increase steadily. The curves of Zhejiang and Shanghai reach their high point in 2008, but there is a gradual decline from this year on. Meanwhile, there is an upward trend in the numbers of Jiangsu.
Third, the development of the general equipment industrial cluster can be divided into three stages in general: the emerging stage from 1993 to 1998, during which the clusters in all three areas experienced slow development; the growth stage from 1999–2006, during which the cluster started rapid development; and in 2007–2010, Zhejiang and Shanghai entered the maturity stage but Jiangsu remained in the growth stage and the speed of the maturity clusters’ development slowed down. Therefore, the indicators first experience a decline period before the increase as demonstrated by the relevant characteristics of enterprise amount and employee amount. This occurred closely for the development characteristic of the industrial cluster in the Yangtze River Delta area. As a result of the industrial cluster’s distinct directivity in this area, the growth advantage was particularly susceptible to the cheap pool of labour power during the emerging stage. In contrast to the vast amount of factories and workers, the industrial output value was small during this period, and the majority of the industrial cluster consisted of less technical and labour-intensive small- and medium-sized enterprises.
Finally, there is no sign of showing the coming of the decline stage in the figure. Thus, the productivity advantage of the general equipment industrial cluster exists. However, the industrial cluster would confront severe pressures in the future because the increase of the productive input costs with the labour cost and rare land, other resources, and the price of productive factors to increase and may impel the productive input cost to increase. Furthermore, associated with the geography condition of the Yangtze River Delta area, the terrain, landform, weather conditions and other natural elements of these regions are similar in general. With similar geographic situations and close economic connections, the mutual emulation between native enterprises and foreign capital rush in, and the manufacturing industry concentrates in the area. This may also be due to the lack of authority and a systemic and normative governance regulatory framework that would lead to and promote the cooperation mechanism of the industry in the Yangtze River Delta area. In this case, there would be strong resistance by enterprises to compete for productive elements and privilege policies. Limited by these unsolved issues, the general equipment industrial cluster in the Yangtze River Delta area will inevitably enter the decline stage in the future.
To overcome the difficulties in data collection in the existing research of agglomeration degree measurement, we averaged the data by the number of people. The amount of enterprise, employees, and total industrial value per person, income per person and profit per person were used as indicators in a factor analysis. By measuring the agglomeration degree of the industrial cluster with a factor analysis on the Yangtze River Delta area general equipment manufacturing industry, we can test the partition results of the industrial cluster life cycle in Figure .
Comparing the industrial indicators of 23 provinces, four direct-controlled cities and five special municipalities from the 2010 Chinese statistical yearbook, with the regions of low key indicators being rejected, we run a correlation test and conduct a factor analysis with SPSS 16.0. The results are shown in Table below.
Table 3. Result of the KMO and Bartlett’s Test.
From the test result, the KMO index is 0.759, which is higher than the critical value and is thus suitable for a factor analysis. The factor loading matrix is shown in Table below.
Table 4. Factor loading matrix after orthogonal rotation.
Table suggests that two common factors should be selected: the first one has larger load on the total industrial value per person, income per person and profit per person. The second one has a larger load on the amount of enterprise and employees. The main characteristic indicators of the industrial cluster life cycle of the Yangtze River Delta area general equipment manufacturing industry has been well classified.
Proceeding with the calculation of the factor score coefficient matrix, we obtain the score of each factor. Considering the principle of factor score coefficient matrix and the original indicators, the agglomeration degree and the corresponding change rate of this cluster can be deduced as shown in Table .
Table 5. Factor score coefficient matrix.
The plot in Figure below shows the tendency of the cluster indicators of the general equipment manufacturing industry from 1993 to 2010. It is based on the agglomeration degree results of the three areas from Table . The development process indicated by the plot also further establishes the partition result in Figure .
Figure 3. Tendency of cluster indicators of General Equipment Manufacturing Industry of Yangtze River Delta area.
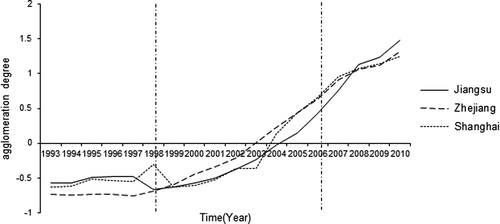
Table 6. Comprehensive index and change rate of the general equipment manufacturing industry of the Yangtze River Delta area in 1993–2010.
Based on the analysis of the quantitative indicators and agglomeration degree measurement of the general equipment manufacturing industrial cluster, we briefly analyse the reason of life cycle phenomenon considering the economic environment and the development of the equipment manufacturing industry in the Yangtze River Delta region. From the above plotting, it is obvious that the turning point of the industrial cluster life cycle appear in 1998 and 2008, during which the global financial crisis erupts and leads to a tough global economic environment. China is seriously affected by the economic recession of the EU and US because it is the largest export market in the world. Meanwhile, to recover the local economy and reduce the unemployment rate, many EU countries and the US start strict trade policies. For the manufacturing enterprises in the Yangtze River Delta area are mainly private and highly dependent on exports, the change of international economic environment highly influences the development level and scale of entire cluster. Take the machine tool industry in the Yangtze River Delta area as an example. The orders decreases from May 2008, the inventory increases, the profitability drops, and the growth of the output value slows. The change and influence of these indicators constitute the phenomenon of the industrial clusters’ life cycle.
5. Impact of the industrial cluster on regional innovation capacity in various stages of its life cycle
5.1 Modelling the industrial cluster’s impact on regional innovation capacity
Based on the partition result of the life cycle stages, we investigate how the industrial cluster influence regional innovation capacity during different life cycle stages. It is previously and commonly believed that technical input is the main driving force of innovation (Castells & Hall, Citation1994; Ma, Citation2013; Tsai & Wang, Citation2007). However, along with the deep research on the innovation process, the non-linear relationship between technical input and innovation has been found, and the input scale of R&D resources is proved not to be the only determining factor of innovation performance. In recent years, points of view from evolution theory and organisation learning theory have influenced research on innovation and have led people to realise that innovation is an evolutionary process operating on individuals and groups. On one hand, innovation is an individual activity in a particular field and technology. On the other, it is also a collective activity, and the interactive learning between various departments and enterprises plays an important role. The research shows that most innovation in regional economies comes from industrial clusters (Asheim, Smith, & Oughton, Citation2011; Karlsen, Citation2013).
Most of the key innovations in modern economies cannot be completed by a single enterprise because a very small portion of the requisite knowledge for innovation is found internally. Indeed, there is a diversity of innovation modes. Referring to the conduction process of ‘industrial cluster, innovation network, regional innovation’ in which the innovation network is the medium, we need to quantitatively measure each element mentioned in the process.
5.1.1 Measurement index of regional innovation capacity
Based on the research of regional innovation capacity indicators (Asheim et al., Citation2011; Bhattacharya & Bloch, Citation2004; European Commission, Citation2014) and considering the practical situation and data, we choose the number of patents applications and the new product sale income as the measurement index. During the selection of indicators, the following two aspects should be considered.
The first is the number of patents applications. Many of the empirical literatures use the number of patents applications as an important indicator of measuring technology innovation level for the following reasons (Yueh, Citation2009; van Zeebroeck, Stevnsborg, van Pottelsberghe de la Potterie, Guellec, & Archontopoulos, Citation2008). The patents applications exhibit the most valuable part of regional innovation activities and also carry the objective economic benefits to reflect the capacity of technological innovation of the cluster. As an important step of the enterprise innovation activities, patents applications imply potential subsequent technology application and development which also represent the market promotion value of the innovation in the future. We choose patents applications rather than patents obtained because there is usually a long time gap from application to approval which may affect the results of the model. The information of patents applications also contains most the patents obtained (Pan & Liu, Citation2008).
The second is new product sale income. Research shows that the process of technology innovation includes not only innovation achievements, but also market application of innovation techniques (Norberg, Citation1999). The concept of new product indicates the one produced by the enterprise but not authorised by government, and the time period from production date is less than one year. Generally the new products contain new elements such as technology, design and manufactory process, which will improve the quality and feature of the product. Otherwise, the prominent features can expand the social needs and application scope of products, thus it plays a significant role in promoting economic efficiency for enterprise. Meanwhile, some outstanding performances of new products may lead the other similar producers to improve applicability and quality through potential radiation scope. It can be seen that new product sale income reflects market application value of innovation technology and also represents economic benefits after technical innovation for enterprises in industrial cluster.
5.1.2 Modelling of the impact
The nature of regional innovation capacity is the process of regional knowledge creation and diffusivity cultivation. According to the literature review and impact mechanism, resources such as human resource and capital are necessary for technical innovation. We make following hypothesis:
Hypothesis 1: R&D human resource input is an important factor which affects regional innovation capacity, and is helpful to improve regional innovation capacity.
Hypothesis 2: R&D capital investment has a significant impact on regional innovation capacity, and there is positive correlation relationship between them.
Hypothesis 3: Industrial agglomeration degree is an important factor which affects regional innovation capacity, and there is positive correlation relationship between them.
Hypothesis 4: In addition to necessary elements, we do not take into any other factors, such as market and policy.
Thus, we construct the function as follows:(2)
where L represents the human resource input, K represents the capital investment, and AE denotes the agglomeration degree. By further calculation, the number of patents applications, and the sale income for the new product can be obtained.
Take the logarithm of both sides of equation (Equation2(2) ):
(3)
5.2 Impact of the industrial cluster life cycle of the Yangtze River Delta area on the regional innovation capacity
Referring to the calculation of the cluster agglomeration degree and the basic principle of impact model above, we can construct the regression equation with the number of patents applications and the new product sale income as variables, respectively. The filtering yields 54 samples. In general, a statistical grouping method would be adopted to measure the impact of the industrial cluster on the regional innovation capacity. By dividing the samples into groups and estimating the model, the quantity dependency relationship between the explanatory variable and the explained variable of each group are displayed, which is convenient for distinguishing the discrepancy of the intercept and slope of each model. This is the advantage of a statistical grouping method. On the other hand, grouping cuts the original sample number into a small set and decreases the number of variables for estimating each model. Furthermore, the grouping method weakens the mutual relationships between each model and cuts off the direct contact contained between the qualitative variables, quantitative variables, and the quantitative and qualitative variables; this method even neglects the comprehensive influence of the contact. To strengthen the theoretical stringency of the result and overcome the limit of the sample size, a time virtual variable is drawn into the traditional segmentation regression model. The virtual variable is also referred to as dummy variable or binary variable which is generally represented by D. The values of 0 and 1 are assigned to the mutually exclusive property or character of the same factor in the virtual variable method. In a piecewise regression analysis, the virtual variable method can be used in modelling when the turning point appears during a certain period or exceeds a certain quantitative limit. Compared to traditional statistic grouping method, importing the proper virtual variable is beneficial for fitting all the samples from different groups in a model, and each group model can be deduced by the general model. The virtual variable method can balance the weaknesses of insufficient samples of the traditional statistic grouping method. There are now three stages, including the emerging, growth and maturity stages of the general equipment industry in the Yangtze River Delta area. Considering the situation of the empirical object, the specific equations are as below:(4)
Transform equation (Equation3(3) ):
(5)
where X1 represents human resource input, X2 represents capital investment, and X3 denotes agglomeration degree. Bring in the time virtual variable:(6)
Equation (Equation6(6) ) was constructed in Eviews 6.0 with the data of the Yangtze River Delta area. The results of the structure equations are shown in Table below.
Table 7. Impact of the industrial cluster life cycle of the Yangtze River Delta area on the regional innovation capacity.
The settings of the time virtual variables in the various stages of the industrial cluster life cycle are combined in formula (4). The relevant regression equations are as below.
Take the new product sale income as the dependent variable:(7)
(8)
(9)
Take the number of patents applications as the dependent variable:(10)
(11)
(12)
The analysis of formulas (7) to (12) shows the following:
Firstly, the impact of the industrial cluster life cycle of the Yangtze River Delta area on the regional innovation capacity varies in each stage. All the coefficient of X3 are positive, which demonstrates a positive impact of the cluster on the regional innovation capacity and corresponds to hypothesis 3. In addition, all the coefficient of X1andX2are positive, which demonstrates a positive impact of the input of R&D human resources and capital on the regional innovation capacity and corresponds to hypothesis 1 and hypothesis 2.
Secondly, there is a change of the impact on the regional innovation capacity during the entire life cycle of the general equipment manufacturing industry cluster in the Yangtze River Delta area. From the horizontal development process of life cycle with the new product sale income as the dependent variable, the coefficient of X3 rises from 0.130807 to 0.674488. Taking the number of patents applications as the dependent variable, the coefficient of X3 rises from 0.027316 to 0.771737. In both cases, the increment of coefficient X3 demonstrates a significantly increasing impact of the industrial cluster with time, which reaches its peak during the maturity stage. In addition, when the cluster enters the decline stage, the impact will become less significant.
Thirdly, comparisons of the regression coefficients reveals that the regression coefficients of X1 and X2are higher than those ofX3 in all stages of the life cycle. This indicates that the improvement of the regional innovation capacity depends on the input of R&D human resources and capital, which are still the key factors. The influence of the industrial cluster on the regional innovation capacity by utilising the innovation network increases with time, which is also the reality of the current development situation of the Chinese industrial cluster.
6. Conclusion and prospects
From the technical innovation perspective, the impact of industrial clusters on regional innovation capacity differs in the various stages of the clusters’ life cycle due to the integral degree of the cluster innovation networks. Generally speaking, innovation networks become more completed and functional from the emerging to the maturity stage of the clusters, which contributes to the regional innovation capacity.
In this article, we provided the identification methodology of industrial clusters by combining the graphical index method with the agglomeration degree measurement method. By applying this methodology, we identified the life cycle of the general equipment manufacturing industry cluster in the Yangtze River Delta area from various perspectives, and the results are confirmed. From this empirical study, we identified three stages in the development process: 1993–1998 as the emerging stage, 1999–2006 as the growth stage and 2007–2010 as the maturity stage.
Referring to the C-D production function, we took the industrial agglomeration degree as a factor, and with technical research fund and personnel input, we set up a mathematical model to represent the impact of the industry cluster on the regional innovation capacity. The model was verified quantitatively by using the general equipment manufacturing industrial cluster of the Yangtze River Delta area. The result shows that the impact of the industrial cluster on the regional innovation capacity is a periodical changing process that goes from enhancing to weakening with the evolution of the cluster life cycle.
From the results of the empirical study, although the impact of the industrial cluster and the internal innovation network has a positive effect on improving the regional innovation capacity, they are clearly less important than labour, capital and other factors. This fact denotes the deficient element, the structure of the innovation network, and the low degree of cooperation innovation in the Yangtze River Delta area. The root cause is that the low-level industrial cluster and regional innovation capacity still highly rely on the traditional low cost advantage in competition. Furthermore, industrial clusters are not formed for technical innovation but for location and resource advantages and the market environment. The government should undertake efficient actions and establish relevant policies to enhance the impact of industrial clusters on regional innovation capacities and promote the upgrading of China’s industrial clusters. In future research, we will deepen and expand this research, including factor weight distribution in location quotient composite indexes, construction of regional innovation capacity index system and comparative study of more regions. In addition, there is another question worth discussing: does mutual influence exist between industrial clusters and regional innovation capacity?
Disclosure statement
No potential conflict of interest was reported by the authors.
References
- Arvanitis, S., Lokshin, B., Mohnen, P., & Wörter, M. (2013). Impact of external knowledge acquisition strategies on innovation. KOF Working Papers, 2013, NO. 325.
- Asheim, B. T., & Isaksen, A. (2002). Regional innovation systems: The integration of local “stick” and global “ubiquitous” knowledge. The Journal of Technology Transfer, 27, 77–86.10.1023/A:1013100704794
- Asheim, B. T., Smith, H. L., & Oughton, C. (2011). Regional innovation systems: Theory, empirics and policy. Regional Studies, 45, 875–891.10.1080/00343404.2011.596701
- Audretsch, D. B. (1998). Agglomeration and the location of innovation activity. Oxford Review of Economic Policy, 14, 18–29.10.1093/oxrep/14.2.18
- Balland, P. A., Vaan, M. D., & Boschma, R. (2013). The dynamics of interfirm networks along the industry life cycle: The case of the global video game industry, 1987-2007. Journal of Economic Geography, 13, 741–765.10.1093/jeg/lbs023
- Bhattacharya, M., & Bloch, H. (2004). Determinants of innovation. Small Business Economics, 22, 155–162.10.1023/B:SBEJ.0000014453.94445.de
- Boix, R., Hervás, J. L., & De Miguel, B. (2014). Micro-geographies of creative industries clusters in Europe: From hot spots to assemblages. Papers in Regional Science. doi: 10.1111/pirs.12094.
- Boutellier, R., Gassmann, O., & Zedtwitz, M. (2008). Managing global innovation: Uncovering the secrets of future competitiveness. Berlin: Springer.10.1007/978-3-540-68952-2
- Brenner, T. (2006). Identification of local industrial clusters in Germany. Regional Studies, 40, 991–1004.10.1080/00343400601047408
- Brenner, T., Cantner, U., Fornahl, D., Fromhold, M., & Werker, C. (2011). Regional innovation systems, clusters, and knowledge networking. Papers in Regional Science, 90, 243–249.10.1111/j.1435-5957.2011.00368.x
- Breschi, S., & Lissoni, F. (2009). Mobility of skilled workers and co-invention networks: An anatomy of localized knowledge flows. Journal of Economic Geography, 9, 1–30.
- Broekel, T., & Boschma, R. A. (2012). Knowledge networks in the Dutch aviation industry: The proximity paradox. Journal of Economic Geography, 12, 409–433.10.1093/jeg/lbr010
- Broersma, L., & Oosterhaven, J. (2009). Regional labor productivity in the Netherlands: Evidence of agglomeration and congestion effects. Journal of Regional Science, 49, 483–511.10.1111/jors.2008.49.issue-3
- Brusco, S. (1990). The idea of the industrial district: Its genesis. In F. Pyke, G. Becattini, & W. Sengenberger (Eds.), Industrial districts and inter-firm cooperation in Italy (pp. 10–19). Geneva: International Institute for Labour Studies.
- Caniëls, M. C. J., & Romijn, H. A. (2005). What drives innovativeness in industrial clusters? Transcending the debate. Cambridge Journal of Economics, 29, 497–515.10.1093/cje/bei018
- Carbonara, N., Giannoccaro, I., & Pontrandolfo, P. (2002). Supply chains within industrial districts: A theoretical framework. International Journal of Production Economics, 76, 159–176.10.1016/S0925-5273(01)00159-1
- Carlino, G. A., Chatterjee, S., & Hunt, R. M. (2007). Urban density and the rate of invention. Journal of Urban Economics, 61, 389–419.10.1016/j.jue.2006.08.003
- Castells, M., & Hall, P. (1994). Technopoles of the world: The making of twenty-first -century industrial complexes. London: Routledge.
- Chen, L. F., & Yan, L. (2012). Identification of industrial clusters of oil and gas in china based on composite location quotient. China Population, Resources and Environment, 2, 152–158.
- Chiu, Y. H. (2009). How network competence and network location influence innovation performance. Journal of Business & Industrial Marketing, 24, 46–55.
- Cooke, P. (2002a). Knowledge economies: Clusters, learning and cooperative advantage. London and New York: Routledge.10.4324/9780203445402
- Cooke, P. (2002b). Regional innovation systems: General findings and some new evidence from biotechnology clusters. The Journal of Technology Transfer, 27, 133–145.10.1023/A:1013160923450
- Costa, M., & Iezzi, S. (2004). Technology spillover and regional convergence process: A statistical analysis of the Italian case. Statistical Methods and Applications, 13, 375–398.
- Duque, J. C., Rey, S. J., & Gomez, D. A. (2010). Identifying industry clusters in Colombia based on graph theory. Ensayos Sobre Poitica Economica, 59, 14–45.
- Durlauf, S. N., & Blume, L. E. (2012). The new Palgrave dictionary of economics (2nd ed.). London: Palgrave Macmillan.
- European Commission (2014). Regional innovation scoreboard 2014. Brussels: European Commission.
- Feser, E. J., & Bergman, E. M. (2000). National industry cluster templates: A framework for applied regional cluster analysis. Regional Studies, 34, 11–19.
- Freeman, C. (1991). Network of innovators: A synthesis of research issues. Research Policy, 20, 499–514.10.1016/0048-7333(91)90072-X
- Fri, W., Pehrsson, T., & Søilen, K. S. (2013). How phases of cluster development are associated with innovation - the case of China. International Journal of Innovation Science, 5, 31–44.10.1260/1757-2223.5.1.31
- Fritsch, M., & Graf, H. (2010). How general conditions affect regional innovation system- the case of the two Germanys. Papers in Regional Science, 90, 331–354.
- Fritsch, M., & Slavtchev, V. (2010). How does industry specialization affect the efficiency of regional innovation systems? The Annals of Regional Science, 45, 87–108.10.1007/s00168-009-0292-9
- Galarraga, J. M., Paluzie, E., Pons, J., & Fabregat, D. T. (2008). Agglomeration and labor productivity in span over the long term. Clinometrica, 2, 195–212.10.1007/s11698-007-0017-1
- Gersbach, H., & Schmutzler, A. (1999). External spillovers, internal spillovers and the geography of production and innovation. Regional Science and Urban Economics, 29, 679–696.10.1016/S0166-0462(99)00026-5
- Gilbert, B. A., McDougall, P. P., & Audretsch, D. B. (2008). Clusters, knowledge spillovers and new venture performance: An empirical examination. Journal of Business Venturing, 23, 405–422.10.1016/j.jbusvent.2007.04.003
- Gong, J. L., Jin, R. W., & Wang, F. R. (2008). Study on the life cycle of regional industrial clusters. Economic Tribune, 23, 4–7.
- Gordon, I. R., & McCann, P. (2005). Innovation, agglomeration, and regional development. Journal of Economic Geography, 5, 523–543.10.1093/jeg/lbh072
- Hassink, R., & Klaerding, C. (2012). The end of the learning region as we knew it: Towards learning in space. Regional Studies, 46, 1005–1066.
- Holmen, M., & Jacobson, S. (2003). A cluster analysis of the maritime sector in Norway. International Journal of Transport Management, 1, 203–215.
- Hu, J., & Jiao, B. (2008). Impact of oil and gas industry cluster on innovation ability of regions. Modern Economic Science, 30, 30–35.
- Isaksen, A. (1997). Regional clusters and competiveness: The Norwegian case. European Planning Studies, 5, 65–76.10.1080/09654319708720384
- Karlsen, J. (2013). The role of anchor companies in thin regional innovation systems lessons from Norway. Systemic Practice and Action Research, 26, 89–98.10.1007/s11213-012-9266-4
- Keeble, D., & Wilkinson, F. (1999). Collective learning and knowledge development in the evolution of regional clusters of high technology SMEs in europe. Regional Studies, 33, 295–303.10.1080/00343409950081167
- Kelton, C., Margaret, K. P., & Robert, P. R. (2007). Using the North American Industry Classification System (NAICS) to identify national industry cluster templates for applied regional analysis. Regional Studies, 42, 305–321.
- Lawson, C., & Lorenz, E. (1999). Collective learning, tacit knowledge and regional innovative capacity. Regional Studies, 33, 305–317.10.1080/713693555
- Lissoni, F. (2001). Knowledge codification and the geography of innovation: The case of Brescia mechanical cluster. Research Policy, 30, 1479–1500.10.1016/S0048-7333(01)00163-9
- Liu, H. J., & Chen, J. X. (2004). Statistics of foreign industrial cluster and the development countermeasures of China. Statistical Research, 10, 18–23.
- Liu, B., Chen, Q., & Wang, Z. H. (2007). Research on identification of industrial cluster - taking Jiangsu as an example. Studies in Science of Science, 25, 85–88.
- Lu, J. W., & Beamish, P. W. (2006). Partnering strategies and performance of SMEs’ international joint ventures. Journal of Business Venturing, 21, 461–486.10.1016/j.jbusvent.2005.02.002
- Ma, J. W. (2013). Review and future prospects of researches on venture capital investment clusters. Foreign Economics & Management, 35, 43–51.
- Martin, R. L., & Sunley, P. J. (2011). Conceptualising cluster evolution: Beyond the life cycle model? Regional Studies ( Special Issue on Cluster Evolution R. Boschma & D. Fornahl (Eds.), 45 (pp. 1295–1318).
- Menzel, M. P., & Fornahl, D. (2010). Cluster life cycles – dimensions and rationales of cluster evolution. Industrial and Corporate Change, 19, 205–238.10.1093/icc/dtp036
- Molina, B. M., Boix, R., & Oliver, J. H. (2012). The importance of creative industry agglomerations in explaining the wealth of European regions. European Planning Studies, 20, 1263–1280.10.1080/09654313.2012.680579
- Morosini, P. (2004). Industrial clusters, knowledge integration and performance. World Development, 32, 305–326.10.1016/j.worlddev.2002.12.001
- Mothe, J., & Paquet, G. (1998). Local and regional systems of innovation. New York, NY: Springer Science & Business Media.10.1007/978-1-4615-5551-3
- Norberg, V. (1999). Stimulating”green”technological innovation: An analysis of alternative policy mechanisms. Policy Sciences, 32, 13–38.10.1023/A:1004384913598
- Palmatier, R. W., Dant, R. P., & Crewel, D. A. (2007). Comparative longitudinal ana1ysis of theoretical perspectives of interorganizational relationship performance. Journal of Marketing, 71, 172–194.10.1509/jmkg.71.4.172
- Pan, X. F., & Liu, F. C. (2008). Regional differences analysis of patent development of China. Forum on Science and Technology in China, 8, 93–96.
- Porter, M. E. (1998). Clusters and the new economics of competition. Harvard Business Review, 76, 77–90.
- Storper, M., & Venables, A. J. (2004). Buzz: Face-to-face contact and the urban economy. Journal of Economic Geography, 4, 351–370.10.1093/jnlecg/lbh027
- Sullivan, M. G., & Weerawardena, J. (2006). Networking capability and international entrepreneurship: How networks function in Australian born global firms. International Marketing Review, 23, 549–572.
- Sun, J., & Gong, Z. L. (2010). Research on the maturity model and evaluation index system of industry cluster. Technoeconomics & Management Research, S2, 120–124.
- Tavassoli, S., & Tsagdis, D. (2014). Critical success factors and cluster evolution: A case study of the Linköping ICT cluster lifecycle. Environment and Planning A, 46, 1425–1444.10.1068/a46258
- Tichy, G. (1998). Cluster: Less dispensable and more risky than ever (pp. 226–237). Pion Limited: Clusters and Regional Specialisation.
- Titze, M., Brachert, M., & Kubis, A. (2011). The identification of regional industrial clusters using Qualitative Input–Output Analysis (QIOA). Regional Studies, 45, 89–102.10.1080/00343400903234688
- Tsai, K. H., & Wang, J. C. (2007). Inward licensing, firm performance: A longitudinal study. R&D Mannagement, 37, 151–160.
- Varga, A. (2006). Spatial knowledge spillovers and university research: Evidence from Austria. Innovation, Networks, and Knowledge Spillovers, 211–232.10.1007/3-540-35981-8
- Wallsten, S. J. (2001). An empirical test of geographic knowledge spillovers using geographic information systems and firm-level data. Regional Science and Urban Economics, 31, 571–599.10.1016/S0166-0462(00)00074-0
- Walter, A., Auer, M., & Ritter, T. (2006). The impact of network capabilities and entrepreneurial orientation on university spin off performance. Journal of Business Venturing, 21, 541–567.10.1016/j.jbusvent.2005.02.005
- Wang, B., & Hou, M. Z. (2007). Research on the correlation between local industrial clusters’ international development and regional innovation system. Finance & Trade Economics, 3, 11–19.
- Watts, H. D., Wood, A. M., & Wardle, P. (2003). ‘Making friends or making things?’: Interfirm transactions in the sheffield metal-working cluster. Urban Studies, 40, 615–630.10.1080/0042098032000053950
- Ye, D. F. (2009). Study on regional innovation environment of high tech industry in Pearl River Delta. Modern Science, 6, 19.
- Yueh, L. (2009). Patent laws and innovation in China. International Review of Law and Economics, 29, 304–313.10.1016/j.irle.2009.06.001
- van Zeebroeck, N., Stevnsborg, N., van Pottelsberghe de la Potterie, B., Guellec, D., & Archontopoulos, E. (2008). Patent inflation in Europe. World Patent Information, 30, 43–52.10.1016/j.wpi.2007.05.010
- Zhang, D. Z., & Du, W. J. (2008). Analysis on technological innovation diffusion of industrial cluster based on the life cycle of cluster. Commercial Research, 11, 54–57.