Abstract
The purpose of this paper is to study how clustering affects the results of operations of small and medium hospitality enterprises (SMHEs) in Croatia and to support SMHEs tend to cluster, forming critical masses in one place, to take advantage of synergies such as increased productivity, a higher pace of innovation and in essence the possibility of becoming more competitive. What are clusters and in what way do they increase business performance and the competitiveness are frequently asked questions. The paper presents the results of the impact of clustering in SMHEs in the Republic of Croatia on business performance. The special focus is given to financial and non-financial indicators that are significant for measuring business performance in SMHEs. The methodology of research includes a literature search of clustering in hospitality enterprises research, collection of data directly from managers of SMHEs and from the Croatian Bureau of Statistics, analysis of the financial and non-financial data of 72 SMHEs in the Republic of Croatia. The research takes a longitudinal approach, examining SMHEs that are in clusters in Croatia over a two-year period as well as a control group of non-clustered SMHEs for comparison. The emphasis is on seeking to establish the impact of clustering on business performance. In this paper, the impact of clustering of 72 SMHEs involved in the study is discussed. The research has shown that the advantages of clustering in SMHEs have not been utilised in Croatia. The originality of this research is based on the fact that it refers to an area that has not been explored enough in the Republic of Croatia.
1. Introduction
The pressure of globalisation is having a major impact on the small and medium hospitality enterprises (SMHEs) of the European tourism industry. Globally-acting suppliers, decreasing transportation costs and emerging new destinations have put pressure on the European SMEs in traditional destinations. As many European countries are economically very dependent on tourism, a need for effective policy support arises. Because hospitality enterprises are exposed to strategic risks related to increased uncertainty in their economic, technological, political, competitive, and market environments (Olsen, West, & Tse, Citation1998), organisational survival becomes an ever more pressing issue. Enterprises have realised, especially SMHEs, that value-adding resources are becoming increasingly scarce, and they have used different measures to address this issue. SMHEs that have used collaborative ventures to address this issue have realised that entering into clusters with competitors, suppliers and customers, and enterprises in other industries such as agriculture, is an opportunity to build strengths. It is important for hospitality professionals to investigate the concept underlying cluster theory in order to assess the potential of this business strategy to be a source of competitive advantage that can improve and sustain SMHEs’ growth and competitiveness. Clustering allows SMHEs to achieve economies of scale and synergies.
Clusters are the critical moving force of open innovation and the key source of competitive advantage in the global economy. An increase in competitiveness of Croatian tourism must be achieved by strengthening the clustering process, and this especially refers to the SMHEs sector. SMHEs in a cluster primarily have the following advantages: an increase in employment and productivity, an increase in the quality of hotel products and services and an innovation boost. Over the last century, there was a sudden increase in interest regarding hospitality clustering, and the growth of globalisation inevitably imposes the necessity to develop tourist clustering as the only possible survival of global tourism, which includes SMHEs. SMHEs develop more quickly thanks to the impact of clustering, by applying contemporary working methods faster and drawing the maximum from the market environment. Through these, SMHEs gain competitive advantages in relation to others. The competitiveness of local SMHEs, which operate in the business environment, will increasingly depend on the ability to cooperate and form clusters on the local level, and competitiveness of any SMHEs in relation to others will depend in the future on the effectiveness and productivity of its clusters. The impact of clustering of SMHEs greatly improves effectiveness and productivity. SMHEs are clustering to be able to offer a high quality hotel product. This paper will attempt to explore the impact of clustering on business performance, especially on the effectiveness and productivity of SMHEs, which cluster with others touristic enterprises because of SMHEs’ development in the future. The starting point was a survey conducted in 2011 in selected hotels that are clustering, and SMHEs which are not clustering.
The paper is structured as follows: Section 2 examines the literature review on the importance of clusters for business operations and clustering in SMHEs. The methods applied in the study are described in Section 3 and research findings are presented in Section 4. Section 5 presents concluding comments.
2. Literature review
Entrepreneurial activities are increasingly regarded as important to firms, but in today’s complex global economy, entrepreneurship has become even more crucial in obtaining a sustainable competitive advantage (Wiklund & Shepherd, Citation2003). Due to globalisation, small and medium sized enterprises (SMEs) face increasing pressure from competition from across the world. When compounded with the changing sophistication of customers worldwide it becomes apparent that SMEs face increasing difficulty in maintaining and improving business performance in time, unless they can actively manage these pressures. SMEs are encouraged to implement an entrepreneurial mindset to recognise the threats and opportunities in the environment of the firm in order to make sure that the firm will continue to exist in the future (Krueger, Citation2000). In periods of economic and environmental turbulence, it becomes even more apparent that firms face particularly high levels of market instability and complex business uncertainty that obliges them to act upon such change (Grewal & Tansuhaj, Citation2001; Lin & Carley, Citation2001). A firm-level response is therefore needed (Chattopadhyay, Glick, & Huber, Citation2001). Fitzgerald, Johnston, Brignall, Silvestro, and Voss (Citation1991) suggest that there are two basic types of property management (PMs) in any organisation: those that relate results (competitiveness and financial performance) and those that focus on the determinants of the results (quality, flexibility, resource utilisation, and innovation). Van Landeghem and Persoons (Citation2001) build a causal model relating the use of best practices to the resulting performance grouped under four objectives: flexibility, reaction time, quality and cost. According to Gunasekaran and Kobu (Citation2007) there are 27 key performance indicators. The most widely used are financial performance indicators. Time and productivity have significant weightiness in measuring the performance. Nonfinancial performance measuring received due attention in supply chain systems.
Clustering phenomena are today an increasingly popular and significant topic with researchers, scholars (economists, urban planners, and sociologists), professionals and policymakers. Today, clusters represent an important lever for the development of territories. Moreover, nowadays the competitive environment requires not only individual enterprises but all of a cluster’s economic actors to have the ability to build and plan a networking system to develop programmes. This represents a task that economic actors are not able to provide individually. In fact, the increasing systemic nature of innovation processes implies the need for effective relational networks matching different economic actors, sectors and geographic areas (Brown, Burgess, Festing, & Royer, Citation2013). Clusters and SME networks can get better results than individual enterprises and facilitate the development of foreign markets and innovations. Secondly, they reduce the financial needs of enterprises, both for the components of circulating and of fixed capital. Third, the share of investment leads to a spreading of risk between enterprises. This last aspect is extremely convenient for banks as it has been verified that an organised network of enterprises with fewer failures helps to improve the rating of enterprises. Clusters in a narrow sense are usually only a small part of the regional economy, but are often central as transmitters of knowledge and innovation to the individual enterprises or entrepreneur. Therefore, clusters and cluster policy are often considered to be an efficient tool in transferring policy into economic growth and competitiveness, and play an increasingly prominent role in business development and industrial policy (Brown et al., Citation2013)
In Porter’s view ‘a cluster is geographically proximate group of interconnected firms and associated institutions in related industries’ (Porter, Citation1998). Cooperation among participants in the cluster generates a synergy effect improving efficiency and competitiveness on the market and reducing uncertainty regarding the future. Enterprises acting in clusters can exploit available resources and means of production in a more efficient and effective way. By using relatively cheap resources, a cluster stimulates productivity growth allowing members to increase their profits. Nevertheless, effective clusters support employees’ earnings growth enhancing higher levels of motivation. A cluster’s members can collaborate and organise joint distribution channels and supply systems. Inside the cluster collective learning is promoted, and innovation is usually stimulated, which leads to improved operational efficiency. Based on increased confidence among cluster’s members, specialisation and higher diffusion of information are stimulated and, as a consequence, costs can be cut, quality can be improved and turnover can increase significantly (Dragusin, Constantin, & Petrescu, Citation2010). The clusters’ positive impact is measured using indicators such as: production volume, costs, sales volume, number of generated jobs, wages, productivity, prices, value added, number of enterprises, innovation, etc. Clustering affected on the productivity growth. Contemporary competitiveness depends on productivity rather than on access to inputs or enterprises size. The characteristic of clusters is constantly improving and innovating to maintain advantages over imitators. Cluster allows each member has the benefit for increasing scope of business and other activities, that is networked with the others, without the requirement that is each participant renounces of its flexibility (Dragičević & Obadić, Citation2013). Therefore, cluster development in certain industries, regions or countries has multiple significance. In fact, clusters not only lead to increased competitiveness in an industry or region, but also increase employment and the mutual confidence of the participants in the cluster.
Clusters in the hospitality industry are favoured by endogenous factors such as: advantageous geographical location, developed transportation and telecommunication links, developed technical infrastructure and tradition (Pasieczny, Citation2007). All the case studies reveal examples of wider linkages to other vertical and/or horizontal networks and, in this sense, one can see the formation of networks in clusters in the hospitality industry. Such networks individually and collectively provide value for the network participant by leveraging knowledge and resources they would not otherwise readily gain and thereby aid their competitive capabilities and effectiveness in the hospitality industry (Michael et al., Citation2007).
Micro and small hospitality enterprises dominate the tourism sector. Despite their size, they assume an important role in local economic development, delivering an enormous contribution to the development of peripheral areas. Recent transformations in the global market have however posed a challenge to these enterprises, which now recognise the importance of cooperation with other tourism partners as a way to develop strategic positioning and capacity to operate in a competitive environment (Scott, Baggio, & Cooper, Citation2008). By cooperating in the form of geographical and product-based clusters, SMHEs can function as dynamic and innovative organisations.
A hospitality cluster belongs to the service class of clusters and can be stimulated by certain natural characteristics of an area. Clusters in SMHEs are mainly informal, shallow with a weak relationship. They are more stable and less fragile when facing economic depressions. The clusters’ effects on SMHEs are strong and their presence is a key part of the attractiveness of a specific location. The clusters’ development is frequently supported by regional professional associations and the establishment of enterprises involved in promotion and consulting, capable of attracting foreign capital. Clusters in SMHEs can also emerge with the appearance of an important investor.
Tourist clusters include the interaction between the various players, including competing enterprises from various sectors, such as: accommodation, transportation, travel agencies and tour operators, all kinds of suppliers, tourist attractions, non-economic organisations (banks, insurance companies, etc.) educational and government institutions. The interaction applies to connections among SMHEs, their suppliers and the tourists themselves. In this case, there is the ‘vertical model’ connectivity, which will result in partner relations based on the exchange of information, joint operations and importantly confidence. Concentration in the cluster based on integrating of touristic offer, which operates on the principle of networking tourist attraction, physical and communications infrastructure, accommodation, transport, travel agencies, agricultural producers and the others ancillary services. As vertically connecting SMHEs, which usually includes chains of travel agencies and airlines, one of the common models of connectivity, especially at the international level, it is their influence on the same level of crucial importance for the positioning of certain regions or destinations in the development of the tourism market. The second model involves linking ‘horizontal’ links between and within the hospitality industry (from tourism and related activities) in the cluster, where each enterprise manages the dual objectives of competitiveness and cooperation in business, which is the subject of common interest and operations (they join forces in their demands to the government to obtain certain benefits, or to avoid some of the negative consequences of government activities). Therefore, it’s about the numerous interactions between the different players that determine the ability of tourism clusters to be competitive, to grow and develop, and at the same time be aware of its benefits. Other players who are outside the cluster should adjust their roles and principles that determine the competitiveness of the cluster.
3. Methodology: research design and research objectives
Research on the impact of clustering on the business performance of SMHEs is based on 72 samples collected in the period July to September 2011. The survey collected data on the current state of clustering of SMHEs in Croatia. The obtained data were taken as a starting point to explore the impact of clustering on the business performance of SMHEs in Croatia. The survey was sent to 200 SMHEs, and 72 managers responded. Of the total number of hotel enterprises that are included in this study 52.8% are in clusters. During the interviews, the managers of SMHEs were asked to assess the competitive capacity of enterprises and then financial data for 2011 and 2012 was collected from the Croatian Bureau of Statistics to measure business performance. The Croatian Bureau of Statistics delivered the financial indicators for 85% of the SMHEs. SMHEs were divided into two groups, the first one is SMHEs that are in a cluster and the other is SMHEs that are not. In order to determine the impact of clustering on the business performance of SMHEs we set the following hypotheses:
H1: SMHEs that are in clusters have the same rate of productivity as those who are not in a cluster.
H2: SMHEs that are in clusters have the same rate of effectiveness as those who are not in a cluster.
The use of dominant financial indicators and a focus on short-term goals are considered to be outdated measurement systems. It is considered important that the focus be on achieving a balanced framework that addresses operational issues including financials and managers factors. During the interviews, the managers were first asked a number of open-ended questions and were then presented with a list of non-financial indicators for assessing competitive capabilities (using a five point Likert Scale, where one represented ‘very bad’ and five ‘very satisfying’). It is very important to incorporate into regular business performance evaluation non-financial measures such as quality, market share, customer satisfaction, human resources, innovation and learning. According to the above, we have the following hypotheses::
H3: Evaluation results of the service quality SMHE managers who are in the cluster are not significantly different from those who are not in a cluster.
H4: Evaluation results of the market share SMHE managers who are in the cluster are not significantly different from those who are not in a cluster.
H5: Evaluation results of the customer satisfaction SMHE managers who are in the cluster are not significantly different from those who are not in a cluster.
H6: Evaluation results of the market potential SMHE managers who are in the cluster are not significantly different. from those who are not in a cluster.
4. Research results
First, we tested a differences in financial indicators among two groups. To test H1 it is necessary to calculate the rate of change in productivity and to test H2 the rate of change in efficiency, as significant financial indicators of business performance, and to compare them. Figure shows the surface diagram on which one can see the representation of the particular rate of change in productivity in SMHEs which are associated into clusters and those that are not.
Figure 1. The representation productivity rate in SMHEs that are associated and those that are not associated with a cluster. Source: authors’ data processing.
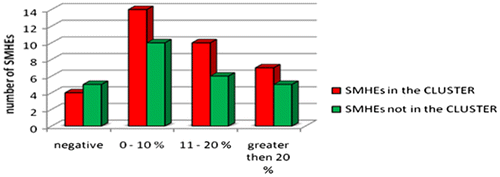
The rates of change in productivity shown in Figure are divided into four grades. SMHEs that are in the cluster are marked with 0 while those that are not in the cluster are marked with 1. From the figure it can be concluded that the number of SMHEs with positive rate of change in productivity that are in clusters is larger than those who are not in clusters.
To test Hypothesis 1,a chi-square test was examined as to whether there is a significant difference in the rate of change in productivity among SMHEs that are in a cluster and those that are not. Test results are shown in Table .
Table 1. Chi-square tests.
The critical value of chi-square is 7.81473 for 5% level of significance and three degrees of freedom. The chi-square test statistics is 1.653 and it is less than the critical value so the null hypothesis is true. Despite the above distribution, the chi-square test showed that there is no significant difference in the rate of change in productivity among small and medium hospitality enterprises that are in a cluster and those which are not.
The same method is applied to the rate of change in efficiency. Figure shows the distribution of a particular rate of change in effectiveness in SMHEs that are associated in the cluster and those that are not.
Figure 2. The representation rate in the effectiveness SMHEs that are associated and those that are not associated with a cluster. Source: Authors’ data processing.
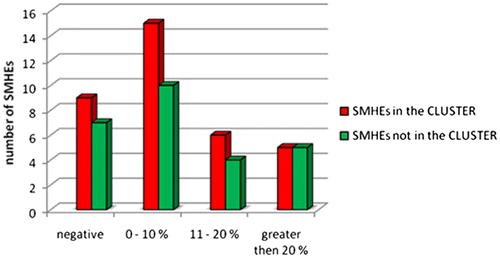
Figure shows that a higher proportion of SMHEs who have a negative rate of change in effectiveness are the ones that are in a cluster. As many as 15 SMHEs have a rate of change in effectiveness between 0% and 10% and ten hospitality enterprises that are not associated in the cluster have the same rate of change in the effectiveness. There are six SMHEs that are associated in the cluster, and have rate of 11–20% and four SMHEs in the same class. The same number of SMHEs that are associated in the cluster and that are not associated in the cluster have the rate of change in effectiveness higher than 20%.
To test the hypothesis, a chi-square test is used to check whether there is a significant difference in the rate of change in the effectiveness among SMHEs that are in a cluster and those that are not. The test results are shown in Table .
Table 2. Chi-square tests.
The critical value of chi-square is 7.81473 for 5% level of significance and three degrees of freedom. The chi-square test statistics is 4.776 and it is less than the critical value so it can be concluded that there is no significant difference in the rate of change in the effectiveness among SMHEs that are in clusters and those that are not in clusters.
As previously mentioned, to draw conclusions about the business performance financial indicators are not sufficient. Therefore, interviews were conducted with the managers of SMHEs to collect non-financial indicators of business performance.
After analysis of these data and testing the difference between the two groups in the assessment of their competitive capabilities, the final results are set out in Table .
Table 3. Chi-square tests of non-financial indicators.
The results in Table indicate that Hypothesis 3, 4 and 6 are confirmed. The empirical values of three non-financial indicators are less than the size of the critical values of the chi-square indicator, only for variable customer satisfaction critical value is greater than empirical value, so the following can be concluded:
• | There is no significant difference between the two groups in the assessment of their competitive capabilities with regard to: service quality, market share and market potential. | ||||
• | There is a significant difference in the assessment of their competitive capabilities with regard to customer satisfaction and for those SMHEs that are in the cluster it is significantly higher than for those SMHEs that are not. |
5. Discussion and study limitations
Clusters can have an important positive impact on participant small and medium hospitality enterprises, including access to local associations of suppliers and entrepreneurs, leading to lower transactional costs, higher public institutions support, establishment of new hospitality enterprises, new job creation, flow of knowledge and access to specialised personnel. Clustering can create major opportunities for undeveloped regions that can, accelerate their technological development and growth, due to the synergy effect.
Most of the authors that have explored the impact of clustering on business performance in enterprises consider that clusters affect the productivity of the enterprises. This paper is based on research conducted on the clustering of small and medium hospitality enterprises and its impact on business performance in selected hospitality enterprises in Croatia. One of the major problems associated with clustering is deciding on appropriate indicators for business performance comparison. This paper shows the impact of clustering in small and medium hospitality enterprises on financial and non-financial indicators. Based on the results of the tests it could be concluded that SMHEs in clusters are not significant different in terms of productivity, effectiveness, service quality, market share and market potential from the others who are not in clusters. This research shows that the benefits of clustering are not sufficiently exploited in Croatia. This paper is a starting point to encourage discussion and debate regarding why SMHEs that are within clusters in Croatia do not take advantage of their competitive possibilities. It must be noted that many elements for a complete analysis of business performance of SMHEs in Croatia are missing at this stage.
There are several limitations of this study. Some empirical results could be affected by measurement problems. For example, the rate of change in productivity and efficiency are not sufficient for assessing the performance of operating hotel enterprises. The data do not provide assessment of quality management. There is also a potential lack of objectivity since the evaluation of non-financial indicators depends on managers’ attitudes expressed in the interview in the absence of clearly defined non-financial ratios that could be compared over the sample. Lastly, the scope of the theory presented in this paper, and the dataset used to test it do not allow the drawing of generalizable conclusion about the impact of clustering in SMHEs.
We hope that the ideas presented in this paper will inspire and enable further research on these issues. We believe that the perspective of SMHEs and concepts developed here for describing and measuring impact of clustering on SMHEs performance are a step toward and improve understanding clustering importance.
6. Conclusions and directions for the future research
This paper shows, on the basis of conducted testing, that small and medium hospitality enterprises that are in clusters are not significant different than those hospitality enterprises that are not in clusters. The following can be concluded.
- | Very little clustering data are available about SMEs in general and even less regarding SMHEs | ||||
- | Accessing existing business data through well-known research agencies such as the Croatian Bureau of Statistics is a difficult, drawn-out and generally unsuccessful process | ||||
- | Obtaining business performance data directly from small and medium enterprises via a survey method is often difficult and not always reliable | ||||
- | It is important to collect financial and non-financial indicators for measuring business performance in SMHEs | ||||
- | The impact of clustering is not recognised as a source of competitive advantage for small and medium hospitality enterprises |
Disclosure statement
No potential conflict of interest was reported by the authors.
References
- Brown, K., Burgess, J., Festing, M., & Royer, S. (2013). Resources and competitive advantage in clusters. München: Rainer Hampp Verlag.
- Chattopadhyay, P., Glick, W. H., & Huber, G. B. (2001). Organizational actions in response to threats and opportunities. Academy of Management Journal, 44, 937–955.10.2307/3069439
- Dragusin, M., Constantin, D. L., & Petrescu, R. M. (2010). Clustering in transition economies: The case of Romanian tourism industry, 20th Biennial International Congress Tourism and Hospitality Industry 2010 “New trends in Tourism and Hospitality Management” (pp. 287–301). Opatija, Croatia: Faculty of Tourism and Hospitality Management.
- Dragičević, M., & Obadić, A. (2013). Klasteri i politike razvoja klastera [Clusters and cluster development policy]. Zagreb: Faculty of Economics University of Zagreb.
- Fitzgerald, L., Johnston, R., Brignall, S., Silvestro, R., & Voss, C. (1991). Performance measurement in service business. London: CIMA.
- Grewal, R., & Tansuhaj, P. (2001). Building organizational capabilities for managing economic crisis: The role of market orientation and strategic flexibility. Journal of Marketing, 65, 67–80.10.1509/jmkg.65.2.67.18259
- Gunasekaran, A., & Kobu, B. (2007). Performance measures and metrics in logistics and supply chain management: A review of recent literature (1995-2004) for research and applications. International Journal of Production Research, 45, 2819–2840.10.1080/00207540600806513
- Krueger, N. F. (2000). The cognitive infrastructure of opportunity emergence. Entrepreneurship Theory and Practice, 24, 5–23.
- Lin, Z., & Carley, K. M. (2001). Organizational design and adaption in response to crises: Theory and practice. Academy of Management Conference Best paper Proceedings, 2001, 1–7.
- Michael, E. J., Gibson, L., Hall, C. M., Lynch, P., Mitchell, R., Morrison, A., & Schreiber, C. (2007). Micro-clusters and networks: The growth of tourism. Oxford: Elsevier Ltd.
- Olsen, M. D., West, J. J., & Tse, E. C. (1998). Strategic management in the hospitality industry. Massachusetts: Wiley.
- Pasieczny, J. (2007). The factors and conditions of clustering processes and the development of clusters. In E. Bojar, & Z. Olesinski (Eds.), The emergence and development of clusters in Poland. Warsaw: Difin.
- Porter, M. E. (1998). Clusters and new economies of competition. Harvard Business Review, 76, 77–90.
- Scott, N., Baggio, R., & Cooper, C. (2008). Network analysis and tourism-from theory to practice. Clevedon: Channel View Publication.
- Van Landeghem, R., & Persoons, K. (2001). Benchmarking of logistical operations based on a causal model. International Journal of Operations & Production Management, 21, 254–267.10.1108/01443570110358576
- Wiklund, J., & Shepherd, D. (2003). Knowledge-based resources, entrepreneurial orientation, and the performance of small and medium sized business. Strategic Management Journal, 24, 1307–1314.10.1002/(ISSN)1097-0266