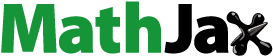
Abstract
The present paper has as main purpose to reveal, in a new-Keynesian manner, the evolution of the Romanian economy, as compared to that of the Eurozone, for a period of 14.5 years, since 2000 to 2014. The analysis is based on dynamic modelling, considering price and wage stickiness, as well as the manifestation of 20 structural shocks acting on and altering the model variables, all captured in the context of the general equilibrium of markets. The estimation of parameters and the standard deviation of shocks, achieved by resorting to the Bayesian approach, via the use of the Metropolis-Hastings algorithm, a Markov Chain Monte Carlo instrument, was rendered and construed accordingly, revealing compatibility not only with other authors’ results, but also with the economic reality of the time.
1. Introduction
The Dynamic Stochastic General Equilibrium (DSGE) models, based on Walrasian premises in terms of general equilibrium, but stochastically analysing, from a dynamic perspective, either closed economies, as in Schorfheide (Citation2013) or open economies, as in Liu & Mumtaz (Citation2011), Almeida et al. (Citation2013) or Merola (Citation2015), are built and used for estimation purposes, as in Adolfson, Laséen, Christiano, Trabandt, & Walentin (Citation2013) or Robinson (Citation2013) or for prediction purposes, as in Lees, Matheson, & Smith (Citation2011), Slobodyan & Wouters (Citation2012), Smets, Warne, & Wouters (Citation2014) or Costantini, Gunter, & Kunst, Citation2017, the capturing of the evolution of macroeconomic variables allowing for well-grounded economic decision making.
Since their launching phase, represented by the Real Business Cycle (RBC), the DSGE models, mainly based on the essential role of technology in generating welfare and on the rational expectations of economic agents, acquired new elements, like the stickiness of prices and wages, therefore turning towards the Keynesian side-approach.
Considering the monetary policy effects rendered by Christiano, Trabandt, & Walentin (Citation2010) or Sargent & Surico (Citation2011), who outlined the persistence of the related shock at the interest rate or monetary mass level, or by Barthélemy, Clerc, & Marx (Citation2011), who insisted upon the non-trivial role of money in the economy, the introduction of the monetary authority within the DSGE models became essentially important, occurring in parallel with their transformation into an instrument largely used by Central Banks worldwide.
Given the quick global spread of this last generation class of models, doubled by the increasing interest manifested for the same in approaching national economies, totally in contrast with their quite insignificant construction or implementation with regard to the Romanian economy, we decided to bring consistent added value into the matter, by dedicating the present paper to its DSGE estimation, perceived in interdependence with the Eurozone, its most important economic partner.
The model used for estimating Romanian economic life is based on the paper of Kolasa (Citation2009), revealing the dynamic evolution of this national economy in parallel with that of the Eurozone, remarkably captured by Smets & Wouters (Citation2003) and resumed, later, by Smets et al. (Citation2014). Such DSGE model, analysed from a new Keynesian perspective, assuming the famous and up-to-date Keynesian hypothesis of sticky prices and wages, à la Calvo (Citation1983) and Erceg, Henderson, & Levin (Citation2000), as in Çebi (Citation2012) or Knell (Citation2013), was selected given its appropriateness for the studied economy, on the one hand, and its relevance among the related DSGE models, on the other.
The above-mentioned form of estimation of the Romanian economy is just the first step in providing very useful information, from a modern perspective, about its manifestation across time, the analysis of impulse-response functions, reflecting the reaction of various variables to different structural shocks or the rendering of forecasts, as indices for the macroeconomic authorities relating to the best possible decisions to adopt, making them the object of further research, given the limited space of this paper.
The work is clearly structured, as follows: Model, in section 2; Data, in section 3; Methodology and Results, in section 4; and Conclusions, in section 5.
2. Model
The present model, developed around the new Keynesian premises laid down by Kolasa (Citation2009), considers five economic blocks, depicted below: households, firms, Central Bank, Government and Foreign sector.
There is a continuous series of domestic households defined on the interval [0,n], and of foreign households, defined on the interval [n,1], n being the size of the Romanian economy relative to the Eurozone economy.
Households are maximising their lifetime utility, depending on the level of consumption and spare time:
(1)
(1)
where Et is the current expected value, βk the subjective discount factor, ζtc the consumption preferences, Cj,t the consumption of household j, θ the consumption habit persistence, ζth the labour time-related preferences, Hj,t, the quantity of labour provided by household j, σ the reverse of the inter-temporal substitution elasticity, and σL the reverse of the labour elasticity in relation to its marginal disutilityunder the budget constraint:
(2)
(2)
where Bj,t is the zero-coupon domestic bonds held by household j, Rt the domestic gross interest rate, Ptc the consumption price index, Pti the investment price index, Ij,t the investment goods held by household j, Wj,t the nominal wage of household j, Rtk the capital rental rate, Kj,t the physical capital of household j, ΠHj,tthe dividends of household j, from producers of tradable goods, ΠNj,tthe dividends of household j from producers of non-tradable goods, and Trt the net governmental transfersand considering the capital stock accumulation equation:
(3)
(3)
where τ is the physical capital depreciation rate, χt the investment shock, and F(●) a function turning investments into physical capital:
(4)
(4)
where S(●) is the function of the investment adjustment cost, with
and
, as in Adolfson, Laséen, Lindé, and Villani (Citation2008)
The first order conditions relating to consumption and investments take the following form:
FOC relating to Ct:
(5)
(5)
where Ct is the aggregate consumption
FOC relating to It:
(6)
(6)
where λtCis the marginal utility of the nominal income, and λtK the marginal utility of an additional unit of resources used for physical capital
The action of domestic households on the market, as monopolistic suppliers of differentiated labour services, opens the possibility for the said households to set their own wage level. The differentiated labour force services thus provided are transformed, by a specialised company, into a homogenous product factor, based on the aggregation function:
(7)
(7)
where Ht is the homogenous labour input, and φw the substitution elasticity between various types of labour services supplied
The FOC resulting from the cost minimisation of the aggregation firm is:
(8)
(8)
where Wt is the homogenous wage
Given the well known approach of wage stickiness à la Erceg et al. (Citation2000), in one period just a part of households make efforts to re-optimise their nominal wage (with a probability of 1-ξW) , as revealed by Equationequations (9)-(11).
Thus, if the households optimising their nominal wages at time t cannot re-optimise them for k periods, they shall solve the optimisation problem:
(9)
(9)
where δWrepresents the level of indexation of wagesunder the labour demand constraint:
(10)
(10)
resulting in the following FOC:
(11)
(11)
The remainder of households simply update their wages, based on the rule below:
(12)
(12)
There is a continuous series of identical monopolistic firms acting in the domestic tradable field, with a Cobb-Douglas production function:
(13)
(13)
where Yt(zH) is the production function for each type of tradable goods, Ht(zH)/Kt(zH) the labour force/capital demand function for each type of tradable goods, εaH,t the total productivity of the tradable field factors, and α the production elasticity in relation to the capital factorwith the various tradable goods being aggregated into homogenous goods:
(14)
(14)
where YtHis the production function for the homogenous tradable goods
The aggregation process implies the cost minimisation, resulting in:
(15)
(15)
where PtH is the price of homogenous tradable goods, and Pt(zH) the price of differentiated tradable goods
The firm acting in the tradable field intends, given the production function, to maximise its profit, this giving birth to the following FOCs:
FOC relating to Ht:
(16)
(16)
FOC relating to Kt:
(17)
(17)
where ςt is the nominal marginal cost of the said firms
Considering the realistic approach of price stickiness à la Calvo (Citation1983), in one period just a fraction of the firms of tradable goods re-optimise their prices (with a probability of 1-ξH), as revealed by Equationequations (18)-(20).
If the firms of tradable goods optimising their prices at time t cannot re-optimise them for k periods, they shall solve the optimisation problem:
(18)
(18)
where δHis the level of indexation of the prices of the tradable goods, and MCtH the real marginal cost of the firms of tradable goodsunder the demand constraint:
(19)
(19)
resulting in the following FOC:
(20)
(20)
The remainder of firms simply update their prices, according to the rule below:
(21)
(21)
There is also a continuous series of identical monopolistic firms acting in the domestic non-tradable field, behaving in the same way as the firms of tradable goods.
The state budget constraints imply the balancing of expenses destined for the governmental consumption of non-tradable goods, for transfers to households and for payments of debt-related interest rates, with the revenues to the state budget, considered herein, given estimation limitations related considerations, as being restricted to the value-added tax and to the state-contingent securities, as follows:
(22)
(22)
where Ptg is the price of the final goods destined for public consumption, Gt the public consumption level, Rt* the external debt-related interest rate, Bt the state-contingent securities, and τtc the value added tax
The monetary authority is assumed to behave according to the rule:
(23)
(23)
where Yt is the output of the entire economy,
the steady state level of it,
the steady state inflation level, and εtm a monetary policy shock
The following risk sharing condition in assumed:
(24)
(24)
where Qt is the real exchange rate, υ a constant, and * an element denoting the foreign equivalent of related variables
The next step consists of studying the equilibrium on the markets of inputs, tradable goods and non-tradable goods.
The domestic market of inputs comes to equilibrium when the aggregate demand for inputs of tradable and non-tradable goods equals the related aggregate supply:
(25)
(25)
(26)
(26)
The tradable goods produced domestically are destined for public and private consumption and investments, and for exportation:
(27)
(27)
The non-tradable goods produced domestically are destined for public and private consumption and investments, and for being used in distribution services.
(28)
(28)
where N element is the H equivalent for non-tradable goods, κ the weight of domestic raw tradable goods in the consumption basket of tradable goods, γc/γi the weight of tradable consumption/investment goods in the related aggregate goods, and PtR the price of homogenous raw tradable goods with PtT=PtR+ωXPtN, ω denoting the units of non-tradable distribution services.
The model includes 12 structural shocks, the same six for both the Romanian and Eurozone economy: the technological shock on tradable/non-tradable goods, the shock on labour time-related preferences, on value added tax, on governmental expenses and the monetary policy shock, all seen as the average between their steady state value and their previous period value:
(29)
(29)
where ξ= {εaH, εaN, ζh, τc, G, εm, ζh∗, εaH∗, εaN∗, τc∗, G*, εm∗}, with ηξ,t having a normal distribution of 0 mean and σξ2variance.
The selected equations are subject to log-linearisation, by Taylor series expansion around the steady state of their logarithm, obtaining a form adequate for implementation in the Dynare tool of the Matlab software.
3. Data
The model encompasses six identical variables for each of the above-mentioned two economies, analysed since 2000 to 2014.
The following observables, rendered by association with their implementation-specific notations, were used for estimation purposes: gross domestic product - gdp, consumption - c, export - x, gross domestic product deflator - pi_d, real wage - w, expressed as wage cost index and interest rate - r, analysed as 3 M ROBOR, for Romania, respectively gross domestic product - y_ze, consumption - c_ze, export - x_ze, gross domestic product deflator - pi_d_ze, real wage – w_ze, expressed as wage cost index, and interest rate - r_ze, used as 3 M EURIBOR, for the Eurozone
The main data source for Romania was the National Institute of Statistics, apart from the real wage and the interest rate, taken from Eurostat, respectively the National Bank of Romania, and for the Eurozone, The International Monetary Funds, the foreign interest rate being downloaded from the National Bank of France.
Several variables were rendered stationary and the steady state value for all of them was captured via the HP filter, called from Eviews 7.0.
4. Methodology and results
In order to estimate the DSGE model approached, we have decided to resort to the well known Bayesian techniques, specifically used for such class of models, usually recommended, on the one hand, considering their capacity of capturing a complex set of equations, unlike other methods, like, for instance, the Generalised method of moments (GMM), that can deal only with disparate equilibrium equations and, on the other hand, given their power to provide a more likely estimation, while avoiding common issues arising from the weak identification or non-identification of parameters (Hudea, Citation2012, Mickelsson, Citation2015).
Specifically, the Bayes theorem allows for the provision of posterior probabilities (probabilities associated with the values of parameters, subsequent to the data observation), by resorting to some prior probabilities (probabilities established before the data observation), in the context of the calibration of the remainder of parameters, the former acting as an updating mechanism based on the absorption of additional information (Hudea, Citation2015), as follows:
(30)
(30)
respectively
(31)
(31)
where
is the density of parameters conditioned by data and by the model structure, namely the posterior distribution,
, the common density of parameters and data conditioned by the model structure,
, the density of data conditioned by parameters and by the model structure,
, the density of parameters conditioned exclusively by the model structure, namely the prior distribution, and
, the marginal density of data conditioned by the model structure, with
By combining (30) and (31), given that the marginal density of data does not influence the value of parameters, we obtain Equationequations (32)(32)
(32) and Equation(33)
(33)
(33) :
(32)
(32)
(33)
(33)
where is the posterior kernel, and
, the likelihood function, with
The estimation procedure involves the computing of the log-likelihood (34) and the identification of the posterior log-kernel (35), the latter being maximised in relation to by resorting to a numerical optimisation routine, getting the mode of the posterior distribution and the related Hessian matrix.
(34)
(34)
(35)
(35)
where
is the set of observables,
the number of observables,
the expected value of
and
, the variance-covariance matrix of
For simulating the posterior distribution, a Markov Chain Monte Carlo (MCMC) method, namely the Metropolis-Hastings algorithm, a highly efficient instrument in the matter provided by the Dynare tool of Matlab software, is approached, this allowing for the generation of an initial distribution (while assuming the asymptotic normality of the posterior distribution), the mean being based on the previously determined mode and the variance on the Hessian matrix adjusted with a scaling factor (36), all iterations from that point on being based on the accumulation of information (37):
(36)
(36)
(37)
(37)
where
is the mode arising from the posterior kernel maximisation,
the Hessian matrix arising from the posterior kernel maximisation, given the mode
,
the scaling factor,
the variance-covariance matrix arising from the computation of the inverse of the Hessian matrix
,
the selected estimated parameter, and
the previously selected estimated parameter.
For all selected parameters the posterior kernel is determined (38), this one being used for identifying the acceptance rate (39) stating that a value higher than unity stands for the acceptance of the estimated parameter, while a value ranging between 0 and 1 for the acceptance of the same with the acceptance rate associated probability and its rejection with the difference up to unity, in module, of the acceptance rate associated probability:
(38)
(38)
(39)
(39)
Thus, we get sequences of possible estimations of parameters which, based on the above-mentioned restrictions, lead to the selection of the most relevant of them, generating a histogram of the selected values, the latter representing nothing else but the posterior distribution.
4.1. Calibration of parameters
As previously stated, just a part of the model parameters are estimated, prior probabilities being set for the same, the remainder of them being calibrated and considered as such all over the estimation process.
Going in line with the specific procedure, we calibrated several parameters given their steady state value, the characteristics of the studied economies or the information provided by the related literature.
Thus, the subjective discount factor - β received the value of 0.99, commonly used as such by Smets & Wouters (Citation2003), Adolfson et al. (Citation2008) and others, the differentiated labour force substitution elasticity for the two economies - φw and φw* being set to 3.00, as per Kolasa (Citation2009).
The size asymmetry between the same economies - n, was determined by computing the ratio of the related GDP averages.
Also, for both economies considered, the weight of tradable consumption goods in aggregate consumption goods - γc and γc*, as well as the weight of domestic raw tradable goods in the consumption basket of tradable goods - κ and κ*, were set as averages, based on real data, leading to a value of 0.84 for Romania and 0.39 for the Eurozone, for the first one, respectively 0.66 for Romania and 0.008 for the Eurozone, for the second one.
As regards the public debt, it was calibrated, for Romania, at 0.30, and for the Eurozone, at 0.60, according to the ceiling of the Maastricht Treaty.
The GDP of Romania and the Eurozone - y, and y* was set to one unit steady state value, while the weight of tradable/non-tradable goods - y(zH) and y*(zH)/y(zN) and y*(zN) in GDP was associated with the corresponding parts of the related unit value: 0.52 and 0.28, respectively 0.47 and 0.71.
The private consumption - c and c* and the Governmental expenses - g and g* were determined based on the output arising from Taylor series expansion.
As for the consumption tax - τc and τc*, we calibrated it at 0.197 for Romania, by resorting to its weighted average, given its fluctuations over time, of 0.18 since 1996, 0.22 since 1998, 0.19 since 2000 and 0.24 since 2010, and at 0.195 for the Eurozone, considering the average registered at the level of its economically powerful countries.
4.2. Setting of priors
As in the calibration case, the features specific to the studied economies, as well as the related field literature helped us in determining the prior distribution types and values. Considering the information arising from the well-known works of Smets & Wouters (Citation2003), Adolfson et al. (Citation2008) or Fernandez-Villaverde (Citation2009), we decided to use the Beta, Gamma and Normal distribution.
The Beta distribution, used for parameters taking values belonging to (0,1) interval, was assigned to the consumption habit persistence - θ and θ*, the inverse of the inter-temporal substitution elasticity - σ and σ*, the inverse of the labour elasticity as to its marginal disutility - σL and σL*, the production elasticity as to capital – α and α*, the price stickiness of tradable/non-tradable goods - ξH and ξH*/ξN and ξN*, the wage stickiness - ξw and ξw*, the price indexation of tradable/non-tradable goods - δH and δH*/δN and δN*, the wage indexation - δw and δw*, and the auto-regressive parameters - ρ and ρ* of the shocks hitting the related economies.
The Gamma distribution, mainly considered for parameters that may exceed 1, was allotted to the indexing factor of GDP - φy and φy*, inflation - φπ and φπ*, and exchange rate - φs, while the normal distribution was set for the remainder of parameters with zero mean and one unit variance.
Thus, the price stickiness was established at a higher level for Romania, of 0.70, than for the Eurozone, of 0.60, given previous results indicating the low power of price optimisation of the former compared to the latter. The same criterion was applied to the wage rigidity, the values being, instead, of 0.84 for Romania and 0.60 for the Eurozone, with a standard deviation constantly set at 0.10, in line with Kolasa (Citation2009) and Almeida (2009).
The price and wage indexation was set ex ante at an identical level for the analysed economies, of 0.50 for mean, as in Adolfson et al. (Citation2008), and 0.20 for standard deviation, according to Kolasa (Citation2009), while the indexing factor of GDP was allotted a mean of 0.50 and a standard deviation of 0.10, unlike the indexing factor of inflation and exchange rate, assigned to a mean of 2.00 and a standard deviation of 0.20.
Finally, the auto-regressive parameters of the model shocks received 0.60 for mean and 0.10 for standard deviation, as in Almeida (Citation2009).
4.3. Estimation results
The calibration and the setting of priors for the model parameters, necessary steps in order to lay the grounds of the Bayesian estimation, opened the road, once the observables introduced in Dynare (Matlab), for obtaining the posterior probabilities, accompanied by the mode and the confidence interval (5th and 95th percentiles) of the same, clearly described across the paper and rendered in brief, simply for centralisation purposes, in .
Table 1. Estimation results for the model parameters.
The model approached, encompassing 45 variables: 29 predetermined, 14 forward-looking and 12 static, as well as 12 structural shocks, was transposed into a specific Dynare code, run in Matlab 7.11.0., generating, via the MCMC type Metropolis-Hastings algorithm, 2000 initial iterations, subsequently reduced to 1000, with average acceptance rates/chain of 0.286, 0.276, 0.276, 0.320 and 0.282.
The output indicates, for the consumption habit persistence, a value of 0.42 for Romania and 0.54 for the Eurozone, both inferior to those set as priors. Such results reveal a rather changing behaviour, mainly as regards the Romanian consumers, easily influenced when making consumption decisions, attitude generated to a large extent by the effects of the last world economic crisis. Yet, given that the value obtained for the Eurozone, of 0.55, is closely related to the one determined for it by Smets & Wouters (Citation2003) before the crisis, we should consider other impacting factors as well.
Concerning the analysed elasticities, the risk aversion reaches 0.77 for Romania and 0.88 for the Eurozone, more than anticipated, suggesting a reserved position, adopted especially by the Eurozone citizens, justifiable given their previously rendered more conservative posture in consumption.
Going forward, we observe that the inverse of the labour elasticity as to its marginal disutility is considerably low compared with the literature in the field, particularly for the Eurozone, while the elasticity of production to capital is very close, at least for Romania, with a value of 0.33, to the one set in advance, of 0.32. Nevertheless, the Eurozone related parameter, of 0.24, has a value inferior to the one established as prior, this revealing a share of labour in production of more than 75%, a little higher in relation to its level of 70%, calibrated, for the Eurozone, by Smets & Wouters (Citation2003).
Another interesting issue of the estimation process is represented by the results related to the stickiness of prices and wages. Although a little lower than previously set, the wage stickiness is significant for Romania, amounting, according to this estimation, to 0.76 level due, on the one hand, to the desire of producers to minimise their production related costs, the wage side being considerably at their will from such perspective, mainly in employment restrictive times, and, on the other hand, to the large periods of time elapsed between two wage negotiations. The same applies for the Eurozone, with mention that the resulted value, of 0.81, higher than the Romanian one, is somehow over-dimensioned.
The tradable and non-tradable goods have in Romania a price stickiness of 0.54, respectively 0.81, therefore suggesting its incapacity to quickly adapt to economic changes, the Eurozone, with lower values, of 0.29, respectively 0.37, proving to be more flexible, such gap between a similar individual economy and the Eurozone economy being also captured by Kolasa (Citation2009).
As for the inflation persistence level, reflecting its projection on the next period prices and wages, we ascertain, as opposed to the unique distribution previously set for the two economies (0.50), an indubitably higher value for prices and lower value for wages for Romania compared to the Eurozone.
Concretely, the price indexation specific to the Romanian tradable and non-tradable goods, of 0.92, respectively 0.97, reveals the tendency of the related producers to translate to a large extent the prior inflation to the following period prices, while those associated with the Eurozone goods, of 0.34 and 0.65, similar on average to those obtained by Smets & Wouters (Citation2003), indicate the orientation of producers to an adjustment of the production related expenses rather than to a substantial increase of the final prices.
A visible difference is observed as concerns the wage indexation, the Eurozone, with a level of 0.97, obviously outclassing Romania, displaying a value slightly exceeding 0.58, which suggests an undesirable situation of the latter, its citizens being affected in their purchasing power both by the inflexibility of wages and by their incomplete adjustment.
The GDP and inflation indexing factors, amounting to 0.63 and 1.76 for Romania and to 0.70 and 1.73 for the Eurozone, as well as and the exchange rate indexing factor, of 2.07, are quite close not only to their related priors, of 0.50 and 2.00, respectively 2.00, but also for both economies considered.
Another analysed aspect regards the auto-regressive parameters of the 12 structural shocks of the model, six for each economy, which range, for Romania, between 0.66, for the governmental expenses, and 0.96, for the preferences on the provided labour hours, or 0.97, for the monetary policy, and for the Eurozone, between 0.53, for the technology of tradable goods, or, with a close value, of 0.54, for the governmental expenses, and 0.96, for the preferences as to the provided labour hours.
We ascertain, on one hand, the realism of such results, the occasional and rigid direction changing of the monetary policy and of the labour force preferences, as for the labour hours provided, being largely transposed into the future period , while the technological shifts relating to tradable goods or governmental expenses, more unpredictable by their nature, being taken over to the next period to a lesser extent and, on the other hand, the comparative results for the studied economies. Besides, the sub-unitary values of the shock auto-regressive parameters, reflecting unit root inexistence, suggest also specific incomplete anticipating power for the same situations.
Overall, the values arising from the estimation process, compliant with those revealed by the authors the papers of whom have been used as a reference basis, are highly justifiable, the ovservable economic evolution of the analysed target country revealing a changing consumption habit, a consistent persistence of inflation at the level of prices of tradable and non-tradable goods, an irregular population, the purchasing power of whom is characterised by a descending trend, the unpredictibility of different shocks and the difficulty of taking adequate measures, in due time, to take advantage of their positive effect or to avoid or, at least, diminish their negative impact.
5. Conclusions
The present paper aimed at dynamically analysing, over more than 14 years, the evolution of the Romanian economy in parallel with the Eurozone economy, by means of a small-size open economy DSGE model.
The model, grounded on the paper of Kolasa (Citation2009) and considering the new Keynesian related nominal factor stickiness for prices and wages, was subject to a Bayesian estimation, resulting in the display of the posterior probabilities for 43 parameters, 21 associated with each economy and a common one related to the exchange rate.
The estimation output indicated a quite low value of the level of consumption habit persistence, both for Romania and the Eurozone, therefore revealing the decisional instability of consumers, susceptible to be influenced to a large extent, mainly concerning Romanians, by economic, social, or political circumstances and measures.
The estimated risk aversion, rendered by the reverse of the inter-temporal substitution elasticity of consumption, comes to strengthen the above-mentioned idea, the individuals manifesting certain reserves and being somehow more conservative in consumption in the Eurozone.
The wage inflexibility, as expected, is quite high for the analysed economies, this being significantly due to the large periods of time elapsed between two consecutive wage renegotiations, as well as to the temptation of producers to avoid increases of their production costs.
Price stickiness, on the other hand, differentiates the two economies, suggesting the inability of the Romanian producers to adapt to the occurring economic changes as quickly as the Eurozone producers.
The inflation persistence level specific to prices indicates the tendency of the Romanian producers to transpose the prior inflation into the product prices set for the following period to a much larger extent than those belonging to the Eurozone, who are rather interested in adjusting their production costs in order to maintain or even increase the related profit.
As for the inflation persistence level specific to wages, Romania, for which the obtained value is significantly below the Eurozone one, is in a quite difficult position, its citizens dealing with a lowering purchasing power due to both stickiness of wages and incomplete adjustment of the same.
The autoregressive parameters of the model shocks, six for each studied economy, have sub-unitary values, even when considering the upper extreme of their confidence interval, therefore standing for the unit-root absence and reflecting the impossibility to fully anticipate such shocks or, in other words, the existence of the surprise element.
Taking a global look at the results obtained from the present analysis and observing their compliance, to a very large extent, with the realities manifested in the daily economic life of the target entity, as revealed across the study, despite some restrictions relating to the possibility of comparing the same with other results obtained, for the studied economy, from a DSGE perspective, we reserve the right to consider such model highly appropriate for its use in a further analysis. This conviction is strengthened by the parallelism featuring this model, the analysed economy being permanently related to a benchmark, this allowing for the identification of the gaps to be surmounted in order to achieve a higher level of development. Given that, the present study should be continued, based on the same modelling pattern, by focusing on the impulse-response function analysis, reflecting the reaction of the model variables to the considered shocks, as well as on the predictive analysis, revealing the possible future trajectory of such variables and opening the way for making pertinent economic decisions.
References
- Adolfson, M., Laséen, S., Lindé, J., & Villani, M. (2008). Evaluating An Estimated New Keynesian Small Open Economy Model. Journal of Economic Dynamics and Control, 32(8), 2690–2721. doi: 10.1016/j.jedc.2007.09.012
- Adolfson, M., Laséen, S., Christiano, L., Trabandt, M., & Walentin, K. (2013). RAMSES II. Model Description, Severiges Risksbank Occasional Paper 12.
- Almeida, V. (2009). Bayesian Estimation of a DSGE Model for the Portuguese Economy, Banco de Portugal Economics and Research Department Working Paper w200914.
- Almeida, V., Castro, C. M., Félix, R., & Maria, J. R. (2013). Fiscal Consolidation in a Small Euro-Area Economy. International Journal of Central Banking, 9(4), 1–38.
- Barthélemy, J., Clerc, L., & Marx, M. (2011). A two-pillar DSGE monetary policy model for the euro area. Economic Modelling, 28(3), 1303–1316. doi: 10.1016/j.econmod.2011.01.010
- Calvo, G. A. (1983). Staggered Prices in a Utility-maximizing Framework. Journal of Monetary Economics, 12(3), 383–398. doi: 10.1016/0304-3932(83)90060-0
- Çebi, C. (2012). The interaction between monetary and fiscal policies in Turkey: An estimated New Keynesian DSGE model. Economic Modelling, 29(4), 1258–1267. doi: 10.1016/j.econmod.2012.04.014
- Christiano, L. J., Trabandt, M., & Walentin, K. (2010). DSGE Models for Monetary Policy Analysis, National Bureau of Economic Research Working Paper 16074.
- Costantini, M., Gunter, U., & Kunst, R. M. (2017). Forecast Combinations in a DSGE-VAR Lab. Journal of Forecasting, 36(3), 305–324. doi: 10.1002/for.2427
- Erceg, C. J., Henderson, D. W., & Levin, A. T. (2000). Optimal Monetary Policy with Staggered Wage and Price Contracts. Journal of Monetary Economics, 46(2), 281–313. doi: 10.1016/S0304-3932(00)00028-3
- Fernandez-Villaverde, J. (2009). The Econometrics of DSGE Models. National Bureau of Economic Research Working Paper 14677.
- Hudea, O. S. (2012). Bayesian versus Maximum Likelihood Estimation in DSGE Modelling. CReBUS, 108–111.
- Hudea, O. S. (2015). Dynamic and Stochastic Modelling of the Romanian Economy in the Context of General Equilibrium, Pro Universitaria, Bucharest
- Knell, M. (2013). Nominal and real wage rigidities. In theory and in Europe. Journal of Macroeconomics, 36, 89–105. doi: 10.1016/j.jmacro.2013.01.006
- Kolasa, M. (2009). Structural heterogeneity or asymmetric shocks? Poland and the Euro Area through the lens of a two-country DSGE model. Economic Modelling, 26(6), 1245–1269. doi: 10.1016/j.econmod.2009.06.001
- Lees, K., Matheson, T., & Smith, C. (2011). Open economy forecasting with a DSGE-VAR: Head to head with the RBNZ published forecasts. International Journal of Forecasting, 27(2), 512–528. doi: 10.1016/j.ijforecast.2010.01.008
- Liu, P., & Mumtaz, H. (2011). Evolving macroeconomic dynamics in a small open economy: an estimated Markov-switching DSGE model for the UK. Journal of Money, Credit and Banking, 43(7), 1443–1474. doi: 10.1111/j.1538-4616.2011.00431.x
- Merola, R. (2015). The role of financial frictions during the crisis: An estimated DSGE model. Economic Modelling, 48, 70–82. (1), doi: 10.1016/j.econmod.2014.10.037
- Mickelsson, G. (2015). Estimation of DSGE models: Maximum Likelihood vs. Bayesian methods. Uppsala University Department of Economics Working Paper 20156
- Robinson, T. (2013). Estimating and Identifying Empirical BVAR-DSGE Models for Small Open Economies, Reserve Bank of Australia Research Discussion Paper 2013-06.
- Schorfheide, F. (2013). Estimation and evaluation of DSGE models: Progress and challenges. Advances in Economics and Econometrics, 51(3), 184–230.
- Sargent, T. J., & Surico, P. (2011). Two Illustrations of the Quantity Theory of Money: Breakdowns and Revivals. American Economic Review, 101(1), 109–128. doi: 10.1257/aer.101.1.109
- Slobodyan, S., & Wouters, R. (2012). Learning in a medium-scale DSGE model with expectations based on small forecasting models. American Economic Journal: Macroeconomics, 4(2), 65–10. doi: 10.1257/mac.4.2.65
- Smets, F., & Wouters, R. (2003). An Estimated Dynamic Stochastic General Equilibrium Model of the Euro Area. Journal of the European Economic Association, 1(5), 1123–1175. doi: 10.1162/154247603770383415
- Smets, F., Warne, A., & Wouters, R. (2014). Professional forecasters and real-time forecasting with a DSGE model. International Journal of Forecasting, 30(4), 981–995. doi: 10.1016/j.ijforecast.2014.03.018