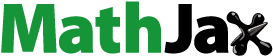
Abstract
This paper uses a direct measure of efficiency to examine the relationship between market structure and the performance of brokerage houses in Turkey. This methodology involves two stages: First, a stochastic translog cost frontier is specified to obtain a direct measure of efficiency. Second, this measure is included in a profitability equation along with market concentration and market share variables. These two equations are then estimated using quarterly data from 112 brokerage houses for the period 2008–2015. The results show that the efficiency of brokerage houses does not influence profitability when measured directly. Market share, on the other hand, is found to be one of the main determinants of profitability, providing support for the relative market power hypothesis.
1. Introduction
Numerous studies have investigated the factors influencing firm profitability. These studies have generally found a positive relationship between market concentration and performance. Two main hypotheses have been proposed to explain this relationship: the traditional ‘structure-conduct-performance (SCP) hypothesis’, which is based on market power, and the ‘efficient structure hypothesis’, which is based on efficiency.
Mason (Citation1939, Citation1949) and Bain (Citation1951, Citation1956) introduced the SCP hypothesis, also known as the ‘collusion hypothesis.’ A slightly different version of this hypothesis also exists, known as the ‘relative market power hypothesis’ (Shepherd, Citation1983, Citation1986). Demsetz (Citation1973, Citation1974) developed the efficient structure hypothesis in response to the traditional collusion hypothesis.
According to the SCP hypothesis, concentration reduces the cost of collusion among incumbent firms, securing supranormal profits (Smirlock, Citation1985). The efficient structure hypothesis, on the other hand, asserts that the observed correlation between profitability and concentration is spurious, as concentration is a result of a given firm’s degree of efficiency. Highly efficient firms increase their market share (and, in turn, their concentration), and become more profitable (Demsetz, Citation1974). Therefore, the relation between profitability and concentration is spurious, and profitability is, in fact, an outcome of efficiency.
The relative market power (RMP) hypothesis argues that firms with large market shares might use their power to determine prices and make profits. According to this hypothesis, such firms increase in their profits through high quality service and differentiated products with higher prices. Therefore, individual market share brings market power and market imperfections.
Studies testing the traditional market power hypothesis and the efficient structure hypothesis for the banking sector include Smirlock, Gilligan, and Marshall (Citation1984); Smirlock (Citation1985); Evanoff and Fortier (Citation1988); Molyneux (Citation1993); Lloyd-Williams, Molyneux, and Thornton (Citation1994); Altunbas and Molyneux (Citation1994); Goldberg and Rai (Citation1996); Berger and Humphrey (Citation1997); Maudos (Citation1998); Aguirre and Lee (Citation2001); Punt and van Rooij (Citation2003); Fernández de Guevara, Maudos, and Pérez (Citation2005); Jeon and Miller (Citation2005); Molyneux and Forbes (Citation1995); Casu and Girardone (Citation2006); Mahathanaseth and Tauer (Citation2012); Garza-Garcia (Citation2012); Trujillo‐Ponce (Citation2013); Mirzaei, Moore, and Liu (Citation2013); Bello and Isola (Citation2014); Chan, Koh, Zainir, and Yong (Citation2015); Talpur, Shah, Pathan, and Halepoto (Citation2016) and Hasan, Jackowicz, Kowalewski, and Kozłowski (Citation2017). Similarly, studies carried out for the Turkish financial sector include Denizer and Cilli (Citation1989); Molyneux (Citation1993); Okumuş (Citation2002); Günalp and Çelik (Citation2004); Çelik and Kaplan (Citation2007); Sayılgan and Yıldırım (Citation2009); Aydemir (Citation2013); Sekmen, Akkus, and Siklar (Citation2015) and Çelik, Kaplan, and Şahin (Citation2015).
Most banking studies have used market share as a proxy for efficiency in regressions. It is assumed that more efficient firms operate at lower cost, thereby capturing a greater market share. On the other hand, some economists have criticised the use of market share as a proxy for efficiency, arguing that the market share variable is likely to reflect not only the effects of efficiency, but also the effects of other variables (Berger, Citation1995).
In this paper, it is aimed to examine the relationship between market structure and profitability of brokerage houses in Turkey using a direct measure of efficiency. Although there is a vast amount of empirical literature that examines the efficiency and profitability of banking systems, there exist only a few empirical studies dealing with the efficiency and productivity of brokerage houses. To the best of our knowledge, there has been no previous work in the literature investigating empirically the profitability of brokerage houses. This subject is of great importance because many of the emerging markets have still protective measures in their financial markets although they are now faced with more pressures to reform their financial markets and remain competitive in the global economy. In Turkey, the number of brokerage houses has not increased since 1997 because the Capital Market Board (CMB) has not allowed the establishment of new brokerage houses; the only way to enter the market is to buy an existing brokerage house. This has constituted a legally enforced barrier to entry in the market. The results of this study, therefore, may provide us valuable insights into the impact of policy makers and regulators in emerging markets on the performance of financial institutions and the market when they engage in protectionist practices to limit competition.
The study utilizes the stochastic frontier approach to estimate a direct measure of efficiency of Turkish brokerage houses. More specifically, we estimate a translog cost frontier function to obtain efficiency scores of brokerage houses. These efficiency scores then enter into the profitability equation as an explanatory variable along with other independent variables. The results of the paper suggest that the relative market power hypothesis is strongly supported in the Turkish brokerage house sector when efficiency is measured directly. The brokerage houses with large market shares seem to use their market power to determine prices and make profits during the period under study. The structure of the paper is as follows: Section 2 provides background information on Turkish capital markets and the development of brokerage houses in the sector. Section 3 outlines the hypotheses on the relationship between market structure and performance. Section 4 describes the measurement of efficiency. The cost and profitability equations are specified in Section 5. Section 6 presents and discusses the estimation results. Finally, Section 7 concludes the paper.
2. An overview of the brokerage house sector in Turkey
Turkish financial markets are divided into three functional categories: insurance, capital market and banking. Generally, financial institutions in the capital market sector cannot operate in the banking and insurance markets or vice versa. Despite this segregation, universal banking takes place more and more within the system. Brokerage houses in Turkey have been operating since the establishment of the Istanbul Stock Exchange in 1986, and all brokerage houses are members of the exchange. The Istanbul Stock Exchange was converted from a public organisation to a private firm and renamed Borsa Istanbul with the amendment of the new Capital Market Law in 2013.
The Turkish equity market includes two main types of intermediaries: banks and brokerage houses. Banks are not permitted to trade shares on exchanges in the secondary market. Brokerage houses can engage in several activities such as intermediation for the trade of capital market instruments in the secondary market and the issue of public offerings of capital market instruments. Furthermore, brokerage houses may also conduct the repurchase and reverse repurchase of capital market instruments, investment consultancy and portfolio management. The function of intermediary institutions outlined in Turkish capital market regulations is similar to that of the United States, which broadly encompasses the operations of brokers, dealers, underwriters and investment bankers (Ünal, Citation2008).
According to Turkish capital market regulations, brokerage houses must be established as joint-stock companies, and their capital must be in the form of registered shares. This capital must be fully paid and not to be less than the amount determined by the CMB. In addition, its founders must not have been subject to any legal prosecution due to bankruptcy or other felony. The establishment of brokerage houses is contingent on the approval of the CMB (Bağdadioğlu, Dinçer, & Yereli, Citation2012). The CMB has not permitted the establishment of new brokerage houses since 1997. The only way to enter the market is to buy an existing brokerage house. It should also be noted that although more than 100 brokerage houses operate in the market, only 5 are listed on Borsa Istanbul.
In order to perform intermediation activities, brokerage houses have to obtain a certificate of activity from the CMB. shows the number of certificates obtained by brokerage houses in each category.
Table 1. Number of certificate of activity held by brokerage houses.
presents the development of the number of banks and brokerage houses in Turkey.
Table 2. Number of banks and brokerage houses in Turkey.
When the Borsa Istanbul was established in 1986, the number of brokerage houses was around 10. This number increased to 130 by 2001. It then dropped following the financial crisis of 2001, declining to 108 in 2005. The number of brokerage houses was 100 in 2011 and 95 in 2015. As seen in , the number of brokerage houses has not increased over the years because there has been an entry barrier to the market since 1997. shows the branch network of brokerage houses.
Table 3. Branch network of brokerage houses in Turkey.
Brokerage houses use three types of networks to reach customers: their own branches, representative offices and bank branches (bank-originated brokerage houses rely mainly on their related bank branches). The number of brokerage house branches reached 323 in 2015 compared to 242 branches in 2001, indicating an increase by 33%. The number of bank branches, on the other hand, has increased by 47% since 2001.
displays the development of total assets in the financial sector and the market capitalisation of companies that are listed on Borsa Istanbul. As seen in the table, the banking sector dominates the financial market. As brokerage houses mainly provide trading services in exchange for commission, most of their holdings are clients’ assets. It can also be observed that the market capitalisation and transaction volume has increased over time.
Table 4. Total assets of banks and brokerage houses, and equity market transaction volume and market capitalisation of Borsa Istanbul (Million TL).
The income of brokerage houses comes primarily from commission collected as a fraction of clients’ trading transactions. The regulatory authority, CMB, imposed a minimum commission rate of 0.2% in 1997. The brokerage houses were allowed to pay back 35% of the commission to their clients in 2002. The payback ratio was 50% in 2003 and 75% in 2004. The limitations on commission paybacks were lifted after 2006. The minimum limit of commission rates, which are taken at the time of transaction, however, is still in force and brokerage houses are allowed to pay back some part of the commission.
As seen in , the volume of transactions has increased steadily. Annual daily trading volume was 642.80 million TL as of end of 2001 and 8,052.84 million TL at the end of 2015, which is more than a 10-fold increase.
displays some selected financial indicators of brokerage houses.
Table 5. Financial indicators of brokerage houses.
It is observed in that the total assets of brokerage houses increased from 950 million TL in 2001 to 3,841 million TL in 2007 and to 15,312 million TL in 2015. The shareholders’ equity climbed at a similar rate during the same period, while return on equity (ROE) and return on asset (ROA) declined from 2001 to 2015. The current ratio has declined slightly since 2001. The ratio of shareholder equity to total assets was 60% in 2001 and 69% in 2003. This ratio dropped to 26% in 2015. reveals that the financial indicators of the brokerage houses did not deteriorate during the financial crisis in the banking sector in 2009 as much as they did in 2001. Short-term liabilities increased nearly 100% from 2001 to 2015. Throughout the period, the short-term debt-to-equity ratio increased while the ratio of long-term debt-to-equity decreased. Meanwhile, the profitability of brokerage houses decreased significantly.
3. The hypotheses on the relationship between market structure and performance
To test the collusion hypothesis against the efficient structure hypothesis, this study estimates two models given by EquationEquations (1)(1)
(1) and Equation(2)
(2)
(2) . The first model (Bağdadioğlu et al., Citation2012; Lloyd-Williams et al., Citation1994; Maudos, Citation1998; Molyneux & Forbes, Citation1995; Punt & van Rooij, Citation2003; Smirlock, Citation1985; Vander Vennet, Citation1993; Weiss, Citation1974) uses a market-share variable as a proxy for efficiency. The second model uses a direct measure of efficiency.
(1)
(1)
(2)
(2)
In both models, π represents the performance of brokerage house (with return on equity – ROE as a proxy). represents the market share of a given brokerage house, while
represents market concentration. X is a vector containing sector- or brokerage house-specific control variables. Finally,
is a direct efficiency measure obtained from the estimation of a stochastic cost frontier.
In the first model, if is positive and statistically significant
equals zero, then it can be concluded that the results give support to the SCP hypothesis. On the other hand, if
is positive and statistically significant while
equals zero, then the results confirm the efficient structure hypothesis. Based on the sign and significance of the coefficients in EquationEquation (2)
(2)
(2) , the results could support the SCP hypothesis, the efficient structure hypothesis, the relative market power hypothesis or the modified efficient structure hypothesis (Gumbau & Maudos, Citation2000; Maudos, Citation1998; Timme & Yang, Citation1991).
In the second model, if concentration is the only factor determining the performance of brokerage houses, then result traditional SCP hypothesis is supported. On the other hand, if profitability is explained only by the direct measure of efficiency, then efficient structure hypothesis is supported. The modified efficient structure hypothesis (Shepherd, Citation1986) asserts that profitability is explained by efficiency as well as by the residual influence of market share, whereas concentration has no direct influence on profitability. The reason for the latter is that market share captures the influence of factors such as product differentiation and/or market power, which are unrelated to efficiency.
In case of market share is the sole determinant of the profitability of a firm, and
equal zero,
is positive and statistically significant, then it can be concluded that the results give support to the relative market power hypothesis. The relative market power hypothesis states that firms having a high proportion of market share and effective product differentiation use their market power to determine their own products’ prices, therefore earning supernormal profits (Shepherd, Citation1983). The difference between the traditional SCP hypothesis and the relative market power hypothesis is that, according to the latter, the advantages gained from size may exist even in markets that are not concentrated.
Over the past 15 years, the number of studies testing these hypotheses for financial sectors by using a direct measure of efficiency has increased. Examples include Aguirre and Lee (Citation2001), Timme and Yang (Citation1991),Vander Vennet (Citation1993), Goldberg and Rai (Citation1996), Maudos (Citation1998), Gumbau and Maudos (Citation2000), Okumuş (Citation2002), Günalp and Çelik (Citation2004), Mensi and Zouari (Citation2010), Aygören and Yeşilyurt (Citation2011), Das and Drine (Citation2011), Ye, Xu, and Fang (Citation2012), Garza-Garcia (Citation2012), Çelik et al. (Citation2015), Zhao and Kang (Citation2015) and Alhassan, Tetteh, and Brobbey (Citation2016). Some of these and some other have formulated a profitability equation for the banking sector and include a direct measure of X-efficiency (inefficiency) as an independent variable (Alhassan et al., Citation2016; Ye et al., Citation2012). The studies of Goldberg and Rai (Citation1996), Maudos (Citation1998) and Günalp and Çelik (Citation2004) used the stochastic frontier approach for the measurement of efficiency (inefficiency), and they assumed a half-normal distribution for the inefficiency term. Meanwhile, many other efficiency studies of the banking sector, do not necessarily estimate a profitability equation. A summary of studies related to efficiency of financial institutions can be found in Bauer, Berger, and Humphrey (Citation1993), Berger and Humphrey (Citation1997) and Fethi and Pasiouras (Citation2010).
Studies investigating the efficiency of brokerage houses in Turkey as well as in other countries are scant. Using data envelopment analysis (DEA), Gündüz, Yılmaz, and Yılmaz (Citation2001) found that only half of the brokerage houses operating in the Turkish brokerage house sector in 1997 and 1998 were efficient. Aktaş and Kargın (Citation2007) analysed the efficiency of brokerage houses in Turkey by using DEA and found that efficiency declined over the period 2000–2005. Aygören and Yeşilyurt (Citation2011) found that the age of brokerage houses and the number of employees have positive impact on efficiency while other firm attributes such as the number of branches, firm size, financial leverage, and service ratio have a negative impact on the efficiency of brokerage houses. Bağdadioğlu et al. (Citation2012) used DEA to calculate efficiency during the period 2000–2008. They found that the Turkish brokerage house sector has very low efficiency scores and concluded that the main sources of inefficiency were managerial incompetence at the individual brokerage house level.
4. The measurement of efficiency
In this study, the term efficiency refers to the concept of X-efficiency, which includes both technical and allocative efficiencies. Technical efficiency means minimising the amount of inputs used to produce a given level of outputs or maximising the amount of outputs produced for a given amount of inputs. Allocative efficiency involves using the right combination of inputs to produce a given level of output at the lowest cost. Cost efficiency is achieved when both technical and allocative efficiencies are present. X-efficiency is directly linked to managerial skills for controlling costs. Therefore, X-efficiency includes both technical efficiency and allocative efficiency. If technical inefficiency is present, a firm operates under its production possibilities frontier, while in the presence of allocative inefficiency, even if the firm operates on the production possibilities frontier, it is not able to minimise its costs. In estimating X-efficiency, this study uses the stochastic frontier approach developed by Aigner, Lovell, and Schmidt (Citation1977) and Meeusen and van den Broeck (Citation1977). This approach involves formulating a stochastic cost frontier function in the following form:
(3)
(3)
Here, is the natural logarithm of the observed costs of firm
is the output vector and
is the vector of input prices. The compound error term,
consists of two components: a one-sided error term (
), and a symmetric, purely random component (
). The variable
reflects managerial and other inefficiencies that are within the firm’s control. The variable
on the other hand, reflects omitted variables, measurement error and stochastic elements beyond managerial control (Berger & Humphrey, Citation1997). The variable
is assumed to follow a half-normal distribution. It is the absolute value of a variable that is distributed as a normal with mean zero and variance
On the other hand,
is assumed to be drawn from a normal distribution with mean zero and variance
Thus,
cannot have a negative value, while
can have either a negative or a positive value.
Assuming that both components of the compound error term are distributed independently, the frontier function can be estimated by maximum likelihood. Inefficiency scores then could be derived from the residuals of the regression. The log-likelihood function of the model can be written as follows:
(4)
(4)
In EquationEquation (4)(4)
(4) ,
equals
while
equals to
In the same equation,
shows the number of firms, and
represents the standard normal cumulative distribution function. Individual inefficiency estimates can be computed using the distribution of the inefficiency term conditional on the estimate of the compound error term. Jondrow, Knox Lovell, Materov, and Schmidt (Citation1982) demonstrated that for the half-normal distribution, the mean of this conditional distribution is given by the following expression:
(5)
(5)
In EquationEquation (5)(5)
(5)
and
are the standard normal density and cumulative distribution functions, respectively. The values of the parameters in this equation can be obtained from the maximum likelihood estimation in EquationEquation (4)
(4)
(4) . Thus, it is possible to calculate an estimate for the expected value of
In other words, individual inefficiency estimates can be calculated using EquationEquation (5)
(5)
(5) . Our primary goal is to obtain the efficiency scores of brokerage houses that form the variable
in our profitability equation. Hence, the next task is to calculate efficiency scores using the translog cost function.
Based on the estimation of a particular functional form for the cost function, in EquationEquation (3)
(3)
(3) , cost efficiency is measured as the ratio between the minimum costs (
) necessary to produce the output vector and the costs actually incurred (
):
(6)
(6)
An efficiency value of implies that without any inefficiency, it would be possible for a brokerage house to produce the same vector of production, saving (
)*100 percent of the costs. The efficiency variable takes its maximum value for the best-practice brokerage house in the sample. Efficiencies of brokerage houses are expressed in raw scores, and no standardisation procedure is applied to them. Therefore, efficiency scores can take zero and positive values.
5. The specification of cost and profitability equations
This study uses a translog cost function to estimate the efficiency variable (). A translog cost function provides a local, second-order approximation in logarithms to any arbitrary twice-differentiable cost function.
Assuming that brokerage houses operating in Turkish capital markets produce one output by using three inputs, the translog cost function takes the following form:
(7)
(7)
In line with the intermediation approach, total costs () include both operational and non-operational costs while output is assumed to be trading volume. In banking studies, total costs are divided into two categories: interest expenses and non-interest expenses. However, the income statement of brokerage houses differs from that of banks. Brokerage houses trade securities for their own portfolio as well as for their customers. Therefore, the total costs of brokerage houses should include all expenses, namely, the cost of sales, operating expenses, expenses and losses from other operations, financial expenses, extraordinary expenses and losses. Here, the cost of sales and operating expenses are regarded as operating costs while other expenses are considered non-operating costs for brokerage houses. The definitions of all variables in our translog cost function are given below:
Following Aslan, Koksal, and Ocal (Citation2011), Aktaş and Kargın (Citation2007) and Bağdadioğlu et al. (Citation2012), the total trading volume is used to represent the output of brokerage houses. The brokerage houses are assumed to produce their output by using three inputs: labour, capital and short/long long-term financial debts (without provisions). The prices of these inputs are defined as follows:
=Price of Capital ((expenses and losses from other operations + extraordinary expenses and losses + amortisation expenses)/(tangible fixed assets)).
= Price of Financial Debt ((financial expenses/(total liabilities - provisions)).
The variables and
the foreign-owned and bank-owned brokerage house dummy variables are defined as follows:
=1 if majority of shareholders are foreigners
0 if majority of shareholders are nationals
=1 if brokerage house is a subsidiary of a bank
0 if brokerage house is not a subsidiary of a bank
Dummy variable is included in the model to control for the fact that the cost structures of bank-owned brokerage houses could be different because they can use all the branches of the owner banks at no cost as their own branches. Dummy variable
on the other hand, is added because the brokerage houses with foreign majority shareholders could gain a cost advantage by using their foreign owners’ established technological and institutional infrastructure in their home countries.
As will be explained in detail below, efficiency scores for brokerage houses can be obtained from the translog cost function (EquationEquation (7)(7)
(7) ) by using the stochastic frontier approach. In the estimation of our stochastic translog cost function, we will impose the usual symmetry and homogeneity conditions:
(symmetry condition)
(symmetry condition)
(homogeneity conditions)
(homogeneity conditions)
Cost and input prices are normalised by the price of labour () to impose linear homogeneity restrictions.
The extended version of the profitability equation (EquationEquation (2)(2)
(2) ), can be rewritten by explicitly specifying the control variables in vector X:
(8)
(8)
where
: Return on equity of the ith brokerage house (net income/total equity),
:Three-firm (brokerage house) concentration ratio based on total assets,
:Market share of the ith brokerage house in terms of total assets,
:Efficiency score of the ith brokerage house that is derived from the estimation of translog cost function,
:Ratio of equity to total assets ratio of the ith brokerage house (owner’s equity/total assets),
: Total assets of the ith brokerage house, and
: Growth rate of daily trading volume of Borsa Istanbul
This study uses to measure performance, which was proposed by Evanoff and Fortier (Citation1988) and is commonly used in empirical studies on financial institutions such as Punt and van Rooij (Citation2003), Mensi and Zouari (Citation2010), Aslan et al. (Citation2011), Garza-Garcia (Citation2012), Akbaş (Citation2012), Ye et al. (Citation2012) and Alhassan et al. (Citation2016). Because
is defined as net income divided by total equity, it is a comparable measure of performance for brokerage houses. The hypotheses on variables
and
and the theoretical rationale behind them have been explained in Section 3.
The ratio of capital to total assets () is introduced into the model in order to reflect the differences in risk levels of brokerage houses. The inclusion of this variable accounts for the fact that the dependent variable is not risk-adjusted. A lower
value reflects a higher risk since it implies a higher liability to total assets ratio. In other words, a significant portion of the assets of brokerage houses is financed by debt. A lower level of
may affect profitability in two ways: First, clients may not wish to work with a high-risk brokerage house. A brokerage house with high risk is likely to lose clients, which affects profitability negatively. Second, such a brokerage house would access to lines of credit under less favourable conditions. It may be subject to a higher interest rate due to a higher risk premium, implying greater financial expenses and lower profitability. Hence, the coefficient for this variable is expected to be positive. In other words, a higher
ratio leads to higher profitability.
The total assets variable is included in the model to control for potential size effects due to economies of scale. In addition, the services of large-scale brokerage houses are possibly more diversified, which implies lower risk. Their larger number of customers increases their trading volume. Therefore, the total assets () variable is expected to be positively associated with the dependent variable. However, because the correlation between total assets and market share variables is very high in our sample (0.907), as in the studies of Okumuş (Citation2002) and Günalp and Çelik (Citation2004), the inverse of total assets (
) is included instead of total assets in order to avoid a multicollinearity problem (the correlation between
and market share falls to -0.1447). After inversing total assets, the data becomes very small; therefore, the inverse of total assets variable (
) is expressed in millions. Needless to say, in contrast to the coefficient of
the coefficient of
is expected to be negative.
The final control variable in the model is the growth rate of Borsa Istanbul’s trading volume (). As Borsa Istanbul stock trading volume is generated by brokerage houses, it is expected that a high rate of growth will positively affect the profitability of brokerage houses.
6. Data and econometric results
This study utilises quarterly unbalanced panel data of Turkish brokerage houses for the period between 2008.Q1 and 2015.Q1. These data have been drawn from the website of The Association of Capital Market Intermediary Institutions of Turkey (TCMA). The TCMA publishes quarterly detailed balance sheet and income statement of brokerage houses that are prepared according to International Financial Reporting Standards. TCMA also publishes the number of employees, trading volume and personnel expenses. The sample is composed of 112 brokerage houses, of which 22 are subsidiary of a bank and 15 are mainly owned by foreigners in 20151.
gives the results of estimating the translog cost function (EquationEquation (7)(7)
(7) ). It is seen from that the output and capital elasticities are positive, as expected, while the financial debt elasticity is negative but insignificant. As for the dummy variables, the coefficient of B is unexpectedly negative, though it is statistically insignificant. The negative and highly significant coefficient on F implies, on the other hand, that foreign-owned brokerage houses have cost advantages over their domestic counterparts.
Table 6. Estimation results of translog cost function.
presents the yearly average efficiency scores for the sample period. As can be seen from the table, the average cost efficiency of the sector declines during 2008–2011 while it slightly increases during 2012–2015; overall, it decreases during the period under examination. The sharp decline in efficiency in 2010 is probably due to the global financial crisis of 2008–2009. The brokerage house sector began to recover gradually after 2011. However, the average efficiency of the sector remained below its pre-crisis level as of 2015.
Table 7. Efficiency scores (yearly averages).
presents the estimation results of the profitability equation. In column (A), we report the fixed-effects (FE) estimates of EquationEquation (8)(8)
(8) without a direct measure of efficiency, which is the most commonly used specification in the literature to test the hypotheses on the relationship between market power and performance. Column (B) shows the results of the same equation with a direct measure of efficiency (
). In order to check for the presence of endogeneity in our models, we apply the Durbin-Wu-Hausman test (Durbin, Citation1954; Hausman, Citation1978; Wu, Citation1973). For both models (with and without variable
), the test produces a chi-square value which is statistically significant at a critical value below 1%; hence, we reject the null hypothesis of exogeneity. Therefore, the equations are estimated also by the two-stage least squares (2SLS) fixed-effects model. For the 2SLS fixed-effects model, all of the explanatory variables are treated as endogenous, and their first differences are used as instruments (so that our equations are exactly identified). Columns (C) and (D) present 2SLS fixed-effects estimates of EquationEquation (8)
(8)
(8) . When there are multiple endogenous regressors, the Cragg and Donald (Citation1993) F-test can be used to assess whether the instruments used are weak. Stock and Yogo (Citation2005) provide critical values for this test that depend on the number of endogenous regressors, the number of instruments, and the desired maximal bias of the IV estimator relative to OLS. Given our number of endogenous regressors and the number of instruments, the Cragg-Donald F-test results suggest that our instruments are not weak in both models at the %5 level of significance (even when the desired maximal bias is set at the 5% level). Similar to FE estimations, model (D) is different from model (C) in that it involves a direct measure of efficiency (
). As explained above, the values of variable
are obtained from estimating the stochastic translog cost frontier specified in EquationEquation (7)
(7)
(7) .
Table 8. Estimation results of profitability equation.
The F statistics in show that all four models are, overall, highly significant. It is also seen that the FE estimations results are qualitatively similar to those obtained from the 2SLS FE estimations. Columns (A) and (C) include no direct measure of efficiency, and, as in traditional studies, the efficiency of brokerage houses is represented by the market share variable (). The model represented in these columns is comparable with earlier studies in the literature testing for the collusion and efficient structure hypotheses. It is seen that the coefficient of market share (
) is positive and highly significant while the coefficient of market concentration (
) is insignificant. Such a result has generally been interpreted as support of the efficient structure hypothesis because the markets share variable is considered to be a proxy for efficiency. As pointed out by Berger (Citation1995), however, if the coefficient of market share maintains its sign and significance when a direct measure of efficiency is introduced into the model, the market share variable should not be interpreted as a proxy of efficiency, but rather as capturing the effect of factors other than efficiency. Therefore, our results (estimates in columns (B) and (D) where there is a direct measure of efficiency and where the variable
still maintains its sign and significance) indicate that it is inappropriate to use the market share variable as a proxy for efficiency.
Estimates (B) and (D) include a direct measure of efficiency (). It is observed from these estimations that the coefficient of
is still highly significant and positive while the coefficients of both
and
are insignificant. These results show that among the four hypotheses, the relative market power hypothesis is supported for the brokerage house sector in Turkey. The efficient structure, the structure-conduct-performance and the modified efficient structure hypotheses, on the other hand, are rejected. A positive relationship between
and market share in Turkish banking sector was also found in the study of Akbaş (Citation2012).
Similar findings from the FE and the 2SLS FE estimations would suggest that the relative market power hypothesis is found a strong support for the Turkish brokerage house sector. Therefore, it seems that the brokerage houses with large market share might use their market power to determine prices and make profits. Although there are no other profitability studies on the Turkish brokerage sector, the findings of this paper could be compared to the results of studies carried out for the Turkish banking industry. For example, a study by Denizer and Cilli (Citation1989) found that over the period 1986–1988, the profitability of Turkish banks was explained mainly by the traditional structure-conduct-performance hypothesis. Similarly, Sekmen et al. (Citation2015) found that Turkish banking sector operates under conditions of monopolistic competition and Aydemir (Citation2013, Citation2014) states that Turkish banks appear to have exercised collusive pricing during 2000–2011. Denizer and Cilli (Citation1989) used market share of banks as a proxy for efficiency instead of measuring it directly. Molyneux (Citation1993), on the other hand, found insufficient evidence to support any of the theories in Turkish banking for the same period. A study by Günalp and Çelik (Citation2004) supported the efficient structure hypothesis for the period 1990–2000, suggesting that banks earn higher profits because their costs are lower. In other words, the most efficient banks yield greater profits and market shares. Consequently, the market becomes more concentrated.
The variable is found to have a positive and highly significant effect on profitability, and its parameter is positive and significant at the 1% significance level in all six estimates. As stated before,
is defined as capital divided by total assets. A high
value indicates that a given brokerage house is less risky. The positive coefficient of
indicates that clients prefer less risky brokerage houses, as expected. A similar relationship was found between the same variables for the Turkish banking sector in studies by Okumuş (Citation2002) and Günalp and Çelik (Citation2004).
The coefficient of the inverse of total assets, is negative and bears a statistical significance of 1%. The negative correlation between
and
implies a positive relationship between
and
This suggests that large brokerage houses are likely to benefit from economies of scale. Large brokerage houses could also be perceived as less risky because they are more likely to offer a more diversified variety of services. As explained above, low risk implies more clients. Studies on the Turkish banking sector yield mixed results regarding the sign and the significance of the coefficient of
Finally, the coefficient of the growth rate of Borsa Istanbul trading volume () is found to be unexpectedly insignificant. This result is surprising; one would expect a high growth rate to positively affect the profitability of brokerage houses given that the Borsa Istanbul stock trading volume is generated solely by brokerage houses.
7. Conclusion
This paper tests the hypotheses concerning the relationship between market structure and performance by using a direct measure of efficiency for the brokerage houses operating in Turkish capital markets. The direct measure of efficiency is calculated from the estimation of a stochastic translog cost function. The profitability equation of brokerage houses is specified with and without employing the direct measure of efficiency. Each equation is then estimated by both the fixed-effects and the 2SLS fixed effects models. The results from the regressions including the direct measure of efficiency indicate that market share is the main determinant of profitability while market concentration and efficiency are both insignificant. Therefore, the results give a strong support to the relative market power hypothesis for the Turkish brokerage sector.
Thus, the findings suggest that brokerage houses with large market share might use their market power to determine prices and make profits. This is not a surprising result considering the fact that the brokerage houses with large market shares in Turkey are able to earn significant amount of profits by engaging in and setting the prices of activities other than stock trading, such as intermediation for public offering, corporate finance, and investment consultancy. There is no doubt that the legally enforced barrier to entry that has been in force since 1997 has played an important role in this finding as it has led to an increase in the market shares of existing brokerage houses. Therefore, the regulatory authority should adopt policies to foster competition in the market, for example, by lowering the legal as well as the other barriers to entry or relying more on universal banking system in activities other than intermediation for trading in securities.
Note
Disclosure statement
No potential conflict of interest was reported by the authors.
Notes
1 Due to data availability, our sample consists of 84 brokerage houses out of 104 in 2008, 87 brokerage houses out of 103 in 2009, 89 brokerage houses out of 103 in 2010, 97 brokerage houses out of 100 in 2011, 94 brokerage houses out of 100 in 2012, 95 brokerage houses out of 100 in 2013, 90 brokerage houses out of 97 in 2014, 90 brokerage houses out of 97 in 2014 and 83 brokerage houses out of 95 in 2014. The data for 59 brokerage houses is available in all quarters from 2008 to 2015.
References
- Aguirre, M. S., & Lee, T. K. (2001). A reevaluation of the market structure performance relationship for banks under different regimes. Paper presented at the Asociación Argentina De Economía Política Xxxvi Annual Meeting. Retrieved from http://citeseerx.ist.psu.edu/viewdoc/download?doi=10.1.1.474.2305&rep=rep1&type=pdf.
- Aigner, D., Lovell, C. A. K., & Schmidt, P. (1977). Formulation and estimation of Stochastic frontier production function models. Journal of Econometrics, 6(1), 21–37. doi: 10.1016/0304-4076(77)90052-5
- Akbaş, H. E. (2012). Determinants of bank profitability: An investigation on Turkish banking sector. ÖNERİ: Marmara Üniversitesi Sosyal Bilimler Enstitüsü Dergisi, 10(37), 103–110.
- Aktaş, H., & Kargın, M. (2007). Türk sermaye piyasasindaki araci kurumlarin etkinlik ve verimliliği. Iktisat Isletme ve Finans, 22(258), 97–117. doi: 10.3848/iif.2007.258.9844
- Alhassan, A. L., Tetteh, M. L., & Brobbey, F. O. (2016). Market power, efficiency and bank profitability: Evidence from Ghana. Economic Change and Restructuring, 49(1), 71–93. doi: 10.1007/s10644-015-9174-6
- Altunbas, Y., & Molyneux, P. (1994). The concentration-performance relationship in European banking: A note. University College of North Wales, Institute of European Finance.
- Aslan, A., Koksal, K., & Ocal, O. (2011). Competitive environment hypothesis in Turkish banking system. International Journal of Economics and Financial Issues, 1(2), 74–77.
- Aydemir, R. (2013). Competition and collusion in the Turkish banking industry. Emerging Markets Finance and Trade, 49(sup5), 31–40. doi: 10.2753/REE1540-496X4905S502
- Aydemir, R. (2014). Empirical analysis of collusive behaviour in the Turkish deposits market. Ekonomska Istraživanja, 27(1), 527–538. doi: 10.1080/1331677X.2014.970452
- Aygören, H., & Yeşilyurt, M. E. (2011). Impact of firm attributes on the efficiency of brokerage houses. BDDK Bankacılık ve Finansal Piyasalar Dergisi, 5(2), 159–181.
- Bağdadioğlu, N., Dinçer, M. R., & Yereli, A. B. (2012). Efficiency and productivity of brokerage houses in Turkey. In T. Çetin, & F. Oguz (Eds.). Regulation and competition in the Turkish banking and financial markets (pp. 213–224). Hauppauge, New York: Nova Science Publishers.
- Bain, J. S. (1951). Relation of profit rate to industry concentration: American manufacturing, 1936–1940. The Quarterly Journal of Economics, 65(3), 293–324. doi: 10.2307/1882217
- Bain, J. S. (1956). Barriers to new competition: Their character and consequences in manu/acturing industries. Cambridge, MA: Harvard University Press. doi: 10.4159/harvard.9780674188037
- Bauer, P. W., Berger, A. N., & Humphrey, D. B. (1993). Efficiency and productivity growth in us banking. In H. O. Fried, S. S. Schmidt, & C. A. K. Lovell (Eds.), The measurement of productive efficiency: Techniques and applications (pp. 386–413). Oxford, UK: Oxford University Press.
- Bello, M., & Isola, W. A. (2014). Empirical analysis of structure-conduct-performance paradigm on Nigerian banking industry. The Empirical Econometrics and Quantitative Economics Letters, 3(3), 24–34.
- Berger, A. N. (1995). The profit-structure relationship in banking–tests of market-power and efficient-structure hypotheses. Journal of Money, Credit and Banking, 27(2), 404–431. doi: 10.2307/2077876
- Berger, A. N., & Humphrey, D. B. (1997). Efficiency of financial institutions: International survey and directions for future research. European Journal of Operational Research, 98(2), 175–212. doi: 10.1016/S0377-2217(96)00342-6
- Casu, B., & Girardone, C. (2006). Bank competition, concentration and efficiency in the single European market. The Manchester School, 74(4), 441–468.
- Chan, S.-G., Koh, E. H. Y., Zainir, F., & Yong, C.-C. (2015). Market structure, institutional framework and bank efficiency in Asean 5. Journal of Economics and Business, 82, 84–112. doi: 10.1016/j.jeconbus.2015.07.002
- Cragg, J. G., & Donald, S. G. (1993). Testing identifiability and specification in instrumental variable models. Econometric Theory, 9(2), 222–240. doi: 10.1017/S0266466600007519
- Çelik, T., & Kaplan, M. (2007). Türk sigortacilik sektöründe rekabet:2002–2004. Iktisat Isletme ve Finans, 22(251), 50–62. doi: 10.3848/iif.2007.251.9048
- Çelik, T., Kaplan, M., & Şahin, F. (2015). Efficiency, concentration and competition in the Turkish banking sector. Iktisat Isletme ve Finans, 30(346), 81–104. doi: 10.3848/iif.2015.346.4281
- Das, S. K., & Drine, I. (2011). Financial liberalization and banking sector efficiency in India: A Fourier flexible functional form and Stochastic frontier approach. International Business and Management, 2(1), 42–58.
- Demsetz, H. (1973). Industry structure, market rivalry, and public policy. The Journal of Law and Economics, 16(1), 1–9. doi: 10.1086/466752
- Demsetz, H. (1974). Two systems of belief about monopoly. In H. J. Goldschmid, H. M. Mann, & W. L. Weber (Eds.), Industrial concentration: The new learning (pp. 164–174). Boston: Little, Brown & Company.
- Denizer, C., & Cilli, H. (1989). Market structure–performance relationship in Turkish Banking system. Staff Paper. Ankara: The Central Bank of the Republic of Turkey.
- Durbin, J. (1954). Errors in variables. Revue de L'Institut International de Statistique/Review of the International Statistical Institute, 22(1/3), 23–32. doi: 10.2307/1401917
- Evanoff, D. D., & Fortier, D. L. (1988). Reevaluation of the structure-conduct-performance paradigm in banking. Journal of Financial Services Research, 1(3), 277–294. doi: 10.1007/BF00114854
- Fernández de Guevara, J., Maudos, J., & Pérez, F. (2005). Market power in European banking sectors. Journal of Financial Services Research, 27(2), 109–137. doi: 10.1007/s10693-005-6665-z
- Fethi, M. D., & Pasiouras, F. (2010). Assessing bank efficiency and performance with operational research and artificial intelligence techniques: A survey. European Journal of Operational Research, 204(2), 189–198. doi: 10.1016/j.ejor.2009.08.003
- Garza-Garcia, J. G. (2012). Does market power influence bank profits in Mexico? A study on market power and efficiency. Applied Financial Economics, 22(1), 21–32. doi: 10.1080/09603107.2011.595681
- Goldberg, L. G., & Rai, A. (1996). The structure-performance relationship for European banking. Journal of Banking & Finance, 20(4), 745–771. doi: 10.1016/0378-4266(95)00021-6
- Gumbau, M., & Maudos, J. (2000). Profitability, market structure and efficiency: An application to the Spanish industry. Ivie Working Papers. Valencia. Instituto Valenciano de Investigaciones Económicas.
- Günalp, B., & Çelik, T. (2004). Türk bankacilik sektöründe piyasa yapisi ve performans ilişkilerinin etkinlik için doğrudan bir ölçüt kullanilarak test edilmesi. Gazi Üniversitesi İktisadi ve İdari Bilimler Fakültesi Dergisi, 6(3), 1–27.
- Gündüz, L., Yılmaz, C., & Yılmaz, M. K. (2001). Türkiye’deki araci kurumlarin performans analizi (1993-1998): kantitatif bir değerlendirme. Ekonomi ve Yönetim Bilimleri Dergisi, 1(3), 38–53.
- Hasan, I., Jackowicz, K., Kowalewski, O., & Kozłowski, Ł. (2017). Do local banking market structures matter for SME financing and performance?: New evidence from an emerging economy. Journal of Banking & Finance, 79, 142–158. doi: 10.1016/j.jbankfin.2017.03.009
- Hausman, J. A. (1978). Specification tests in econometrics. Econometrica, 46(6), 1251–1271. doi: 10.2307/1913827
- Jeon, Y., & Miller, S. M. (2005). Bank performance: Market power or efficient structure? Working Paper Series. Connecticut. University of Connecticut.
- Jondrow, J., Knox Lovell, C. A., Materov, I. S., & Schmidt, P. (1982). On the estimation of technical inefficiency in the Stochastic frontier production function model. Journal of Econometrics, 19(2-3), 233–238. doi: 10.1016/0304-4076(82)90004-5
- Lloyd-Williams, D. M., Molyneux, P., & Thornton, J. (1994). Market structure and performance in Spanish banking. Journal of Banking & Finance, 18(3), 433–443. doi: 10.1016/0378-4266(94)90002-7
- Mahathanaseth, I., & Tauer, L. W. (2012). Market-power versus cost-efficiency in Thailand's banking sector in the post-crisis period (1998–2011). Journal of Asian Economics, 23(5), 499–506. doi: 10.1016/j.asieco.2012.06.002
- Mason, E. S. (1939). Price and production policies of large-scale enterprise. The American Economic Review, 29(1), 61–74.
- Mason, E. S. (1949). The current status of the monopoly problem in the United States. Harvard Law Review, 62(8), 1265. doi: 10.2307/1336466
- Maudos, J. (1998). Market structure and performance in Spanish banking using a direct measure of efficiency. Applied Financial Economics, 8(2), 191–200. doi: 10.1080/096031098333177
- Meeusen, W., & van den Broeck, J. (1977). Efficiency estimation from Cobb-Douglas production functions with composed error. International Economic Review, 18(2), 435–444. doi: 10.2307/2525757
- Mensi, S., & Zouari, A. (2010). Efficient structure versus market power: Theories and empirical evidence. International Journal of Economics and Finance, 2(4), 151–166. doi: 10.5539/ijef.v2n4p151
- Mirzaei, A., Moore, T., & Liu, G. (2013). Does market structure matter on banks’ profitability and stability?: Emerging vs. advanced economies. Journal of Banking & Finance, 37(8), 2920–2937. doi: 10.1016/j.jbankfin.2013.04.031
- Molyneux, P. (1993). Market structure and profitability in European Banking: University College of North Wales. Institute of European Finance.
- Molyneux, P., & Forbes, W. (1995). Market structure and performance in European banking. Applied Economics, 27(2), 155–159. doi: 10.1080/00036849500000018
- Okumuş, H. Ş. (2002). Market structure and efficiency as determinants of profitability in the Turkish banking industry. Yapi Kredi Economic Review, 13(1), 65–88.
- Punt, L. W., & van Rooij, M. C. J. (2003). The profit-structure relationship and mergers in the European banking industry: An empirical assessment. Kredit Und Kapital, 36(1), 1–29.
- Sayılgan, G., & Yıldırım, O. (2009). Determinants of profitability in Turkish banking sector: 2002–2007. International Research Journal of Finance and Economics, 28, 207–214.
- Sekmen, T., Akkus, O., & Siklar, I. (2015). Competitive conditions in the Turkish banking systems. Journal of Business Economics and Finance, 4(1), 52–68. doi: 10.17261/Pressacademia.201519950
- Shepherd, W. G. (1983). Economies of scale and monopoly profits. In J. V. Craven (Ed.), Industrial Organization, Antitrust, and Public Policy (pp. 165–204). Dordrecht: Springer Netherlands.
- Shepherd, W. G. (1986). Tobin's Q and the structure-performance relationship: Comment. American Economic Review, 76(5), 1205–1210.
- Smirlock, M. (1985). Evidence on the (Non) relationship between concentration and profitability in banking. Journal of Money, Credit and Banking, 17(1), 69–83. doi: 10.2307/1992507
- Smirlock, M., Gilligan, T., & Marshall, W. (1984). Tobin's Q and the structure-performance relationship. American Economic Review, 74(5), 1051–1060.
- Stock, J., & Yogo, M. (2005). Asymptotic distributions of instrumental variables statistics with many instruments. In D. W. K. Andrews (Ed.), Identification and Inference for Econometric Models (pp. 109–120). New York. Cambridge University Press.
- Talpur, A. B., Shah, P., Pathan, P. A., & Halepoto, J. A. (2016). Structure conduct performance (scp) paradigm in pakistan banking sector: a conceptual framework and performance of the first woman bank under SCP model. The Women-Annual Research Journal of Gender Studies, 8(8), 83–100.
- Timme, S. G., & Yang, W. K. (1991). On the use of a direct measure of efficiency in testing structure-performance relationships in US commercial banking. Georgia State University.
- Trujillo‐Ponce, A. (2013). What determines the profitability of banks? Evidence from Spain. Accounting & Finance, 53(2), 561–586. doi: 10.1111/j.1467-629X.2011.00466.x
- Ünal, S. (2008). Sigorta şirketlerinde özkaynak maliyetinin belirlenmesinde kullanilan yöntemlerin karşilaştirilması. Eskişehir Osmangazi Üniversitesi İktisadi ve İdari Bilimler Fakültesi Dergisi, 3(2), 129–141.
- Vander Vennet, R. (1993). Cost Characteristics of Credit Institutions in the Ec. Paper presented at the 20th Annual Meeting of the European Finance Association, Copenhagen.
- Weiss, L. W. (1974). The concentration-profits relationship and antitrust. In H. J. Goldschmid, H. M. Mann, J. F. Weston, & C. U. S. O. Law (Eds.), Industrial concentration: The new learning (pp. 201–220). Boston: Little, Brown.
- Wu, D.-M. (1973). Alternative tests of independence between Stochastic regressors and disturbances. Econometrica, 41(4), 733–750. doi: 10.2307/1914093
- Ye, Q., Xu, Z., & Fang, D. (2012). Market structure, performance, and efficiency of the Chinese banking sector. Economic Change and Restructuring, 45(4), 337–358. doi: 10.1007/s10644-012-9123-6
- Zhao, H., & Kang, S. (2015). Cost efficiency of Chinese commercial banks. International Journal of Finance and Accounting, 4(3), 180–186. doi: 10.5923/j.ijfa.20150403.05