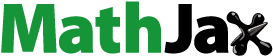
Abstract
This study investigates the causal link between short-term capital flow and interest rate differential with the bootstrap Granger full-sample causality and sub-sample rolling-window methods. The empirical results indicate that there is a unidirectional link from interest rate differential to short-term capital flow. However, when considering structural breaks in the above series, the full-sample causality test cannot be relied on. Hence, the time-varying rolling-window method is employed to examine the dynamic causal nexus. Being influenced by the U.S. monetary, world economic situation, Chinese intervention policies and other factors, interest rate differential has influences on short-term capital flow in some periods, but the reverse effect does not exist. Therefore, it is important for authorities to focus on specific backgrounds (e.g., economic situation, monetary policies) and further utilise interest rate and capital control to alleviate negative effects from violent fluctuation of short-term capital flow on the Chinese economy.
1. Introduction
Against the background of globalisation and internationalisation, short-term capital flow (SCF) would cross borders to pursue arbitrage opportunities in the flourishing international capital market (Wang, Hwang, & Chung, Citation2016). Modest movement of capital is beneficial because it achieves efficient resources allocation, which would raise productivity and economic growth. But the large and volatile capital flows can also create economic distortions and policy changes (Ahmed & Zlate, Citation2014). During the global financial crisis that broke out in 2008, developed economies implemented expansionary monetary policies which led to interest rate cuts towards the zero mark (Acharya & Bengui, Citation2018). Conversely, the policy responses of emerging economies were more subdued owing to fewer influences from the crisis. Consequently, there were obvious interest rate differentials (IRD) between developed and less-developed countries, which resulted in capital inflow surges (Makhetha-Kosi, Mishi, & Ngonyama, Citation2016). However, when the U.S. economy faced recovery and the Federal Reserve quit quantitative easing policies, emerging markets witnessed a slowdown in capital inflows and, in the worst cases, reversal of capital (Zoega, Citation2016). The huge fluctuation of SCF has raised a series of problems among inflow countries. Domestic financial system stability suffers shocks from excessive credit creation and asset price bubbles (Wang et al., Citation2016). Large capital inflows may cause currency appreciation, which finally influences export and growth performance (Ning & Zhang, Citation2018). Besides, the huge fluctuation of capital flows can complicate the pursuit of appropriate macroeconomic policies to maintain solid economic growth without rising inflation. When authorities raise interest rate levels in an effort to prevent overheating, the measure may encourage further capital inflows and boost currency appreciation pressures. Conversely, if they slow the pace of monetary tightening to deter inflows, the ability to follow appropriate monetary policies is compromised (Ahmed & Zlate, Citation2014).
China is the second largest economy in the world; it not only receives economic shocks from major countries passively, but its development also exerts increasingly important influences on other economies (Ning & Zhang, Citation2018). Short-term capital inflow increased dramatically at the beginning of 2003, due to the steady growth of China’s economy and expectation of renminbi (RMB) appreciation. In July 2005, China launched exchange reform and the RMB price returned to its normal track; the SCF was in a net outflow state from 2005 to 2007. Being influenced by U.S. quantitative easing polices, China faced rapid short-term capital inflows during the global financial crisis in 2008. Since 2013, China has faced short-term capital outflows again, which is mainly attributed to U.S. economic recovery, quitting quantitative policies, and falling domestic asset prices. The fluctuation of China’s SCF has become an unneglectable factor that affects its economic development and stability, which would bring higher requirements and serious challenges to macroeconomic policies (Li, Su, Chang, & Ma, Citation2018). To keep economic stability, reduce negative influences from SCF, and continue the momentum of rapid development, the People’s Bank of China (PBOC), Chinese central bank, has implemented a series of monetary policies, including interest rate adjustment. However, the pronounced IRD still exists when compared with the U.S., Japan and the eurozone. Glick and Hutchison (Citation2009) further indicate that in recent years China has implemented a moderate interest policy to reduce the fluctuation of SCF, which has proven ineffective. Consequently, there is imminent concern regarding how the interest rate policy would bring substantial SCF for China. According to Obstfeld (Citation2011), international capital would flow when facing a constant domestic–foreign interest differential. In response to this, we are greatly motivated to pursue an investigation related to the relationship between IRD and SCF in China. We also provide suggestions for preventing significant fluctuation of SCF. The moderate spread of IRD is critical to SCF’s stability and economic development.
Depending on the time-varying rolling-window approach, we aim to recheck the causal relationship between IRD and SCF, and provide convincing policy implications. The previous literature, in general, only considers full-sample causality and is susceptible to misleading conclusions in the presence of parameter instability from structural changes. China experienced obvious economic reconstruction and structural changes from 2006 to 2016. Overholt (Citation2010) indicates that prior to the global financial crisis in 2008, China underwent a paradoxical combination of rapidly rising inflation and spreading bankruptcies. Ho, Shi, and Zhang (Citation2017) argue that Chinese variables, including stock market, exchange rate and interest rate, are sensitive to U.S. shocks such as the quantitative easing policy in 2008, 2010, 2011 and 2012 separately. All have led to structural changes in domestic interest rate and SCF, which indicates that such dynamic linkage existing among the two series would clearly display instability varying across sub-samples. We have utilised a bootstrap Granger full-sample causality test and sub-sample rolling-window estimation to revisit the relationship between IRD and SCF. The bootstrap rolling-window method is different from previous methods, which cannot distinguish full-sample and sub-sample relationship changes over time. Empirical studies verify that causality between two time series may suffer from inaccurate results when the underlying full-sample time series have structural changes (Balcilar & Ozdemir, Citation2013). This can be addressed by allowing the casual relationship between the two series to be time-varying instead of using full-sample data that assume the single causality holds in every time period. The time-varying character that may exist in the causal link between IRD and SCF has been taken fully into consideration in this paper. The test for causality on the full sample is under the assumption that the causal relationship is fixed. We test for causality on the rolling sample, which captures structural changes in the model and the evolution of causality between sub-periods. The empirical results show that there is a unidirectional influence from IRD on SCF in some periods. When external and internal shocks are suffered, IRD cannot fully explain the reasons for SCF. Therefore, it is critical for policymakers to pay attention to specific backgrounds (e.g., economic situation, monetary policies) and further make use of interest rate and fiscal policies to reduce negative influences from SCF on the Chinese economy.
This paper proceeds as follows. Section 2 reviews the literature. Section 3 presents the theoretical model. Section 4 provides the methodology. Section 5 describes the corresponding data. Section 6 presents the empirical results and policy implications. Section 7 concludes.
2. Literature review
Substantial studies have been undertaken to focus on the relationship between IRD and SCF over the past century. Meade (Citation1951) indicates that the changes in interest rates will bring capital flows under the assumption that price and income are stable. Grubel (Citation1968) shows that the level of interest rate differential may not matter for capital flows, with capital able to flow even though the differential is zero or negative. Orlowski (Citation1999) proves international interest rates have been falling since 1994, which promotes capital allocations in central European countries to emerging market economies by international capital investors. Verma and Prakash (Citation2011) show that capital flows are not sensitive to interest rate changes as they are primarily determined by growth prospects of the Indian economy and returns on equities, respectively. Aizenman and Kendall (Citation2012) find that interest rate plays a major role in large flows of capital across borders of emerging market economies. Ahmed and Zlate (Citation2014) show that during the financial crisis, the developed countries implemented a low interest rate policy which generated excessive liquidity that pushed capital flows into emerging countries. Nier, Sedik, and Mondino (Citation2014) indicate that a high interest rate appears to work to stem capital outflow, but it may damage the domestic economy. Globan (Citation2015) proposes a higher interest rate in the eurozone vis-à-vis the host economies should negatively affect capital flows to non-eurozone European Union new member states. Carvalho and Fidora (Citation2015) prove that foreign capital inflow would lower long-term interest rate. Makhetha-Kosi et al. (Citation2016) argue that the capital flows and interest rate differentials can affect each other, and the latter especially shows persistent influences on capital flow during stress periods. Barroso, Da Silva, and Sales (Citation2016) analyse the short-term international capital flows of Brazil and find that U.S. quantitative easing monetary policy triggers capital inflow. Ayanou (Citation2016) finds evidence that foreign capital flows are significantly and negatively related to U.S. 30-year mortgage interest rates. Arteta, Kose, Stocker, and Taskin (Citation2018) indicate that central banks, including the European Central Bank and the Bank of Japan, have employed negative interest rate policies to respond to currency appreciation and capital inflow pressures. Daǧlaroǧlu, Demirel, and Mahmud (Citation2018) argue that quantitative easing policies from advanced economies ignite short-term capital flows into the emerging markets in pursuit of higher yields. Korinek (Citation2018) demonstrates that when U.S. interest rates rise, the emerging economies face abrupt capital outflow.
In the context of globalisation and internationalisation, China has become a key destination for SCF. The fluctuation of China’s short-term international capital flows has become an unneglectable factor that affects economic development and stability and will bring higher requirements and serious challenges to its macroeconomic policies. In consequence, China has gradually started paying attention to the resulting effects from volatile SCF on its economy. Li, Liu, and Ge (Citation2012) further estimate the scale of SCF in China and indicate that massive inflows create inflation and asset price bubbles. Lv and Xu (Citation2012) indicate that SCF in China is largely interpreted by fluctuations of its own, and the rest can be explained by exchange rate arbitrage, interest rate, stock price and housing price. Zhang and Tan (Citation2013) argue that raising the domestic interest rate would bring an expectation of RMB depreciation and restrain asset prices, which would further result in capital outflows. Wang et al. (Citation2016) indicate that in response to the wide interest rate spreads, considerable international capital has flown into China to make arbitrage profits. Cheung, Steinkamp, and Westermann (Citation2016) point out that in the post-crisis period, China’s short-term international capital flows were affected by the U.S. quantitative easing policy, as well as exchange rate fluctuation, capital control, and trade friction. Cai, Dang, and Lai (Citation2016) prove that there is a cointegration relation among macroeconomic condition, IRD and SCF in China. Chen, Yao, Zheng, and Ou (Citation2017) indicate that the nexus between IRD and SCF would be influenced by risk premium. When facing enough risk premium, the higher domestic interest rate leads to capital inflows. Ma (Citation2018) holds that due to the more vulnerable financial system and higher risk premium, interest rate does not have any significant influence on international capital flow.
3. Theoretical model
In the 1960s Mundell (Citation1960) and Fleming (1962), analysing SCF under different exchange rate regimes, built the IS-LM-BP frame system. This is called the Mundell–Fleming model, which assumes capital flow is a function of domestic and international spread if two countries have IRD, which can result in international capital flowing into a higher interest rate country. We use the two-country model to analyse the relationship between IRD and SCF. We choose A and B to present domestic and foreign countries separately. presents the SCF from B to A. IA presents the capital flow without influence from IRD. fA represents that SCF is influenced by IRD. This is expressed as:
(1)
(1)
where fA is positive with RA and fA is negative with RB, which means that
,
. Therefore, we simplify the equation to the following:
(2)
(2)
where RAB = RA – RB and
, which means the capital flow is positive with IRD. When the domestic interest is lower than the interest rate in the rest of the world, capital flows out of the country until the two interest rates become equal again.
4. Methodology
4.1. Bootstrap full-sample causality test
The Granger-causality statistics assume that underlying time series are stationary and they may not have standard asymptotic distributions when the stationarity assumption does not hold. In this condition, there will be difficulties in the estimation levels of vector autoregression (VAR) models (Sims, Stock, & Watson, Citation1990; Toda & Phillips, Citation1993, Citation1994). Shukur and Mantalos (Citation1997a) use Monte Carlo simulations to evaluate power and size properties of the modified Wald test. However, the result indicates that the Wald test does not have the correct size in small- and medium-sized samples. Nevertheless, Shukur and Mantalos (Citation1997b) indicate that as a result of using the residual-based bootstrap (RB) method, critical values are improved in power and size. Moreover, the excellent performance of the RB method over standard asymptotic tests, regardless of cointegration, in a vast number of Monte Carlo simulation studies has been positively verified (Balcilar, Ozdemir, & Arslanturk, Citation2010; Hacker & Hatemi-J, Citation2006; Mantalos, Citation2000; Mantalos & Shukur, Citation1998; Shukur & Mantalos, Citation2000). In particular, Shukur and Mantalos (Citation2000) prove that small sample corrected likelihood ratio (LR) tests display relatively improved properties, such as power and size, regardless of the dimension of the samples – including small ones. Such results point out that, should the cointegration be absent, all standard tests not making use of the RB method would perform inadequately, particularly in those small samples. As a consequence, this article resorts to the option of the RB-based modified-LR statistic to examine causality between IRD and SCF.
To show the RB-based modified-LR causality test, the bivariate VAR (p) process is considered as follows:
(3)
(3)
where
is a zero mean, independent, white noise process with non-singular covariance matrix
. The optimal lag length p is determined by the Schwarz information criteria (SIC) in this paper.
, i, j = 1, 2 and L is the lag operator defined as
.
Based on EquationEquation (3)(3)
(3) , the null hypothesis that IRD does not Granger cause SCF, which means the fluctuations of IRD do not produce influence on SCF, is tested by imposing the restriction,
for k = 1, 2… p. Similarly, the null hypothesis that SCF does not Granger cause IRD, which means the changes of SCF have no impact on IRD, is tested by imposing the restriction
for k = 1, 2… p. As previously debated, the tests of full-sample causality applied in this paper rely on RB-based p-values and modified-LR statistics. If the first null hypothesis
for k = 1, 2… p is rejected, there is a significant causality running from IRD to SCF. This means IRD can predict movements in inflation. Similarly, if the second null hypothesis
for k = 1, 2… p is rejected, IRD is caused by movements in SCF. If the result of the test shows that IRD can cause SCF, the PBOC can adopt moderate interest rate policy to smooth SCF.
4.2. Parameter stability test
The full-sample causality tests usually assume that the parameter of the VAR model used in testing is constant over time. However, when the underlying full-sample time exhibits structural changes, the assumption is probably violated. The results from the full-sample causality tests become invalid, and hence the causal links between series show instability (Balcilar & Ozdemir, Citation2013). Granger (Citation1996) stresses that parameter non-constancy is one of the challenging issues that are being dealt with in recent empirical studies, resulting in further testing regarding short- and long-run parameter stability tests being conducted in this paper. Sup-F, Mean-F and Exp-F tests (Andrews, Citation1993; Andrews & Ploberger, Citation1994) are used to investigate short-run parameter stability. Regardless, the fact has been pinned down that when underlying variables are cointegrated in levels, the first difference in the VAR model is wrongly specified unless it allows for error correction. Hence, it is essential to test for the cointegration and parameter stability of the long-run relationship. This is achieved based on different structural changes and parameter stability tests on the long-run relationship estimated using the modified ordinary least square (FM-OLS) estimator of Phillips and Hansen (Citation1990). Combing with FM-OLS, the test proposed by Nyblom (Citation1989) and Hansen (1992) is applied to check the stability of long-run parameters. The Lc test also serves as a test of cointegration when the underlying series are I(1).
These tests are calculated from the sequence of LR statistics that check the stability of the parameter against the provided substitute of a unique structural break at an unknown time. Since these tests exhibit non-standard asymptotic distributions, critical values and p-values are proposed by means of the parametric bootstrap procedure (Andrews, Citation1993; Andrews & Ploberger, Citation1994). Andrews (Citation1993) also points out that statistics require 15% trimming from both ends of the sample to test the stability of parameters in the short run, and the fraction of the sample in (0.15, 0.85) is needed. The Lc tests are conducted in the current paper for the equations and VAR system separately by applying the FM-OLS estimator.
4.3. Sub-sample rolling-window causality test
Based on the above analysis, it is necessary to use the rolling-window bootstrap method (Balcilar et al., Citation2010). There are two advantages of using the bootstrap rolling-window test. First, a rolling window is applicable when the casual link between variables is time-varying. Second, since structural changes exist, a rolling method is unstable in different sub-samples. The rolling-window technique is grounded on fixed-size sub-samples rolling unceasingly from start to end of the full sample. In particular, when given a fixed-size rolling window including m observations, the full sample is transformed into a sequence of T – m sub-samples. That is, – m + 1,
– m, …, T for
=m, m + 1, …, T. Then, it can apply the RB-based modified-LR causality test to each sub-sample, instead of estimating a single causality test for a full sample. Possible changes in the causal links between IRD and SCF are intuitively identified by calculating the bootstrap p-values of observed LR statistics rolling through T – m sub-samples. The influence of IRD on SCF is defined as the average of the entire bootstrap estimates derived from the formula
, with Nb representing the number of bootstrap repetitions; similarly, the influence of SCF on IRD is obtained from the formula
. Both
and
are bootstrap estimates from the VAR models in EquationEquation (3)
(3)
(3) . The 90% confidence intervals are also computed, for which the lower and upper limits equal the 5th and 95th quantiles of each of the
and
, respectively.
There are two conflicting objectives in the rolling-window estimation, accuracy of the parameter estimates and the representativeness of the model over the sub-sample period. A large window size may improve the accuracy of the estimate but may reduce the representativeness in the presence of heterogeneity. On the contrary, a small one may improve the representativeness and reduce accuracy. Consequently, we must select a suitable number of observations to balance the trade-off between representativeness and accuracy. Pesaran and Timmermann (Citation2005) demonstrate that optimal window size depends on persistence and size of the break and they use Monte Carlo simulations to show that the minimum limit is 20 observations. Taking it and the two conflicting demands, we chose 24 months as the optimal selection in this paper.
5. Data
We use the monthly data covering the period from 2009:M01 to 2018:M12. Because the U.S. subprime crisis broke out in 2007 and spread rapidly to the whole world, the Chinese economy has been in the doldrums and lacked effective market demand from outside. Hence, the sample of our study starts at 2009:M01, which isolates effects from the global economic crisis. The IRD is computed as the difference between the Shanghai Interbank Offered Rate and London Interbank Offered Rate, which represent domestic and foreign interest rate, respectively. The IRD reflects dynamic changes in monetary policies between home and abroad, which can reflect Chinese and global economic conditions (Makhetha-Kosi et al., Citation2016). In China, the measurement of SCF is based on the position of Forex purchase of a financial institution (FPFI), actual utilisation of foreign capital (FDI), trade surplus (TS) and trade misinvoicing (TM), which can be written as SCF = –(FPFI – FDI – TS + TM). The surges and reversals in SCF have become an unneglectable factor that affects economic development and stability and will bring higher requirements and serious challenges to China’s macroeconomic policies (Ning & Zhang, Citation2018). The data sources are from the State Administration of Foreign Exchange and National Bureau of Statistics of the People’s Republic of China.
describes the trend of IRD and SCF, and shows significant changes during the sample. Being affected by the global financial crisis, major advanced countries, including the U.S., Japan and the eurozone, have carried out several rounds of quantitative easing to boost economic growth. The expansionary monetary policy has led to their interest rates being cut towards zero. However, the Chinese policy response has been more subdued, which has meant a pronounced interest rate differential between home and abroad, and led to capital inflow surges and currency appreciation episodes in the latter (Acharya & Bengui, Citation2018; Zoega, Citation2016). Cho and Rhee (Citation2013) indicate that the capital inflows collapsed in 2008 and 2009, and then sharply rebounded to the pre-crisis level when the global economy recovered along with quantitative easing policies. In 2015, the U.S. announced it was increasing its interest rate, which ended the zero interest rate era that had prevailed since the global financial crisis. With the U.S. quitting its quantitative easing policies, China witnessed a slowdown in capital inflows, and even a reversal of capital (Ning & Zhang, Citation2018). Hence the quantitative easing policy, especially in the U.S., had a significant influence on IRD and SCF. Besides, according to previous studies, exchange rate (Hacker, Karlsson, & Mansson, Citation2014), stock price (Li et al., Citation2018) and capital control (Cheung et al., Citation2016) are also considered as factors that influence changes in IRD and SCF. In our paper, the cointegration test (Johansen, Citation1988; Johansen & Juselius, Citation2009) given in the Appendix is employed to assess the long-run relationship among these factors. indicate that both the Trace statistic and the -max statistic demonstrate that the null hypothesis of no cointegration can be rejected, which means short-term capital flow, interest rate differential, exchange rate, stock price and capital control have a cointegrating relationship.
Table 1. Unit root tests.
Table 2. Full-sample Granger-causality tests.
6. Empirical results
To test for stationarity of the underlying variables, several univariate unit root tests – ADF (Dickey & Fuller, Citation1981), PP (Phillips & Perron, Citation1988) and KPSS (Kwiatkowski, Phillips, Schmidt, & Shin, Citation1992) – are employed to examine the null of unit root of the IRD and SCF. The results from clearly demonstrate that the ADF and PP tests fail to reject the null hypothesis of the random-walk process for all of the series in levels. However, they reject the null hypothesis when the series are first differences. The KPSS tests can reject the null hypothesis of stationarity for all of the series in levels. Nevertheless, they cannot reject the null hypothesis of stationarity for all of the series in their first differences. To summarise, the ADF, PP and KPSS unit root tests demonstrate that IRD and SCF are non-stationary processes in levels but they attain stationarity in their first differences. This means that they are both first-order integrated, i.e., I(1), implying that the cointegration test ( test) should be performed to decide whether the first difference VAR model is misspecified by ignoring an error-correction term.
We use the VAR model to test the full-sample causal relationship between IRD and SCF. The bivariate VAR models are the IRD and SCF and are constructed as in EquationEquation (3)(3)
(3) . Upon examination, the optimal lag length based on the Akaike Information Criterion (AIC) of IRD and SCF is four. The full-sample causality results based on the RB-based modified-LR causality tests are reported in . According to the bootstrap p-values, IRD does Granger cause SCF, but SCF does not Granger cause IRD. This finding is consistent with some of the previous studies, including Ahmed and Zlate (Citation2014), Makhetha-Kosi et al. (Citation2016) and Ayanou (2016).
There is, however, a default assumption in the previous literature that in time series structural changes do not exist and there is only a single causal relationship across the whole sample period. In the presence of structural changes, the parameters in the above VAR model estimated using full-sample IRD and SCF will shift with time. The causal relationship between IRD and SCF will accordingly be unstable. Therefore, the full-sample tests considering the assumptions of parameter constancy with a single causal relationship in the whole sample period will no longer be reliable. For this reason, the present paper continues to test for parameter stability and pursues the task of confirming or denying the existence of structural changes. As previously mentioned, we make use of the Sup-F, Mean-F and Exp-F tests that were created by Andrews (Citation1993) and Andrews and Ploberger (Citation1994) to investigate the temporal stability of parameters in the above VAR model formed by IRD and SCF. Nyblom’s (Citation1989) and Hansen’s (1992) Lc test have also been employed hereby in testing for all parameters in the overall VAR system.
The corresponding results are reported in . The Sup-F test under the null hypothesis of parameter constancy against a one-time sharp shift in parameters is reported in the first row. The conclusion shows that a one-time sharp shift exists in the IRD, SCF and VAR system at the 1% level. The Mean-F and Exp-F tests that were run under the null hypothesis, where parameters pursue a martingale process, completely opposed the possibility of parameters gradually evolving, an option which is reported in the second and third rows, respectively. The results from Sup-F, Mean-F and Exp-F tests indicate that equations from the IRD, SCF and VAR system may present a progressive evolution with time. Lc statistics test indicates that the parameters follow random walk process and do not constant in VAR model. As a consequence, these results provide robust evidence that the parameters of the estimated VAR model using full-sample data show short-term instability.
Table 3. Parameter stability tests.
The variables of IRD and SCF are both I(1) processes provided by unit root tests. These imply that the VAR model formed by the variables in their first differences is misspecified when the cointegration exists. Hence, tests for cointegration and parameter stability of the long-run relationship should be conducted in the following step. In particular, the FM-OLS estimator is used to estimate cointegration and the Sup-F, Mean-F and Exp-F as well as the test are used to test parameter stability of the long-run relationship. The corresponding results are shown in . According to the bootstrap p-value of the
statistic, it rejects the null hypothesis of cointegration at the 1% significance level. The Sup-F statistic rejects the null hypothesis of parameter constancy at the 1% significance level. Meanwhile, gradual shifting of parameters in the cointegration equation can be recognised from the Mean-F and Exp-F tests. The results provide robust evidence that no reliable cointegration exists between IRD and SCF.
Table 4. Parameter stability tests in long-run relationship for China.
Based on the above parameter stability tests, both the short-run and long-run parameters in the VAR models estimated using full-sample data show instability because of structural changes, and hence the result of any full-sample causality between SCF and IRD is meaningless. Therefore, the VAR model in EquationEquation (3)(3)
(3) can serve as a basis framework and we continue to perform the rolling-window causality test with sub-sample data. The employed rolling-window estimation takes structural changes into account and allows the causal links between variables to be time-varying across different sub-samples, which differs greatly from existing literature. The bootstrap p-values of LR statistics can be estimated from the VAR models in EquationEquation (3)
(3)
(3) using the rolling sub-sample data. After trimming 24-month1 observations from the beginning of the null sample, these rolling estimates move from 2011:M01 to 2018:M12.
indicates the null hypothesis that IRD does not Granger cause SCF is rejected at the 10% significance level in 2012:M03–2012:M08 and 2015:M03–2015:M09. further demonstrates that IRD has a positive impact on SCF in those periods. As we can see from , in the period 2012:M03–2012:M08 and 2015:M03–2015:M09 both variables of IRD and SCF have the same trend, which is consistent with the Mundell–Fleming model (Mundell, Citation1960; Fleming, Citation1962). Generally, international capital would flow to countries that have a relatively high interest rate and pursue higher returns. Being affected by the global financial crisis, the major developed countries, especially the U.S., implemented expansionary monetary policies which led to interest rates cut towards the zero mark (Acharya & Bengui, Citation2018). However, for China the policy response was more subdued as it was less affected by the crisis. For example, the PBOC just reduced the benchmark of renminbi deposit and lending rates of financial institutions by 0.5% and 0.56% in 2012, which still kept relatively high levels. Hence, there were pronounced interest rate differentials between China and the U.S., which led to capital inflow surges and currency appreciation episodes (Zoega, Citation2016). Ahmed and Zlate (Citation2014) and Makhetha-Kosi et al. (Citation2016) proved the persistent effect of interest rate differentials on capital flows. Ahmed and Zlate (Citation2014) further demonstrate that there have been significant changes in the behaviour of SCF since before the global financial crisis to the post-crisis period, which are partly explained by the greater sensitivity to interest rate differentials. The short-term capital flows to China surged in the aftermath of the crisis, which raised a number of concerns in recipient economies. First, large capital inflows can overwhelm the intermediation capacity of domestic financial systems, leading to excessive credit creation and asset price bubbles that create risks of financial instability. Second, large capital inflows may cause currencies to appreciate, which in turn could hurt export and growth performance. Finally, large inflows can complicate the pursuit of appropriate macroeconomic policies to maintain solid economic growth without rising inflation.
Figure 2. Bootstrap p-value of rolling test statistic testing the null that IRD does not Granger cause SCF. Source: Author’s calculations.
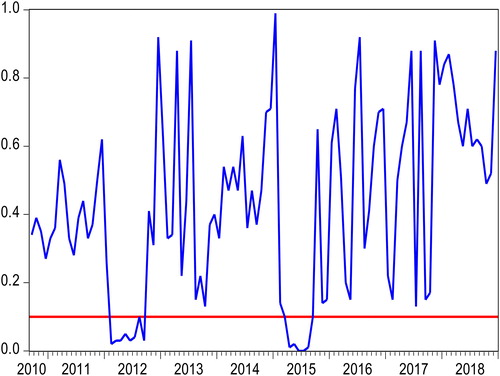
Figure 3. Bootstrap estimates of the sum of the rolling-window coefficients for the impact of IRD on SCF. Source: Author’s calculations.
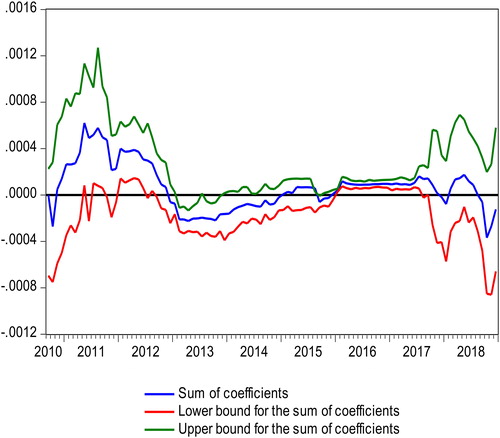
However, in the periods 2011:M01–2012:M02, 2012:M09–2015:M03 and 2015:M10–2018:M12, IRD has no significant impact on SCF, which is mainly because other factors exceed IRD to become the main factors that influence SCF. Chari and Kehoe (Citation2003), Cho and Rhee (Citation2013), Chen and Kong (Citation2014) and Makhetha-Kosi et al. (Citation2016) give their aspects from quantitative easing policy, exchange rate and other factors. This phenomenon may be explained by other factors in different time intervals. In the first period, the Federal Reserve implemented quantitative easing policies, which weakened the U.S. dollar and stimulated capital outflows to emerging economies. These policies are assessed to have largely succeeded at achieving their intended domestic goals, which reduced domestic interest rates and drove capital out of the U.S. In the second interval, China faced a real estate prices surge, which contributed to over-inflows of international speculative capital. Therefore, the central government in China implemented a most stringent real estate policy, which stabilised housing prices and pushed part of SCF out of China. At the same time, the Federal Reserve began to quit its quantitative easing policies and the Federal Reserve’s funds rate was expected to increase, which narrowed the interest rate differential and made East Asian countries (e.g., China) face capital outflow and put pressure on their own interest rate. In the third sub-interval, China’s gross domestic product growth rate was 6.7% in the second quarter, which reached its lowest point. It would reduce employment, consumption and international trade, which further decreased SCF. Meanwhile, Britain’s expected departure from the European Union led to global concerns that Britain’s economy will suffer a recession, which pushed SCF to more secure countries (e.g., U.S.).
reports the rolling bootstrap p-values of the LR statistics with the null hypothesis that SCF does not Granger cause IRD. According to , we can clearly see that the null hypothesis cannot be rejected significantly in the full-sample period, which shows that SCF has no influence on IRD. The empirical results are inconsistent with previous studies, including Warnock and Warnock (Citation2009), Bertaut, DeMarco, Kamin, and Tryon (Citation2012) and Carvalho and Fidora (Citation2015). Being different from previous studies, this study offers new analysis aspects for this phenomenon. First, Chinese capital control policy plays a role in protecting the domestic financial environment from external shocks. Although China has started liberalisation of its capital account, the trend will be altered when the Chinese government needs stricter capital controls to deal with threats to financial stability, e.g., the global financial crisis in 2008. Moreover, China’s capital account liberalisation is uneven across different aspects. For example, capital controls are stricter on capital inflows than outflows. While China opens up swiftly to long-term capital movement, it maintains a fairly tight level of controls on short-term capital. Second, China places a higher priority on exchange rate stability than using monetary policy as a tool for macroeconomic control, which results in the scope for an autonomous monetary policy being constrained. Finally, the PBOC still carries out interest rate control. For example, it stipulates the deposit rate cap and lending rate floor, and sets the range interest rates are allowed to vary for state-owned and commercial banks. Besides, the PBOC also sets interest rates for its operation, including rates of central bank rediscount, excess deposit reserve, and others. These have become increasingly important tools to signal the PBOC’s policy intentions and guide market interest rates towards desired levels.
Figure 4. Bootstrap p-value of rolling test statistic testing the null that SCF does not Granger cause IRD. Source: Author’s calculations.
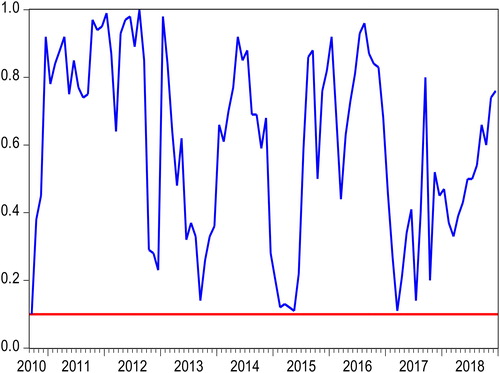
In this paper, the bootstrap Granger full-sample causality test and sub-sample rolling-window estimation provide additional insight into the dynamic relationship between IRD and SCF in China. In general, the relationship between IRD and SCF is not always consistent with the view that SCF changes with IRD in the same direction. It can support the Mundell–Fleming model in the periods of 2012:M03–2012:M08 and 2015:M01–2015:M09. However, in other periods we cannot get the same conclusion. The relationship between IRD and SCF in China is not stable over time and even shows a short-term deviation from the positive link. It is actually suitable as, in the past few decades, China has experienced domestic economic restructuring and global economic fluctuation. That unavoidability produces influences on Chinese monetary policies. Since the financial crisis in 2007, there has been a significant impact on the Chinese economic environment between home and abroad. China began interest rate liberalisation and removed the interest ceiling on deposit rates. Meanwhile, the supply-side structural reforms were put forward at the Central Economic Working Conference in 2015, which pushed the Chinese economy towards high-quality development, speeding up the replacement of the old drivers of growth. All these internal and external events may have a temporary impact on the relationship between IRD and SCF in China and even lead to structural changes.
7. Conclusions
This study has investigated the causal relationship between IRD and SCF using a bootstrap full-sample Granger-causality test and sub-sample rolling-window causality estimation in China. This relation is proved because the full-sample Granger-causality test provides a unidirectional positive influence from IRD to SCF. Nevertheless, taking into consideration the presence of structural changes in the full-sample data, parameter stability tests find that, in the short run, relationships between IRD and SCF are unstable. Further, we employ a sub-sample rolling window-causality estimation, and provide evidence that IRD has an impact on SCF in some periods. Although the relationship between IRD and SCF in China is not stable over time and even exhibits short-run deviations from the positive link, it actually fits well with the fact that China experiences economic transition and structural changes in economic policies. The PBOC employs discretionary policies that accord with the economic environment regulating SCF and achieve various economic goals. In normal economic circumstances without internal and external shocks, IRD can lead to SCF. Otherwise, IRD cannot be the main factor that causes SCF. Hence, this study provides some policy implications for SCF in particular. First, we should correctly understand the importance of capital supervision and pay attention to the speed of international capital liberalisation. The supervision of SCF can somehow release pressure on independent monetary policies, especially interest rate policy. Even though the government has gradually liberalised the original capital supervision, radical capital account liberalisation policy is not desirable. Second, China should establish and improve the supervision mechanism for international short-term capital flow. At present, China employs administrative intervention in the supervision of SCF, and it mostly focuses on the control of quantity, which has proved insufficient. In the future, management tools such as the Tobin tax and Unremunerated Reserve Requirement can also be employed to control the flow of capital. Third, an international cooperation mechanism for SCF should be built. China is involved in the world and cannot isolate relations with other economies. China should depend not only on domestic efforts, but also on international cooperation, such as the World Bank and International Monetary Fund (IMF), to monitor SCF.
Disclosure statement
No potential conflict of interest was reported by the authors.
Notes
1 We wondered whether the results are sensitive to the choice of window size. Thus, we selected different window sizes (e.g., 12, 24 and 36 months) to test and found the results are affected, but very little.
References
- Acharya, S., & Bengui, J. (2018). Liquidity traps, capital flows. Journal of International Economics, 114, 276–298. doi:10.1016/j.jinteco.2018.06.005
- Ahmed, S., & Zlate, A. (2014). Capital flows to emerging market economies: A brave new world. Journal of International Money and Finance, 48, 221–248. doi:10.1016/j.jimonfin.2014.05.015
- Aizenman, J., & Kendall, J. (2012). The internationalization of venture capital. Journal of Economic Studies, 39(5), 488–511. doi:10.1108/01443581211259446
- Andrews, D. W. K. (1993). Test for parameter instability and structural change with unknown change point. Econometrica, 61(4), 821–856. doi:10.2307/2951764
- Andrews, D. W. K., & Ploberger, W. (1994). Optimal test when a nuisance parameter is present only under the alternative. Econometrica, 62(6), 1383–1414. doi:10.2307/2951753
- Arteta, C., Kose, M. A., Stocker, M., & Taskin, T. (2018). Implications of negative interest rate policies: An early assessment. Pacific Economic Review, 23(1), 8–26. doi:10.1111/1468-0106.12249
- Ayanou, T. (2016). Foreign capital inflows to the USA and mortgage interest rates. Journal of Housing Economics, 34, 1–14. doi:10.1016/j.jhe.2016.06.001
- Balcilar, M., Ozdemir, Z. A., & Arslanturk, Y. (2010). Economic growth and energy consumption casual nexus viewed through a bootstrap rolling window. Energy Economics, 32(6), 1398–1410. doi:10.1016/j.eneco.2010.05.015
- Balcilar, M., & Ozdemir, Z. A. (2013). The export-output growth Nexus in Japan: A bootstrap rolling window approach. Empirical Economics, 44(2), 639–660. doi:10.1007/s00181-012-0562-8
- Barroso, J. B. R., Da Silva, L. A. P., & Sales, A. S. (2016). Quantitative easing and related capital flows into Brazil: Measuring its effects and transmission channels through a rigorous counterfactual evaluation. Journal of International Money and Finance, 67, 102–122. doi:10.1016/j.jimonfin.2015.06.013
- Bertaut, C., DeMarco, L. P., Kamin, S., & Tryon, R. (2012). ABS inflows to the United States and the global financial crisis. Journal of International Economics, 88(2), 219–234. doi:10.1016/j.jinteco.2012.04.001
- Cai, T., Dang, V. Q. T., & Lai, J. T. (2016). China’s capital and hot money flows: An empirical investigation. Pacific Economic Review, 21(3), 276–294. doi:10.1111/1468-0106.12091
- Carvalho, D., & Fidora, M. (2015). Capital inflows and euro area long-term interest rates. Journal of International Money and Finance, 54, 186–204. doi:10.1016/j.jimonfin.2015.02.021
- Chari, V. V., & Kehoe, P. J. (2003). Hot money. Journal of Political Economy, 111(6), 1262–1292. doi:10.1086/378525
- Chen, X. J., & Kong, L. L. (2014). The affecting factors of inflows of international hot money to China. Proceedings of the Eighth International Conference on Management Science and Engineering Management, 3, 1531–1540.
- Chen, C., Yao, S., Zheng, T., & Ou, J. (2017). Interest rate marketization, exchange rate restructuring and international capital flow. International Monetary Review, 57, 100–119.
- Cheung, Y., Steinkamp, S., & Westermann, F. (2016). China’s capital flight: Pre- and post- crisis experiences. Journal of International Money and Finance, 66, 88–112. doi:10.1016/j.jimonfin.2015.12.009
- Cho, D., & Rhee, C. (2013). Effects of quantitative easing on Asia: Capital flows and financial markets. ADB Economics Working Paper. doi:10.2139/ssrn.2282304
- Daǧlaroǧlu, T., Demirel, B., & Mahmud, S. F. (2018). Monetary policy implications of short-term capital flows in Turkey. Empirica, 45, 747–763. doi:10.1007/s10663-017-9388-0
- Dickey, D. A., & Fuller, W. A. (1981). Likelihood ratio statistics for autoregressive time series with a unit root. Econometrica, 49(4), 1057–1072. doi:10.2307/1912517
- Fleming, J. M. (1962). Domestic financial policies under fixed and floating exchange rates. Staff Papers - International Monetary Fund, 9(3), 369–380. doi:10.2307/3866091
- Glick, R., & Hutchison, M. (2009). Navigating the trilemma: Capital flows and monetary policy in China. Journal of Asian Economics, 20(3), 205–224. doi:10.1016/j.asieco.2009.02.011
- Globan, T. (2015). Financial integration, push factors and volatility of capital flows: Evidence from EU new member states. Empirica, 42(3), 643–672. doi:10.1007/s10663-014-9270-2
- Granger, C. W. J. (1996). Can we improve the perceived quality of economic forecasts? Journal of Applied Econometrics, 11(5), 455–473. doi:10.1002/(SICI)1099-1255(199609)11:5<455::AID-JAE408>3.0.CO;2-E
- Grubel, H. G. (1968). Internationally diversified portfolios: Welfare gains and capital flows. American Economic Review, 58, 1299–1314.
- Hacker, R. S., Karlsson, H. K., & Mansson, K. (2014). An investigation of the causal relations between exchange rates and interest rate differentials using wavelets. International Review of Economics & Finance, 29, 321–329. doi:10.1016/j.iref.2013.06.004
- Hacker, R. S., & Hatemi-J, A. (2006). Tests for causality between integrated variables based on asymptotic and bootstrap distributions: Theory and application. Applied Economics, 38(13), 1489–1500. doi:10.1080/00036840500405763
- Hansen, B. E. (1992). Tests for parameter instability in regression with I(1) processes. Journal of Business & Economic Statistics, 10, 321–336. doi:10.2307/1391545
- Ho, K., Shi, Y., & Zhang, Z. (2017). Does news matter in China’s foreign exchange market? Chinese RMB volatility and public information arrivals. International Review of Economics & Finance, 52, 302–321. doi:10.1016/j.iref.2017.01.016
- Johansen, S. (1988). Statistical analysis of cointegration vectors. Journal of Economic Dynamics and Control, 12(2–3), 231–254. doi:10.1016/0165-1889(88)90041-3
- Johansen, S., & Juselius, K. (2009). Maximum likelihood estimation and inference on cointegration with applications to the demand for money. Oxford Bulletin of Economics and Statistics, 52(2), 169–210.
- Korinek, A. (2018). Regulating capital flows to emerging markets: An externality view. Journal of International Economics, 111, 61–80. doi:10.1016/j.jinteco.2017.12.005
- Kwiatkowski, D., Phillips, P., Schmidt, P., & Shin, Y. (1992). Testing the null hypothesis of stationary against the alternative of a unit root: How sure are we that economic time series have a unit root? Journal of Econometrics, 54(1–3), 159–178. doi:10.1016/0304-4076(92)90104-Y
- Li, J., Liu, Y., & Ge, S. (2012). China’s alternative remittance system: Channels and size of hot money flows. Crime, Law and Social Change, 57(3), 221–237. doi:10.1007/s10611-011-9350-1
- Li, X., Su, C., Chang, H., & Ma, J. (2018). Do short-term international capital movements play a role in exchange rate and stock price transmission mechanism in China? International Review of Economics & Finance, 57, 15–25. doi:10.1016/j.iref.2018.02.010
- Lv, G., & Xu, M. (2012). China’s short-term international capital flows: A triple-motive analysis based on monthly VAR model. Studies of International Finance, 300, 61–68.
- Ma, H. (2018). A study on the influence factors of short-term international capital flows-the evidence from emerging markets. Modern Economy, 9(4), 758–774. doi:10.4236/me.2018.94050
- Makhetha-Kosi, P., Mishi, S., & Ngonyama, N. (2016). The response of capital flows to interest rate differentials: The case of South Africa. Journal of Economics, 7, 119–129. doi:10.1080/09765239.2016.11907827
- Mantalos, P., & Shukur, G. (1998). Size and power of error correction model cointegration test: A Bootstrap Approach. Oxford Bulletin of Economics and Statistics, 60(2), 249–255. doi:10.1111/1468-0084.00097
- Mantalos, P. (2000). A graphical investigation of the size and power of the ganger-causality tests in integrated-cointegrated VAR systems. Studies in Nonlinear Dynamics and Econometrics, 4(1), 17–33. doi:10.1162/108118200569162
- Meade, J. E. (1951). The theory of international economic policy. London: Oxford University Press.
- Mundell, R. A. (1960). The monetary dynamics of international adjustment under fixed and flexible exchange rates. The Quarterly Journal of Economics, 84, 227–257. doi:10.2307/1884252
- Nier, E., Sedik, T. S., & Mondino, T. (2014). Gross private capital flows to emerging markets: Can the global financial cycle be tamed? IMF Working Papers, 14, 1–12. doi:10.5089/9781498351867.001
- Ning, Y., & Zhang, L. (2018). Modeling dynamics of short-term international capital flows in China: A Markov regime switching approach. The North American Journal of Economics and Finance, 44, 193–203. doi:10.1016/j.najef.2018.01.002
- Nyblom, J. (1989). Testing for the constancy of parameters over time. Journal of the American Statistical Association, 84(405), 223–230. doi:10.1080/01621459.1989.10478759
- Obstfeld, M. (2011). International liquidity: The fiscal dimension. NBER Working Paper No. 17379. doi:10.3386/w17379
- Orlowski, L. T. (1999). Capital inflows and convertibility in the transforming economies of Central Europe. London: Palgrave Macmillan.
- Osterwald-Lenum, M. (1992). A note with quantiles of the asymptotic distribution of the maximum likelihood cointegration rank test statistics. Oxford Bulletin of Economics and Statistics, 54(3), 461–472. doi:10.1111/j.1468-0084.1992.tb00013.x
- Overholt, W. H. (2010). China in the global financial crisis: Rising influence, rising challenges. The Washington Quarterly, 33(1), 21–34. doi:10.1080/01636600903418652
- Perron, P. (1989). The Great Crash, the Oil Price Shock, and the Unit Root Hypothesis. Econometrica, 57(6), 1361–1401. doi:10.2307/1913712
- Pesaran, M. H., & Timmermann, A. (2005). Small sample properties of forecasts from autoregressive models under structural breaks. Journal of Econometrics, 129(1-2), 183–217. doi:10.1016/j.jeconom.2004.09.007
- Phillips, P. B. C., & Hansen, B. E. (1990). Statistical inference in instrumental variables regression with I (1) processes. The Review of Economic Studies, 57(1), 99–125. doi:10.2307/2297545
- Phillips, P. C. B., & Perron, P. (1988). Testing for a unit root in time series regression. Biometrika, 75(2), 335–346. doi:10.1093/biomet/75.2.335
- Shukur, G., & Mantalos, P. ( (1997a). ). Size and power of the RESET test as applied to systems of equations: A bootstrap approach. Working paper, Department of Statistics, University of Lund, Sweden.
- Shukur, G., & Mantalos, P. ( (1997b). ). Tests for Granger causality in integrated-cointegrated VAR system. Working paper, Department of Statistics, University of Lund, Sweden.
- Shukur, G., & Mantalos, P. (2000). A simple investigation of the granger-causality test in integrated-cointegrated VAR Systems. Journal of Applied Statistics, 27(8), 1021–1031. doi:10.1080/02664760050173346
- Sims, C. A., Stock, J. H., & Watson, M. W. (1990). Inference in linear time series with some unit roots. Econometrica, 58(1), 113–144. doi:10.2307/2938337
- Toda, H. Y., & Phillips, P. C. B. (1993). Vector autoregression and causality. Econometrica, 61(6), 1367–1393. doi:10.2307/2951647
- Toda, H. Y., & Phillips, P. C. B. (1994). Vector autoregression and causality: A theoretical overview and simulation study. Econometric Reviews, 13(2), 259–285. doi:10.1080/07474939408800286
- Verma, R., & Prakash, A. (2011). Sensitivity of capital flows to interest rate differentials: An empirical assessment for India. RBI Working Paper Series: 7/2011, Department of Economic and Policy Research, New Delhi, India.
- Wang, C., Hwang, J., & Chung, C. (2016). Do short-term international capital inflows drive China’s asset market? The Quarterly Review of Economics and Finance, 60, 115–124. doi:10.1016/j.qref.2015.10.006
- Warnock, F. E., & Warnock, V. C. (2009). International capital flows and U.S. interest rates. Journal of International Money and Finance, 28(6), 903–919. doi:10.1016/j.jimonfin.2009.03.002
- Zhang, M., & Tan, X. (2013). The driving factors of short-term capital flow in China. The World Economy, 11, 93–116.
- Zoega, G. (2016). Responding to capital flows in a very small economy. Atlantic Economic Journal, 44(2), 159–170. doi:10.1007/s11293-016-9497-3
Appendix
We use the cointegration test to examine the long-term relationship among interest rate differential (IRD), short-term capital flow (SCF), exchange rate (ER), stock price (SP) and capital control (CC). Because these variables are not stationary, we take their first difference. For comparison, we introduce the augmented Dickey–Fuller (1981, ADF), the Phillips and Perron (Citation1988, PP) and the Kwiatkowski, Phillips, Schmidt, & Shin (Citation1992, KPSS) tests in our study. shows the results from non-stationary tests for the IRD, SCF, SP, ER and CC. Again, the test results further indicate that these variables are integrated of order one, I(1). In light of the results, the conventional cointegration test is employed to examine whether a long-term relationship exists among these variables. presents the conclusion from the Johansen (Citation1988) and Johansen and Juselius (Citation2009) cointegration tests. As shown, both the Trace statistic and the -max statistic demonstrate that the null hypothesis of no cointegration can be rejected, which means short-term capital flow, interest rate differential, exchange rate, stock price and capital control have a cointegrating relationship.
Table A1. Unit root tests.
Table A2. Unit root tests.