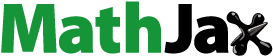
Abstract
The main aim of the research is to investigate whether small- and medium-sized enterprises (SMEs) employment affects total European Union (EU-28) employment using panel data models during the 2005–2016 period. The panel econometric results confirm the significant association between SMEs employment in service sectors and overall economy employment, whereas the effect of SMEs industry sectors employment was found insignificant. The results are robust in a spite of the fact that during the studied period the EU-28 countries experienced economic crisis suggesting the important role that SMEs can play in job creation and in reduction of unemployment even when macro-economic enabling environment might be less favourable.
1. Introduction
Globalisation and technological changes enable small- and medium-sized enterprises (SMEs) reaching new opportunities. The importance of SMEs has also been recognised in the European Union (EU) countries by setting the framework to promote entrepreneurship, simplifying the regulatory and policy environment for SMEs and removing the remaining barriers to their development. Namely, SMEs are seen as the most important driver of economic growth and play a significant role in employment creation and therefore fighting against unemployment. In recent years, macroeconomic environment has been more favourable for growth of SMEs, particularly due to real growth in all components of aggregate demand. The vast majority of enterprises active within the EU are SME Considering the employment and other performance figures, SMEs are also contributing towards delivering the key objectives of the Europe 2020 strategy to reach smart, sustainable and inclusive growth. SMEs are seen as a vital part of national economies contributing to employment and value added. In the EU-28 SMEs account for more than 99% of all enterprises in the non-financial business economy out of which 93% are micro enterprises, less than 6% are small enterprises and less than 1% are medium enterprises. Micro enterprises contribute around 30% of total employment, whereas SMEs contribute approximately 20% and 17% of total employment respectively. Altogether, SMEs contribute more than 66% of total EU employment, the rest contribute large enterprises. Additionally, from a value added perspective SMEs contribute around 57% of total value added with more equally distributed shares among SMEs (Muller et al., Citation2017). Encouraging the growth of SMEs in the EU contributes to economic growth and employment therefore reaching the key objectives of the Europe 2020 strategy. In contrast to large enterprises SMEs are able to respond to the needs in the economy faster due to their flexibility and capability of networking (Alpkan, Yilmaz, & Kaya, Citation2007). On the other hand, small versus large often represent the barrier taking into account general business environment, access to strategic resources and exporting opportunities of SMEs (see, for example, Krammer, Strange, & Lashitew, Citation2018; Paul, Parthasarathy, & Gupta, Citation2017).
The main aim of this research is to investigate whether SME employment affects total EU employment using panel data models. To provide insight into which SME sector employment adds more to total EU employment we have additionally decomposed SME employment into SME service sector employment and into SME industry sector employment. The results obtained by the applied advanced panel data modelling approach to study SMEs employment contributions in the EU-28 countries are of practical and policy relevance. Boosting SME employment potential not only has benefits for the economy as a whole but also for the society and human well-being decreasing the pressures on social transfers (OECD, Citation2017). Boosting SME employment potential depends to a great degree on simplifying the regulatory and policy environment for SMEs and removing the remaining barriers to their development. This study is important in order to address structural and targeted policies to enable SMEs reaching new opportunities in a globalised and digital economy with implications to employment, investment and growth.
2. Literature review
Various studies have investigated SMEs contributions focusing on employment, innovation activity, and economic growth. SMEs have been identified as the prevailing form of enterprise and the most important driver of employment (OECD, Citation2017). Andersson and Noseleit (Citation2011) using longitudinal data for Swedish regions explore the effects of start-ups on employment growth. They report that the sector in which start-ups operate is important and that the positive effect of start-ups on employment change is evident in the same sector in which start-ups operate. The employment effect is either positive or negative when across-sector comparisons are taken into account. What is more, Andersson and Noseleit (Citation2011) reveal that high-tech and service start-ups have negative employment impact on other sectors. The view that SMEs contribute the vital part of employment has also been researched by Haltiwanger, Jarmin, and Miranda (Citation2013). Using data from longitudinal database they control for age of an enterprise and conclude that age of an enterprise is important factor when explaining relationships between SMEs and employment contribution in the U.S. and that young enterprises are driving force of employment. On the other hand, Neumark, Wall, and Zhang (Citation2011) based on the National Establishment Time Series data argue that SMEs are generating net employment, whereas enterprise size negatively affects employment contribution in manufacturing and services sectors. Questioning whether age or size play important role in explaining SMEs employment contribution is present in a study of Lawless (Citation2014). Lawless (Citation2014) finds that there are evident differences in employment contribution regarding size of an enterprise and that smaller enterprises do provide the main source of employment (confirmed also in a study of Dogan, Qamarul Islam, & Yazici, Citation2017), but the driving force of such results is age of an enterprise confirming that younger enterprises are more dynamic than older ones. Similar conclusions can be find in a study of Anderson and Eshima (Citation2013), Huber, Oberhofer, and Pfaffermayr (Citation2017), Heyman, Norbäck, and Persson (Citation2018), Love, Roper, and Zhou (Citation2016), Ayyagari, Demirguc-Kunt, and Maksimovic (Citation2014). On the other hand, the role of innovation on SMEs employment contribution has also been studied by Elejalde, Giuliodori, and Stucchi (Citation2015) who find out that product innovations have positive effect on employment whereas process innovations have not. Due to complexity of innovation activity Audretsch, Coad, and Segarra (Citation2014) propose multidimensional approach when investigating the relation between innovation and employment contributions, whereas Calvino and Virgillito (Citation2018) argue that based on the literature the effect of innovation on employment contributions is not straightforward. Based on panel data study for Italian enterprises Barbieri, Piva, and Vivarelli (Citation2016) report on positive impact of total innovation expenditures on employment, but this positive effect is only evident in high-tech industries and large enterprises, whereas no positive effect on employment is present in SMEs and traditional sectors. Coad, Segarra, and Teruel (Citation2016) have taken into account age of an enterprise when explaining relation between innovation and employment growth. Based on panel quantile regressions they argue that younger Spanish enterprises gain more benefits from innovation activity in terms of employment growth and other performance indicators but their innovation activity tends to be characterised as riskier in comparison to mature enterprises. Moreover, Classen et al. (Citation2014) highlight the significant differences in innovation between family and non-family SMEs where family SMEs have greater readiness to invest in innovation in comparison with non-family SMEs but have lower labour productivity in terms of product and process innovation. As argued by Memili et al. (Citation2015), distinction between family and non-family controlled SMEs regarding their contribution to economic growth can be found important. More specifically, they argue that the number of family and non-family controlled SMEs in the economy should be varied if the economic growth is to be maximised. Additionally, the role of institutional factors in order to promote economic growth is studied by Aparicio, Urbano, and Audretsch (Citation2016). Results show that informal institutions are as important as formal institutions for entrepreneurial activities that present mechanism which adds positively to economic growth. Importance of SMEs in terms of their potential to employment, innovation, economic growth has been synthesised by Du and Temouri (Citation2015) emphasising the role of high-growth firms in achieving productivity growth. Botric (Citation2012) emphasises the role of entrepreneurship as a way out of unemployment, whereas Radovic-Markovic (Citation2014) argues that barriers like low competitiveness, access to finance, and inadequate labour market structure prevent SMEs in Serbia to develop its potential. De Kok et al. (Citation2011) revealed that workers in SMEs tend to receive lower wages than workers in large enterprises. Some of the reasons for such observed differences are (see, for example Butani et al., Citation2006; De Kok et al., Citation2011; Oi and Idson, Citation1999): due to the benefits of specialisation from economies of scale and bigger investment of large enterprises in firm-specific human capital labour productivity increases with size of enterprise; large enterprises have more financial resources which enable them to pay higher wages and in order to prevent from risk-aversion of workers working responsibilities, large enterprises pay higher wages. According to the European Commission (Citation2018) new technology and globalisation also bring the increase in non-standard (atypical) work and such trend has been observed in all EU Member States since 2002 but to different degrees. The occurrence of non-standard work resulted also in higher income volatility and lower job insecurity, which can be more obvious in SMEs than in large enterprises (see, for example Lise, Meghir, & Robin, Citation2016).
3. Methodology
3.1. Data
Our data vector consists of total employment (TEit), SME service sector employment (SMESSit), SME industry sector employment (SMEISit), labour productivity (LPRODit) from the Eurostat database (Eurostat, Citation2018a, Citation2018b, Citation2018c) and gross domestic product (GDP) per capita (GDPPCit) from the OECD.Stat database (OECD, Citation2018). Indication of data sources is presented in .
Table 1. Indication of data sources.
Total employment (TEit) measured in thousand persons is applicable to employees, self-employed persons and family workers and comprises persons aged 15 to 64 years who were in one of the following categories: (1) persons who during the reference week worked for at least one hour for pay or profit or family gain; and (2) persons who were not at work during the reference week but had a job or business from which they were temporarily absent.
To provide insight into which SME sector employment adds more to total EU employment we have additionally decomposed SME employment into SME service sector employment (SMESSit) and into SME industry sector employment (SMEISit). According to the structural business statistics source, SME service sector employment (SMESSit) refers to SME persons employed in economic activities covered by NACE Rev. 2 which are transportation and storage services, accommodation and food service activities, information and communication services, real estate activities, professional, scientific and technical activities, administrative and support service activities, repair of computers and personal and household goods. On the other hand, SME industry sector employment (SMEISit) refers to SME persons employed in economic activities covered by NACE Rev. 2 which are mining and quarrying, manufacturing, electricity, gas, steam and air conditioning supply, water supply, sewerage, waste management and remediation activities. SME persons employed is defined as the total number of persons who work in the SME as well as persons who work outside the SME who belong to SMEs and are paid by SMEs (e.g., sales representatives).
As a control variable, we also included labour productivity (LPRODit) and GDP per capita (GDPPCit). Labour productivity (LPRODit) measures the amount of goods and services produced relative either to the number of persons employed or to the number of hours worked. We have calculated labour productivity per hour worked as real output per unit of labour input which is measured by the total number of hours worked. Calculating labour productivity per hour worked instead of per persons employed is motivated by the fact that the former provides better picture of productivity developments in the economy as it eliminates differences in the full time/part time employment. In order to control for macroeconomic environment, we included GDP per capita (GDPPCit) in U.S. dollars (USD) at Purchasing Power Parity (PPP). GDP per capita (GDPPCit) is a measure for the level of economic development, while GDP itself measures the economic activity and is defined as the value of goods and services produced less the value of any goods and services used in their creation (see, for example. Blanchard, Amighini, & Giavazzi, Citation2010; Dornbusch, Fischer, & Startz, Citation2010; Mankiw, Citation2016).
The time period of data is 2005–2016 for the EU-28 Member States. Our data set therefore contains both dimensions: cross-section dimension and time series/period dimension therefore offering large number of data points and as such improving the efficiency of econometric estimates. For each cross-section dimension we have the same number of time series observations, therefore our panel is balanced. On top of that, such structure of data enable researcher other benefits from the use of panel data as for example the researcher can address broader range of issues and tackle more complex problems, power of the test can be increased and additional variation introduced by combining cross-section and time series/period dimension data as well the impact of certain forms of omitted variables bias can be removed (see, for example Baltagi, Citation2015; Kennedy, Citation2008). The vast majority of data were obtained from the two main Eurostat statistical sources of data namely structural business statistics and labour force survey (Eurostat, Citation2018d) except the data for the GDP which was obtained from the OECDStat database.
3.2. Panel data approach
This research applies panel data regression models which are one of the modern econometric approaches when facing with panel data sets. There are two main panel data models to be discussed namely the fixed effects model (FE) and the random effects model (RE).
In the FE model the intercepts are different for different cross-sectional individuals whereas slope coefficients are assumed to be constant for all individuals. So called individual heterogeneity which relates to all behavioural differences between individuals is assumed to be captured by the intercept term. Individual intercepts are included to control for individual-specific, time-invariant characteristics which do not change over time. The FE model can be written as:
Yit is the dependent variable, αi represents the unknown intercepts to be estimated one per individual, xit is a k x 1 vector of independent variables, eit is the regression random error (see, for example Baltagi, Citation2009; Hill, Griffiths, & Lim, Citation2012; Stock & Watson, Citation2015).
Furthermore, in the RE model it is again assumed that all individual differences are captured by the intercept terms, but one recognises that individuals were randomly selected, so the individual differences are treated as random. Due to random individual differences the error term in the RE model (εit) consist of random individual effect (ui) and the usual regression random error (eit), so εit = ui + eit. The RE model is therefore often called an error components model (see, for example Baltagi, Citation2009; Hill, Griffiths, & Lim, Citation2012; Wooldridge, Citation2010). The RE model can be written as:
Total employment (TEit) was treated as the dependent variable of the panel data regression models, whereas SME service sector employment (SMESSit), SME industry sector employment (SMEISit), labour productivity (LPRODit) and GDP per capita (GDPPCit) were treated as the independent, explanatory/control variables of the panel data regression models. Specification of the basic regression function was the following:
where Ψ and Π are vectors of regression coefficients measuring the association of total employment (TEit) with the explanatory variables (SMESSit, SMEISit) and control variables (LPRODit, GDPPCit), respectfully.
4. Results
Relations between the total employment and SME service sector employment and SME industry sector employment (including control variables) were estimated using both FEs and REs. According to Wooldridge (Citation2010) this prevents biases from different estimation approaches. Analysis was conducted on the panel of the EU-28 Member States during the 2005–2016 period. Estimation results are presented in .
Table 2. Estimation results of the fixed effects and random effects panel regression models.
indicates similar results across FEs and REs confirming robustness of results. Estimation results suggest positive and significant association between SME service sector employment and total employment, whereas association between SME industry sector employment and total employment is not significant. In order to decide between FEs and REs we additionally performed Hausman test where the null hypothesis is that the preferred model is RE vs the alternative which is the FE (see, for example Greene, Citation2012). The estimates of Hausman test showed that FE models are preferred (see ). Therefore, we use FE models for further analysis. We carried out our empirical analysis with the estimation of pooled OLS regression, one way FE with cross-section effects and one way FE with period effects. The estimation results are presented in .
Table 3. Estimation results of robustness tests.
Being faced with different panel data models one has to decide which model is proper and could take into consideration for example the estimates of model fit statistics and others. While estimation of pooled regression model is indeed a simple way to proceed, it has some severe limitations. Most importantly, pooling the data in such a way implicitly assumes that the intercepts are the same for each country and for each year. Having panel data set at disposal, pooling the data would be sub-optimal way to proceed as such approach would not take into account any common variation present in the series over time (see, for example Brooks, Citation2014). In such a way we conclude that the pooled regression model is inappropriate to continue with, therefore we focus on FE models which previously conducted Hausman test confirmed as preferred ones (see ). However, the model selection is justified with the redundant FE test statistics. Test results of the redundant FE test statistics are presented in .
Table 4. Redundant fixed effects test statistics.
The redundant FE test statistics p-value for cross-section effect (p = 0.0000 < α = 0.05) shows that cross-section effects are significant. On the other hand, the redundant FE test statistics p-value for period effect (p = 0.0027 < α = 0.05) shows that period effects is significant as well. Proceeding with the estimation of the FE model including both cross-section and period effects is justified. Following the results of the redundant FE test statistics we proceed with the cross-section and period effects in the panel data regression model specification. Two way FE with cross-section and period effects model specification was also controlled for heteroscedasticity and autocorrelation. We performed modified Wald test for group heteroscedasticity and Wooldridge test for autocorrelation, whereas the presence of heteroscedasticity and autocorrelation was confirmed by aforementioned tests, respectfully. To address the issue and to obtain more reliable estimates we use robust standard errors. Two way FE with cross-section and period effects was estimated (model 6) and the variables SMEIS and LPROD were found insignificant and dropped from the regression model when estimating model 6. In model 6 all parameters are statistically significant and the model fits the data well (F = 17.79, p-value = 0.000 < α = 0.05). The coefficient of determination value (0.8433) shows that SME service sector employment and GDP per capita together explain more than 84% of the total variation of total employment. The estimation results of model 6 are presented in .
Table 5. Estimation results of preferable panel regression model.
5. Discussion and implications
Regression estimates suggest positive association between SME service sector employment and total employment, positive association between GDP per capita and total employment. Regression estimates are statistically significant for all mentioned parameters. Based on regression estimates we can see that the effect of SME industry sector employment was found insignificant and therefore dropped from the regression model highlighting the importance of job-creating SMEs in service sector.
These findings can be explained by a fact that SMEs are predominant in the contingent of all firms and they provide the main source of employment. Furthermore, they are also important for creating values such as they can have effect on GDP and SME development can contribute to economic diversification (OECD, Citation2017).
The research period covers crisis years, which might have biased results. Therefore, labour market performance and empirical results during this period can be effected by real GDP declines.
As argued by European Commission (Citation2016), from 2008 to 2016, the job-creating SMEs were primarily those providing services, while net employment creation was particularly strong from 2008 to 2014 in knowledge intensive services. On the other hand, all of the four types of technology-intensive goods producing sectors (ranging from low, medium-low, medium-high to high technology) showed net job losses between 2008 and 2013. In that period more technology-intensive SMEs lost smaller proportion of their jobs than the SMEs that were more technology-intensive. It is interesting to note that the young SMEs (aged less than 10 years) were the main net employment creators, making together with »older« SMEs stabile labour market.
In 2016, the employment recovery in SMEs was most dynamic in service industries with the reported employment growth in wholesale and retail trade, accommodation and food services’ and in business services (European Commission, Citation2017). SME employment in manufacturing still has not reached before crisis levels. Technology intensive sectors enhanced SME employment performance also in this period clearly indicating where policy makers have to focus. It is worth mentioning that European Commission (Citation2017) policy in relation to SMEs is concentrated in five priority areas such as: the promotion of entrepreneurship and skills, the improvement of SMEs’ access to markets, cutting red tape, the improvement of SMEs’ growth potential, and strengthening dialogue and consultation with SME stakeholders (Eurostat, Citation2018a). The EU is making an effort to support the SMEs growth, which can be also seen through the funds that are oriented on achieving SMEs expansion.
In the programmes from 2007 to 2013, 70 billion euros from European Regional Development and European Social Funds was invested in SMEs support, which helped to create more than 250,000 new jobs in SMEs. The EU initiative can also be seen if we look at Small Business Act (SBA) for Europe (dating from 2008) that applied to all companies that were economically independent, had fewer than 250 employees and an annual turnover of less than 50 million euro and/or a balance sheet total of less than 43 million euro. The SBA included a variety of measures the EU used to strengthen SMEs – from facilitating financing, better access to public procurement procedures, and through to encouraging start-ups by women (European Commission, Citation2013, Citation2014.) The SBA Review, launched in 2011, is a major landmark in tracking the implementation of the SBA with the aim to integrate the SBA with the Europe 2020 Strategy. Consultation with the public regarding the SBA, showed stakeholders’ interest and support what is encouraging for the policymakers.
Till 2020 with European Structural and Investment Funds and European Regional Development Fund, the European Union will further increase its strategic focus on SMEs by enhancing their innovative potential as the key to their competitiveness and growth. Finally, policy makers should also take into consideration new working conditions emerging from new technology and globalisation bringing non-standard work, higher income volatility and lower job stability with more obvious trend in SMEs.
6. Conclusion
This research contributes to investigation whether SME employment affects total EU employment using panel data models. SME employment is decomposed into SME service sector employment and into SME industry sector employment to estimate which of these sectors add more to total EU employment. In the panel data regression models total employment was treated as the dependent variable; SME service sector employment, SME industry sector employment, labour productivity and GDP per capita were treated as independent, control variables of used model.
Regression estimates suggest positive association between SME service sector employment and total employment, positive association between GDP per capita and total employment. Regression estimates are statistically significant for all mentioned parameters. On the other hand, the effect of SME industry sector employment was found insignificant and dropped from the regression model. Based on results it is possible to emphasise the importance of job-creating SMEs in the service sector.
On EU and national levels policy should focus on enhancing SMEs sectors through making enabling macroeconomic environment more friendly for SMEs development, engaging all the key stakeholders in supporting the process, but also highlighting the service sector which showed the most positive results. Simplifying the policy framework should particularly boost the SMEs development. To sum up, enhancing SME employment potential would have positive impacts on economic and social dimension society well-being. More rapid SMEs development could be also beneficial for opportunities in labour markets. SMEs have a main part in achieving innovation potential, enhancing employment rate and creating added value which all effects national growth and development.
Among limitations, it is conducted at the aggregated EU-28 country and two-sectors (industry and services) levels, which provides opportunities for research at disaggregated firm level with using firm-specific variables in the model specifications such as firm age, size, family and non-family ownership, management structures, role of innovation and intangible resources as well as some additional enabling environment variables such as institutional factors and institutional environments. Finally, analysing the situations in every country individually could help to make a proper policy on national/regional levels, which is a good start for further researches.
Disclosure statement
No potential conflict of interest was reported by the authors.
References
- Alpkan, L., Yilmaz, C., & Kaya, N. (2007). Market orientation and planning flexibility in SMEs: Performance implications and an empirical investigation. International Small Business Journal: Researching Entrepreneurship, 25(2), 152–172. doi: 10.1177/0266242607074518
- Anderson, B. S., & Eshima, Y. (2013). The influence of firm age and intangible resources on the relationship between entrepreneurial orientation and firm growth among Japanese SMEs. Journal of Business Venturing, 28(3), 413–429. doi: 10.1016/j.jbusvent.2011.10.001
- Andersson, M., & Noseleit, F. (2011). Start-ups and employment dynamics within and across sectors. Small Business Economics, 36(4), 461–483. doi: 10.1007/s11187-009-9252-0
- Aparicio, S., Urbano, D., & Audretsch, D. (2016). Institutional factors, opportunity entrepreneurship and economic growth: Panel data evidence. Technological Forecasting and Social Change, 102, 45–61. doi: 10.1016/j.techfore.2015.04.006
- Audretsch, D. B., Coad, A., & Segarra, A. (2014). Firm growth and innovation. Small Business Economics, 43(4), 743–749. doi: 10.1007/s11187-014-9560-x
- Ayyagari, M., Demirguc-Kunt, A., & Maksimovic, V. (2014). Who creates jobs in developing countries? Small Business Economics, 43(1), 75–99. doi: 10.1007/s11187-014-9549-5
- Baltagi, B. H. (2009). A companion to econometric analysis of panel data. Chichester, UK: Wiley.
- Baltagi, B. H. (2015). Econometric analysis of panel data. Chichester, UK: Wiley.
- Barbieri, L., Piva, M., & Vivarelli, M. (2016). R&D, embodied technological change and employment: Evidence from Italian microdata. IZA Discussion Paper No. 10354.
- Botric, V. (2012). Regional differences in self-employment: Evidence from Croatia. Economic Research-Ekonomska Istraživanja, 25(sup1), 243–266. doi: 10.1080/1331677X.2012.11517564
- Blanchard, O., Amighini, A., & Giavazzi, F. (2010). Macroeconomics. A European Perspective. Harlow, UK: Pearson.
- Brooks, C. (2014). Introductory econometrics for finance. Cambridge, UK: Cambridge University Press.
- Butani, S. J., Clayton, R. L., Kapani, V., Spletzer, J. R., Talan, D. M., & Werking, G. S. (2006). Business employment dynamics: Tabulations by employer size. Monthly Labour Review, 129(3), 3–22.
- Calvino, F., & Virgillito, M. E. (2018). The innovation-employment nexus: A critical survey of theory and empirics. Journal of Economic Surveys, 32(1), 83–117. doi: 10.1111/joes.12190
- Classen, N., Carree, M., Van Gils, A., & Peters, B. (2014). Innovation in family and non-family SMEs: An exploratory analysis. Small Business Economics, 42(3), 595–609. doi: 10.1007/s11187-013-9490-z
- Coad, A., Segarra, A., & Teruel, M. (2016). Innovation and firm growth: Does firm age play a role? Research Policy, 45(2), 387–400. doi: 10.1016/j.respol.2015.10.015
- De Kok, J., Vroonhof, P., Verhoeven, W., Timmermans, N., Kwaak, T., Snijders, J., & Westhof, F. (2011). Do SMEs create more and better jobs? Zoetermeer: EIM Business and Policy Research.
- Dogan, E., Qamarul Islam, M., & Yazici, M. (2017). Firm size and job creation: Evidence from Turkey. Economic Research-Ekonomska Istraživanja, 30(1), 349–367. doi: 10.1080/1331677X.2017.1305804
- Dornbusch, R., Fischer, S., & Startz, R. (2010). Macroeconomics. Boston, MA: McGraw-Hill.
- Du, J., & Temouri, Y. (2015). High-growth firms and productivity: Evidence from the United Kingdom. Small Business Economics, 44(1), 123–143. doi: 10.1007/s11187-014-9584-2
- Elejalde, R., Giuliodori, D., & Stucchi, R. (2015). Employment and innovation: Firm-level evidence from Argentina. Emerging Markets Finance and Trade, 51(1), 27–47. doi: 10.1080/1540496X.2015.998088
- European Commission. (2016). Annual report on European SMEs (2014/2015).
- European Commission. (2017). Annual report on European SMEs (2017/2018).
- European Commission. (2013). Regional growth for smart growth of SMEs. Brussels: European Commission.
- European Commission. (2014). Regional implementation of the SBA. Belgium: European Commission.
- European Commission. (2018). Employment and Social Developments in Europe. Luxembourg: Publication Office of the European Union.
- Eurostat. (2018a). Small and medium-sized enterprises. Retrieved from https://ec.europa.eu/eurostat/web/structural-business-statistics/structural-business-statistics/sme
- Eurostat. (2018b). Eurostat database. Retrieved from https://ec.europa.eu/eurostat/data/database
- Eurostat. (2018c). Structural business statistics. Retrieved from https://ec.europa.eu/eurostat/cache/metadata/en/sbs_esms.htm
- Eurostat. (2018d). EU labour force survey – Methodology. Retrieved from https://ec.europa.eu/eurostat/statistics-explained/index.php/EU_labour_force_survey_-_methodology
- Greene, W. H. (2012). Econometric Analysis. Harlow, UK: Pearson.
- Haltiwanger, J., Jarmin, R. S., & Miranda, J. (2013). Who creates jobs? Small versus large versus young. Review of Economics and Statistics, 95(2), 347–361. doi: 10.1162/REST_a_00288
- Heyman, F., Norbäck, P. H., & Persson, L. (2018). Who creates jobs and who creates productivity? Small versus large versus young versus old. Economics Letters, 164, 50–57. doi: 10.1016/j.econlet.2017.12.034
- Hill, R. C., Griffiths, W. E., & Lim, G. C. (2012). Principles of econometrics. Hoboken, NJ: Wiley.
- Huber, P., Oberhofer, H., & Pfaffermayr, M. (2017). Who creates jobs? Econometric modeling and evidence for Austrian firm level data. European Economic Review, 91, 57–71. doi: 10.1016/j.euroecorev.2016.09.008
- Kennedy, P. (2008). A guide to econometrics. Oxford, UK: Wiley-Blackwell.
- Krammer, S. M. S., Strange, R., & Lashitew, A. (2018). The export performance of emerging economy firms: The influence of firm capabilities and institutional environments. International Business Review, 27(1), 218–230. doi: 10.1016/j.ibusrev.2017.07.003
- Lawless, M. (2014). Age or size? Contributions to job creation. Small Business Economics, 42(4), 815–830. doi: 10.1007/s11187-013-9513-9
- Lise, J., Meghir, C., & Robin, J. M. (2016). Matching, sorting and wages. Review of Economic Dynamics, 19(1), 63–87. doi: 10.1016/j.red.2015.11.004
- Love, L. H., Roper, S., & Zhou, Y. (2016). Experience, age and exporting performance in UK SMEs. International Business Review, 25(4), 806–819. doi: 10.1016/j.ibusrev.2015.10.001
- Mankiw, N. G. (2016). Macroeconomics. New York, NY: Macmillan.
- Memili, E., Fang, H., Chrisman, J. J., & De Massis, A. (2015). The impact of small- and medium-sized family firms on economic growth. Small Business Economics, 45(4), 771–785. doi: 10.1007/s11187-015-9670-0
- Muller, P., Julius, J., Herr, D., Koch, L., Peycheva, V., & McKiernan, S. (2017). Annual report on European SMEs (2016/2017). Luxembourg: European Commission. doi: 10.2873/742338
- Neumark, D., Wall, B., & Zhang, J. (2011). Do small businesses create more jobs? New evidence for the United States from the national establishment time series. Review of Economics and Statistics, 93(1), 16–29. doi: 10.1162/REST_a_00060
- OECD. (2017). Enhancing the contributions of SMEs in a global and digitalised economy. Paris: OECD.
- OECD. (2018). OECD statistics. Retrieved from https://stats.oecd.org/
- Oi, W. J., & Idson, T. L. (1999). Firm size and wages. In O. C. Ashenfelter & D. Card (Eds.), Handbook of labor economics (Vol. 3, part B, pp. 2165–2214). Amsterdam: North_Holland. doi: 10.1016/S1573-4463(99)30019-5
- Paul, J., Parthasarathy, S., & Gupta, P. (2017). Exporting challenges of SMEs: A review and future research agenda. Journal of World Business, 52(3), 327–342. doi: 10.1016/j.jwb.2017.01.003
- Radovic-Markovic, M. (2014). Unleashing the potential of the small and medium enterprise sector in Serbia. Economic Research-Ekonomska Istraživanja, 27(1), 700–712. doi: 10.1080/1331677X.2014.974341
- Stock, J. H., & Watson, M. W. (2015). Introduction to econometrics. Harlow, UK: Pearson.
- Wooldridge, J. M. (2010). Econometric analysis of cross section and panel data. Cambridge, UK: The MIT Press.