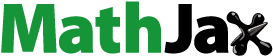
Abstract
This paper explores the time-varying interaction between El Niño phenomenon and the oil market by applying the wavelet analysis. Few studies have explored the time-varying interrelationship between El Niño phenomenon and oil price (also the prices of petroleum products) by considering the time and frequency domains, and this paper will fill the above gaps. The empirical results reveal that El Niño index (NINO) which reflects the strength of El Niño phenomenon has a negative influence on oil price (OP) in the long run, but this view does not hold in the short and medium terms. These results are not consistent with the intertemporal capital asset pricing model (ICAPM), which indicates that there is a positive influence from NINO to OP. In turn, OP positively affects NINO in the medium term. Through comparing the five petroleum products, we can conclude that heating oil price (HOP) is the most relevant to NINO, while gasoline price (GOP) and diesel fuel price (DOP) have relatively weak relationships with it. Understanding the interactions between El Niño phenomenon and the oil market can provide insights for the investors, oil enterprises and related authorities.
1. Introduction
The main purpose of this paper is to investigate the mutual influence between EL Niño phenomenon and the oil market. El Niño phenomenon mainly refers to the abnormally warming of the sea temperature in the tropical oceans of the Eastern and Central Pacific Ocean (Rensch et al., Citation2015). Then, the global climate pattern is changed by El Niño phenomenon (Martin et al., Citation2013; Staupe-Delgado et al., Citation2018; Rojas et al., Citation2019), which affects the demand and supply in the oil market, as well as the fluctuations in its price (Changnon, Citation1999; Cruz & Krausmann, Citation2013; Cashin et al., Citation2017). In general, the occurrence of El Niño phenomenon brings high temperatures (Fan et al., Citation2017), which is reflected in several periods with super El Niño phenomenon (e.g., in 1925–1926, 1997–1998, 2014–2016). As a heating fuel, the occurrences of El Niño phenomena and high temperatures lead the demand for oil and its price to fall. In addition, El Niño phenomenon can cause severe natural disasters, such as droughts, floods and hurricanes (Cane, Citation2004; Alajo et al., Citation2006; Miyakawa et al., Citation2017; Hu & Fedorov, Citation2019). On the one hand, it makes countries be worried about the ecological environment. The governments implement policies to reduce the pollutant emissions which will aggravate the negative impact of El Niño phenomenon, such as the Paris Climate Agreement (Jacobs, Citation2016). The burn and leakage of oil also have a huge negative impact on the global ecology, such as the Gulf of Mexico oil spill in 2010 (Lavrova & Kostianoy, Citation2011). Thus, the decrease of oil consumption is an inevitable measure to achieve sustainable development, which causes oil price to decline. On the other hand, bad weather adversely affects oil exploration, refining and transportation, which leads to a decline in supply and an increase in oil price (Behravesh, Citation2005). For instance, hurricane Harvey has swept across the Gulf Coast in 2017, which forces the refinery in this region to stop production. More importantly, the most intuitive impact of El Niño phenomenon is reflected in the agriculture (Adams et al., Citation2003; Reyes & David, Citation2009; Gelcer et al., Citation2013; Salcedo et al., Citation2019), which results in a decline in the output of some agricultural products (e.g., wheat, white sugar and palm oil). The income of large agricultural production countries (e.g., India and Australia) has decreased, which makes the ability to purchase oil decline. Also, the decrease in agricultural products leads to a reduction in the use of oil in the processing chain, then there is a decline in the demand for oil and its price.
In turn, the development of the global economy is closely related to the oil consumption (Nel & Cooper, Citation2008; Al-Mulali, Citation2011; Saboori et al., Citation2017; Lahiani et al., Citation2019). The rise in oil demand leads to an increase in the carbon dioxide emissions, which causes to the global warming (Ramanathan, Citation2006; Alkhathlan & Javid, Citation2015; Agbanike et al., Citation2019; Kashif & Nimra, Citation2019). Under this condition, the warming of the ocean surface is faster than the subsurface, which may induce the occurrences of El Niño phenomena (Cai et al., Citation2014). The National Oceanic and Atmospheric Management Climate Prediction Center states that global warming makes the extreme weather more severe, including an increase in the frequency of super El Niño phenomenon. Therefore, there may be a close relationship between El Niño phenomenon and oil market. However, after the oil is mined from the ground, it cannot be directly used except for fuel. Thus, in order to provide enough energy support for various demands, oil needs to refine petroleum products with different characteristics (Borenstein et al., Citation1997; Indjehagopian et al., Citation2000; Coetzer et al., Citation2018). Since diverse petroleum products have different characteristics, interactions between their prices and the strength of El Niño phenomenon may have some differences (Fink et al., Citation2010). Then, we choose gasoline, heating oil, diesel fuel, jet fuel and propane to explore the relationships between El Niño phenomenon and petroleum product market. Also, we can conclude which petroleum product price is the most relevant to the El Niño phenomenon. The above explorations can help investors to optimize asset allocation and avoid investment risks, and assist oil enterprises to reduce their costs and control pollutant emissions, as well as provide insights for countries to promote the agricultural stable production, citizen’s personal safety and healthy economic development.
There are several marginal contributions to this paper. Firstly, most of the previous studies are based on the theoretical analysis and event research method to explore the impacts of El Niño phenomenon to the oil market. They hardly consider the quantitative analyses and the different strengths of various El Niño phenomena, so we choose the El Niño index developed by U.S. National Weather Service to conduct our analysis. Secondly, previous studies mostly explore the influences from El Niño phenomenon to oil price or vice versa. However, there are rare studies explore the mutual influence between these two variables. Also, we choose five petroleum products, in order to investigate which kind of price is the most relevant to El Niño phenomenon. The empirical results are not consistent with the hypothesis in the intertemporal capital asset pricing model, which highlights that the occurrence of El Niño phenomenon drives oil price to increase. With this exploration, our results can give insights to investors, they can grasp the trend of oil price based on the El Niño phenomenon, in order to adjust their investment decisions and maintain their wealth. Energy enterprises can redeploy oil production, delivery and disposal strategies to avoid losses from the fluctuations in oil price and reduce pollutant emissions while pursuing profit maximization. Also, these results provide implications for countries to readjust the energy pattern and minimize the adverse impacts of El Niño phenomenon to the oil market, as well as ensure that agricultural production, citizens, national economy and sustainable development are not suffering huge damages. Thirdly, this paper is a pioneering effort to explore the time-varying relationship between the El Niño phenomenon and oil market (also petroleum product market). The traditional methods must ensure that there is no unit root in the time series, which is hard to satisfy in reality. Also, the interaction between these two variables may not be constant, which is ignored by the existing studies. In this paper, we employ the wavelet analysis, which does not limit the stationarity of the selected variables, to improve the accuracy of our results (Roueff & Sachs, Citation2011). By considering the time and frequency domain, we can investigate the time-varying interaction between the El Niño phenomenon and oil market (also petroleum product market).
The structure of the rest of this paper is organized as follows: Section 2 reveals the related studies about this topic. Section 3 introduces the intertemporal capital asset pricing model between El Niño phenomenon and oil market. Section 4 performs the method of wavelet analysis. Section 5 describes the data. Section 6 analyzes the empirical results. Section 7 summarizes the study of this paper.
2. Literature review
The question of whether the EL Niño phenomenon affects the oil market has attracted attention over the past decades. Changnon (Citation1999) reveals that EL Niño phenomenon leads to crop damages and major economic losses, as well as the decline in heating oil demand and its price. Cruz & Krausmann suggest that hurricanes Katrina and Rita which are related to El Niño phenomenon hit the centre of the U.S. petrochemical industry, which leads to the reduction in oil supply and drives its price to increase. This view is also supported by Behravesh (Citation2005). Cruz and Krausmann (Citation2013) indicate that a changing climate and more frequent extreme weather events pose threats to oil extraction, transportation, processing and delivery, which is related to oil price. Cashin et al. (Citation2017) use oil price as a proxy for fuel prices, and point out that the occurrence of El Niño phenomenon has a significant and positive influence to oil price in short term due to the increasing demand from several major economies (e.g., China, the U.S. and the European countries). El Niño phenomenon can cause severe natural disasters (Cane, Citation2004; Alajo et al., Citation2006; Miyakawa et al., Citation2017; Hu & Fedorov, Citation2019), Riza et al. (Citation2018) evidence that the West Texas Intermediate (WTI) oil returns and volatility can be obviously influenced by the rare disaster-risks, which can effectively predict oil price. Rojas et al. (Citation2019) state that EL Niño phenomenon brings potential damages to every sector of the society, such as agriculture, fishery and energy.
In turn, the increasing oil demand leads to high carbon dioxide emissions and global warming (Ramanathan, Citation2006; Alkhathlan & Javid, Citation2015; Agbanike et al., Citation2019; Kashif & Nimra, Citation2019), Collins et al. (Citation2010) prove that under the influence of global warming, the characteristics of El Niño phenomenon will probably be modified. Ciprian-Beniamin and Adrian (Citation2012) suggest that the past and present pattern of oil consumption have negative influences on the environment and climate changes, which may cause EL Niño phenomenon. Cai et al. (Citation2014) present climate modelling to evidence that there is a doubling in the occurrences of the extreme El Niño phenomenon in the future as the response to global warming. Shaeri et al. (Citation2016) indicate that among the non-financial subsectors, airlines has the largest negative oil price risk exposures. This sector may related to the oil demand, which may indirectly affect the occurrences of the EL Niño phenomenon. Salcedo et al. (Citation2019) announce that extreme weather events, such as El Niño phenomenon, influence society through different ways particularly under the condition of global warming. In addition, oil price also has significant impacts on the macroeconomic field (Sodeyfi & Katircioglu, Citation2016; Shaeri & Katircioğlu, Citation2018; Su et al., Citation2019b; Qin et al., Citation2020), but the economic growth is based on the increased consumption of energy (Batrancea et al., Citation2019), which may cause to the changes in the global environment and climate.
The previous studies have mainly investigated a one-way influence from El Niño phenomenon to oil price or vice versa, but few studies explore the interaction between these two variables. Also, the time-varying interrelationship between El Niño phenomenon and oil price by considering the time and frequency domains is hardly identified in the existing studies. Thus, this paper employs the wavelet analysis method to analyze the noisy and complicated time series (Roueff & Sachs, Citation2011; Su et al., Citation2019a; Qin et al., Citation2020). Then, we can obtain the global and local time-frequency relationship between El Niño phenomenon and oil price, as well as the leading and lagging effects of these two variables. More importantly, the different interactions between El Niño phenomenon and the prices of petroleum products are ignored by the existing studies, and this article tries to fill the gaps in previous researches on this topic.
3. The intertemporal capital asset pricing model
Cifarelli and Paladino (Citation2010) develop the intertemporal capital asset pricing model (ICAPM), in order to analyze the influences of systemic risks to asset price, which are represented by EL Nino index (NINO) and oil price (OP) in this paper. There are two assumptions in our theoretical model. First, the oil market has two kinds of investors: informed traders who account for the systemic risk-return and feedback traders who consider the previous OP while investing. Second, the systemic risks cannot be removed by optimizing asset allocation in the oil market, which is represented by NINO, an index to measure the abnormal weather phenomenon. The informed traders will reasonably predict OP according to NINO, and then they decide the amount of oil to store. The oil demand of this group can be obtained by EquationEquation (1)(1)
(1) :
(1)
(1)
where
is the proportion of the oil stored by informed traders at time t.
indicates that its value is positive, and
reveals that the increase in NINO will lead
to rising.
is the return of oil, which means that there is no El Niño phenomenon occurred. At time t-1, the conditional expectation of OP can be represented by
is an ex-post OP at time t. If oil market only has informed traders, the value of
is 1, then EquationEquation (1)
(1)
(1) can be rewritten as EquationEquation (2)
(2)
(2) , which is actually the standard capital asset pricing model (Sharpe, Citation1964). Thus, we can conclude that the increase in NINO will drive OP to rise, and vice versa. Also, the occurrence of El Niño phenomenon affects the oil market.
(2)
(2)
Then, we consider feedback traders, another group of oil investors. They will determine the amount of oil to invest currently by considering the previous OP, and their proportion of the oil demand () can be written as EquationEquation (3)
(3)
(3) :
(3)
(3)
where
indicates that if the change of OP at time t-1 is positive (or negative), the oil demand of feedback traders will increase (or decrease) at time t.
if there are both informed and feedback traders in the oil market. Then, EquationEquation (1)
(1)
(1) can be rewritten as follow:
(4)
(4)
By comparing to EquationEquation (2)(2)
(2) , EquationEquation (4)
(4)
(4) adds a new term:
which indicates that the behaviour of feedback traders will have some influences on the oil market. The coefficient of
is
and it is a positive value since
Therefore, there is a positive influence from NINO to OP, whether there are feedback traders in the oil market or not. The rise in NINO indicates the occurrence of the El Niño phenomenon, and OP will increase in order to compensate for the systemic risk of the abnormal weather, and vice versa. From the ICAPM, we can conclude that the El Niño phenomenon will have certain influences on the oil market, NINO and OP move in the same direction.
4. Wavelet analysis
The Fourier analysis is the basis of the modern time-frequency approach, which is the origin of the wavelet analysis. The wavelet method has two advantages over the Fourier analysis: it can apply natural local analysis (Aguiar-Conraria & Soares, Citation2011b) and include an estimation of spectral properties as a function of time (Aguiar-Conraria et al., Citation2008). In addition, the wavelet analysis can investigate the time-varying interactions between the selected variables, which based on the results from the time and frequency domain. There exist two types of wavelets, discrete wavelet transform (DWT) and continuous wavelet transform (CWT). DWT is appropriate to the de-noising and decomposing time series and mostly applied to analyze the economic variables (Crowley, Citation2007). However, CWT is useful for extracting information and detecting the self-similarity and appropriates to analyze the time series with noisy and complicated. Furthermore, we explore the relationship between NINO (not an economic variable) and OP (not a de-noising time series), so CWT is more suitable than DWT in this paper (Aguiar-Conraria & Soares, Citation2011a, Aguiar-Conraria & Soares, Citation2011b). In fact, most of the variables (e.g., OP) have unit roots, but CWT needs not perform the unit root and cointegration tests. Thus, the wavelet analysis can accurately investigate the mutual influences between the non-stationary variables (Roueff & Sachs, Citation2011).
3.1. The continuous wavelet transform (CWT)
We consider a time series which can be obtained by EquationEquation (5)
(5)
(5) :
(5)
(5)
where
should satisfy the so-called admissibility condition, that is
and
is the Fourier transform of a specific wavelet
This condition allows the reconstruction of
from the CWT
which can be written as EquationEquation (6)
(6)
(6) :
(6)
(6)
where * means the application of complex conjugation; s and u indicate the scaling and the location parameters, respectively. Moreover, the CWT preserves the power of
which is shown in EquationEquation (7)
(7)
(7) . We use this characteristic to define the wavelet coherence, which can weigh the size of the local interactions between NINO and OP.
(7)
(7)
3.2. Wavelet coherence
Through the cross wavelet transform and power, we can evaluate the wavelet coherency (Torrence & Webster, Citation1999). Following the method of Torrence and Compo (Citation1998), the cross wavelet transform of OP and NINO can be defined as EquationEquation (8)(8)
(8) :
(8)
(8)
where * indicates complex conjugation.
and
mean the CWT of OP and NINO, respectively. The cross wavelet power is
which reveals areas in the time-frequency space where the variables show a high common power. However, the wavelet coherency indicates the region where the variables co-move, and may not have high common power. The squared wavelet coherence coefficient can be written as follow (Torrence & Webster, Citation1999), and S indicates the smoothing operator.
(9)
(9)
3.3. Partial wavelet coherence and phase difference
Due to OP is denominated in the U.S. dollar, which may have effects on the interaction between OP and NINO (Sun et al., Citation2017; Wen et al., Citation2018; Mcleod & Haughton, Citation2018; Anjum, Citation2019). Thus, we choose the U.S. dollar index (USDX) as a control variable, EquationEquation (10)(10)
(10) points out the squared partial wavelet coherency (Aguiar-Conraria & Soares, Citation2014).
(10)
(10)
where
which is obtained by the time and frequency scales. This value close to 1 indicates that the correlation between OP and NINO is strong, and vice versa. The theoretical distribution for the wavelet coherence can not be identified, so we employ Monte Carlo methods to test the significance (Torrence & Compo, Citation1998; Grinsted et al., Citation2004). The phase difference, which can be defined as EquationEquation (11)
(11)
(11) , reveals the leading or lagging relationship between OP and NINO, and the direction of their influence can also be obtained.
(11)
(11)
where
indicates the complex partial wavelet coherency;
and
denote the theoretical and real portion of CWT.
refers to the association between NINO and OP, under the control of USDX. When
NINO and OP move in the same direction. If
OP positively influences NINO; if
NINO negatively impacts OP; and vice versa.
5. Data
In this study, we use monthly data, covering the period from 1997:M1 to 2019:M8, to investigate the influence of El Niño phenomenon on oil market. During the period of 1997–1998, there is a super El Niño phenomenon occurred, which has a significant impact on the tropical atmospheric circulation and subsequently affects the changes in the atmospheric circulation at the middle and high latitudes. Since the abnormal changes in these atmospheric circulations, global climate anomalies have occurred, and the temperature has risen sharply, which sets an average temperature record. In 1997, the global average temperature is 14.46 °C, which is 0.37 °C higher than the 30-year average temperature from 1961 to 1990. We choose the EL Niño index (NINO) to represent the strength of the El Niño phenomenon, which is compiled by the U.S. National Weather Service. The higher NINO means the El Niño phenomenon is stronger and severer, and vice versa. Affected by the insufficient demand, international oil price has plummeted in 1997. We use the West Texas Intermediate (WTI) crude oil price (OP) to reflect the international oil market (Chiroma et al., Citation2015), which is published by the U.S. Energy Information Administration. Then, gasoline price (GOP), heating oil price (HOP), diesel fuel price (DOP), jet fuel price (JOP) and propane price (POP) are also applied in this paper, in order to explore the relationships between these prices and NINO. In 2019, NINO has experienced cliff-like fluctuations, which may affect the demand for oil and OP (also GOP, HOP, DOP, JOP and POP). Thus, we can believe that EL Niño phenomenon may have some interactions with oil market, and we use NINO and OP to investigate whether the occurrence of El Niño phenomenon is always accompanied by a fluctuation in the prices of oil. Also, we can explore which kind of petroleum product price (GOP, HOP, DOP, JOP or POP) is the most relevant to NINO.
indicates the trends of NINO, OP, GOP, HOP, DOP, JOP and POP. In general, when the El Niño phenomenon occurs, the change in demand for oil and OP is a long-term process. Therefore, the negative impact of increasing NINO on OP is shown in the long-term, while there may be a positive relationship in the short and medium terms. This view is reflected in several periods with high NINO, such as 1997–1998, 2002–2003, 2006–2007, 2015–2016. Similarly, after the end of El Niño phenomenon, NINO decreases but OP may have certain inertia and will not immediately change in the opposite direction. Hence, the influence of decreasing NINO to OP is also a long-term process. However, there are differences in the effects of NINO on OP. Although NINO in 2007–2008 is lower than 1997–1998, the degree of the decrease in OP is even higher. Therefore, the interaction between El Niño phenomenon and oil market is complicated and time-varying. Since OP is denominated in the U.S. dollar, which has a negative influence on the prices of oil. The depreciation of the U.S. dollar (e.g. U.S. quantitative easing policies) will drive OP to increase, while an appreciation of it (e.g. U.S. interest rates hikes) may cause to the decline in OP. Therefore, the value of the U.S. dollar may affect the relationship between NINO and OP. In order to ensure the accuracy and responsibility of the results, we choose the USDX as a control variable. For different petroleum products, the trends of GOP, HOP, DOP, JOP and POP are similar to OP, but the fluctuation of POP is smaller than others. In addition, the relationships between the prices of petroleum products and NINO also change with time. Then, we employ the wavelet analysis to capture the mutual influences between NINO and OP (also GOP, HOP, DOP, JOP and POP) by considering the time and frequency domain.
6. Empirical results
presents descriptive statistics. The means of NINO, OP, GOP, HOP, DOP, JOP and POP indicate that their series is concentrated at the -0.013, 56.051, 1.610, 1.636, 1.750, 1.655 and 0.785, respectively. The standard deviation indicates the fluctuation of time series, and OP has the largest volatility, followed by NINO, and the smallest is POP. The positive skewness shows that these seven variables are right-skewed distribution. The kurtosis of NINO is more than 3, thereby demonstrating leptokurtic distributions. Meanwhile, OP, GOP, HOP, DOP, JOP and POP satisfy the platykurtic distributions, since the kurtoses are less than 3Footnote1. Moreover, the Jarque-Bera test evidence that these seven variables are significantly non-normally distributed at 1% level.
Table 1. Descriptive statistics for NINO, OP, GOP, HOP, DOP, JOP and POP.
highlights the partial wavelet coherency and the phase difference between NINO and OP. In the long term, NINO negatively leads OP in 1997–2016, with an average partial wavelet coherency of 0.8. There are five strong El Niño phenomena with high NINO occurred during this period, which can be observed in . In 1997–1998, the super El Niño phenomenon, which has lasted for 14 months (1997:M4-1998:M5), leads to the highest global average temperature ever. Also, it affects the global climate pattern (e.g., South America and East Africa have heavy rains and floods, South Africa and Central America have experienced high temperature and drought), which causes more than 20,000 deaths and $34 billion economic losses (Cane, Citation2004; Miyakawa et al., Citation2017). The El Niño phenomenon in 2002–2003 causes the global average temperature higher than that in 1999–2001. During this period, there are lots of disasters around the world (Alajo et al., Citation2006). For instance, Australia has experienced the worst drought ever, with a sharp decline in wheat production and its processed products, which has a negative effect on the economy. In 2006–2007, the global average temperature is also at a high level, which causes extreme weather and low agricultural output (e.g., wheat, white sugar and palm oil). In 2009–2010, except for the significant increase in temperature in 2009–2010 compared to 2008, this El Niño phenomenon also triggers global climate anomalies (e.g., storms in the U.K. and persistent droughts in Africa and Asia). In addition, droughts in Bolivia, Argentina, Northern China and inland in South America, have a major impact on food and water supply, also on the global supply and demand for agricultural products. In 2014–2016, the global average temperature reaches new high during the period of this supper El Niño phenomenon. In the winter of 2015, the eastern part of North America is unusually warm, Brazil suffers the worst drought ever and Central South America experiences the strongest rainfall for decades. In 2016, Australia and Southeast Asia (e.g. Thailand and Vietnam) experience severe heat and drought (Hu & Fedorov, Citation2019). From the above phenomena, we can sum up the three main effects of the El Niño phenomenon during the period of 1997–2016: the rise in global temperature, frequent natural disasters and agricultural crises (Trenberth et al., Citation2002; Reyes & David, Citation2009; Shultz et al., Citation2014; Staupe-Delgado et al., Citation2018). Thus, there are three transmission paths to explain the negative influence of NINO to OP. Firstly, the El Niño phenomenon causes global temperature to increase, which makes winter warmer and reduces the use of fuel for heating. As an important fuel in the heating system, the demand for oil decreases, which in turn causes OP to fall. Secondly, the El Niño phenomenon has led to frequent climate disasters, which results in human deaths and huge economic losses (Ogallo, Citation2010; Yeh et al., Citation2009). The combustion of fossil energy produces carbon dioxide emissions (Ramanathan, Citation2006; Alkhathlan & Javid, Citation2015; Agbanike et al., Citation2019; Kashif & Nimra, Citation2019), which worsens the negative impact of the El Niño phenomenon. In order to protect the ecological environment and maintain sustainable development, most countries have taken measures to reduce carbon dioxide emissions and fossil energy use (e.g., the Paris Climate Conference has reached an agreement to control the carbon dioxide emissions). Coupled with the rapid increase in the consumption of clean energy, the demand for oil and OP are falling (Obama, Citation2017). Thirdly, the agricultural crises caused by El Niño phenomenon have two way to impact OP. On the one hand, the decline in income of large agricultural producing countries (e.g., India) leads to a decrease in the purchasing power of oil (Collier et al., Citation2009). On the other hand, the decline in agricultural production has led to a reduction in the oil demand for its processed products, which also causes OP to fall. Although the El Niño phenomenon may hinder oil extraction which affects oil supply, it does not obviously reflect in the long term. The impact of NINO on oil demand is a long-term process, and this influence is generated through the above transmission paths. Thus, in the long run, the negative impact of NINO on OP depends more on the demand side. In turn, the mechanism of the impact of low NINO on OP can be similarly explained. Therefore, NINO has a negative influence on OP in the long term during the period of 1997–2016. This result is not supported by the ICAPM, which points out that high NINO leads OP to increase.
Figure 2. The partial wavelet coherency and the phase difference between NINO and OP.
Note: (a) presents the partial wavelet coherency between NINO and OP, with USDX as a control variable; and (b) - (c) present the partial phase difference between NINO and OP. The black contour presents the 5% significance level which is obtained by applying the Monte-Carlo-simulations with the iterations of 10000. On the right side, the colour bar evidences the strength of the correlation.
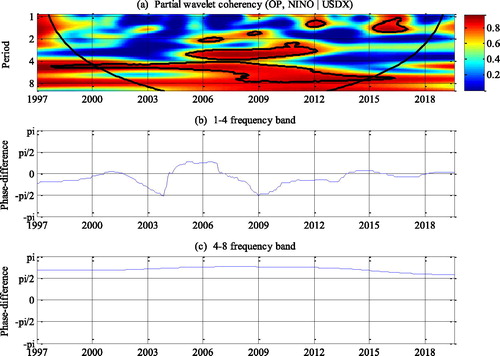
In the medium term, OP positively affects NINO in 2005–2006, and NINO positively leads OP in 2007–2011, with an average partial wavelet coherency of 0.8. In 2005, hurricane Katrina has reduced oil production in the Gulf of Mexico, which causes the decrease in oil supply (Behravesh, Citation2005). More than 8% of the gross world product (GWP) growth is inseparable from the contribution of oil consumption, which leads the demand for oil to rise (Nel & Cooper, Citation2008; Al-Mulali, Citation2011; Saboori et al., Citation2017; Lahiani et al., Citation2019). Then, OP increases due to the effects of supply and demand sides. Also, the rise in oil demand has led to an increase in carbon dioxide emissions (Ramanathan, Citation2006; Alkhathlan & Javid, Citation2015; Agbanike et al., Citation2019; Kashif & Nimra, Citation2019), which is one of the important reasons to exacerbate the El Niño phenomenon, then it makes NINO higher. Therefore, the positive influence from OP to NINO in the medium term during the period of 2005–2006 can be proved. There are three reasons to explain the positive effect of 2007–2011. Firstly, through the demand side, the negative impact of NINO on OP is reflected in the long-term, but it does not appear in the medium term. Thus, the effect of NINO on oil demand is a long-term process, and this entire conduction process takes a long time. Secondly, the price of oil has inertia (An et al., Citation2014), that is when NINO rises or falls, OP does not change immediately. After the end of El Niño phenomenon, NINO decreases and OP continues to move with the previous trend, so there may be a movement in the same direction between NINO and OP in the medium term. Thirdly, the outbreak of the global financial crisis has caused the value of the U.S. dollar to fluctuate (Rose & Spiegel, Citation2012). Since OP is denominated in U.S. dollar, OP presents an opposite trend of USDX (Sun et al., Citation2017; Wen et al., Citation2018; Mcleod & Haughton, Citation2018; Anjum, Citation2019). In addition, the Gulf of Mexico oil spill has caused a decline in oil supply, which further drives OP to increase. After this global financial crisis, U.S. has implemented the quantitative easing policy to revive the economy, which causes the fall in USDX and the rise in OP. Then, as the changes of U.S. dollar value, the fluctuations of OP may just be in the same direction with NINO in the medium term. Therefore, the positive effect of NINO on OP in the medium term during the period of 2007–2011 can be evidenced.
In the short term, NINO positively leads OP in 2015–2016, with an average partial wavelet coherency of 0.8. The positive influence from NINO to OP in 2015–2016 can also be explained by three reasons of medium-term (2007–2011): the negative impact of NINO on OP is not fully reflected in the short term, the characteristic of inertia in OP and the negative linkage between USDX and OP. Furthermore, the El Niño phenomenon has caused global climate turmoil (Ogallo, Citation2010; Yeh et al., Citation2009), which is also associated with the Atlantic and Pacific hurricane seasons in 2015. Hurricanes threaten the refinery facilities and pipeline systems, then weaken the supply of oil and drive OP to increase. Through the supply side, the positive impact of NINO on OP can be observed in the short term, while the influence from the demand side does not reflect completely. Therefore, we can prove that NINO has a positive impact on OP in the short term during the period of 2015–2016.
In order to clearly compare the interactions between the El Niño phenomenon and petroleum products, reveals the time-varying relationships between NINO and OP, GOP, HOP, DOP, JOP, POP and establish the partial wavelet coherency and the phase difference. Since petroleum products are made from crude oil, their relationships with NINO have some similarities to . However, as the various features, the differences between them can also be obviously observed. HOP has the strongest correlation with NINO, which can be explained in two ways. On the one hand, heating oil is a refined oil that is commonly used to heat buildings and keep warm in winter. The El Niño phenomenon causes a rise in global temperature, which means a warm winter (Trenberth et al., Citation2002; Shultz et al., Citation2014). Then, the decrease in heating oil demand leads HOP to fall. On the other hand, heating with oil will increase carbon dioxide emissions (Ramanathan, Citation2006; Alkhathlan & Javid, Citation2015; Agbanike et al., Citation2019; Kashif & Nimra, Citation2019), which increases the negative impact of the El Niño phenomenon. In order to reduce environmental pollution, heating oil has gradually replaced by clean energy, so the demand and HOP decrease (Obama, Citation2017). Therefore, the heating capacity promotes HOP to have closest relationship with NINO. JOP is another variable that is highly correlated with NINO. Jet fuel can meet the requirements of oil flow in cold and low-temperature areas and high-altitude flights, and it can be used for the aircraft engines (Coetzer et al., Citation2018). The El Niño phenomenon makes the anomalous climate increase, the bad weather is not conducive to aircraft flight (Theodore & Caracena, Citation1977). Thus, the demand for jet fuel drops significantly, which causes JOP to decline. Also, the aircraft has a huge fuel consumption and emits exhaust gas at high altitudes which pollutes the environment (Moore et al., Citation2015). The replacement of the traditional jet fuel with bio-aviation kerosene is an inevitable trend for sustainable development, which further leads to a fall in JOP. Thus, the characteristic of fueling the aircraft makes the relationship between NINO and JOP be close. The consumption of clean energy can reduce environmental pollution, and it is an important measure to solve the global ecological problems (Obama, Citation2017). As a clean fuel, in recent years, the role of propane in engines, barbecue foods and home heating systems has become more prominent. The El Niño phenomenon makes the weather warmer, which has a significant impact on POP recently. Therefore, the clean feature makes this negative effect from NINO to POP continues until 2017. Since gasoline and diesel fuel are the main fuels for automobile engines, and they do not have the above three properties: heating capacity, fueling the aircraft and clean feature. Therefore, the relationship between NINO and GOP (also, NINO and DOP) is relatively weak. However, after Donald J. Trump becomes the U.S. President, the relationships between NINO and OP (also GOP, HOP, DOP, JOP and POP) are not significant. The U.S. has withdrawn from the Paris Climate Agreement, which has made the U.S. no longer targets carbon reduction, and the demand for fossil fuels increases (Bordoff, Citation2017). The success of the U.S. shale oil revolution has accelerated the growth of oil supply and demand (Kilian, Citation2016), which means that oil consumption is less affected by the fluctuations in NINO. In addition, U.S. sanctions against Iran and the control of oil production in Saudi Arabia have caused OP to fluctuate. Since the U.S. has launched global trade wars, even if El Niño phenomenon affects agricultural output, its prices are more affected by trade factors. Therefore, after Trump becomes the U.S. President, NINO and OP no longer have significant interaction.
Figure 3. The partial wavelet coherency and the phase difference between NINO and GOP, HOP, DOP, JOP, POP.
Note: (a) presents the partial wavelet coherency between NINO and the prices of petroleum products (GOP, HOP, DOP, JOP and POP), with USDX as a control variable; and (b) - (c) present the partial phase difference between NINO and the prices of petroleum products. The black contour presents the 5% significance level which is obtained by applying the Monte-Carlo-simulations with the iterations of 10000. On the right side, the colour bar evidences the strength of the correlation.
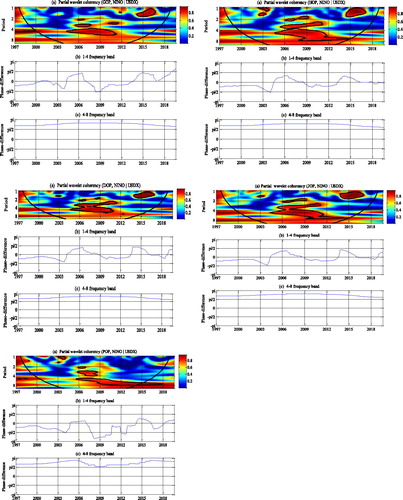
Table 2. Relationships between NINO and OP, GOP, HOP, DOP, JOP, POP.
To sum up, it can be observed from the trends of NINO, OP, GOP, HOP, DOP, JOP and POP, the relationship between the El Niño phenomenon and the oil market is not static. The wavelet analysis is applied to obtain the time-varying relationship between NINO and OP (also GOP, HOP, DOP, JOP and POP) by considering the time and frequency domain. NINO has a negative influence on OP in the long term, which is mainly caused by changes in oil demand. However, there is a positive effect of NINO to OP in the short and medium terms, which can be explained from the impact of demand-side is reflected in the long term, the characteristic of inertia in OP and the negative relationship between USDX and OP, as well as the effect from supply aspect. These results are not consistent with the ICAPM, which highlights that NINO has a positive influence on OP. In turn, OP has a positive impact on NINO, since the carbon dioxide emissions from oil consumption is an important contributor to deteriorate the negative effects of the El Niño phenomenon. By comparing the five petroleum products, we can observe that HOP is the most relevant to NINO due to the heating capacity. Also, JOP and POP are highly related to NINO as fueling the aircraft and having clean feature, while GOP and DOP have relatively weak relationships with NINO. After Trump becomes the U.S. President, the mutual influence between NINO and OP (also GOP, HOP, DOP, JOP and POP) is not significant. Through the above results, we can evidence that NINO has an impact on OP but not always established, and various petroleum products have different interactions with NINO.
6. Conclusion
This paper investigates the interaction mechanism between the El Niño phenomenon and oil market, in order to solve the problem whether NINO matters for OP and which kind of petroleum product price (GOP, HOP, DOP, JOP and POP) has the closest relationship with NINO. Since the features in different variables are various, we apply the wavelet analysis to evidence the time-varying relationship between NINO and OP (also GOP, HOP, DOP, JOP and POP). In the long term, NINO has a negative influence on OP, but this view does not hold in the short and medium terms. These results are not supported by the ICAPM, which states that there is a positive impact from NINO to OP. In turn, OP has a positive influence on NINO, since the carbon dioxide emissions from oil consumption can exacerbate the El Niño phenomenon. By comparing the five petroleum products, we can conclude that HOP is the most relevant to NINO due to the heating capacity, and JOP and POP are also highly related to it, while GOP and DOP have relatively weak relationships with it. However, after Trump becomes the U.S. President, the interaction between NINO and OP (also GOP, HOP, DOP, JOP and POP) can not be obviously observed.
Under the background of the significant relationship between the El Niño phenomenon and oil market, investors, energy enterprises and authorities can learn from this interaction to predict the trend of OP and avoid losses caused by El Niño phenomenon. The effects of NINO to OP are negative in the long run, and positive in the short and medium terms. Investors can predict the trend of OP based on the NINO. Then, they can adjust their investment decisions and optimize asset allocation, sell oil-related products in the long run and buy them in the short and medium terms, in order to avoid risks and maintain wealth. Energy enterprises can redeploy oil production, delivery and disposal strategies, in order to avoid losses from the fluctuations in OP and maximize their profits. Authorities need to readjust the energy pattern and take protective measures timely, as well as minimize the adverse impacts of NINO to the oil market, in order to avoid the public panic and stabilize the national economy. The positive impact from OP to NINO means that the carbon dioxide emissions from oil consumption deteriorate the negative effects of the El Niño phenomenon. Energy enterprises should reduce pollutant emissions while pursuing profit maximization, which can reduce the damages to the ecological environment. At the same time, the protective measures must be issued in advance to prevent the damages of refinery facilities and pipeline systems, as well as reduce the enterprises’ costs. Related authorities should encourage the research and development of new energy, reduce carbon dioxide emissions and promote global sustainable development. Also, when NINO is expected to be high, preventive measures should be taken in advance to ensure that agricultural production, citizens and national economy are not subject to huge losses. Since different petroleum products have various relationships with NINO, they must pay attention and make new investment decisions, enterprise development plans and national energy policies to achieve the decentralized investments, efficient operation and stable oil market. Although the relationship between NINO and OP is not significant after Trump becomes the U.S. President, El Niño phenomenon is also an important factor to oil market which cannot be ignored. In the future researches, we will explore which factors (economic, political or natural) has the best influence on OP. Moreover, we will predict OP more accurately by considering the above factors, thereby new predictive models (e.g., generalized autoregressive conditional heteroskedast-mixed data sampling model) should be taken into account.
Disclosure statement
No potential conflict of interest was reported by the author(s).
Notes
1 The leptokurtic distribution shows a much higher peak around the mean value, and fat tails, or higher densities of values at the extreme ends of the probability curve. The platykurtic distribution is exactly the opposite.
References
- Adams, R. M., Houston, L. L., Mccarl, B. A., Tiscareño, M. L., Matus, J. G., & Weiher, R. F. (2003). The benefits to Mexican agriculture of an El Niño-Southern Oscillation (ENSO) early warning system. Agricultural and Forest Meteorology, 115(3–4), 183–194. doi:10.1016/S0168-1923(02)00201-0
- Agbanike, T. F., Nwani, C., Uwazie, U. I., Anochiwa, L. I., Onoja, T. C., & Ogbonnaya, I. O. (2019). Oil price, energy consumption and carbon dioxide (CO2) emissions: Insight into sustainability challenges in Venezuela. Latin American Economic Review, 28(1), 1–26. doi:10.1186/s40503-019-0070-8
- Aguiar-Conraria, L., Azevedo, N., & Soares, M. J. (2008). Using wavelets to decompose the time-frequency effects of monetary policy. Physica A: Statistical Mechanics and Its Applications, 387(12), 2863–2878. doi:10.1016/j.physa.2008.01.063
- Aguiar-Conraria, L., & Soares, M. J. (2011a). Business cycle synchronization and the Euro: A wavelet analysis. Journal of Macroeconomics, 33(3), 477–489. doi:10.1016/j.jmacro.2011.02.005
- Aguiar-Conraria, L., & Soares, M. J. (2011b). Oil and the macroeconomy: Using wavelets to analyze old issues. Empirical Economics, 40(3), 645–655. doi:10.1007/s00181-010-0371-x
- Aguiar-Conraria, L., & Soares, M. J. (2014). The continuous wavelet transform: Moving beyond uni- and bivariate analysis. Journal of Economic Surveys, 28(2), 344–375. doi:10.1111/joes.12012
- Alajo, S. O., Nakavuma, J., & Erume, J. (2006). Cholera in endemic districts in Uganda during El Niño rains: 2002–2003. African Health Sciences, 6(22), 93–97.
- Alkhathlan, K., & Javid, M. (2015). Carbon emissions and oil consumption in Saudi Arabia. Renewable and Sustainable Energy Reviews, 48, 105–111. doi:10.1016/j.rser.2015.03.072
- Al-Mulali, U. (2011). Oil cnsumption, CO2 emission and economic growth in MENA countries. Energy, 36(10), 6165–6171. doi:10.1016/j.energy.2011.07.048
- An, H., Gao, X., Wei, F., Xuan, H., & Ding, Y. H. (2014). The role of fluctuating modes of autocorrelation in crude oil prices. Physica A: Statistical Mechanics and Its Applications, 393, 382–390. doi:10.1016/j.physa.2013.08.055
- Anjum, H. (2019). Estimating volatility transmission between oil prices and the US dollar exchange rate under structural breaks. Journal of Economics and Finance, 43(4), 750–714. doi:10.1007/s12197-019-09472-w
- Batrancea, I., Batrancea, L., Nichita, A., Gaban, L., Morar, I. D., Masca, E., Fatacean, G., & Moscviciov, A. (2019). An econometric approach on production, costs and profit in Romanian coal mining enterprises. Economic Research-Ekonomska Istraživanja, 32(1), 1019–1036. doi:10.1080/1331677X.2019.1595080
- Behravesh, N. (2005). Impact on U.S. economy of hurricane Katrina. Fairfield County Business Journal, 44(38), 30.
- Bordoff, J. (2017). Withdrawing from the Paris Climate Agreement hurts the US. Nature Energy, 2(9), 17145. doi:10.1038/nenergy.2017.145
- Borenstein, S., Cameron, A. C., & Gilbert, R. (1997). Do gasoline prices respond asymmetrically to crude oil price changes? Quarterly Journal of Economics, 112(1), 305–339. doi:10.1162/003355397555118
- Cai, W. J., Borlace, S., Lengaigne, M., Rensch, P. V., Collins, M., Vecchi, G., Timmermann, A., Santoso, A., McPhaden, M. J., Wu, L. X., England, M. H., Wang, G. J., Guilyardi, E., & Jin, F. F. (2014). Increasing frequency of extreme El Niño events due to greenhouse warming. Nature Climate Change, 4(2), 111–116. doi:10.1038/nclimate2100
- Cane, M. A. (2004). El Nino in history: Storming through the ages. Journal of World History, 15(1), 87–88. doi:10.1353/jwh.2004.0004
- Cashin, P., Mohaddes, K., & Raissi, M. (2017). Fair weather or foul? The macroeconomic effects of El Niño. Journal of International Economics, 106, 37–54. doi:10.1016/j.jinteco.2017.01.010
- Changnon, S. A. (1999). Impacts of 1997—98 El Niño generated weather in the United States. Bulletin of the American Meteorological Society, 80(9), 1819–1827. doi:10.1175/1520-0477(1999)080<1819:IOENOG>2.0.CO;2
- Chiroma, H., Abdulkareem, S., & Herawan, T. (2015). Evolutionary neural network model for West Texas intermediate crude oil price prediction. Applied Energy, 142, 266–273. doi:10.1016/j.apenergy.2014.12.045
- Cifarelli, G., & Paladino, G. (2010). Oil price dynamics and speculation: A multivariate financial approach. Energy Economics, 32(2), 363–372. doi:10.1016/j.eneco.2009.08.014
- Ciprian-Beniamin, B., & Adrian, B. (2012). The geopolitics of oil and climate change. Annals of Faculty of Economics, 1(2), 70–74.
- Coetzer, R. L. J., Joubert, T. S., Viljoen, C. L., Nel, R. J. J., & Strydom, C. A. (2018). Response surface models for synthetic jet fuel properties. Applied Petrochemical Research, 8(1), 39–53. doi:10.1007/s13203-018-0196-7
- Collier, B., Skees, J., & Barnett, B. (2009). Weather index insurance and climate change: Opportunities and challenges in lower income countries. The Geneva Papers on Risk and Insurance - Issues and Practice, 34(3), 401–424. doi:10.1057/gpp.2009.11
- Collins, M., An, S. I., Cai, W., Ganachaud, A., Guilyardi, E., Jin, F. F., Jochum, M., Lengaigne, M., Power, S., Timmermann, A., Vecchi, G., & Wittenberg, A. (2010). The impact of global warming on the Tropical Pacific Ocean and El Niño. Nature Geoscience, 3(6), 391–397. doi:10.1038/ngeo868
- Crowley, P. (2007). An intuitive guide to wavelets for economists. Journal of Economic Surveys, 21(2), 207–267. doi:10.1111/j.1467-6419.2006.00502.x
- Cruz, A., & Krausmann, E. (2013). Vulnerability of the oil and gas sector to climate change and extreme weather events. Climatic Change, 121(1), 41–53. doi:10.1007/s10584-013-0891-4
- Fan, J., Meng, J., Ashkenazy, Y., Havlin, S., & Schellnhuber, H. J. (2017). Network analysis reveals strongly localized impacts of El Niño. Proceedings of the National Academy of Sciences, 114(29), 7543–7548. doi:10.1073/pnas.1701214114
- Fink, J. D., Fink, K. E., & Russell, A. (2010). When and how do tropical storms affect markets? The case of refined petroleum. Energy Economics, 32(6), 1283–1290. doi:10.1016/j.eneco.2010.03.007
- Gelcer, E., Fraisse, C., Dzotsi, K., Hu, Z. J., Mendes, R., & Zotarelli, L. (2013). Effects of El Nino Southern Oscillation on the space–time variability of agricultural reference index for drought in Midlatitudes. Agricultural and Forest Meteorology, 174–175, 110–128. doi:10.1016/j.agrformet.2013.02.006
- Grinsted, A., Moore, J. C., & Jevrejeva, S. (2004). Application of the cross wavelet transform and wavelet coherence to geophysical time series. Nonlinear Processes in Geophysics, 11(5–6), 561–566. doi:10.5194/npg-11-561-2004
- Hu, S., & Fedorov, A. V. (2019). The extreme El Niño of 2015–2016: The Role of westerly and easterly wind bursts, and preconditioning by the failed 2014 event. Climate Dynamics, 52(12), 7339–7357. doi:10.1007/s00382-017-3531-2
- Indjehagopian, J. P., Lantz, F., & Simon, V. (2000). Dynamics of heating oil market prices in. Energy Economics, 22(2), 225–252. doi:10.1016/S0140-9883(99)00034-1
- Jacobs, M. (2016). High pressure for low emissions: How civil society created the Paris Climate Agreement. Juncture, 22(4), 314–323. doi:10.1111/j.2050-5876.2016.00881.x
- Kashif, M., & Nimra, R. (2019). Energy consumption and environmental quality in South Asia: Evidence from panel non-linear ARDL. Environmental Science and Pollution Research International, 26, 29307–29315.
- Kilian, L. (2016). The impact of the shale oil revolution on U.S. oil and gasoline prices. Review of Environmental Economics and Policy, 10(2), 185–205. doi:10.1093/reep/rew001
- Lahiani, A., Benkraiem, R., Miloudi, A., & Shahbaz, M. (2019). New evidence on the relationship between crude oil consumption and economic growth in the US: A quantile causality and cointegration approach. Journal of Quantitative Economics, 17(2), 397–420. doi:10.1007/s40953-018-0147-2
- Lavrova, O. Y., & Kostianoy, A. G. (2011). Catastrophic oil spill in the Gulf of Mexico in April–May 2010. Izvestiya, Atmospheric and Oceanic Physics, 47(9), 1114–1118. doi:10.1134/S0001433811090088
- Martin, E. A., Paczuski, M., & Davidsen, J. (2013). Interpretation of link fluctuations in climate networks during El Niño periods. EPL (Europhysics Letters)), 102(4), 48003. doi:10.1209/0295-5075/102/48003
- Mcleod, R. C. D., & Haughton, A. Y. (2018). The value of the US dollar and its impact on oil prices: Evidence from a non-linear asymmetric cointegration approach. Energy Economics, 70, 61–69. doi:10.1016/j.eneco.2017.12.027
- Miyakawa, T., Yashiro, H., Suzuki, T., Tatebe, H., & Satoh, M. (2017). A Madden-Julian Oscillation event remotely accelerates ocean upwelling to abruptly terminate the 1997/1998 super El Niño. Geophysical Research Letters, 44(18), 9489–9495. doi:10.1002/2017GL074683
- Moore, R. H., Shook, M., Beyersdorf, A. J., Corr, C., Herndon, S., Knighton, W. B., Miake-Lye, R., Thornhill, K. L., Winstead, E. L., Yu, Z. H., Ziemba, L. D., & Anderson, B. E. (2015). Influence of jet fuel composition on aircraft engine emissions: A synthesis of aerosol emissions data from the NASA APEX, AAFEX, and ACCESS missions. Energy & Fuels, 29(4), 2591–2600. doi:10.1021/ef502618w
- Nel, W. P., & Cooper, C. J. (2008). A critical review of IEA’s oil demand forecast for China. Energy Policy, 36(3), 1096–1106. doi:10.1016/j.enpol.2007.11.025
- Obama, B. (2017). The irreversible momentum of clean energy. Science (New York, N.Y.), 355(6321), 126–129. doi:10.1126/science.aam6284
- Ogallo, L. (2010). The mainstreaming of climate change and variability information into planning and policy development for Africa. Procedia Environmental Sciences, 1, 405–410. doi:10.1016/j.proenv.2010.09.028
- Qin, M., Su, C. W., Hao, L. N., & Tao, R. (2020). The stability of U.S. economic policy: Does it really matter for oil price? Energy, 198, 117315. doi:10.1016/j.energy.2020.117315
- Ramanathan, R. (2006). A multi-factor efficiency perspective to the relationships among world GDP, energy consumption and carbon dioxide emissions. Technological Forecasting and Social Change, 73(5), 483–494. doi:10.1016/j.techfore.2005.06.012
- Rensch, P., Gallant, A. J. E., Cai, W., & Nicholls, N. (2015). Evidence of local sea surface temperatures overriding the Southeast Australian rainfall response to the 1997–1998 El Niño. Geophysical Research Letters, 42(21), 9449–9456. doi:10.1002/2015GL066319
- Reyes, M. L. F. D., & David, W. P. (2009). The effect of El Niño on rice production in the Philippines. Philippine Agricultural Scientist, 92(2), 170–185.
- Riza, D., Rangan, G., Tahir, S., & Mark, W. E. (2018). Time-varying rare disaster risks, oil returns and volatility. Energy Economics, 75(C), 239–248.
- Rojas, O., Piersante, A., Cumani, M., & Li, Y. Y. (2019). Understanding the drought impact of El Niño/La Niña in the grain production areas in Eastern Europe and Central Asia (World Bank No. 31467). World Bank Publications.
- Rose, A. K., & Spiegel, M. M. (2012). Dollar illiquidity and central bank swap arrangements during the global financial crisis. Journal of International Economics, 88(2), 326–340. doi:10.1016/j.jinteco.2012.02.014
- Roueff, F., & Sachs, R. (2011). Locally stationary long memory estimation. Stochastic Processes and Their Applications, 121(4), 813–844. doi:10.1016/j.spa.2010.12.004
- Saboori, B., Rasoulinezhad, E., & Sung, J. (2017). The nexus of oil consumption, CO2 emissions and economic growth in China, Japan and South Korea. Environmental Science and Pollution Research International, 24(8), 7436–7455. doi:10.1007/s11356-017-8428-4
- Salcedo, D. S. A., Melo-Velandia, L. F., & Parra-Amado, D. (2019). Nonlinear relationship between the weather phenomenon El Niño and Colombian food prices (Working Paper Borradores de Economia 1085). Banco de la Republica de Colombia.
- Shaeri, K., Adaoglu, C., & Katircioglu, S. (2016). Oil price risk exposure: A comparison of financial and non-financial subsectors. Energy, 109, 712–723. doi:10.1016/j.energy.2016.05.028
- Shaeri, K., & Katircioğlu, S. (2018). The nexus between oil prices and stock prices of oil, technology and transportation companies under multiple regime shifts. Economic Research-Ekonomska Istraživanja, 31(1), 681–702. doi:10.1080/1331677X.2018.1426472
- Sharpe, W. F. (1964). Capital asset prices: A theory of market equilibrium under conditions of risk. The Journal of Finance, 19(3), 425–442. doi:10.1111/j.1540-6261.1964.tb02865.x
- Shultz, J. M., Shepherd, J. M., Bagrodia, R., & Espinel, Z. (2014). Tropical cyclones in a year of rising global temperatures and a strengthening El Niño. Disaster Health, 2(3–4), 151–162. doi:10.1080/21665044.2014.1111722
- Sodeyfi, S., & Katircioglu, S. (2016). Interactions between business conditions, economic growth, and crude oil prices. Economic Research-Ekonomska Istraživanja, 29(1), 980–990. doi:10.1080/1331677X.2016.1235504
- Staupe-Delgado, R., Kruke, B. I., Ross, R. J., & Glantz, M. H. (2018). Preparedness for slow-onset environmental disasters: Drawing lessons from three decades of El Niño impacts. Sustainable Development, 26(6), 553–563. doi:10.1002/sd.1719
- Su, C. W., Khan, K., Tao, R., & Nicoleta-Claudia, M. (2019a). Does geopolitical risk strengthen or depress oil prices and financial liquidity? Evidence from Saudi Arabia. Energy, 187, 116003. doi:10.1016/j.energy.2019.116003
- Sun, X., Lu, X., Yue, G., & Li, J. F. (2017). Cross-correlations between the US monetary policy, US dollar index and crude oil market. Physica A Statistical Mechanics & Its Applications, 467, 326–344.
- Su, C. W., Wang, X. Q., Tao, R., & Oana-Ramona, L. (2019b). Do oil prices drive agricultural commodity prices? Further evidence in a global bio-energy context. Energy, 172, 691–701. doi:10.1016/j.energy.2019.02.028
- Theodore, F. T., & Caracena, F. (1977). An analysis of three weather-related aircraft accidents. Bulletin of the American Meteorological Society, 58(11), 1164–1181. doi:10.1175/1520-0477(1977)058<1164:AAOTWR>2.0.CO;2
- Torrence, C., & Compo, G. P. (1998). A practical guide to wavelet analysis. Bulletin of the American Meteorological Society, 79(1), 61–78. doi:10.1175/1520-0477(1998)079<0061:APGTWA>2.0.CO;2
- Torrence, C., & Webster, P. J. (1999). Interdecadal changes in the ENSO-Monsoon System. Journal of Climate, 12(8), 2679–2690. doi:10.1175/1520-0442(1999)012<2679:ICITEM>2.0.CO;2
- Trenberth, K. E., Caron, J. M., Stepaniak, D. P., & Worley, S. (2002). Evolution of El Niño-Southern Oscillation and global atmospheric surface temperatures. Journal of Geophysical Research, 107(D8), 5–1. AAC AAC 5-17. doi:10.1029/2000JD000298
- Wen, F., Xiao, J., Huang, C., & Xia, X. H. (2018). Interaction between oil and US dollar exchange rate: Nonlinear causality, time-varying influence and struactural breaks in volatility. Applied Economics, 50(3), 319–334. doi:10.1080/00036846.2017.1321838
- Yeh, S. W., Kug, J. S., Dewitte, B., Kwon, M. H., Kirtman, B. P., & Jin, F. F. (2009). El Nino in a changing climate. Nature, 461(7263), 511–514. doi:10.1038/nature08316