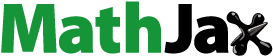
Abstract
Online big data provides large amounts of decision information to decision makers, but supporting and opposing information are present simultaneously. Dual hesitant fuzzy sets (DHFSs) are useful models for exactly expressing the membership degree of both supporting and opposing information in decision making. However, the application of DHFSs requires an improved distance measure. This paper aims to improve distance measure models for DHFSs and apply the new distance models to generate a technique for order preference by similarity to an ideal solution (TOPSIS) method for multiple attribute decision making (MADM).
1. Introduction
Multiple attribute decision making is a general problem of selecting the optimal alternative from a group of alternatives with more than one common attribute. The format of decision information of attributes may vary among cases, including the real number, intervals, intuitionistic fuzzy sets, and hesitant fuzzy sets. With the development of the internet, multiple sources of data can be recorded, distributed, and stored on a network. For one attribute value of one alternative, there is a large amount of supporting and opposing information. DHFSs can be used to exactly express the diversity of supporting and opposing information for MADM. TOPSIS is a classic MADM method based on the distance measure between alternatives and ideal alternatives (Liu, Citation2009; Lan et al., Citation2018; Zeng, Chen, et al., Citation2020; Zeng, Luo, et al., Citation2020). The TOPSIS method has been widely applied in economics and management (Boran et al., Citation2012; Erkayman et al., Citation2011; Kaynak et al., Citation2017; Mandić et al., Citation2017; Mangir & Erdogan, Citation2011; Masca, Citation2017; Önder et al., Citation2015), including in the evaluation of renewable energy technologies (Boran et al., Citation2012), logistics center location selection (Erkayman et al., Citation2011), comparisons of innovation performance for EU candidate countries (Kaynak et al., Citation2017), analyses of the efficiency of insurance companies (Mandić et al., Citation2017), and comparisons of countries' economic performance (Mangir & Erdogan, Citation2011; Masca, Citation2017; Önder et al., Citation2015). When the decision information in MADM is fuzzy sets or other styles, TOPSIS is still useful. Zeng, Chen, et al., (Citation2020), Zeng, Luo, et al., (Citation2020) successfully applied TOPSIS in MADM when the decision information was an interval-valued intuitionistic fuzzy set or single-valued neutrosophic information. When decision information is provided by DHFSs, the distance measure of the DHFS is the key to utilizing TOPSIS to solve MADM with DHFS information. In this paper, the distance measure for DHFSs will be studied and used to generate a TOPSIS method to solve MADM problems.
The concept of fuzzy sets (FSs) was first formalized by Zadeh (Citation1965). Since then, fuzziness has been widely employed to handle a type of uncertainty that is different from probability (Yang & Hussain, Citation2019). The membership function in fuzzy sets shows a membership degree of to
Atanassov (Citation1986) extended the nonmembership degree (i.e.,
does not belong to
) to fuzzy sets and defined intuitionistic fuzzy sets (IFSs). In real-world decision making, when a group of experts is invited to evaluate the membership degree and the nonmembership degree, they tend to argue that it seems impossible in most cases. Under this condition, IFSs cannot be used, especially when experts conduct decisions in an anonymous way. Hesitant fuzzy sets (HFSs) (Torra, Citation2010; Torra & Narukawa, Citation2009) can help express the anonymous group opinions of membership degree. Meng et al. (Citation2016) extended HFSs to linguistic interval hesitant fuzzy sets and applied them in decision making. Ji et al. (Citation2018) applied the extended multi-Hesitant Fuzzy Linguistic Term Sets to generate an outranking method for hotel location selection. Zhu et al. (Citation2012) extended the characteristics of both IFSs and HFSs and introduced dual hesitant fuzzy sets (DHFSs), which encompass FSs, IFSs, HFSs, and fuzzy multisets (Yager, Citation1986) as special cases. Due to their flexibility in practical decision making, DHFSs are widely studied. Farhadinia (Citation2014) and Tyagi (Citation2015) considered the significant role of correlation in data analysis and studied the correlation model between two DHFSs. Due to the important roles of distance and similarity in decision making and pattern recognition, Wang et al. (Citation2014) proposed a variety of distance measures for DHFSs and generated a technique for order preference by similarity to an ideal solution (TOPSIS) method for a weapon selection problem. Su et al. (Citation2015) introduced some distance and similarity measures for DHFSs. Qiu et al. (Citation2016) studied cosine similarity measures for DHFSs. Singh (Citation2017) proposed the axiom definition of distance and similarity measures and developed distance and similarity measures based on the geometric distance model, the set-theoretic approach and matching functions. Xue et al. (Citation2018) designed a generalized distance measure between dual hesitant fuzzy elements with a parameter and established models to generate the parameters. Additionally, due to the importance of entropy in the measurement of information uncertainty, Zhao and Xu (Citation2015) proposed an entropy measure for DHFSs and Ye (Citation2016) studied the cross entropy of DHFSs. Furthermore, to integrate the dual hesitant fuzzy decision information, Zhao et al. (Citation2017) proposed new operations and aggregation operators on DHFSs based on the Einstein t-conorm and t-norm. Xu and Wei (Citation2017) developed aggregation operators for aggregating dual hesitant bipolar fuzzy information; these operators include the dual hesitant bipolar fuzzy weighted average operator, the dual hesitant bipolar fuzzy weighted geometric operator, the dual hesitant bipolar fuzzy ordered weighted average operator, the dual hesitant bipolar fuzzy ordered weighted geometric operator, the dual hesitant bipolar fuzzy hybrid average operator and the dual hesitant bipolar fuzzy hybrid geometric operator. For dual hesitant fuzzy information, Zhao et al. (Citation2016) proposed a dual hesitant fuzzy preference relation and used it to generate a group decision method. Along with these theoretical studies, DHFSs have been applied in energy policy decision making (Jamil & Rashid, Citation2018), urban traffic mode recognition (Zhang et al., Citation2017, Citation2018), investment alternative selection (Yang & Ju, Citation2015), risk evaluation (Hao et al., Citation2017), information systems security assessment (Yu et al., Citation2016), mechanical product design quality evaluation (Xu, Citation2016), and customs service management evaluation (Wang et al., Citation2016).
Distance measures are used to provide a natural description of the distance between two objects (Liu, Citation1992; McCulloch et al., Citation2013; Cao et al., Citation2019). Cao et al. (Citation2019) studied the distance measure for single-valued neutrosophic linguistic information. To estimate the distance between FSs, Liu (Citation1992), McCulloch et al. (Citation2013) and Zhao and Luo (Citation2017) researched distance measurement models. Zheng et al. (Citation2012) and Luo and Zhao (Citation2018) studied the distance measures of IFSs. Xu and Xia (Citation2011), Li et al. (Citation2015), Zhang et al. (Citation2018) and Hu et al. (Citation2018) defined distance and similarity measures of HFSs. As an extension of both IFSs and HFSs, Wang et al. (Citation2014), Su et al. (Citation2015), and Singh (2017) studied the distance measures between DHFSs by ordering the elements in dual hesitant fuzzy elements and extending them to the same length; additionally, based on the Hamming distance, the Euclidean distance and the Hausdorff distance, they defined a dual hesitant normalized Hamming distance, a dual hesitant normalized Euclidean distance, a generalized dual hesitant normalized Hausdorff distance, and a generalized dual hesitant weighted distance. In these distance measures, three points should be considered.
These distance measure models can be used only after extending all dual hesitant fuzzy elements to the same length. Although the extension process is the same as that in Xu and Xia (Citation2011) while defining the distance measure for HFSs, the process changes the original group decision information, as both the mean and variance change. In this way, the final decision is not based on the original group decision information.
These distance measure models are conducted after decreasing the values in
and
where
=
is a set of dual hesitant fuzzy elements. Thus, the distances generated from these models depend on the order of the values in
and
Existing distance models are not sufficiently robust.
The property of triangular inequality is one of the axioms for distance in metric space. These distance measure models cannot satisfy the property of triangular inequality well. Take three DHFSs and the dual hesitant normalized Hamming distance for example:
Then,
and
Thus,
The distance measure models (Zheng et al., Citation2012; Luo & Zhao, Citation2018) for IFSs do not need to extend or decrease the set of elements and maintain triangular inequality well. As an extension of IFSs, the distance models of DHFSs should also retain the characteristics of IFSs. To fill this gap, this paper aims to improve existing distance measures for DHFSs, generate a distance axiom for DHFSs and propose a series of improved distance models. Furthermore, the new improved distant measure will applied to generate a TOPSIS method for MADM problems.
The contributions of this paper are as follows: (1) The distance axiom for DHFSs is improved such that the distance axiom is concordant with the distance axiom in Euclidean space; (2) a series of distance measure models are proposed for DHFSs that do not require extension or ordering of the elements and that maintain triangular inequality well; (3) a TOPSIS method is constructed using the new distance models for MADM problems.
The rest of this paper is organized as follows. In Section 2, we review some necessary concepts related to IFSs and DHFSs. Section 3 proposes the axiom for distance measure and a series of distance measure models. Section 4 compares the new models with existing models. In Section 5, the new models are applied to multiple attribute decision making problems and a numerical example is illustrated to show the process. The paper concludes in Section 6.
2. Preliminaries
DHFSs are an extension of IFSs and HFSs. In this section, we briefly review basic definitions related to IFSs, DHFSs, their distance axioms, and distance measure models.
2.1. IFSs
IFSs are used to describe both membership degree and nonmembership degree. They were first proposed by Atanassov (Citation1986). A distance measure is used to compare positive or negative alternatives. Zheng et al. (Citation2012) proposed an axiom for distance between IFSs. Some distance measure models have been studied by Li (Citation2004), Szmidt and Kacprzyk (Citation2000), Grzegorzewski (Citation2004), Yang and Chiclana (Citation2012) and Wang and Xin (Citation2005).
Definition 1.
(Atanassov, Citation1986). An intuitionistic fuzzy set in the universe of discourse is given by:
where
with the condition
denote a membership function and a nonmembership function of
to
We call
the intuitionistic index (hesitancy degree) of
to
Definition 2.
(Zheng et al., Citation2012). Let map
Suppose
satisfies the following properties:
(A1)
(A2) if and only if
(A3) is maximum if and only if
and
(A4)
(A5)
(A6) if then
and
Then, is a distance measure between IFSs.
Now, we briefly review the existing distance measures between IFSs as follows.
Intuitionistic fuzzy Hamming distance:
(Li, Citation2004)
(Szmidt & Kacprzyk, Citation2000)
Intuitionistic fuzzy Euclidean distance:
(Szmidt & Kacprzyk, Citation2000)
Intuitionistic fuzzy Hausdorff distance:
(Grzegorzewski, Citation2004)
(Yang & Chiclana, Citation2012)
Intuitionistic fuzzy compound distance:
(Wang & Xin, Citation2005)
2.2. DHFSs
DHFSs were proposed by Zhu et al. (Citation2012) and are an extension of IFSs. They involve a group of membership degree and nonmembership degree, which is very useful when a group of experts participate in decision making. Distance measures play the same role in DHFSs as in IFSs. They are essential evaluation models for decision making. Wang et al. (Citation2014), Su et al. (Citation2015) and Singh (2017) have studied the axiom and distance models.
Some basic concepts, distance axioms and distance models for DHFSs are introduced as follows.
Definition 3.
(Zhu et al., 2012) Suppose that X is a nonempty set and that the mathematical model for DHFS D on X is defined as:
which should satisfy the condition, for all
that
where
and
and
are subsets of [0,1].
describes the group of membership values of the
member of set D.
describes the group of nonmembership values.
Definition 4.
(Zhu et al., 2012) Let be any DHFS. The score function of
is defined by:
The accuracy function of is defined by
where
is the element number in a set. Then,
If
then
is superior to
(
);
If
then
iii. If
then
is equivalent to
(
);
iv. If
then
is superior to
(
).
The distance axiom for DHFSs was addressed by Wang et al. (Citation2014), Su et al. (Citation2015), and Singh (2017).
Definition 5
(Wang et al., Citation2014; Su et al., Citation2015; Singh, 2017). Let and
be two DHFSs on X. A distance measure
should satisfy the following:
(P1)
(P2)
if and only if
(P3)
(P4) Let C be any DHFS; if
then
and
In real applications, the number of elements in different DHFSs may be not equal, i.e.,
Let and
To define the distance measure for DHFSs, the shorter set is extended to the same length as the other sets by Wang et al. (Citation2014), Su et al. (Citation2015) and Singh (2017). Pessimists may extend the minimum membership degree and the maximum nonmembership degree, whereas optimists extend the maximum membership degree and the minimum nonmembership degree. Let
and
be two DHFSs on X. Wang et al. (Citation2014), Su et al. (Citation2015) and Singh (2017) defined the distance measures for DHFSs as follows:
The dual hesitant normalized Hamming distance
The dual hesitant normalized Euclidean distance
The generalized dual hesitant normalized distance
The generalized dual hesitant weighted (DGW) distance
where
is the jth largest value in
and
is the jth largest value in
and
and
and
2.3. Notation description
Descriptions of some of the main notations are shown in .
Table 1. Main notation description.
3. Distance models for DHFSs
In this part, the distance axiom for classic metric space is reviewed. Then, an improved axiom is proposed by incorporating the triangle inequality. Based on the improved axiom, some distance measure models are generated and shown to satisfy the improved axiom. Considering the weights of attributes and membership or nonmembership, two generalized distance measure models are generated.
To introduce the axiom definition of distance measure for DHFSs, we first review the axiom for metric space as follows.
Definition 6.
(Lawvere, Citation1973). Suppose X is a nonempty set. is a distance measure if it satisfies four properties:
(AX1)
(AX2)
if and only if
(AX3)
(AX4)
Clearly, AX4 in Definition 6 is not included in the distance axiom (Definition 5). For a metric space, the property of triangular inequality is especially important. Here, we will add the triangular inequality property to Definition 5 and generate a new distance axiom for DHFSs.
Definition 7.
Suppose and
are two DHFSs on X. A distance measure
should satisfy the following:
(Ph1)
(Ph2)
if and only if
and
(Ph3)
(Ph4) Let C be any DHFS. Then,
(Ph5) Let C be any DHFS; if
then
and
With the completed distance axiom, DHFSs become a metric space. In this metric space, we define new distance measures for the existing distance measurement models that cannot satisfy (Ph4) well (see Section 4).
Definition 8.
Let and
be two DHFSs. The distance measures between each pair can be defined as follows:
The Hamming distance
The Euclidean distance
The generalized distance
Definition 8 can satisfy the distance axiom in Definition 7, as proposed in Theorem 1.
Lemma 1.
(Minkowski’s inequality) (Flanders, Citation1968). Let
and
. Then,
Lemma 2.
Let
and
. Then,
Proof.
Let Thus,
Theorem 1.
Let and
be two DHFSs on X.
and
satisfy the properties in Definition 7.
(The proof is in the appendix.)
Extension 1:
the generalized dual hesitant weighted distance
Considering the weight of each we note the weight for membership
and that for nonmembership
where
and
The generalized dual hesitant weighted distance can be defined as
Extension 2:
the generalized dual hesitant weighted distance with preference for membership and nonmembership
In practical decision making, the decision maker would prefer either a membership degree or nonmembership degree. A positive decision maker would focus his/her attention on the distance between the membership degree and the positive idea point, while a negative decision maker pays attention to the distance between the nonmembership degree and the negative idea point. With this in mind, we set a parameter on the generalized dual hesitant weighted distance
and generate the generalized dual hesitant weighted distance with preference to membership and nonmembership as follows:
where
is the decision maker’s preference of membership degree and nonmembership degree;
indicates that the decision maker only focuses on the distance of the membership degree, while
indicates that the decision maker only focuses on the distance of the nonmembership degree. When
will decrease to the generalized dual hesitant weighted distance
It can be proved in the same way as that of Theorem 1 that and
also satisfy the properties in Definition 7. In the next section, we compare the new proposed distance models with existing models.
4. Advantages of the proposed distance measure models
This section outlines the advantages of the proposed distance measure models compared with existing distance models (Wang et al., Citation2014; Su et al., Citation2015; Singh, 2017). These advantages include the lack of a requirement to change decision information and their robust and satisfying triangle inequality.
4.1. Unlike existing distance models, the new distance models do not need to change the original decision information
The existing distance models, i.e.,
(Wang et al., Citation2014; Su et al., Citation2015; Singh, 2017), require the number of elements in different DHFSs to remain the same, i.e.,
and
In real applications, when the numbers of elements in different DHFSs are not equal,
or
To utilize the existing distance models, Wang et al. (Citation2014), Su et al. (Citation2015) and Singh (2017) extended the shorter set (the set with fewer values of the membership degree set and the nonmembership degree set) to the length of the longer set. Pessimists extend the minimum membership degree and the maximum nonmembership degree, whereas optimists extend the maximum membership degree and the minimum nonmembership degree. For example, when
and
to calculate the distance between them, a pessimist would change
to
while an optimist would change
to
In this process, they change the mean and variance (see ). The pessimist decreases the mean of the original membership degree set, and the optimist increases the mean of the original membership degree set. At the same time, both decrease the variance of the original membership degree.
During this extension process, the existing model takes the distance between and
and
and
as a proxy of the distance between
and
However, there is bias in this substitution. The distances between
and
and
and
and
are measured by
with
The results are shown in . Bias 1 exists when taking the distance between
and
as the proxy of the distance between
and
while bias 2 exists when taking the distance between
and
as the proxy, no matter the value of
Table 2. Changes of mean and variance.
4.2. Unlike existing distance models, the new distance models are robust
To utilize existing distance models, Wang et al. (Citation2014), Su et al. (Citation2015) and Singh (2017) decreased the numbers in both the membership degree set and the nonmembership degree set. Thus, the distance generated from the existing models largely depends on the order of values in the membership degree set or the nonmembership degree set. Take the distance between and
the existing Euclidean distance
and the new proposed Euclidean distance
for example. The Euclidean distances between increasing, decreasing and random order of values in membership degree and nonmembership degree are measured separately by the existing models and new models. The results in show that the increasing order of values in the membership degree set and the decreasing order of values in the membership degree set generate different distances between
and
using the existing distance models
With the new proposed distance measure models
the distance between
and
is constant. Thus, the new distance models are robust compared with existing distance measure models for DHFSs.
Table 3. The Euclidean distance with different orders.
4.3. The new distance models maintain (Ph4) well while the existing distance measure models do not satisfy (Ph4) in Definition 7
Theorem 1 promises that the new proposed distance models maintain (Ph4) well. The following example illustrates that the existing distance measure models (Wang et al., Citation2014; Su et al., Citation2015; Singh, 2017) do not satisfy (Ph4) in Definition 7.
Example 1.
For three DHFSs, i.e.,
The distances between pairs are
Hence, we find that
Similarly,
Thus, we have
The comparison clearly shows that the new distance measurement models are a success in terms of the existing distance measure models in the three points.
The new distance models do not need to extend the values in the membership degree set or in the nonmembership degree set.
The new distance models are robust and do not depend on the order of the values in the membership degree set or in the nonmembership degree set.
The new distance models satisfy property (Ph4) in the axiom definition.
5. The application of the distance measures for MADM
In this section, the new proposed distance measure models are utilized to develop a TOPSIS method (Chen & Hwang, Citation1992) for multiple attribute decision making, whose decision information is provided by DHFSs. TOPSIS is a common multiple attribute decision making method and aims to identify a solution from a finite set of alternatives. The core idea of the TOPSIS method is to define the ideal solution and negative ideal solution, evaluate the distance between each alternative and the ideal solution/negative ideal solution, and identify the optimal solution, which should have the shortest distance from the positive ideal solution and the longest distance from the negative ideal solution. When decision information is provided by DHFSs, the new proposed distance measure models can help generate the distance between alternatives and the ideal and negative ideal solutions.
Suppose a multiple attribute decision making problem contains alternatives and
attributes. Let
be the alternative set and
be the attribute set. The decision information for each alternative is:
The process of the generated TOPSIS method based on the new proposed distance measure models is shown in .
A numerical example for multiple attribute decision making taken from Singh (2017) is adopted to show the TOPSIS process. A company wants to invest a sum of money. They have four investment choices: a car company (), a food company (
), a computer company (
), and an arms company (
). They consider the attributes of risk analysis (
), growth analysis (
) and environmental impact analysis (
). The attribute weights for the membership and nonmembership degrees are
and
respectively. For a good economic environment, the company takes an optimistic attitude toward this investment and sets the decision parameter
to 0.7. Three experts evaluated these choices by satisfying the attributes using a DHFS. The dual hesitant fuzzy information is listed in .
Table 4. Dual hesitant fuzzy information.
Set the ideal solution
and the negative ideal solution
We set and calculate the distances between
and
and
and
by the generalized dual hesitant weighted distance with preference models
and
The distances and results are shown in . The distance between
(i = 1,2,3,4) and
are 0.0713, 0.0163, 0.0426 and 0.0033, respectively.
is closest to
The distances between
and
are 0.0058, 0.0513, 0.0248 and 0.0788, respectively.
is farthest from
The relative closeness values of
(i = 1,2,3,4) are 0.0759, 0.7586, 0.3680 and 0.9594. The distances between the alternatives and the ideal solution and negative ideal solution and the relative closeness show that alternative
(an arms company) is the optimal solution.
Table 5. The results of the TOPSIS process.
6. Conclusions
The distance measures of DHFSs proposed by Wang et al. (Citation2014), Su et al. (Citation2015) and Singh (2017) did not consider the property of triangular inequality, required the extension of the shorter membership set or the nonmembership set and lacked robustness. This paper improves the functionality of the axiom definition and the distance models for DHFSs. The property of triangular inequality is added in the new axiom definition for the distance measure. Based on the improved axiom definition, a series of distance models, such as the Hamming distance model, the Euclidean distance model and the generalized distance model, are generated. Theorem 1 proved that these models satisfy the new axiom definition well. To consider the preference of the decision maker in the attribute, the membership weight and the nonmembership weight of each attribute are added to the general model, and the generalized dual hesitant weighted distance model is generated. Additionally, a generalized dual hesitant weighted distance with preference model
is generated by integrating the decision maker’s preference with the membership degree and the nonmembership degree. The generalized dual hesitant weighted distance with preference model
can take the new proposed distance models as a special case. To show their advantages, the new distance measure models are compared with existing ones. The comparison results show that the new distance models do not need to extend the shorter set, are robust and satisfy the property of triangular inequality well, while the existing models have to extend the shorter set, lack robustness and do not satisfy the property of triangular inequality.
To show the application of new distance measure models, we generate a TOPSIS process with new models to solve the multiple attribute decision making problem with dual hesitant fuzzy information. An investment example taken from Singh (2017) helps show the TOPSIS process and the advantages of our distance measure. The newly proposed method can be used in economic investments, supplier selection and other decision maker problems. With the TOPSIS process, comprehensive decision information is collected and utilized from supporting and opposing aspects. Compared with the existing distance measures proposed by Wang et al. (Citation2014), Su et al. (Citation2015) and Singh (2017), this numerical example highlights two advantages of the new distance measure. First, the new proposed method is based on the original decision information. If the existing distance measure is utilized to calculate the distance between the ideal solution or negative ideal solution and alternatives, the value of the ideal solution and negative ideal solution have to be extended to keep the same length as another DHFS. As a result, the original information is changed. With our distance measure, the ideal solution and negative ideal solution do not need to be extended to meet the length of the membership degree set and nonmembership degree set of alternatives. Second, the membership degree values and nonmembership degree values of DHFS do not need to be ranked in decreasing or increasing order. The distance calculating from existing distance measures (Wang et al., Citation2014; Su et al., Citation2015; Singh, 2017) is associated with the order of the values in the membership degree set and nonmembership degree set of a DHFS. Compared to existing distance models, the distance calculated from the new proposed model is robust.
The contributions of this paper include the following: (1) the distance axiom for DHFSs is improved, and the improved axiom is concordant with the distance axiom on classic sets; (2) a series of distance measures are generated for DHFSs that can be used to update the decision method; (3) these new distance measures are applied to generate a TOPSIS method for the MADM problem; (4) both supporting and opposing information are incorporated in decision making.
There are also limitations. First, for DHFS information, the importance of experts cannot be recognized. Second, when utilizing distance models for DHFS, the attribute weights for membership degree and nonmembership degree are somewhat difficult to determine. Third, the numerical example of this paper refers to the existing literature. Future studies can focus on generating attribute weights and the weights of membership/nonmembership, similarity measures and their application to pattern recognition and machine learning, and conducting empirical studies on economic or management problems.
Acknowledgments
The authors thank the anonymous reviewers for their insightful and constructive comments.
Disclosure statement
No potential conflict of interest was reported by the author(s).
Additional information
Funding
References
- Atanassov, K. T. (1986). Intuitionistic fuzzy sets. Fuzzy Sets and Systems, 20(1), 87–96. https://doi.org/10.1016/S0165-0114(86)80034-3
- Boran, F. E., Boran, K., & Menlik, T. (2012). The evaluation of renewable energy technologies for electricity generation in Turkey using intuitionistic fuzzy TOPSIS. Energy Sources, Part B: Economics, Planning, and Policy, 7(1), 81–90. https://doi.org/10.1080/15567240903047483
- Cao, C., Zeng, S., & Luo, D. (2019). A single-valued neutrosophic linguistic combined weighted distance measure and its application in multiple-attribute group decision-making. Symmetry, 11(2), 275. https://doi.org/10.3390/sym11020275
- Chen, S. J., & Hwang, C. L. (1992). Multiple attribute decision making—An overview. In Fuzzy Multiple Attribute Decision Making (pp. 16–41). Springer.
- Erkayman, B., Gundogar, E., Akkaya, G., & Ipek, M. (2011). A fuzzy TOPSIS approach for logistics center location selection. Journal of Business Case Studies (JBCS), 7(3), 49–54. https://doi.org/10.19030/jbcs.v7i3.4263
- Farhadinia, B. (2014). Correlation for dual hesitant fuzzy sets and dual interval‐valued hesitant fuzzy sets. International Journal of Intelligent Systems, 29(2), 184–205. https://doi.org/10.1002/int.21633
- Flanders, H. (1968). A proof of Minkowski's inequality for convex curves. The American Mathematical Monthly, 75(6), 581–593. https://doi.org/10.1080/00029890.1968.11971034
- Grzegorzewski, P. (2004). Distances between intuitionistic fuzzy sets and/or interval-valued fuzzy sets based on the Hausdorff metric. Fuzzy Sets and Systems, 148(2), 319–328. https://doi.org/10.1016/j.fss.2003.08.005
- Hao, Z., Xu, Z., Zhao, H., & Su, Z. (2017). Probabilistic dual hesitant fuzzy set and its application in risk evaluation. Knowledge-Based Systems, 127, 16–28. https://doi.org/10.1016/j.knosys.2017.02.033
- Hu, M., Lan, J., & Wang, Z. (2019). A distance measure, similarity measure and possibility degree for hesitant interval-valued fuzzy sets. Computers & Industrial Engineering, 137, 106088. https://doi.org/10.1016/j.cie.2019.106088
- Jamil, R. N., & Rashid, T. (2018). Application of Dual Hesitant Fuzzy Geometric Bonferroni Mean Operators in Deciding an Energy Policy for the Society. Mathematical Problems in Engineering, 2018, 1–14. https://doi.org/10.1155/2018/4541982
- Ji, P., Zhang, H. Y., & Wang, J. Q. (2018). A projection-based outranking method with multi-hesitant fuzzy linguistic term sets for hotel location selection. Cognitive Computation, 10(5), 737–751. https://doi.org/10.1007/s12559-018-9552-2
- Kaynak, S., Altuntas, S., & Dereli, T. (2017). Comparing the innovation performance of EU candidate countries: an entropy-based TOPSIS approach. Economic research-Ekonomska Istraživanja, 30(1), 31–54. https://doi.org/10.1080/1331677X.2016.1265895
- Lan, J., Yang, M., Hu, M., & Liu, F. (2018). Multi-attribute group decision making based on hesitant fuzzy sets, TOPSIS method and fuzzy preference relations. Technological and Economic Development of Economy, 24(6), 2295–2317. https://doi.org/10.3846/tede.2018.6768
- Lawvere, F. W. (1973). Metric spaces, generalized logic, and closed categories. Rendiconti del Seminario Matematico e Fisico di Milano, 43(1), 135–166. https://doi.org/10.1007/BF02924844
- Li, D. F. (2004). Some measures of dissimilarity in intuitionistic fuzzy structures. Journal of Computer and System Sciences, 68(1), 115–122. https://doi.org/10.1016/j.jcss.2003.07.006
- Li, D., Zeng, W., & Zhao, Y. (2015). Note on distance measure of hesitant fuzzy sets. Information Sciences, 321, 103–115. https://doi.org/10.1016/j.ins.2015.03.076
- Liu, P. (2009). Multi‐attribute decision‐making method research based on interval vague set and TOPSIS method. Technological and Economic Development of Economy, 15(3), 453–463. https://doi.org/10.3846/1392-8619.2009.15.453-463
- Liu, X. (1992). Entropy, distance measure and similarity measure of fuzzy sets and their relations. Fuzzy Sets and Systems, 52(3), 305–318.
- Luo, M., & Zhao, R. (2018). A distance measure between intuitionistic fuzzy sets and its application in medical diagnosis. Artificial Intelligence in Medicine, 89, 34–39. https://doi.org/10.1016/j.artmed.2018.05.002
- Mandić, K., Delibašić, B., Knežević, S., & Benković, S. (2017). Analysis of the efficiency of insurance companies in Serbia using the fuzzy AHP and TOPSIS methods. Economic Research-Ekonomska istraživanja, 30(1), 550–565.
- Mangir, F., & Erdogan, S. (2011). Comparison of economic performance among six countries in global financial crisis: The application of fuzzy TOPSIS method. Economics, Management and Financial Markets, 6(2), 122.
- Masca, M. (2017). Economic performance evaluation of European union countries by Topsis method. North Economic Review, 1(1), 83–94.
- McCulloch, J., Wagner, C., & Aickelin, U. (2013, September). Measuring the directional distance between fuzzy sets. In Computational Intelligence (UKCI), 2013 13th UK Workshop on. (pp. 38–45). IEEE.
- Meng, F., Wang, C., & Chen, X. (2016). Linguistic interval hesitant fuzzy sets and their application in decision making. Cognitive Computation, 8(1), 52–68. https://doi.org/10.1007/s12559-015-9340-1
- Önder, E., Taş, N., & Hepsen, A. (2015). Economic performance evaluation of fragile 5 countries after the great recession of 2008-2009 using analytic network process and topsis methods. Journal of Applied Finance & Banking, 5(1), 1–17.
- Qiu, W., Zhang, G., & Zhu, L. (2016). Cosine similarity measures for dual hesitant fuzzy sets [Paper presentation]. International Conference on Machinery. https://doi.org/10.2991/icmmct-16.2016.102
- Singh, P. (2017). Distance and similarity measures for multiple-attribute decision making with dual hesitant fuzzy sets. Computational and Applied Mathematics, 36(1), 111–126. https://doi.org/10.1007/s40314-015-0219-2
- Su, Z., Xu, Z., Liu, H., & Liu, S. (2015). Distance and similarity measures for dual hesitant fuzzy sets and their applications in pattern recognition. Journal of Intelligent & Fuzzy Systems, 29(2), 731–745. https://doi.org/10.3233/IFS-141474
- Szmidt, E., & Kacprzyk, J. (2000). Distances between intuitionistic fuzzy sets. Fuzzy Sets and Systems, 114(3), 505–518. https://doi.org/10.1016/S0165-0114(98)00244-9
- Torra, V. (2010). Hesitant fuzzy sets. International Journal of Intelligent Systems, 25(6), n/a–539. https://doi.org/10.1002/int.20418
- Torra, V., & Narukawa, Y. (2009, August). On hesitant fuzzy sets and decision. In Fuzzy systems 2009. FUZZ-IEEE 2009. IEEE International Conference on (pp. 1378–1382). IEEE.
- Tyagi, S. K. (2015). Correlation coefficient of dual hesitant fuzzy sets and its applications. Applied Mathematical Modelling, 39(22), 7082–7092. https://doi.org/10.1016/j.apm.2015.02.046
- Wang, L., Wang, Q., Xu, S., & Ni, M. (2014, May). Distance and similarity measures of dual hesitant fuzzy sets with their applications to multiple attribute decision making. In Progress in Informatics and Computing (PIC), 2014 International Conference on (pp. 88–92). IEEE.
- Wang, S. W., Ding, X. Q., & Ding, Z. Z. (2016). Model for performance evaluation in customs service management with dual hesitant fuzzy information. Journal of Intelligent & Fuzzy Systems, 30(4), 2131–2137. https://doi.org/10.3233/IFS-151926
- Wang, W., & Xin, X. (2005). Distance measure between intuitionistic fuzzy sets. Pattern Recognition Letters, 26(13), 2063–2069. https://doi.org/10.1016/j.patrec.2005.03.018
- Xu, X. R., & Wei, G. W. (2017). Dual hesitant bipolar fuzzy aggregation operators in multiple attribute decision making. International Journal of Knowledge-Based and Intelligent Engineering Systems, 21(3), 155–164. https://doi.org/10.3233/KES-170360
- Xu, Y. (2016). Model for evaluating the mechanical product design quality with dual hesitant fuzzy information. Journal of Intelligent & Fuzzy Systems, 30(1), 1–6. https://doi.org/10.3233/IFS-141426
- Xu, Z., & Xia, M. (2011). Distance and similarity measures for hesitant fuzzy sets. Information Sciences, 181(11), 2128–2138. https://doi.org/10.1016/j.ins.2011.01.028
- Xue, M., Fu, C., & Chang, W. J. (2018). Determining the Parameter of Distance Measure Between Dual Hesitant Fuzzy Information in Multiple Attribute Decision Making. International Journal of Fuzzy Systems, 20(6), 2065–2082. https://doi.org/10.1007/s40815-018-0512-5
- Yager, R. R. (1986). On the theory of bags. International Journal of General Systems, 13(1), 23–37. https://doi.org/10.1080/03081078608934952
- Yang, M. S., & Hussain, Z. (2019). Distance and similarity measures of hesitant fuzzy sets based on Hausdorff metric with applications to multi-criteria decision making and clustering. Soft Computing, 23(14), 5835–5814. https://doi.org/10.1007/s00500-018-3248-0
- Yang, S., & Ju, Y. (2015). A GRA method for investment alternative selection under dual hesitant fuzzy environment with incomplete weight information. Journal of Intelligent & Fuzzy Systems, 28(4), 1533–1543. https://doi.org/10.3233/IFS-141436
- Yang, Y., & Chiclana, F. (2012). Consistency of 2D and 3D distances of intuitionistic fuzzy sets. Expert Systems with Applications, 39(10), 8665–8670. https://doi.org/10.1016/j.eswa.2012.01.199
- Ye, J. (2016). Cross-entropy of dual hesitant fuzzy sets for multiple attribute decision-making. International Journal of Decision Support System Technology (IJDSST), 8(3), 20–30.
- Yu, D., Merigó, J. M., & Xu, Y. (2016). Group decision making in information systems security assessment using dual hesitant fuzzy set. International Journal of Intelligent Systems, 31(8), 786–812. https://doi.org/10.1002/int.21804
- Zadeh, L. A. (1965). Fuzzy sets. Information and Control. 8(3), 338–353. https://doi.org/10.1016/S0019-9958(65)90241-X
- Zeng, S., Chen, S. M., & Fan, K. Y. (2020). Interval-valued intuitionistic fuzzy multiple attribute decision making based on nonlinear programming methodology and TOPSIS method. Information Sciences, 506, 424–442. https://doi.org/10.1016/j.ins.2019.08.027
- Zeng, S., Luo, D., Zhang, C., & Li, X. (2020). A correlation-based TOPSIS method for multiple attribute decision making with single-valued neutrosophic information. International Journal of Information Technology & Decision Making, 19(01), 343–358. https://doi.org/10.1142/S0219622019500512
- Zhang, F., Chen, J., Zhu, Y., Li, J., Li, Q., & Zhuang, Z. (2017). A Dual Hesitant Fuzzy Rough Pattern Recognition Approach Based on Deviation Theories and Its Application in Urban Traffic Modes Recognition. Symmetry, 9(11), 262. https://doi.org/10.3390/sym9110262
- Zhang, F., Chen, S., Li, J., & Huang, W. (2018). New distance measures on hesitant fuzzy sets based on the cardinality theory and their application in pattern recognition. Soft Computing, 22(4), 1237–1245. https://doi.org/10.1007/s00500-016-2411-8
- Zhao, H., Xu, Z., & Liu, S. (2017). Dual hesitant fuzzy information aggregation with Einstein t-conorm and t-norm. Journal of Systems Science and Systems Engineering, 26(2), 240–264. https://doi.org/10.1007/s11518-015-5289-6
- Zhao, N., & Xu, Z. (2015, April). Entropy measures for dual hesitant fuzzy information. In Communication Systems and Network Technologies (CSNT), 2015 Fifth International Conference on (pp. 1152–1156). IEEE.
- Zhao, N., Xu, Z., & Liu, F. (2016). Group decision making with dual hesitant fuzzy preference relations. Cognitive Computation, 8(6), 1119–1143. https://doi.org/10.1007/s12559-016-9419-3
- Zhao, R., & Luo, M. (2017, November). Modified distance measure between fuzzy sets. In Intelligent Systems and Knowledge Engineering (ISKE), 2017 12th International Conference on (pp. 1–4). IEEE.
- Zheng, M., Shi, Z., Liu, Y., & Han, G. (2012). Distance and similarity measures between intuitionistic fuzzy sets. In Quantitative Logic and Soft Computing (pp. 589–596). https://doi.org/10.1142/9789814401531_0079
- Zhu, B., Xu, Z., & Xia, M. (2012). Dual hesitant fuzzy sets. Journal of Applied Mathematics., 2012, 1–13. https://doi.org/10.1155/2012/879629
Appendix:
The proof of Theorem 1
Theorem 1.
Let and
be two DHFSs on X.
and
satisfy the properties in Definition 7.
Proof.
and
are special cases of the generalized dual hesitant normalized distance
Therefore, we prove only that
satisfies the properties in Definition 7.
(Ph1)
For
Clearly,
Because
are the membership values in
and
and
are the nonmembership values in
and
Thus,
According to Lemma 1, we have
Thus,
(Ph2)
if and only if
If
then for all
For
and
Then, we have
Therefore,
According to Definition 2,
Conversely, if
then for all
We have
Thus,
and
(Ph3)
is clear.
(Ph4) For any DHFS
on X,
Let
Then,
when
v.(Ph5) Let
be any DHFSs; if
then
and
For
we have
Then,
Furthermore, we have
Thus,
and
According to the proven process of (Ph4), the above is equal. Thus,
and