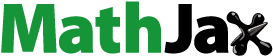
Abstract
Based on panel data from 2002 to 2017 in China, this paper analyses the influence of internal migration on regional innovation. The results show that internal migration not only has a significant promoting effect on improving regional innovation, but also presents a significant spatial agglomeration phenomenon. That is, internal migration has a significant positive impact on regional innovation according to the regression without spatial effects. And although internal migration will promote input of regional innovation, it will also have a negative impact on output of regional innovation. Meanwhile, internal migration will have a significant negative impact on innovation input in adjacent areas, and a significant positive impact on innovation output. Through decomposition, from the input of innovation, the migrant population will have a significant impact on local innovation, but it will also inhibit the innovation input of adjacent regions through indirect effects. Although the migrant population will have a significant negative impact on output of innovation, it will also promote innovation output significantly in adjacent regions through indirect effects, and have a positive impact on the improvement of overall innovation.
1. Introduction
In economic production activities, population is an important factor; it is not only the consumer but also the producer of the initial stage of the product. Migration across regions is essentially the spatial reconstruction of labour, human capital and consumer groups. According to the new economic growth theory, technological progress is at the core of promoting economic growth. However, technological progress stems largely from innovation, so migration has an important impact on urban innovation. Migration has a positive contribution to innovation, especially high-quality or diverse migration. First, migration has brought about the concentration of the total population and increased opportunities for communication. Second, skilled migration, enthusiasm of young people, self-realisation, courage to take risks and entrepreneurship will drive innovation and development. Third, migration will bring racial and cultural diversity to cities, promote the integration and exchange of urban ideas, and provide a good atmosphere for innovation. With the change of the household registration system in China, the scale of migration is expanding. According to the Statistical Bulletin of National Economic and Social Development, the total migrant population was 2.44 billion in 2018, so the proportion of the migrant population is still large. The tide of the migrant population has become a unique landscape in the period of social and economic structural change in China. In terms of international migration, western scholars demonstrated that human capital and knowledge spill-over caused by migration can significantly improve the innovation of emigration countries. Meanwhile, in terms of the distribution of migration in China, the spatial distribution of internal migration is extremely uneven. The Midwest is the main region of emigration; the East is the main region of immigration. The large internal migration further enhanced the agglomeration effect of coastal areas, and formed a positive feedback mechanism. The large amount of immigrant labour made up for the shortage of labour supply in the eastern region and it became an important factor to promote economic development (Wang & Huang, Citation2005). The large-scale internal migration is bound to have an important impact on regional economic development (Yu et al., Citation2013) as the basic flow of migration is from low-income areas to high-income areas, or economically backward areas flow to economically developed areas. Therefore, in view of the migration situation of China's inter-provincial region, the following questions are raised. Will inter-provincial migration have an impact on regional innovation in China? Can migration not only effect regional innovation, but also have spatial spill-over effects on adjacent areas? This is worthy of further study.
Based on the perspective of regional innovation, this paper not only investigates the impact of immigration on regional innovation from a static point of view, but also uses the spatial measurement method to analyse the spatial spill-over effect of immigration on regional innovation, including innovation input and output. This can further inspire us to support areas with weak regional innovation due to migration.
2. Literature review
Generally, population migration refers to the population distribution of geographical spatial change, including time and space. The migration referred to in this paper is mainly the movement of the population that crosses the provinces, and becomes resident. Since Joseph Schumpeter first put forward the ‘innovation theory’, literature about technological innovation has gradually enriched. With the development of the economy, the scale of expanding migration, and the impact on the economy and society gradually deepens. Many scholars have linked migration with regional innovation, and try to study their internal mechanisms. And with the coming of the era of the knowledge economy, human capital as the core capital of innovation has drawn more and more attention. Some scholars believe that regional innovation is largely reflected in the quantity, quality and structural relationships of human capital (Yang, Citation2015). On the relationship between human capital and regional innovation, scholars have carried out a considerable amount of research. In terms of the amount of human capital, Qian et al. (Citation2010), using inter-provincial data and the spatial regression method, including the spatial lag model and the spatial error model, estimated the influence of human capital on the level of technological innovation. They found that the proportion of higher education for practitioners has a significant positive correlation with provincial technological innovation activities. Wang (Citation2015) used the threshold model to explore the non-linear relationship between human capital and regional innovation. Ma (Citation2016) thought that human capital has a spatial spill-over effect, which not only has an impact on technological progress in the region, but also a significant impact on the innovation of neighbouring regions through transportation infrastructure. In terms of human capital structure, Zhang and Shi (Citation2018) considered that human capital has a significant positive impact on basic innovation in China, and that higher education plays a key role in the structure of human capital in regional innovation. In terms of human capital mobility, Simonen and Mccann (Citation2008) used the probit model to explore the impact of human capital flows on corporate innovation. They found that local human capital investments had no significant impact on innovation, but inter-regional human capital inflows could promote product innovation and process innovation significantly. The impact on regional innovation caused by migration can be reflected in the following.
First, in terms of the total population, immigration increases the total population of the region, and it has an impact on innovation in the regional economy. Immigration increases the supply of labour in the local region, it can increase the competitiveness of regional labour and stimulating innovation (Freeman, Citation1997). So, the increase of foreign students would increase the patents (Chellaraj et al., Citation2008).
Second, the age structure and individual characteristics of immigration have influence on regional innovation. Migration aged 20–30 will reduce the average age of labour and because they have a higher ability to innovate, it can bring more innovation to the area of immigration (Poot, Citation2008). The study was based on the urban population and showed that there was a significant positive correlation between the population aged 15–64 and innovation (Huang et al., Citation2014). At the same time, education and the proportion of the urban population were positively correlated with regional innovation. The survey data of college graduates found that, with the increase of highly skilled migration, the level of regional patents showed a rapid growth trend (Hunt & Gauthier-Loiselle, Citation2010). The study used the three-stage least square method to analyse the relationship between migration and innovation in the UK. It was found that the migration of college graduates brought about human capital and improved the level of regional innovation (Faggian & Mccann, Citation2008). There is a positive correlation between the characteristics of migrant labour and the average innovation in the labour market. The migration of skilled labour has a significant promoting effect on innovation (Mare et al., Citation2011). When skilled labour comes from developed countries, it will increase the number of patent applications (Lissoni, Citation2018; Partridge & Furtan, Citation2008). Bosetti et al. (Citation2015) analysed the effect of skilled migration on two measures of innovation – patenting and citations of scientific publications – in a panel of 20 European countries. Skilled migrants positively contribute to knowledge formation in host countries. High-quality labour is one of the key factors for the success of innovation and maintaining the advantages of innovation in the metropolis. There are problems in the process of urban innovation in Shanghai, such as relatively lagging population growth and insufficient mobility, diversity and openness, so population and industrial control should be relaxed (Zuo & Wang, Citation2009). Fan and Liu (Citation2012) analysed the influence of transnational migration on the structure of human capital and technological innovation in developing countries. Under the influence of the cost difference between domestic and foreign coordination, transnational migration will stimulate the labour of the migrants to choose more specialised education, thus changing the structure of human capital in the source country of migration and affecting the efficiency of technological innovation.
Third, the cultural diversity of immigrants has an impact on innovation. Immigrants can promote innovation by increasing regional cultural diversity. Cultural diversity has a positive impact on regional productivity and innovation (Ozgen et al., Citation2011, Citation2014). There is a positive correlation between multiculturalism and innovation in corporate labour (Lee & Nathan, Citation2010). The scholar studied the impact of international migration on innovation and considered that with the background of cultural diversity in R&D activities, the inflow of talent can increase innovation output (Niebuhr, Citation2010). Other scholars studied the influence of high-quality migration on innovation from the individual and national level. They believed that the migration of college graduates will improve the level of innovation significantly (Hunt & Gauthier-Loiselle, Citation2010). However, the cultural diversity of immigrants also has a certain negative impact on innovation. On the one hand, transnational migration will encourage labour in the source countries of migration to accumulate more innovative knowledge. It will distort the knowledge structure of labour and reduce the speed of economic growth (Fan & Liu, Citation2012). On the other hand, when the population inflow capacity is insufficient, it will restrict the social, economic and innovation of the region. The increasing rate of migration is also an important reason for the difference of innovation ability.
In summary, most empirical studies demonstrated a positive impact on the innovation of migration, when considering the number of inflow population, high skills and young immigrants, diversity and so on. The domestic research on migration basically shows that migration plays a significant role in promoting regional economic development. At present, although the domestic research on the relationship between migration and economic growth is relatively rich, the impact of migration on regional innovation is relatively scarce. The orderly flow or migration of population between regions can form an agglomeration effect. It will enhance regional innovation and promote the development of the regional economy (Wang, Citation2009). This study used provincial data from 1990 to 2009 to explore the spill-over effects caused by the concentration of human capital to cities under the background of rapid urbanisation in China. The spill-over of human capital brought by population concentration will promote the improvement of regional innovation (Shi, Citation2013). This paper established an empirical model to analyse the relationship between the accumulation of scientific and technological talent and regional innovation in 31 provinces of China. The results show that there is an interactive relationship between the effect of scientific and technological talent and regional innovation, and it shows a spiral upward trend. Although these studies discuss the impact of migration on innovation from different angles, there was no literature to take spatial factors into account (Gao et al., Citation2014). The importance of empirical analysis by introducing the spatial panel model is that it can reflect the spatial correlation and spatial spill-over effects of regional innovation activities. Based on the theory of endogenous growth, this paper holds that population migration mainly affects regional innovation by producing human capital accumulation and knowledge spill-over effects.
3. Model
3.1. Basic model
According to the mode of knowledge production function, we set innovation as a function of the number of R&D personnel and the investment of R&D capital, that is:
(1)
(1)
where
represent the region and time, respectively.
represents the output efficiency.
denotes the knowledge output.
denotes the knowledge stock.
denote the number of R&D personnel and R&D capital input in the R&D sector engaged in innovation activities, respectively. The parameters
and
denote the knowledge stock, the innovation output elasticity of R&D personnel and R&D capital, respectively.
There is an independent labour age effect caused by research ability and innovation motivation in innovation activities. Inter-provincial population migration will directly affect the working age composition of the region (Frosch & Tivig, Citation2009). Therefore, we have introduced a migration factor to capture the demographic effect of inter-provincial migration on regional development ability and innovation motivation. The
varies with age.
(2)
(2)
The logarithmic transformation of formula (2) is carried out, i.e. the basic model of this paper is obtained:
(3)
(3)
By simplifying formula (3), we can test whether immigration of the population will have a different impact on the innovation activities in the regions. The basic econometric model equation is set as follows:
(4)
(4)
In the model EquationEquation (4)(4)
(4) , the variable
is the agent index used to measure the innovation activities of the
provinces and the year
represents the migration. In order to solve the possible bias problem caused by missing variables in EquationEquation (4)
(4)
(4) as far as possible, we set the control variable as
In addition, we also control the virtual variables of the fixed effects of the provinces and the years in EquationEquation (4)
(4)
(4) . The former is used to control the differences in regional innovation activities due to the differences in government support policies, technological levels and even geographical location in different provinces. The latter is used to control the impact of changes in government support policies or external macroeconomic changes.
represents a random error term.
3.2. Spatial metrological model
Considering that innovation may be affected not only by the internal migration, but also by migration and other related factors in the adjacent region. The spatial metrological model used in the empirical analysis of this paper is obtained by adding spatial factors on the basis of the general panel model. In general, spatial measurement models include the spatial lag model (SAR), spatial error model (SEM) and spatial Durbin model (SDM).
Generally, the spatial lag model (SAR) is used when considering endogenous interactions between interpreted variables. The SAR model mathematical expression is:
(5)
(5)
where
represents region,
represents year,
represents the regional innovation,
represents the space weight matrix,
represents the spatial lag term of the regional innovation,
represents all others explanatory variables,
and
are the correlation coefficients,
is the random disturbance term.
When considering the interaction effect between error terms, the spatial error model (SEM) is used. The mathematical expression of the SEM model is:
(6)
(6)
(7)
(7)
is a spatial error factor, measuring the spatial dependence of sample observations on random perturbations. Other variables mean the same as formula (5).
The spatial Durbin model (SDM) considers the spatial correlation of dependent variables and the spatial correlation of the arguments. That is, the dependent variables are not only affected by the regional arguments, but also by the other regions of the arguments and dependent variables.
(8)
(8)
where
is the spatial lag term of the explanatory variable,
is the individual fixed effect,
is the time fixed effect and
is the random disturbance term, respectively. Other variables mean the same as formula (5).
3.3. The spatial weight matrix
The spatial weight matrix commonly used in the existing research includes adjacent matrix (0 − 1 matrix), economic weight matrix and geographical distance matrix. The spatial dependence between variables is investigated from three aspects: geographical proximity, economic correlation and inter-regional distance. However, it is worth noting that the spatial correlation mainly comes from the spatial interaction formed by the flow of elements (Cai & Qian, Citation2013). High-skilled and high-quality labour is the driving force for the improvement of regional innovation. Therefore, this paper establishes the following three spatial weight matrices:
The spatial weight matrix of the geographical distance.
The geographic distance weight matrix is the relation between the further spatial units based on the adjacency matrix, and the form of which is shown in (9). The matrix element of row
and column
is the matrix element
the row and column correspond to the spatial unit, and the elements on the diagonal line are zero. The space
is the geographic distance between the space unit
and the space unit
We use the linear Euclidean distance between the provincial capital cities.
is a coefficient, and in order to eliminate the influence of distance measurement units on the results, and to avoid the error caused by the weight calculation result being too small, we replace it with
the reciprocal of the shortest distance between cities. In order to simplify the model and make the results easy to explain, the spatial weight matrix is often standardised to the sum of each row element to 1, bearing in mind that the standardised weight is
(9)
(9)
The spatial weight matrix of economic characteristic space distance.
Although the space spill-overs of the innovation have the distance attribute, the spatial relationship of the regional innovation with the geographical features only appears to be rough, and there is a certain deviation from the fact. As a systematic activity, regional innovation is bound to be affected by many other non-geographical adjacent factors, such as the degree of economic development and the difference of infrastructure. Therefore, we need to depict more complex spatial connections of innovation from the point of view of economic characteristics. In this paper, the social and economic characteristics of regions are divided into economic base and human capital, and the spatial weight matrix is established respectively. The potential meaning is that the more developed the economy in a region, the more basic elements represented by human capital, the higher the efficiency of the innovation overflow in the neighbouring area, and the improvement of its own innovation efficiency.
The form of economic distance space weight matrix is as follows:
(10)
(10)
(11)
(11) where
is the spatial distance weight matrix,
is the GDP mean value of the province
during the observation period, and
is the GDP mean value is the total observation period.
The spatial weight matrix of human capital distance.
The spatial weight matrix of human capital is as follows:
(12)
(12)
(13)
(13) where
is the average value of human capital, and
is the mean value of human capital in the total observation period.
The above spatial weight matrix is set up in both socio-economic factors and geographical distance factors. Therefore, the above spatial weight matrix can show that the areas with high economic development level or human capital level have stronger spatial influence and radiation effect on the economic level or the low human capital level. For example, the influence intensity of Beijing on Hebei is obviously greater than that of Hebei on Beijing.
3.4. Moran’s I
Because of the need to verify the spatial correlation between variables in the construction of spatial model, it is usually necessary to use Moran’s I to test the spatial correlation of variables.
The indicators are constructed as follows:
(14)
(14)
where
represents the observed value of the
spatial element,
is the number of the space elements, the space
is the spatial weight matrix element. The range [–1, 1] of the Moran’s I indicates the spatial positive correlation of economic behaviour when the value is greater than 0. There are similar properties, and there is a spatial correlation between economic behaviour when it is less than 0. The absolute value of Moran’s I represents the degree of spatial correlation. The larger the absolute value is, the greater the degree of spatial correlation is, or the smaller the degree of spatial correlation is.
4. Variable and data
Based on the existing research, this paper uses two kinds of agency indicators to measure regional innovation. First, from the perspective of innovation input, we use R&D expenditure (the amount of scientific and technological activities funds) to represent the innovation in provinces of China. Second, from the perspective of innovation output, it is expressed by the patent authorisation of local legal entity or natural persons in provinces of China. On the one hand, the patent of the invention is used to represent independent innovation at the national, regional or enterprise level, and the way is used by literature extensively (Hashmi & Van Biesebroeck, Citation2016). On the other hand, compared with the form of application, the patent in the form of authorisation obviously reflects the substantive connotation of innovation. The data are derived from China Statistical Yearbook on Science and Technology.
The migration is the core explanatory variable, and it is expressed by the rate of internal migration in each region and calculated in the form of internal migration divided by the average population at the end of the year during the survey period. The data came from the sample data of 1% population sample survey in 2005, 2010 and 2015, and 1% population change survey sample data in other years.
In order to ensure the robustness of the model estimation results, we add the relevant control variables.
4.1. The growth rate of per capita GDP
In the base period of 1997, we adjusted the GDP deflator index in all provinces of China, divided by the total population, and finally calculated the growth rate of per capital GDP. The index can reflect the difference of economic development level, technical level and the scale of domestic demand in different provinces, all of which are important factors that affect the activity and the difference of regional innovation.
4.2. Human capital
Human capital is an important factor that influences innovation (Cohen, Citation2010; Sun & Shen, Citation2009). Usually, there is no direct measure of human capital, so it is mostly measured by the level of education per capita. Based on the measurement of human capital (Zhang et al., Citation2016), the index variables of per capita education in different provinces of the corresponding years are calculated. The relevant data come from China Population & Employment Statistics Yearbook and China Statistics Yearbook.
4.3. Industrial structure
Because there are some differences in the development of industrial structure among the regions in China, the level of innovation will be affected (Liang & Zhan, Citation2006; Yu & Liu, Citation2011). This paper is measured by dividing the added value of the tertiary industry by the added value of the secondary industry in each province.
4.4. Regional innovation environment
The urbanisation rate is used to express the environment of regional innovation. In general, it is directly related to the level of economic development. The higher the regional economic development, the higher the rate of urbanisation. In addition, the urbanisation rate also reflects the concentration level of the region to a certain extent. Regional economic development and agglomeration constructed the environment of regional innovation, and have an important influence on regional innovation. In order to facilitate, the rate of urbanisation is calculated by dividing the urban population by the total population at the end of the year.
4.5. The extent of opening to the outside world
From both macro and micro perspectives, many studies found that trade opening was an important factor to affect innovation activities (Bloom et al., Citation2013). Regarding export, the effects of export on China's innovation activities are as follows. On the one hand, exports can promote the improvement of China's micro-sector innovation ability through learning effect (Wang & Yu, Citation2012). On the other hand, exports may also lead Chinese micro-enterprises to be locked at the lower end of the global value chain controlled by developed countries, curbing the improvement of China's ability to innovate independently (Zhang et al., Citation2007). Regarding import, because the imported goods contain a certain proportion of advanced production equipment and key spare parts with high technical content, it is not only possible to form a complementary effect on the innovation activities of the micro-enterprise sector (learning effect in import), but also possible to produce alternative effects (competitive effects) (Kasahara & Rodrigue, Citation2008; Lu & Ng, Citation2012). Whatever the effect of imports on innovation activities is dominant, import factors need to be controlled.
4.6. Technology spill-over
In the context of economic globalisation, regional foreign economic relations are becoming closer. The expansion of international trade and international investment accelerates the dissemination of knowledge, information and technology. The technology spill-over effect will have a great impact on regional innovation. Usually, technology spill-over can be expressed by the total amount of foreign capital actually utilised in that year. Due to the fact that the actual amount of foreign investment is denominated by United States dollars, the average annual exchange rate value of official publications is converted into the corresponding RMB. In order to ensure the comparability of the obtained data, the price fluctuation factors need to be eliminated so that 1997 is the base period. We use the consumer price index to adjust the actual utilisation of foreign capital in the current year. The exchange rate comes from the World Bank Database, and the total amount of foreign capital actually utilised in that year comes from the Statistical Yearbooks of the provinces over the years.
5. Empirical analysis
5.1. Regression without spatial effects
reports the results of internal migration on regional innovation without spatial effects. The fixed-effect model and the random-effect model are used for estimation. According to the Hausman test, the fixed-effect model is more suitable. Therefore, we mainly analyse the results of the fixed-effect model.
Table 1. Definitions and related descriptions of variables.
Table 2. The impact of internal migration on regional innovation.
The results show that the coefficient of internal migration is positive which indicates that internal migration has a significant positive impact on regional innovation for both innovation inputs and innovation outputs. That is, the higher rate of the regional migration, the higher the regional innovation. Man is the carrier of knowledge, and migration provides an effective way for the accumulation of human capital and knowledge spill-over. On the one hand, migration can affect regional innovation directly by increasing the accumulation of local human capital. On the other hand, the knowledge contained in migration will also have an indirect effect on regional innovation through spill-over effects. This kind of knowledge overflow includes both the overflow of explicit knowledge and the overflow of implicit knowledge. Stealth knowledge is generally produced in production practice, and its spread and diffusion require full proximity of geographical space. Migration can bring the opportunity to exchange information, ideas and technologies. It promotes the spread and overflow of hidden knowledge, thereby increasing the output of regional innovation. Immigration has injected new vitality into the innovation of regional development and created the conditions for the improvement of regional innovation. Internal migration changes the geographical spatial distribution of population and optimises the allocation of factors of production. Therefore, the scale and quality of migration must have an important impact on regional innovation. The estimate of the coefficient of education is positive, which indicates that there is a significant positive correlation between regional innovation and human capital. In the case of other variables unchanged, the amount of invention and the investment of R&D will be improved when the average level of education in the region increased. In the era of the knowledge economy, the competition between countries or regions is a competition of talent and human capital has become the main force to promote regional innovation and even regional economic development. Therefore, the higher the education in a region, the higher the region's human capital stock, the greater the region's ability to transform knowledge into new products, processes and services, and the greater the driving force for regional innovation. The coefficient of urbanisation rate is positive, which indicates that the urbanisation rate plays a significant positive role in promoting regional innovation. The urbanisation rate reflects regional economic development and concentration to a certain extent. It indicates that urbanisation had a positive effect on regional innovation. The influence coefficient of the actual utilisation of foreign capital on regional innovation is significantly positive, i.e. technology spill-over has a positive effect on improving innovation. The coefficient of import is significantly negative. Due to the fact that imported goods and products can make up for the demand of the domestic market to a certain extent, domestic enterprises can reduce the demand of innovation promotion.
5.2. Regression with spatial effects
5.2.1. Space self-correlation test
In order to test whether regional innovation is random or specific in distribution, it is necessary to conduct space self-correlation test to judge whether the model of space measurement is suitable for the analysis. Based on the setting of the above model, index selection and the construction of space weight matrix, the global self-correlation analysis of the innovation of 31 provinces (or cities) in China during the period 2006–2017 is carried out using the Moran’s I test, and the results are shown in .
Table 3. Test results of the Moran’s I of regional innovation in China.
show that the Moran's I value of innovation always fluctuates above 0.1, which deviated significantly from random distribution. Therefore, there is significant global spatial correlation in the innovation of provincial regions in the sample area which needs to be analysed empirically by constructing spatial model. Firstly, the appropriate model is selected by LR test and Wald test. The test results show that the original hypothesis of spatial lag model and spatial error model is rejected at 1% level. It indicated that the spatial Durbin model should be selected. At the same time, for the spatial Durbin model, the Hausman test shows that the fixed-effect model should be selected. In order to compare with the results of the spatial Durbin model, the spatial self-regression model, the estimation result of the spatial error model and the robustness of the estimation result are also presented in the following analysis. In addition to the spatial weight matrix based on geographical distance, the economic distance space weight matrix and the human capital space weight matrix are also introduced. This paper used maximum likelihood method to estimate each model.
5.2.2. The impact of internal migration on the input of regional innovation
show that the goodness of fit in each model is high, and the statistics have passed in the test of 1%, which shows that the fit of the model is better and the overall credibility is higher. Whether based on the spatial weight matrix of geographical distance, economic distance or human capital, the influence of internal migration on the input of each province innovation is significantly positive except in the spatial weight matrix of economic distance in the SAR model. Based on China's current situation, the regions with high population inflow are mainly concentrated in the eastern cities. And because of its high potential for innovation, the government will increase the input of innovation naturally. In addition, the impact of urbanisation rate (urb) and total foreign capital utilisation (lnfi) on R&D investment is significantly positive, but the effect of industrial structure (ISP) on R&D investment is significantly negative. These results are consistent with the reality of China. The relatively high urban population also reflects the high level of concentration in the region, thus investing in R&D funds is also higher. The higher the total amount of foreign capital utilisation, the closer regional and foreign economic ties are, and investment in R&D funds is also higher.
Table 4. Empirical results of spatial measurement of R&D investment.
In the SDM model, from the result of spatial weighting coefficient, the different spatial weight matrix models all show that internal migration will have a significant negative impact on the innovation output of adjacent regions. Due to the region absorbing a large amount of young labour from the adjacent regions, this can not only improve the labour productivity of the local labour market, but also enhance the innovation potential of the region. And because the adjacent region has lost a large amount of high-quality labour, it will have a negative impact on the investment of innovation in adjacent regions (Cai, Citation1995; Zhang & Zhu, Citation2004). The urbanisation rate will also have a significant positive impact on the innovation output of adjacent regions (Yang et al., Citation2019). In addition, industrial structure and exports will also have a negative impact on the innovation output of adjacent regions. Due to the gradual optimisation and upgrading of the local industrial structure, demand in the labour market will also be improved; in particular, high-quality labour will increase. The expansion of exports will also have a significant negative spatial spill-over effect on the adjacent region. This can be explained as an impact on the innovation input of adjacent regions when the region improves the capacity to innovate through foreign trade routes.
In the general econometric model, the regression coefficient of each explanatory variable represents the degree of its influence on the explained variable while in the spatial econometric model, the regression coefficient contains direct and indirect effects. The coefficient of the spatial lag of the explanatory variable can also have an effect on the feedback effect. Therefore, in order to ensure the preciseness of the estimated, the regression coefficients of the spatial Durbin model should be further decomposed. That is, the spatial spill-over effects should be decomposed into direct effects and indirect effects (Xu, Citation2016). Direct spill-over effects are defined as the influence of independent variables of a region on its dependent variables, and defined indirect spill-over effects as the influence of independent variables of a region on adjacent dependent variables with spatial correlation (Ugarte, Citation2011). Therefore, the estimation results of direct and indirect effects of spatial Durbin model are also listed in .
Table 5. Estimates of direct and indirect effects of R&D funds input.
shows that the migrant population will have a significant impact on the input of local innovation, but will also inhibit the innovation input of adjacent regions through indirect effects. Increase in the urbanisation rate will significantly promote the innovation input of local and adjacent areas. The optimisation of industrial structure will not only significantly reduce the innovation input, but also reduce the innovation input of adjacent regions through indirect effects. At the same time, according to the results of the direct effects, the utilisation of foreign capital has a significant positive impact on the region's innovation input. The indirect effect of exports has a significant negative effect on innovation investment of the adjacent area. On the whole, the spatial effect decomposition is similar to the previous results.
5.2.3. The impact of internal migration on the output of regional innovation
According to , in the spatial Durbin model, the coefficient of internal migration on output of innovation is negative. Combining with , it can be found that although internal migration will promote the regional input of regional innovation (R&D funds), it will also have a negative impact on output of regional innovation (patent authorisation). Usually, it will take a long time from input of elements to output of innovation, but the migrant population belongs to unstable factors, and the impact on regional innovation cannot form a long-term trend. Therefore, in order to maintain the positive effect of the migrant population on innovation, it is necessary for local government to make efforts not only to introduce more proactive talent policies, but also to retain innovative talent. Meanwhile, the impact factor of education, the urbanisation rate and the actual utilisation of foreign capital on innovation output is significantly positive.
Table 6. The spatial measurement of patent authorisation for inventions.
From the result of the spatial weighting, the different spatial weight matrices of SDM models show that the internal migration and the urbanisation rate will have a significant positive impact on innovation output in the adjacent regions. The improvement of education will also have a significant negative impact on the innovation output of adjacent regions in the geographical distance weight matrix and human capital spatial weight matrix SDM model. In addition, the different spatial weight matrices of SDM models show that the actual utilisation of foreign capital, imports and exports also have negative effects on innovation outputs of adjacent regions.
presents the results of direct and indirect effects of innovative output. The results show that although migration will have a significant negative impact on output of innovation, it will also promote innovation output significantly in adjacent regions through indirect effects, and have a positive impact on the improvement of overall innovation. Tsvetkova et al. (Citation2020), builds on recent developments in evolutionary economics combined with a more traditional spill-overs perspective. The results suggest reinforcing dynamics between proximity to the metropolitan industry mix and metropolitan patenting intensity in promoting survival of non-patenting companies. Education will promote innovation output in a region directly, but it will also have an inhibited effect on the adjacent areas through indirect effects. The increase of urbanisation rate will significantly promote innovation output and that of its adjacent regions, and the optimisation of industrial structure will also reduce the level of innovation output directly. The actual utilisation of foreign capital will have a positive impact on innovation of the local region through direct effects, but it will have a negative impact on the innovation output of adjacent regions though indirect effects. At the same time, both imports and exports will have a significant negative impact on the innovation output in neighbouring regions through indirect effects.
Table 7. The direct and indirect effects of patent authorisation of inventions.
6. The conclusion and enlightenment
Based on the panel data in China, the paper analyses the effect of internal migration on regional innovation by building a panel model and a space model. The results show the following. First, internal migration has a significant positive impact on regional innovation according to the regression without spatial effects. That is, the higher the rate of migration, the higher the regional innovation. In the spatial model, although internal migration will promote regional input of regional innovation, it will also have a negative impact on output of regional innovation. Usually, it will take a long time from input of elements to output of innovation, and the migrant population is an unstable factor. So, the impact on regional innovation cannot form a long-term trend. Therefore, in order to maintain the positive effect of migration on innovation, it is necessary for local government to make efforts not only to introduce more proactive talent policies, but also to retain innovative talent. Meanwhile, the impact factor of education, the urbanisation rate and the actual utilisation of foreign capital on innovation output is significantly positive.
Second, internal migration not only has a significant promoting effect on improving regional innovation, but also shows a significant spatial agglomeration phenomenon. From the results of spatial model, internal migration will have a significant negative impact on innovation input of adjacent regions and a significant positive impact on innovation output of adjacent regions due to the region absorbing a large amount of young labour from adjacent regions, which cannot only improve the labour productivity of the local labour market, but also enhance the innovation potential of the region. And because the adjacent region has lost a large amount of high-quality labour, it will have a negative impact on the investment of innovation in adjacent regions.
Third, the estimation results of the direct and indirect effects show that, from the input of innovation, the migrant population will have a significant impact on local innovation, but will also inhibit the innovation input of adjacent regions through indirect effects. From the output of innovation, although migration will have a significant negative impact on the output of innovation, it will also promote innovation output significantly in adjacent regions through indirect effects, and have a positive impact on the improvement of innovation overall.
Combined with the conclusions of this paper, the following policy suggestions are put forward. First, there is inconsistency between input and output in the influence of internal migration on the spatial spill-over effect of regional innovation. In order to maintain the positive effect of migration on innovation, it is necessary for local government to make efforts not only to introduce more proactive talent, but also to retain innovative talent.
Second, in order to promote the improvement of productivity, we should improve the level of urbanisation and increase investment in higher education. At present, China has entered the new normal of slow economic growth. In order to maintain innovation vitality and promote the sustained and healthy growth of the economy, it is necessary to further increase investment in education in order to reserve sufficient human resources and innovation.
Third, in order to prevent a gap in regional innovation and to avoid further widening the gap in regional economic development, financial support for the central and western regions should be strengthened.
Disclosure statement
No potential conflict of interest was reported by the authors.
Additional information
Funding
References
- Bloom, N., Romer, P. M., Terry, S. J., & Reenen, J. V. (2013). A trapped-factors model of innovation. American Economic Review, 103(3), 208–213. https://doi.org/https://doi.org/10.1257/aer.103.3.208
- Bosetti, V., Cattaneo, C., & Verdolini, E. (2015). Migration of skilled workers and innovation: A European perspective. Journal of International Economics, 96(2), 311–322. https://doi.org/https://doi.org/10.1016/j.jinteco.2015.04.002
- Cai, F. (1995). Causes, trends and policies of migration and movement. Chinese Journal of Population Science, 51(06), 8–16. doi: CNKI:SUN:ZKRK.0.1995-06-001
- Cai, G. W., & Qian, J. B. (2013). The origin of spatial correlation: A theoretical model and empirical evidence. China Economic Quarterly, 12(3), 869–894. https://doi.org/https://doi.org/10.13821/j.cnki.ceq.2013.03.009
- Chellaraj, G., Maskus, K. E., & Mattoo, A. (2008). The contribution of international graduate students to US innovation. Review of International Economics, 16(3), 419–444. https://doi.org/https://doi.org/10.1111/j.1467-9396.2007.00714.x
- Cohen, W. M. (2010). Fifty years of empirical studies of innovative activity and performance. In B. H. Hall & N. Rosenberg (Eds.), Handbook of the economics of innovation (Vol. 1, pp. 129–213). Elsevier. https://doi.org/https://doi.org/10.1016/S0169-7218(10)01004-X
- Mare, D. C., Fabling, R. M., & Stillman, S. (2011). Immigration and Innovation [CReAM Discussion Paper Series 1110]. Centre for Research and Analysis of Migration (CReAM), Department of Economics, University College, London.
- Faggian, A., & Mccann, P. (2008). Human capital, graduate migration and innovation in British regions. Cambridge Journal of Economics, 33(2), 317–333. https://doi.org/https://doi.org/10.1093/cje/ben042
- Fan, Z. B., & Liu, D. X. (2012). Knowledge composition: Emigration and economic growth. World Economic Study, 31(3), 10–16. https://doi.org/https://doi.org/10.13516/j.cnki.wes.2012.03.002
- Freeman, C. (1997). The economics of industrial innovation. Social Science Electronic Publishing, 7(2), 215–219. https://doi.org/https://doi.org/10.1016/0144-8188(83)90019-4
- Frosch, K., & Tivig, T. (2009). Age, human capital and the geography of innovation. Thuenen-Series of Applied Economic Theory 71, University of Rostock, Institute of Economics. http://hdl.handle.net/10419/39773.
- Gao, X. X., Rui, X. Q., & Song, Y. (2014). Dynamic research on regional innovation capability in China: Based on panel data during 2001 to 2010. Science & Technology Management Research, 34(2), 15–19. https://doi.org/https://doi.org/10.15957/j.cnki.jjdl.2015.06.013
- Hashmi, A. R., & Van Biesebroeck, J. (2016). The relationship between market structure and innovation in industry equilibrium: A case study of the global automobile industry. Social Science Electronic Publishing, 98(1), 1–41. https://doi.org/https://doi.org/10.2139/ssrn.1984567
- Huang, R., Liang, Q. J., & Lu, L. C. (2014). Relationship of urban population structure and innovation ability: A case study of urban China. Urban Development Studies, 21(9), 84–91. https://doi.org/https://doi.org/10.3969/j.issn.1006-3862.2014.09.013
- Hunt, J., & Gauthier-Loiselle, M. (2010). How much does immigration boost innovation? American Economic Journal: Macroeconomics, 2(2), 31–56. https://doi.org/https://doi.org/10.2307/25760296 https://doi.org/https://doi.org/10.1257/mac.2.2.31
- Kasahara, H., & Rodrigue, J. (2008). Does the use of imported intermediates increase productivity? Plant-level evidence. Journal of Development Economics, 87(1), 106–118. https://doi.org/https://doi.org/10.1016/j.jdeveco.2007.12.008
- Lee, N., & Nathan, M. (2010). Knowledge workers, cultural diversity and innovation: Evidence from London. International Journal of Knowledge-Based Development, 1(1/2), 53–78. https://doi.org/https://doi.org/10.1504/IJKBD.2010.032586
- Liang, Q., & Zhan, Y. J. (2006). Regional specialization, Technology progress and industrial upgrading: Proved by Yangtze River delta. Economic Theory & Business Management, 26(1), 58–64. doi: CNKI:SUN:JJLL.0.2006-01-009.
- Lissoni, F. (2018). International migration and innovation diffusion: An eclectic survey. Regional Studies, 52(5), 702–714. https://doi.org/https://doi.org/10.1080/00343404.2017.1346370
- Lu, Y., & Ng, T. (2012). Do imports spur incremental innovation in the South? China Economic Review, 23(4), 819–932. https://doi.org/https://doi.org/10.1016/j.chieco.2012.04.005
- Ma, M. (2016). Study of the nonlinear relationship between adjacent regional human capital and regional innovation capability. Journal of Technical Economics & Management, 37(10), 119–123.
- Niebuhr, A. (2010). Migration and innovation: Does cultural diversity matter for regional R&D activity? Papers in Regional Science, 89(3), 563–585. https://doi.org/https://doi.org/10.1111/j.1435-5957.2009.00271.x
- Ozgen, C., Nijkamp, P., & Poot, J. (2011). The impact of cultural diversity on innovation: Evidence from Dutch firm-level data. Social Science Electronic Publishing, 2(1), 1–24. https://doi.org/https://doi.org/10.1186/2193-9039-2-18
- Ozgen, C., Peters, C., Niebuhr, A., Nijkamp, P., & Poot, J. (2014). Does cultural diversity of migrant employees affect innovation? International Migration Review, 48(1_suppl), 377–416. https://doi.org/https://doi.org/10.1111/imre.12138
- Partridge, J., & Furtan, W. H. (2008). Increasing Canada's international competitiveness: Is there a link between skilled immigrants and innovation? 2008 Annual Meeting, July 27-29, 2008, Orlando, Florida 6504, American Agricultural and Applied Economics Association.
- Poot, J. (2008). Demographic change and regional competitiveness: The effects of immigration and ageing. International Journal of Foresight & Innovation Policy, 4(1/2), 129–145. https://doi.org/https://doi.org/10.1504/IJFIP.2008.016910
- Qian, X. Y., Chi, W., & Li, B. (2010). The role of human capital in regional innovation activities and economic growth: Spatial econometric study. The Journal of Quantitative & Technical Economics, 27(4), 107–121. https://doi.org/https://doi.org/10.1016/S1876-3804(11)60009-8
- Shi, H. N. (2013). Urbanization, population concentration and human capital spillovers under China's rapid process of urbanization. Economic Geography, 33(10), 56–62.
- Simonen, J., & Mccann, P. (2008). Firm innovation: The influence of R&D cooperation and the geography of human capital inputs. Journal of Urban Economics, 64(1), 146–154. https://doi.org/https://doi.org/10.1016/j.jue.2007.10.002
- Sun, W. J., & Shen, K. R. (2009). Industry characteristic, Human capital accumulation path and the difference of innovative of Chinese industrial enterprises. China Industrial Economics, 27(3), 81–91. https://doi.org/https://doi.org/10.19581/j.cnki.ciejournal.2009.03.008
- Tsvetkova, A., Conroy, T., & Thill, J. C. F. (2020). Surviving in a high-tech manufacturing industry: The role of innovative environment and proximity to metropolitan industrial portfolio. International Entrepreneurship & Management Journal, 16(2), 501–527. https://doi.org/https://doi.org/10.1007/s11365-019-00591-8
- Ugarte, M. D. (2011). Introduction to spatial econometrics. Journal of the Royal Statistical Society, 174(2), 513–514. https://doi.org/https://doi.org/10.1111/j.1467-985x.2010.00681_13.x
- Wang, C. L. (2009). Human capital spillover and independent innovation ability. Science & Technology Management Research, 29(7), 338–340. doi:CNKI:SUN:KJGL.0.2009-07-108
- Wang, G. X., & Huang, Y. Y. (2005). Inter-provincial migration and economic development in East China: 1995-2000. Population Research, 29(1), 19–28. https://doi.org/https://doi.org/10.3969/j.issn.1000-6087.2005.01.003
- Wang, Y. H. (2015). The study of the non-linear relationship between human capital and regional innovation ability in China: Based on the empirical evidence of the panel threshold model. On Economic Problems, 37(06), 52–55. https://doi.org/https://doi.org/10.16011/j.cnki.jjwt.2015.06.009
- Wang, Z., & Yu, Z. (2012). Trading partners, Traded products, and firm performances: Evidence from China’s exporter-importers. The World Economy, 35(12), 1795–1824. https://doi.org/https://doi.org/10.1111/twec.12019
- Xu, C. H. (2016). The tendency of general rate of profit to fall in post-crisis Era: Performance, national differences and influencing factors. The Journal of World Economy, 39(5), 3–28.
- Yang, Q. (2015). A study of the interaction mechanism of population migration and regional innovation:Based on a simultaneous equations model. Northwest Population, 36(5), 22–27. https://doi.org/https://doi.org/10.15884/j.cnki.issn.1007-0672.2015.05.004
- Yang, W., Yao, C., & Su, M. Y. (2019). The level of urbanization affects the regional differences and spatial dependence of innovation output: An empirical analysis based on non-spatial panel and spatial panel model. China Soft Science, 34(7), 71–101.
- Yu, P. L., & Liu, R. (2011). An empirical study on the influence of regional industry structure on innovation performance. Journal of Northeastern University (Natural Science), 32(12), 1786–1789. https://doi.org/https://doi.org/10.1007/s11769-011-0450-8
- Yu, X., Li, Y., & Lei, J. Y. (2013). Analysis on China's provincial population migration and its impact on regional economic development since the new century. Population Journal, 35(3), 5–14. https://doi.org/https://doi.org/10.3969/j.issn.1004-129X.2013.03.001
- Zhang, H., & Shi, L. (2018). Human capital and regional innovation: An empirical study based on spatial Durbin model. Journal of Hunan University (Social Sciences), 32(05), 55–63. https://doi.org/https://doi.org/10.16339/j.cnki.hdxbskb.2018.05.008
- Zhang, J., Liu, Z. B., & Zheng, J. H. (2007). Industrial chain positioning, divide, agglomeration and innovation: An empirical study based on questionnaire of manufacturing firms in Jiangsu province. Chinese Industrial Economy, 25(7), 39–47. https://doi.org/https://doi.org/10.19581/j.cnki.ciejournal.2007.07.006
- Zhang, J., Yang, L. X., & Xin, F. (2016). Does real estate hinder innovation in China? An explanation based on the maturity structure of loans in the financial system. Management World, 32(5), 64–80. https://doi.org/https://doi.org/10.19744/j.cnki.11-1235/f.2016.05.007
- Zhang, W. X., & Zhu, L. (2004). An assessment and researches on migration in China in last decade. Human Geography, 19(2), 88–92. https://doi.org/https://doi.org/10.13959/j.issn.1003-2398.2004.02.020
- Zuo, X. J., & Wang, H. X. (2009). International comparison of metropolitan innovation and population development: A case study of New York, Tokyo, London and Shanghai. Social Sciences, 31(2), 44–52. doi:CNKI:SUN:SHKX.0.2009-02-008