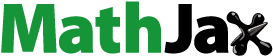
Abstract
Research in strategic human resource management indicates that high performance work systems (HPWS) have a positive impact on the overall performance of an organization as a result of better human resource (HR) outcomes. Regarding the multi-dimensional and complex nature of these factors, common statistical models are not useful for examining the performance of HPWS. Using the capabilities of multi-attribute decision-making (MADM) methods to deal with various criteria that may be contradictory, this study proposes a MADM-based framework that provides the opportunity to prioritize HR practices. Based on this framework, high-performance HR practices and their related HR outcomes were identified after studying the theoretical literature and ascertaining the views of decision-makers and HR experts. Then, after looking at the interactions among HR outcomes, the weights of the criteria were calculated using the method of the decision making trial and evaluation laboratory (DEMATEL). Then, the alternatives were ranked using the multi-attributive border approximation area comparison (MABAC) method. Finally, the designed framework was implemented in an organization active in the banking industry. This framework can be used to improve employees’ performance and, consequently, the performance of the organization. Accordingly, taking into account the resource constraints organizations face, the priorities presented can be helpful in budgeting human-resource-management (HRM) improvement projects and making an appropriate resource allocation for this.
1. Introduction
Over the past two decades, high-performance work systems (HPWS) have attracted a great deal of attention both from academic researchers and practitioners who have been looking to gain a sustained competitive advantage by investing in human capital (Chen et al., Citation2018). Organizations that focus on their employees believe that employee attitudes and behaviors play a key role in satisfying their customers and subsequently play a key role when one is looking to improve the performance of an organization (Cheah et al., Citation2019; Jha et al., Citation2017). This is even more important in the service industries such as banking. In this sector, the quality of communication between frontline employees and customers is one of the most important determinants of customer satisfaction and thus organizational performance (Paes de Faria et al., Citation2020; Ranjan et al., Citation2015). The philosophy derived from the strategic human resource management (SHRM) approach in which interests are based on the design of a set of high-performance work systems is called HPWS. HPWS is a set of human resource (HR) practices that leverage organizational human and social capital to gain a competitive advantage for a firm (Guthrie et al., Citation2009; Huselid, Citation1995).
Much of the academic SHRM research has emphasized how HPWS is a bundle of HR practices that ought bring about positive organizational outcomes, including customer satisfaction (Ogbonnaya & Valizade, Citation2018), return on investment (ROI) (Katou, Citation2011), organizational coordination (Fu et al., Citation2019), market share, organizational innovation (Bin Saeed et al., Citation2019; Fu et al., Citation2015), and workforce productivity (Datta et al., Citation2005; Guthrie, Citation2001; Guthrie et al., Citation2009; Huselid, Citation1995; MacDuffie, Citation1995).
Research findings indicate that HPWS has a positive impact on the overall performance of an organization through an improvement of HR outcomes such as employee job satisfaction, organizational commitment, organizational citizenship behavior, and employee empowerment (Messersmith et al., Citation2011). A survey conducted by Takeuchi et al. (Citation2007) of both managers and employees shows that the average performance of managers and employees can increase significantly after the implementation of HPWS.
Despite the importance of this issue, few studies have examined the effects of implementing HPWS in the banking industry (Cooke et al., Citation2019; Kloutsiniotis & Mihail, Citation2018; Liao et al., Citation2009). In this context, the research conducted by Cooke et al. (Citation2019) confirms the link in the Chinese banking industry between the implementation of HPWS and an improvement in employee resilience and engagement. According to the results of this study, when employee resilience, which is a set of individual skills and characteristics, is improved, this can have benefits both at the individual and organizational levels due the effective application of HPWS. In another study that looked at the banking industry, Mahmood et al. (Citation2019) confirmed that HPWS has a positive effect on organizational commitment of employees by enhancing their levels of job satisfaction.
There is general agreement among human resource management (HRM) researchers and scholars that a systematic approach to HR practices is better than a one-dimensional approach. Nevertheless, there is still no consensus as to what exactly is to be included in the combination of practices that constitute HPWS (Boxall & Macky, Citation2007; Cooke et al., Citation2019; Van De Voorde & Beijer, Citation2015). Therefore, it is important to choose the right practices for HPWS that help an organization be better aligned with its goals and strategies in order to achieve its desired HR outcomes and ultimately improve its organizational performance.
However, despite the important role HPWS can have on HR outcomes and thus the performance of an organization, there are still no precise indicators and criteria for measuring the impact of high-performance HR practices, and there is no approach yet that takes into account their impact on how to best select for the most effective practices. Some researchers argue that common statistical models do not have the capability of examining the performance of HPWS due to their simplicity and superficiality. Nor, these same researchers argue, can these models account for the complex and multi-dimensional nature of decision-making on the choice of high-performance HR practices that would optimally impact HR outcomes (Zhang et al., Citation2014).
In order to compensate for this limitation, multi-attribute decision-making (MADM) methods are used in this study. Due to the high capability of MADM methods for choosing the best alternative based on a number of criteria, some of which may be in contradiction with each other, these methods have become one of the most popular topics in the decision theory literature (Amiri et al., Citation2020; Barak & Dahooei, Citation2018; Stanujkic et al., Citation2015; Yazdani et al., Citation2019). These methods have also been used in the field of HRM on certain issues such as performance management, staff selection, and recruitment (Abdullah & Zulkifli, Citation2015; Balezentis et al., Citation2012; Beskese et al., Citation2018; Chou et al., Citation2012; Dursun & Karsak, Citation2010; Ensslin et al., Citation2013; Heidary Dahooie et al., Citation2018; Horváthová et al., Citation2019; Kelemenis & Askounis, Citation2010; Mammadova & Jabrayilova, Citation2018; Polychroniou & Giannikos, Citation2009; Rahmanniyay & Yu, Citation2019; Sang et al., Citation2015; Tuana, Citation2018; Zhang & Liu, Citation2011).
The main objective of this study is to use MADM develop a framework for decision-making regarding the selection of high-performance HR practices that best maximize their impact on the desired HR outcomes, all in order to gain a deeper understanding of the performance of HPWS and to avoid the limitations inherent to current analytical methods. This framework can provide HR decision-makers with a systematic approach for making more effective decisions while enabling them to analyze and consider all of the relevant criteria. Given the interrelations among the various HR outcomes and the fact that a few MADM methods take into account the interrelations among the criteria, a combination of decision-making trial, evaluation laboratory (DEMATEL), and multi-attributive border approximation area comparison (MABAC) methods is used in this study.
The rest of this article is structured as follows. Section 2 deals with the theoretical foundations of the study. The executive steps of the research are presented in section 3. Section 4 outlines the methods used in this study. Section 5 is devoted to presenting the findings from the implementation of the proposed framework in a real case. Finally, an analysis of the research findings and conclusions are presented in Sections 6 and 7, respectively.
2. Literature review
2.1. High-performance work systems
HPWS is a bundle of HR practices derived from HRM best practices that are said to lead to a superior level of performance in organizations (Takeuchi et al., Citation2007). These set of HR practices are internally coordinated and reinforce each other in order to achieve inclusive goals (Lepak et al., Citation2006). In addition, the HR practices that simultaneously reinforce one other, according to the systematic approach, create synergistic effects that deliver the effects of the sum of practices beyond the sum from the practices individually. This set of HR practices is well known in the current literature on strategic HRM as high-performance work systems (HPWS) or high-performance work practices (HPWP). However, other studies give it titles such as high-involvement work practices (HIWP) (Guthrie et al., Citation2009), high-performance management practices (Zacharatos et al., Citation2005), human-capital-enhancing HR systems (Youndt et al., Citation1996), and high commitment work practices (HCWP) (McClean & Collins, Citation2011).
Regardless of its title, HPWS comprises a set of HR practices that are integrated and aligned both vertically and horizontally. This set of high performance HR practices collectively transform an organization’s employees into information-driven, motivated, and action-oriented individuals. Although there is still no clear consensus on the ideal configuration for HPWS (Cooke et al., Citation2019), previous studies suggest that the most important practices include training, selective recruitment, performance-based pay, employee career path, performance management, teamwork, job design, and employee participation programs. These studies have specifically emphasized the adoption of these practices for service-oriented industries (Aryee et al., Citation2012; Cooke et al., Citation2019; Figueiredo et al., Citation2016; Gibbs & Ashill, Citation2013; Liao et al., Citation2009; Ning et al., Citation2018; Wang & Xu, Citation2017).
Although there is agreement among HRM experts and scholars that the systematic approach to HR practices is more appropriate than the one-dimensional approach, there is still no consensus concerning the best composition of the practices that form the HPWS in an organization (Boxall & Macky, Citation2007; Cooke et al., Citation2019; Van De Voorde & Beijer, Citation2015). Therefore, researches in the field emphasize the need for providing a framework for selecting the optimal combination of these practices at the organizational level.
2.2. HPWS–Performance relationship
Most researchers believe that HPWS is one of the most important sources of attaining a competitive advantage (Guthrie et al., Citation2009; Huselid, Citation1995). For this reason, many of the analyses of HPWS comes from SHRM researchers who have examined the impact of HR practices on organizational outcomes (Jiang et al., Citation2012). An important point emphasized in the literature is how HPWS has strong and positive effects on employees and organization performance (Combs et al., Citation2006). Although many steps have been taken in recent years to understand how to obtain the best level of performance for HRM, there are still many uncertainties regarding the relationship between HPWS and organizational performance (Boxall et al., Citation2011; Chuang & Liao, Citation2010; Messersmith et al., Citation2011; Sun et al., Citation2007).
What is clear is that HPWSs can positively influence individual and organizational performance. According to Boxall (Citation2012), HPWS positively affects employee outcomes and behaviors at the individual level before affecting an organization’s performance. Based on a common framework, ability motivation opportunity theory (AMO), HPWS is said to positively influence employee performance by affecting the abilities, motivation, and their opportunity to participate (Jiang et al., Citation2012).
Experimental and theoretical studies in the field of SHRM suggest that there are various mechanisms involved in shaping the individual and collective characteristics of employees in a way that positively influences organizational performance (Lepak et al., Citation2006). Accordingly, one can adopt a macro-level approach, in which the impact of HR practices on organizational performance is examined (Combs et al., Citation2006), or adopt a micro-level approach, in which the main focus is on the impact of HR practices on employee performance when examining the impact of HPWS (Haar & White, Citation2013).
As shown in , HPWS directly or indirectly affects the performance of an organization. Accordingly, HPWS shapes employee performance by means of HR outcomes, and their performance ultimately influences the organization’s performance.
The HR outcomes that are considered central to mediating between HPWS and employee performance (Cooke et al., Citation2019) include job satisfaction, the rate of absenteeism, organizational commitment, occupational safety, employee resilience, and employee motivation.
By looking at , one can see the relationship between HPWS and organizational performance with regard to the HR outcomes that can improve performance and have effects as a mediating mechanism. In this study, when considering these relationships, more of an emphasis is placed on analyzing the first part, namely the relationships among employee performance, HR outcomes, and HPWS in terms of the micro-level approach. This is the focus of the research. An important point in exploring the interrelations among the outcomes that can positively influence the performance of employees. Therefore, while analyzing these relationships, in addition to considering the direct impact of each outcome on performance, it is essential to consider the impact of the outcomes on each other and ultimately on organizational performance. This demonstrates once again the need to apply an appropriate methodology for analyzing these interrelations.
In this study, after reviewing the theoretical literature, a list of HR outcomes by which HPWS can influence the performance of employees was prepared. The list was presented to the experts for final selection. The identified HR outcomes are listed in .
Table 1. Human resource outcomes list based on literature review.
3. Research steps
The purpose of this study is to provide a framework for selecting the best high-performance HR practices for organizations. As explained above, the basis for this selection is how each of the identified HPWS impacts provide significant HR outcomes that ultimately lead to improvements in organizational performance. The research process is presented in .
As noted in , the initial list of HR practices and HR outcomes are first identified through a review of the literature. This list is then made available to an HR committee, which in turn selects a number of HR outcomes that are aligned with banking industry strategies. The committee does so by modeling HRM key performance indicators (KPIs) and considering them in terms of decision criteria. As mentioned in Section 2, the HR outcomes are not independent and are interrelated. Because of this important point, methods such as stepwise weight assessment ratio analysis (SWARA), analytic hierarchy process (AHP), and the best-worst method (BWM) are not suitable for determining the relative importance of HR outcomes and their impact on HPWS selection. In this research, the DEMATEL method was used to calculate the criteria weights among the methods that can take into account the relationships among the criteria. To this end, the committee members used their knowledge to determine the relationships among the criteria using linguistic variables. Then the criteria were weighted based on the DEMATEL method (the steps are described in Section 4). The committee then finalized the list of high performance HR practices. Specifically, HRM strategies and the views of decision-makers, particularly board members, were crucial in determining the list. The committee was then asked to express in linguistic variables the degree of impact that the HR practices have on the realization of various HR outcomes based on their specialized knowledge of HRM. In the following, the steps of the MABAC method are applied to calculate the scores of alternatives and to rank the HR practices. Finally, the designed framework is implemented in the banking industry. Specifically, the designed framework acts as a decision support tool, and the results are available to industry decision-makers with a view to how the policies and constraints they have when choosing the final list of high-performance HR practices and budget allocations.
4. Methodology
MADM methods have been developed for the purpose of ranking or evaluating a limited number of alternatives in light of criteria that may be contradictory to one other (Barak & Dahooei, Citation2018). Given the increasing importance of decision-making in daily life, numerous methods have been developed by researchers and applied in different fields (Kaklauskas et al., Citation2018; Mohagheghi et al., Citation2019; Zavadskas et al., Citation2014). One of the main reasons for the development of new approaches is that traditional MADM approaches do not consider the conditions of real-world decision-making problems. Examples of development in approaches are the extensions needed to overcome the challenge of uncertainty and hesitation during the decision-making process (e.g., fuzzy, intuitive fuzzy, hesitant sets, etc.) (Xu & Yager, Citation2008).
Many MADM approaches assume that the decision criteria are independent of one other. However, in many real-world cases, this assumption is unrealistic and there are various types of interactions among the criteria that affect the traditional decision-making process (Baykasoğlu et al., Citation2013). Despite the importance of this issue, the interactions among the criteria have received little attention in the literature (Gölcük & Baykasoğlu, Citation2016).
Gölcük and Baykasoğlu (2016) classified the criteria interactions into two categories, criterion dependency and criteria interactivity, and they identified the methods presented so far in each category. The Battelle Memorial Institute conducted a project using the DEMATEL method through its Geneva Research Centre (Gabus & Fontela, Citation1972, Citation1973). In recent years, the DEMATEL method has been widely used due to its great ability to model cause-and-effect relationships, and various MADM methods have been used in combination with it (Gölcük & Baykasoğlu, Citation2016). The MABAC method was first proposed by Pamučar and Ćirović (Citation2015). They presented a powerful new MADM combination using both DEMATEL and previous MABAC methods simultaneously. Their sensitivity analysis on this combination showed that the MABAC method has higher levels of stability and consistency than other methods such as simple additive weighting (SAW), complex proportional assessment of alternatives (COPRAS), technique of order preference similarity to the ideal solution (TOPSIS), multi-objective optimization on the basis of ratio analysis (MOORA), and vlsekriterijumska optimizcija i kaompromisno resenje (VIKOR) (Pamučar & Ćirović, Citation2015). Therefore, this new method is considered to be a useful and reliable tool for decision-making. Accordingly, due to the fact that the criteria in this study are not independent of one other and because there are causal relationships among the HR outcomes, we use the modified fuzzy DEMATEL method (Dalalah et al., Citation2011) to weight the criteria, and we used the developed MABAC method (Pamučar & Ćirović, Citation2015) to prioritize HPWSs. The steps of this hybrid method are described in the following.
Suppose there are m alternatives (Ai; i = 1,2,…,m) that are evaluated according to n different criteria (Xj; j = 1,2,…,n). The evaluation value of ith alternative according to the jth criterion is represented by xij.
Calculation the weight of each criteria.
Because each criterion may have a different impact on the choice of the best alternative, the weight of each criterion is determined as wj; j = 1,2,…,n (). At this stage of the model, the relationship between the criteria is obtained using the modified fuzzy DEMATEL method. The steps to implement this method are as follows:
Collect experts’ opinions and construct the average matrix
Given k as the number of committee members, an n × n matrix
is formed for eth expert which indicates the direct-relation between criteria (n represents the number of criteria). Each element of this matrix (
) represents the degree to which the criterion Ci affects the criterion Cj. Then the fuzzy matrix
which indicates the aggregated opinions of experts, is obtained by EquationEquation (1)
(1)
(1) .
(1)
(1)
Calculate the normalized initial direct-relation matrix
The normalized direct-relation matrix
is shown as follows:
(2)
(2)
All values of this matrix are in the range [0,1] and are calculated based on EquationEquations (3)
(3)
(3) and Equation(4)
(4)
(4) .
(3)
(3)
(4)
(4)
Calculate the total relation matrix
The total relation matrix
is obtained by EquationEquation (5)
(5)
(5) .
(5)
(5)
Here matrix I is n × n identity matrix. Accordingly, the total relation matrix is represented as EquationEquation (6)
(6)
(6) .
(6)
(6)
Where
represents the indirect effects of factor i on factor j. Then the total relation matrix
was defuzzified using EquationEquation (7)
(7)
(7) . Defuzzification was carried out using EquationEquation (7)
(7)
(7)
(7)
(7)
Calculate the sum of rows and columns of the matrix
In this step, the sum of the rows and columns of the matrix
which are represented by
and
respectively, are computed by the following equations:
(8)
(8)
(9)
(9) where n and m are the numbers of criteria and alternatives, respectively.
Calculate the initial weight coefficients of the criteria.
The initial weight of each criterion is calculated based on the EquationEquation (10)
(10)
(10) .
(10)
(10)
Normalization and calculation of the final weight coefficients of the criteria.
After determining the weight of each criterion, the weights are normalized as follows:
(11)
(11)
Ranking the alternatives
Step 1. Form the initial decision matrix X.
At this stage, each of the m alternatives is evaluated in terms of n criteria. specifies the value of alternative i according to the criterion j (i = 1,2,.,m; j = 1,2,…,n).
(12)
(12)
Step 2. Normalize the elements from the matrix X.
The elements of normalized matrix N (EquationEquation (13)(13)
(13) ) is obtained using EquationEquations (14)
(14)
(14) and Equation(15)
(15)
(15) .
Where and
are maximum and minimum values of alternatives according to a given criterion.
(13)
(13)
(14)
(14)
(15)
(15)
Step 3. Calculate the weighted matrix V.
The elements of weighted matrix V are obtained using EquationEquation (16)(16)
(16) .
(16)
(16)
Where is the element of normalized matrix N and
is the weight of criterion i (EquationEquation (11)
(11)
(11) ). Accordingly, the weighted matrix V is as follows:
(17)
(17)
Step 4. Determine the border approximation area matrix G.
At this stage, the border approximation area for each criterion is calculated based on the EquationEquation (18)(18)
(18) .
(18)
(18)
After calculating for each criterion, the border approximation area matrix G with the format
is formed.
(19)
(19)
Step 5. Calculate the distance of alternatives from the border approximation area Q.
The distance of alternatives from the border approximation area is calculated based on the difference between elements of the weighted matrix V and the border approximation area matrix G (EquationEquation (20)(20)
(20) ).
(20)
(20)
Alternative can belong to the border approximation area
upper approximate area
or lower approximate area
that is,
The upper approximate area
represents the area where the ideal alternative
is located, while the lower approximate area
presents the area where anti-ideal alternative
is located. Belonging of the alternative
to the approximate area
or
is obtained by EquationEquation (21)
(21)
(21) .
(21)
(21)
For alternative to be the best one in the set of alternatives, it is necessary to have a higher number of criteria above the upper approximate area
than the other alternatives.
Step 6. Ranking alternatives.
The final criterion functions for alternatives are calculated by summing across the rows of the matrix Q (EquationEquation (22)(22)
(22) ). The alternatives are then ranked based on the values obtained.
(22)
(22)
5. Case study
The banking industry is one of the most competitive and service-oriented industries, and it spends significant amounts on HR practices. HR plays a key role in gaining a competitive advantage because in service businesses human capital has a greater impact on organizational performance than in production organizations. In addition, service businesses are more dependent on interactions with customers. In these situations, employees need to have developed the skills and attributes that have a greater impact on their performance (Bailly & Léné, Citation2013). Despite its importance, there seems to be an insufficient understanding of HR systems in the banking industry (Cooke et al., Citation2019; Zhao et al., Citation2012). In light of this and the requirements presented in the previous sections, the framework designed in this study was used to select the best high-performance HR practices after considering HR outcomes in the banking industry in Iran.
In order to go through the decision-making process, a specialized committee was formed. The committee members were chosen based on their levels of expertise and experience in the HR field. Taking into account the need for a better evaluation of HR practices, three working groups were formed under the supervision of the main committee: the HR managers, the members of the board of directors, and the HR consultants, who were prominent university professors in the field of HRM. The latter had an academic background in HR as well as relevant work experience in the banking industry. Their expertise in HR and experience in the banking industry (at least 15 years) allowed researchers to test their proposed framework while using valid data.
According to the steps outlined in Section 3, the initial list of HR outcomes was obtained through a review of the literature, which is the initial list presented in . The list was then made available to members of each of the working groups using the fuzzy Delphi method. The working groups’ members selected a number of HR outcomes that, based on the HRM KPIs, were consistent with the industry’s strategies, and then they incorporated them as decision criteria. Finally, HR outcomes, including skills (C1), collaboration (C2), motivation (C3), organizational commitment (C4), job satisfaction (C5), turnover (C6), and presence (C7), were selected as the decision criteria.
In the next step, the DEMATEL method was used to calculate the relative importance of each identified outcome, that is, the method was used to calculate the impact of each outcome on the selection of high-performance HR practices. represents the degree of the direct relationships among the identified criteria from the perspective of each working group.
Table 2. The degree of the direct relation between criteria based on linguistic variables.
As illustrated in this table, linguistic variables were used to indicate the extent to which a criterion was influenced by another criterion. The fuzzy Likert scale used in this paper is presented in (Li, Citation2013).
Table 3. Fuzzy Likert scale to assess the extent of the impact of the criteria on each other.
Then, the direct-relation matrices of three working groups were aggregated into the average matrix using EquationEquation (1)
(1)
(1) (). For example,
(the degree to which the criterion C1 affects the criterion C3) is calculated by:
Table 4. Average direct-relation matrix (.
Next, the elements of the normalized initial direct-relation matrix were determined using EquationEquation (3)
(3)
(3) . In order to obtain the elements of the initial direct-relation matrix, it was necessary to calculate the sum of each row of elements in matrix
and then choose the maximum element
from the obtained amounts using EquationEquation (4)
(4)
(4) . shows the normalized initial direct-relation matrix.
Table 5. Normalized initial direct-relation matrix.
Then the total relation matrix was calculated using EquationEquation (5)
(5)
(5) . The defuzzified total relation matrix is presented in . Defuzzification was carried out using EquationEquation (7)
(7)
(7)
Table 6. Defuzzified total relation matrix.
and
were obtained by calculating the sum of the rows and columns of the defuzzified total relation matrix using EquationEquations (8)
(8)
(8) and Equation(9)
(9)
(9) . Finally, the weights of criteria were determined using EquationEquations (10)
(10)
(10) and Equation(11)
(11)
(11) . summarizes these calculations.
Table 7. Calculating the weights of each criteria.
Based on the results presented in , among the HR outcomes, turnover (C6) and job satisfaction (C5) were deemed the most important criteria from the viewpoint of the committee members. In the next step, committee members were asked to finalize the list of practices that could be implemented in their organization after consulting the HR practices extracted from the literature, taking into account the HRM strategies, and taking in the opinions of the committee members while prioritizing the high-performance HR practices. The selected practices included training (A1), selective hiring (A2), performance-based pay (A3), employee career development (A4), performance management (A5), teamwork (A6), job design (A7), and employee participation programs (A8).
The members of the committee were then asked to express the extent to which each of the criteria influences the realization of the HR outcomes identified in the previous stage by using linguistic variables and their expertise in HRM. The definitions of the linguistic variables and the corresponding fuzzy numbers are presented in (Saremi et al., Citation2009).
Table 8. Linguistic variables for the ratings.
The preferences of each working group are presented in .
Table 9. Evaluation of alternatives by each working group.
Since linguistic variables were used for evaluation, these values must be converted into crisp variables according to the methodology. For this purpose, the fuzzy evaluations from the working groups were aggregated using EquationEquation (1)(1)
(1) and were defuzzified using EquationEquation (7)
(7)
(7) . The results are presented in .
Table 10. Defuzzified aggregate working group’s criteria evaluation.
Now, the normalized matrix N is calculated using EquationEquations (14)(14)
(14) and Equation(15)
(15)
(15) . The results are presented in .
Table 11. Normalized matrix N.
Taking into account the weights in , the weighted matrix V is calculated using EquationEquation (16)(16)
(16) (shown in ).
Table 12. Weighted normalized decision matrix V.
Then, the border approximation area matrix G was obtained using EquationEquations (18)(18)
(18) and Equation(19)
(19)
(19) which is presented in .
Table 13. Border approximation area matrix (G).
In the next step, the distance of each HPWS to the border approximation area Q was calculated using EquationEquation (20)(20)
(20) ().
Table 14. The distance of the alternatives to the border approximation area (Q).
In the final step, the final scores and ranking of HPWS were calculated based on the MABAC method ().
Table 15. Prioritization of HPWS based on the MABAC method.
Using the MABAC method, performance-based pay (A3) and employee participation programs (A8) were ranked first and second, respectively, among the high-performance HR practices based on their significant HR outcomes.
6. Discussion and comparison
In order to analyze the results of the method used, we first compared the findings from the current study with previous research in the field. Previous research did not use MADM methods to prioritize HPWSs. Therefore, in order to evaluate the stability of our method, the results were compared with those of other MADM methods.
As presented in , employee turnover and employee job satisfaction were the most important HR outcomes from the committee’s point of view. These results confirm findings from previous studies that emphasized the attitudes of employees as the central variable in the relationship between HPWS and employee performance (Bonias et al., Citation2010; Cooke et al., Citation2019; Jensen et al., Citation2013; Kloutsiniotis & Mihail, Citation2017). From Yang’s (Citation2010) perspective, in particular in service-oriented industries, employee attitudes and behavior in the workplace can have a positive impact on meeting customer expectations (Yang, Citation2010).
The results of a recent study conducted by Zungbey et al. (Citation2019) emphasized the negative relationship between the HPWS and employee turnover. This means that the effective implementation of HRM practices in the industry can reduce employee turnover at the individual level (Guthrie et al., Citation2009; Selden & Sowa, Citation2015; Zungbey et al., Citation2019). The results of various studies show that this impact can be the direct or indirect result of variables such as job satisfaction, organizational commitment, job engagement and psychological attachment, unions, organizational citizenship behavior, motivation, and trust (Chen et al., Citation2018; Heffernan & Dundon, Citation2016; Kloutsiniotis & Mihail, Citation2017; Kundu & Gahlawat, Citation2016; Zungbey et al., Citation2019).
In line with our study’s findings, the impact of HPWS on employee job satisfaction (Heffernan & Dundon, Citation2016; Kloutsiniotis & Mihail, Citation2017; Mahmood et al., Citation2019) as well as the impact of job satisfaction on organizational performance (Budie et al., Citation2019; Delaney & Huselid, Citation1996; Schyns & Croon, Citation2006) has been confirmed in several studies.
These studies indicate the necessity of paying attention to the interrelations among the criteria, which has been the main focus of this research.
On the other hand, the results from using the MABAC method () show that performance-based pay and employee participation programs are among the most important high-performance HR practices and should, therefore, be taken into account in organizational planning. A review of the research in this field also shows that among high-performance HR practices, a significant proportion of previous studies focused on the role of performance-based pay (Gerhart et al., Citation2009; Gooderham et al., Citation2018; Nyberg et al., Citation2016). Performance-based pay affects current and future employee performance by influencing employee attitudes (Fulmer et al., Citation2003), enhancing employees’ psychological sense of empowerment in the organization (Messersmith et al., Citation2011; Spreitzer, Citation1995), and gaining emotional achievements (Nyberg et al., Citation2016; Perry et al., Citation2017), which ultimately can enhance organizational performance (Gardner et al., Citation2011). The findings of Sturman et al. (Citation2012) also confirm that performance-based pay is one of the organizational tools that is best for retaining high-performance employees.
According to the literature, having an employee participation program is categorized as an opportunistic practice of HPWS (Lepak et al., Citation2006). In most organizations, the top management usually makes the final decisions on key issues. However, employee perceptions about how managers pay attention to their ideas are one of the most important factors to shape employee perceptions of the effectiveness of collaborative systems. If employees perceive that managers do pay attention to their ideas, this can lead to more positive attitudes such as job satisfaction and organizational commitment (Appelbaum et al., Citation2000). McMahon and Lawler (Citation1995) showed that organizations using employee engagement systems achieve a higher level of performance than other organizations. As Combs et al. (Citation2006) indicate, HPWS can positively influence the performance of an organization while enhancing employee skills, knowledge, and abilities by improving the organization’s internal social structures to better facilitate employee communication and cooperation (Combs et al., Citation2006). In other words, the key feature of HPWS is the reorganization of work processes so that employees have the opportunity to coordinate their efforts in the organization (Choi, Citation2008). Organizations that introduce their own HPWS have relatively flat structures that emphasize the decentralization of employee information processing to allow employees to use information in decision-making and problem-solving processes. In addition, the collaborative organizational climate created by HPWS promotes a sense of psychological empowerment among employees (Messersmith et al., Citation2011; Spreitzer, Citation1995).
In order to analyze the stability of the method used, the results of the MABAC method were compared with the results of simple additive weighting (SAW) (Hwang & Yoon, Citation1981), technique for order of preference by similarity to ideal solution (TOPSIS) (Hwang & Yoon, Citation1981), additive ratio assessment (ARAS) (Zavadskas & Turskis, Citation2010) and weighted aggregated sum product assessment (WASPAS) (Zavadskas et al., Citation2012). These methods are commonly used MCDM methods for ranking alternatives.
Based on the criteria weights obtained using the DEMATEL method, the ranking results for the solutions are derived through these four methods. The final rankings of the solutions are presented in .
Table 16. The ranking results derived from the selected MCDM methods.
As seen in the table, the results for the decision-making methods used in this case rarely changed. Therefore, the results of the applied model have an acceptable level of stability.
7. Conclusion
HPWS is a set of practices that seeks to transform employees so that they can be a source of sustainable competitive advantage for the organization by enhancing their skills levels, competency, and productivity (Datta et al., Citation2005). Specifically, researchers look at those HPWSs that are more likely to influence employee attitudes and, consequently, individual and organizational performance to select and implement more appropriate improvement strategies (Zopiatis et al., Citation2014). Despite the importance of this issue, there are still major challenges in the theoretical field. Although researchers in the field of HRM agree that a systematic approach to HR practices is more appropriate than a one-dimensional approach, there is no consensus concerning the configuration and importance of the practices that constitute HPWS (Boxall & Macky, Citation2007; Cooke et al., Citation2019; Van De Voorde & Beijer, Citation2015). That is why choosing the right HPWS to aligns an organization with its goals and strategies is crucial for achieving the desired HR outcomes and ultimately enhance organizational performance. On the other hand, despite acknowledging the importance of the role of HPWS, the way HPWS affect HR outcomes and consequently organizational performance remains unclear (Chuang & Liao, Citation2010).
An important point in determining the priorities for human resource managers’ decision-making processes in an organization so that they can achieve the best HR outcomes through HPWS is that the factors that can influence these decisions are multi-dimensional and complex. This is the reason why the common statistical models are not useful for examining the relationships between HPWS and performance (Zhang et al., Citation2014). Consequently, there is no specific model or process for prioritizing high-performance HR practices at the organizational level. Given the capability of MADM methods to deal with various criteria that may be contradictory, this study aimed to propose a MADM-based framework that provides the means for prioritizing HR practices by taking into account their impact on various HR outcomes. The important point is that there is a need to pay attention to the interactions among the various HR outcomes that few of the MADM methods have addressed.
Based on this framework, high-performance HR practices and HR outcomes were identified based on the review of theoretical literature and the views of decision-makers and HR experts. Then, due to the interactions among HR outcomes, a DEMATEL-MABAC method was used to prioritize identified actions. Based on this method, the weights of the criteria were calculated using DEMATEL method, and then the alternatives were ranked using the MABAC method. Finally, the designed framework was implemented in an organization active in the banking industry. According to the results, employee turnover and employee job satisfaction were the most important HR outcomes. In addition, performance-based pay and employee participation programs were ranked first and second, respectively, among the HR practices. Managers and decision-makers of organizations can use these results to improve employee performance and, consequently, the performance of the organization. Accordingly, while taking into account the resource constraints in organizations, the priorities presented can be helpful in budgeting HRM improvement projects and the necessary resource allocation for them.
This research is the first attempt to use MADM methods for selecting HPWSs and applying this framework in the banking industry. Future research can utilize this framework for other industries. On the other hand, the proposed framework ultimately provides only a ranking of the HPWSs, though future research in this field may focus on selecting HRM improvement projects or an HPWS combination while considering resource constraints. In addition, given the complexity of the issue and the need for strong theoretical and empirical knowledge to make the expected comparisons and evaluations, utilizing decision-making methods that can better reflect the ambiguity of experts (e.g., intuitive fuzzy numbers with interval values) or incorporating the experts’ uncertainty into the designed framework (e.g., hesitant fuzzy numbers) may be useful.
Disclosure statement
No potential conflict of interest was reported by the authors.
References
- Abdullah, L., & Zulkifli, N. (2015). Integration of fuzzy AHP and interval type-2 fuzzy DEMATEL: An application to human resource management. Expert Systems with Applications, 42(9), 4397–4409. https://doi.org/https://doi.org/10.1016/j.eswa.2015.01.021
- Amiri, M., Hashemi-Tabatabaei, M., Ghahremanloo, M., Keshavarz-Ghorabaee, M., Zavadskas, E. K., & Banaitis, A. (2020). A new fuzzy BWM approach for evaluating and selecting a sustainable supplier in supply chain management. International Journal of Sustainable Development & World Ecology, 1–18. https://doi.org/https://doi.org/10.1080/13504509.2020.1793424
- Appelbaum, E., Bailey, T., Berg, P., & Kalleberg, A. L. (2000). Manufacturing advantage: Why high performance work systems pay off. Cornell University Press.
- Aryee, S., Walumbwa, F. O., Seidu, E. Y., & Otaye, L. E. (2012). Impact of high-performance work systems on individual-and branch-level performance: Test of a multilevel model of intermediate linkages. The Journal of Applied Psychology, 97(2), 287–300. https://doi.org/https://doi.org/10.1037/a0028116
- Bailey, T., Berg, P., & Sandy, C. (2001). The effect of high-performance work practices on employee earnings in the steel, apparel, and medical electronics and imaging industries. ILR Review, 54(2A), 525–543. https://doi.org/https://doi.org/10.1177/001979390105400228
- Bailly, F., & Léné, A. (2013). The personification of the service labour process and the rise of soft skills: A French case study. Employee Relations, 35(1), 79–97. https://doi.org/https://doi.org/10.1108/01425451311279429
- Bal, P. M., Kooij, D. T., & De Jong, S. B. (2013). How do developmental and accommodative HRM enhance employee engagement and commitment? The role of psychological contract and SOC strategies. Journal of Management Studies, 50(4), 545–572. https://doi.org/https://doi.org/10.1111/joms.12028
- Balezentis, A., Balezentis, T., & Brauers, W. K. M. (2012). Personnel selection based on computing with words and fuzzy MULTIMOORA. Expert Systems with Applications, 39(9), 7961–7967. https://doi.org/https://doi.org/10.1016/j.eswa.2012.01.100
- Barak, S., & Dahooei, J. H. (2018). A novel hybrid fuzzy DEA-Fuzzy MADM method for airlines safety evaluation. Journal of Air Transport Management, 73, 134–149. https://doi.org/https://doi.org/10.1016/j.jairtraman.2018.09.001
- Baykasoğlu, A., Kaplanoğlu, V., Durmuşoğlu, Z. D., & Şahin, C. (2013). Integrating fuzzy DEMATEL and fuzzy hierarchical TOPSIS methods for truck selection. Expert Systems with Applications, 40(3), 899–907. https://doi.org/https://doi.org/10.1016/j.eswa.2012.05.046
- Beskese, A., Kahraman, C., Ender Buyukbay, S., & Bozbura, F. T. (2018). An intuitionistic fuzzy multi-expert and multi-criteria system for effective performance management. Technological and Economic Development of Economy, 24(6), 2179–2201. https://doi.org/https://doi.org/10.3846/tede.2018.6462
- Bin Saeed, B., Afsar, B., Shahjehan, A., & Imad Shah, S. (2019). Does transformational leadership foster innovative work behavior? The roles of psychological empowerment, intrinsic motivation, and creative process engagement. Economic Research-Ekonomska Istraživanja, 32(1), 254–281. https://doi.org/https://doi.org/10.1080/1331677X.2018.1556108
- Bonias, D., Bartram, T., Leggat, S. G., & Stanton, P. (2010). Does psychological empowerment mediate the relationship between high performance work systems and patient care quality in hospitals? Asia Pacific Journal of Human Resources, 48(3), 319–337. https://doi.org/https://doi.org/10.1177/1038411110381667
- Boxall, P. (2012). High‐performance work systems: What, why, how and for whom? Asia Pacific Journal of Human Resources, 50(2), 169–186. https://doi.org/https://doi.org/10.1111/j.1744-7941.2011.00012.x
- Boxall, P., Ang, S. H., & Bartram, T. (2011). Analysing the ‘black box’of HRM: Uncovering HR goals, mediators, and outcomes in a standardized service environment. Journal of Management Studies, 48(7), 1504–1532. https://doi.org/https://doi.org/10.1111/j.1467-6486.2010.00973.x
- Boxall, P., & Macky, K. (2007). High‐performance work systems and organisational performance: Bridging theory and practice. Asia Pacific Journal of Human Resources, 45(3), 261–270. https://doi.org/https://doi.org/10.1177/1038411107082273.
- Budie, B., Appel-Meulenbroek, R., Kemperman, A., & Weijs-Perree, M. (2019). Employee satisfaction with the physical work environment: The importance of a need based approach. International Journal of Strategic Property Management, 23(1), 36–49. https://doi.org/https://doi.org/10.3846/ijspm.2019.6372
- Cafferkey, K., & Dundon, T. (2015). Explaining the black box: HPWS and organisational climate. Personnel Review, 44(5), 666–688. https://doi.org/https://doi.org/10.1108/PR-12-2012-0209
- Chang, S., Jia, L., Takeuchi, R., & Cai, Y. (2014). Do high-commitment work systems affect creativity? A multilevel combinational approach to employee creativity. The Journal of Applied Psychology, 99(4), 665–680. https://doi.org/https://doi.org/10.1037/a0035679
- Cheah, J. H., Ng, S. I., Ting, H., Memon, M. A., & Loo, S. C. S. (2019). Customer orientation and office space performance: Assessing the moderating effect of building grade using PLS-MGA. International Journal of Strategic Property Management, 23(2), 117–129. https://doi.org/https://doi.org/10.3846/ijspm.2019.7437
- Chen, Y., Kim, Y. K., Liu, Z., Wang, G., & Zhao, G. (2018). Can HPWS and unions work together to reduce employee turnover intention in foreign MNCs in China? In D. Lewin, & P. J. Gollan (Eds.), Advances in Industrial and Labor Relations, 2017: Shifts in workplace voice, justice, negotiation and conflict resolution in contemporary workplaces (pp. 213–242). Emerald Publishing Limited.
- Choi, J. H. (2008). Opening the black box: The mediating effect of employee attitudes in the relationship between high performance work systems and firm performance [Doctoral dissertation]. ProQuest Information & Learning.
- Chou, Y. C., Sun, C. C., & Yen, H. Y. (2012). Evaluating the criteria for human resource for science and technology (HRST) based on an integrated fuzzy AHP and fuzzy DEMATEL approach. Applied Soft Computing, 12(1), 64–71. https://doi.org/https://doi.org/10.1016/j.asoc.2011.08.058
- Chuang, C. H., & Liao, H. U. I. (2010). Strategic human resource management in service context: Taking care of business by taking care of employees and customers. Personnel Psychology, 63(1), 153–196. https://doi.org/https://doi.org/10.1111/j.1744-6570.2009.01165.x
- Combs, J., Liu, Y., Hall, A., & Ketchen, D. (2006). How much do high-performance work practices matter? A meta-analysis of their effects on organizational performance. Personnel Psychology, 59(3), 501–528. https://doi.org/https://doi.org/10.1111/j.1744-6570.2006.00045.x
- Cooke, F. L., Cooper, B., Bartram, T., Wang, J., & Mei, H. (2019). Mapping the relationships between high-performance work systems, employee resilience and engagement: A study of the banking industry in China. The International Journal of Human Resource Management, 30(8), 1239–1260. https://doi.org/https://doi.org/10.1080/09585192.2015.1137618
- Dalalah, D., Hayajneh, M., & Batieha, F. (2011). A fuzzy multi-criteria decision making model for supplier selection. Expert Systems with Applications, 38(7), 8384–8391. https://doi.org/https://doi.org/10.1016/j.eswa.2011.01.031
- Datta, D. K., Guthrie, J. P., & Wright, P. M. (2005). HRM and labor productivity: Does industry matter? Academy of Management Journal, 48(1), 135–145. https://doi.org/https://doi.org/10.5465/amj.2005.15993158
- Delaney, J. T., & Huselid, M. A. (1996). The impact of human resource management practices on perceptions of organizational performance. Academy of Management Journal, 39(4), 949–969. https://doi.org/https://doi.org/10.2307/256718
- Dursun, M., & Karsak, E. E. (2010). A fuzzy MCDM approach for personnel selection. Expert Systems with Applications, 37(6), 4324–4330. https://doi.org/https://doi.org/10.1016/j.eswa.2009.11.067
- Ensslin, S. R., Ensslin, L., Back, F., & de Oliveira Lacerda, R. T. (2013). Improved decision aiding in human resource management: A case using constructivist multi-criteria decision aiding. International Journal of Productivity and Performance Management, 62 (7), 735–757. https://doi.org/https://doi.org/10.1108/IJPPM-04-2012-0039
- Fan, D., Cui, L., Zhang, M. M., Zhu, C. J., Härtel, C. E., & Nyland, C. (2014). Influence of high performance work systems on employee subjective well-being and job burnout: Empirical evidence from the Chinese healthcare sector. The International Journal of Human Resource Management, 25(7), 931–950. https://doi.org/https://doi.org/10.1080/09585192.2014.876740
- Figueiredo, E., Pais, L., Monteiro, S., & Mónico, L. (2016). Human resource management impact on knowledge management: Evidence from the Portuguese banking sector. Journal of Service Theory and Practice, 26(4), 497–528. https://doi.org/https://doi.org/10.1108/JSTP-12-2014-0269
- Fu, N., Bosak, J., Flood, P. C., & Ma, Q. (2019). Chinese and Irish professional service firms compared: Linking HPWS, organizational coordination, and firm performance. Journal of Business Research, 95, 266–276. https://doi.org/https://doi.org/10.1016/j.jbusres.2018.08.021
- Fu, N., Flood, P. C., Bosak, J., Morris, T., & O'Regan, P. (2015). How do high performance work systems influence organizational innovation in professional service firms? Employee Relations, 37(2), 209–231. https://doi.org/https://doi.org/10.1108/ER-10-2013-0155
- Fulmer, I. S., Gerhart, B., & Scott, K. S. (2003). Are the 100 best better? An empirical investigation of the relationship between being a “great place to work” and firm performance. Personnel Psychology, 56(4), 965–993. https://doi.org/https://doi.org/10.1111/j.1744-6570.2003.tb00246.x
- Gabus, A., & Fontela, E. (1972). World problems, an invitation to further thought within the framework of DEMATEL. Battelle Geneva Research Centre.
- Gabus, A., & Fontela, E. (1973). Perceptions of the world problematique: Communication procedure, communicating with those bearing collective responsibility. Battelle Geneva Research Centre.
- Gardner, T. M., Wright, P. M., & Moynihan, L. M. (2011). The impact of motivation, empowerment, and skill‐enhancing practices on aggregate voluntary turnover: The mediating effect of collective affective commitment. Personnel Psychology, 64(2), 315–350. https://doi.org/https://doi.org/10.1111/j.1744-6570.2011.01212.x
- Gerhart, B., Rynes, S. L., & Fulmer, I. S. (2009). 6 pay and performance: Individuals, groups, and executives. The Academy of Management Annals, 3(1), 251–315. https://doi.org/https://doi.org/10.1080/19416520903047269
- Gibbs, T., & Ashill, N. J. (2013). The effects of high performance work practices on job outcomes: Evidence from frontline employees in Russia. International Journal of Bank Marketing, 31(4), 305–326. https://doi.org/https://doi.org/10.1108/IJBM-10-2012-0096
- Gölcük, İ., & Baykasoğlu, A. (2016). An analysis of DEMATEL approaches for criteria interaction handling within ANP. Expert Systems with Applications, 46, 346–366. https://doi.org/https://doi.org/10.1016/j.eswa.2015.10.041
- Gooderham, P., Fenton-O’Creevy, M., Croucher, R., & Brookes, M. (2018). A multilevel analysis of the use of individual pay-for-performance systems. Journal of Management, 44(4), 1479–1504. https://doi.org/https://doi.org/10.1177/0149206315610634
- Guthrie, J. P. (2001). High involvement work practices, turnover and productivity: Evidence from New Zealand. Academy of Management Journal, 44(1), 180–190. https://doi.org/https://doi.org/10.2307/3069345
- Guthrie, J. P., Flood, P. C., Liu, W., & MacCurtain, S. (2009). High performance work systems in Ireland: Human resource and organizational outcomes. The International Journal of Human Resource Management, 20(1), 112–125. https://doi.org/https://doi.org/10.1080/09585190802528433
- Haar, J. M., & White, B. J. (2013). Corporate entrepreneurship and information technology towards employee retention: A study of New Zealand firms. Human Resource Management Journal, 23(1), 109–125. https://doi.org/https://doi.org/10.1111/j.1748-8583.2011.00178.x
- Heffernan, M., & Dundon, T. (2016). Cross‐level effects of high‐performance work systems (HPWS) and employee well‐being: The mediating effect of organisational justice. Human Resource Management Journal, 26(2), 211–231. https://doi.org/https://doi.org/10.1111/1748-8583.12095
- Heidary Dahooie, J., Beheshti Jazan Abadi, E., Vanaki, A. S., & Firoozfar, H. R. (2018). Competency‐based IT personnel selection using a hybrid SWARA and ARAS‐G methodology. Human Factors and Ergonomics in Manufacturing & Service Industries, 28(1), 5–16. https://doi.org/https://doi.org/10.1002/hfm.20713
- Horváthová, P., Čopíková, A., & Mokrá, K. (2019). Methodology proposal of the creation of competency models and competency model for the position of a sales manager in an industrial organisation using the AHP method and Saaty’s method of determining weights. Economic Research-Ekonomska Istraživanja, 32(1), 2594–2613. https://doi.org/https://doi.org/10.1080/1331677X.2019.1653780
- Huselid, M. A. (1995). The impact of human resource management practices on turnover, productivity, and corporate financial performance. Academy of Management Journal, 38(3), 635–672. https://doi.org/https://doi.org/10.2307/256741
- Hwang, C. L., & Yoon, K. (1981). Methods for multiple attribute decision making. In C.-L. Hwang, & K. Yoon (Eds.), Multiple attribute decision making (pp. 58–191). Springer.
- Jensen, J. M., Patel, P. C., & Messersmith, J. G. (2013). High-performance work systems and job control: Consequences for anxiety role overload, and turnover intentions. Journal of Management, 39(6), 1699–1724. https://doi.org/https://doi.org/10.1177/0149206311419663
- Jha, S., Balaji, M. S., Yavas, U., & Babakus, E. (2017). Effects of frontline employee role overload on customer responses and sales performance: Moderator and mediators. European Journal of Marketing, 51(2), 282–303. https://doi.org/https://doi.org/10.1108/EJM-01-2015-0009
- Jiang, K., Lepak, D. P., Hu, J., & Baer, J. C. (2012). How does human resource management influence organizational outcomes? A meta-analytic investigation of mediating mechanisms. Academy of Management Journal, 55(6), 1264–1294. https://doi.org/https://doi.org/10.5465/amj.2011.0088
- Kaklauskas, A., Banaitis, A., Ferreira, F. A. F., Ferreira, J. J. M., Amaratunga, D., Lepkova, N., Ubartė, I., & Banaitienė, N. (2018). An evaluation system for university–industry partnership sustainability: Enhancing options for entrepreneurial universities. Sustainability, 10(2), 119. https://doi.org/https://doi.org/10.3390/su10010119
- Katou, A. (2011). Test of a causal human resource management-performance linkage model: Evidence from the Greek manufacturing sector. International Journal of Business Science & Applied Management (IJBSAM), 6(1), 16–29.
- Kehoe, R. R., & Wright, P. M. (2013). The impact of high-performance human resource practices on employees’ attitudes and behaviors. Journal of Management, 39(2), 366–391. https://doi.org/https://doi.org/10.1177/0149206310365901
- Kelemenis, A., & Askounis, D. (2010). A new TOPSIS-based multi-criteria approach to personnel selection. Expert Systems with Applications, 37(7), 4999–5008. https://doi.org/https://doi.org/10.1016/j.eswa.2009.12.013
- Kloutsiniotis, P. V., & Mihail, D. M. (2017). Linking innovative human resource practices, employee attitudes and intention to leave in healthcare services. Employee Relations, 39(1), 34–53. https://doi.org/https://doi.org/10.1108/ER-11-2015-0205
- Kloutsiniotis, P. V., & Mihail, D. M. (2018). The link between perceived high-performance work practices, employee attitudes and service quality: The mediating and moderating role of trust. Employee Relations, 40(5), 801–821. https://doi.org/https://doi.org/10.1108/ER-08-2017-0201
- Kroon, B., Van de Voorde, K., & Van Veldhoven, M. J. P. M. (2009). Cross-level effects of high-performance work practices on burnout: Two counteracting mediating mechanisms compared. Personnel Review, 38(5), 509–525. https://doi.org/https://doi.org/10.1108/00483480910978027
- Kundu, S. C., & Gahlawat, N. (2016). High performance work systems and employees’ intention to leave: Exploring the mediating role of employee outcomes. Management Research Review, 39(12), 1587–1615. https://doi.org/https://doi.org/10.1108/MRR-04-2015-0088
- Lepak, D. P., Liao, H., Chung, Y., & Harden, E. E. (2006). A conceptual review of human resource management systems in strategic human resource management research. In J. J. Martocchio (Ed.), Research in personnel and human resources management (pp. 217–271). Emerald Group Publishing Limited.
- Li, Q. (2013). A novel Likert scale based on fuzzy sets theory. Expert Systems with Applications, 40(5), 1609–1618. https://doi.org/https://doi.org/10.1016/j.eswa.2012.09.015
- Liao, H., Toya, K., Lepak, D. P., & Hong, Y. (2009). Do they see eye to eye? Management and employee perspectives of high-performance work systems and influence processes on service quality. The Journal of Applied Psychology, 94(2), 371–391. https://doi.org/https://doi.org/10.1037/a0013504
- MacDuffie, J. (1995). Human resource bundles and manufacturing performance: Organizational logic and flexible production systems in the world auto industry. ILR Review, 48(2), 197–221. https://doi.org/https://doi.org/10.1177/001979399504800201
- Mahmood, A., Akhtar, M. N., Talat, U., Shuai, C., & Hyatt, J. C. (2019). Specific HR practices and employee commitment: The mediating role of job satisfaction. Employee Relations, 41(3), 420–435. https://doi.org/https://doi.org/10.1108/ER-03-2018-0074
- Mammadova, M. H., & Jabrayilova, Z. G. (2018). Fuzzy multi-criteria method to support group decision making in human resource management. In L. Zadeh, R. Yager, S. Shahbazova, M. Reformat, & V. Kreinovich (Eds.), Recent developments and the new direction in soft-computing foundations and applications (pp. 209–222). Springer.
- McClean, E., & Collins, C. J. (2011). High‐commitment HR practices, employee effort, and firm performance: Investigating the effects of HR practices across employee groups within professional services firms. Human Resource Management, 50(3), 341–363. https://doi.org/https://doi.org/10.1002/hrm.20429
- McMahon, G. C., & Lawler, E. E. III, (1995). Effects of union status on employee involvement: Diffusion and effectiveness. Research in Organisational Change and Development, 8, 47–76.
- Messersmith, J. G., Patel, P. C., Lepak, D. P., & Gould-Williams, J. S. (2011). Unlocking the black box: Exploring the link between high-performance work systems and performance. The Journal of Applied Psychology, 96(6), 1105–1118. https://doi.org/https://doi.org/10.1037/a0024710
- Mohagheghi, V., Mousavi, S. M., Antuchevičienė, J., & Dorfeshan, Y. (2019). Sustainable infrastructure project selection by a new group decision-making framework introducing MORAS method in an interval type 2 fuzzy environment. International Journal of Strategic Property Management, 23(6), 390–404. https://doi.org/https://doi.org/10.3846/ijspm.2019.10536
- Ning, N., Wang, J., Lin, Z., & Zheng, Z. (2018). The direct and moderating effect of learning orientation on individual performance in the banking industry in China: Contextualization of high‐performance work systems. Asia Pacific Journal of Human Resources, 56(3), 360–383. https://doi.org/https://doi.org/10.1111/1744-7941.12141
- Nyberg, A. J., Pieper, J. R., & Trevor, C. O. (2016). Pay-for-performance’s effect on future employee performance: Integrating psychological and economic principles toward a contingency perspective. Journal of Management, 42(7), 1753–1783. https://doi.org/https://doi.org/10.1177/0149206313515520
- Ogbonnaya, C., & Valizade, D. (2018). High performance work practices, employee outcomes and organizational performance: A 2-1-2 multilevel mediation analysis. The International Journal of Human Resource Management, 29(2), 239–259. https://doi.org/https://doi.org/10.1080/09585192.2016.1146320
- Paes de Faria, A. C. C., Ferreira, F. A. F., Dias, P. J. V. L., & Çipi, A. (2020). A constructivist model of bank branch front-office employee evaluation: An FCM-SD-based approach. Technological and Economic Development of Economy, 26(1), 213–239.
- Pamučar, D., & Ćirović, G. (2015). The selection of transport and handling resources in logistics centers using multi-attributive border approximation area comparison (MABAC). Expert Systems with Applications, 42(6), 3016–3028. https://doi.org/https://doi.org/10.1016/j.eswa.2014.11.057
- Perry, J. L., Engbers, T. A., & Jun, S. Y. (2017). Back to the future? Performance-related pay, empirical research, and the perils of persistence. In R. F. Durant, & J. R. S. Durant (Eds.), Debating Public Administration (pp. 27–65). Routledge.
- Polychroniou, P. V., & Giannikos, I. (2009). A fuzzy multicriteria decision-making methodology for selection of human resources in a Greek private bank. Career Development International, 14(4), 372–387. https://doi.org/https://doi.org/10.1108/13620430910979853
- Rahmanniyay, F., & Yu, A. J. (2019). A multi-objective stochastic programming model for project-oriented human-resource management optimization. International Journal of Management Science and Engineering Management, 14(4), 231–239. https://doi.org/https://doi.org/10.1080/17509653.2018.1534220
- Ranjan, K. R., Sugathan, P., & Rossmann, A. (2015). A narrative review and meta-analysis of service interaction quality: New research directions and implications. Journal of Services Marketing, 29(1), 3–14. https://doi.org/https://doi.org/10.1108/JSM-01-2014-0029
- Safavi, H. P., & Karatepe, O. M. (2018). High-performance work practices and hotel employee outcomes: The mediating role of career adaptability. International Journal of Contemporary Hospitality Management, 30(2), 1112–1133. https://doi.org/https://doi.org/10.1108/IJCHM-07-2016-0367
- Sang, X., Liu, X., & Qin, J. (2015). An analytical solution to fuzzy TOPSIS and its application in personnel selection for knowledge-intensive enterprise. Applied Soft Computing, 30, 190–204. https://doi.org/https://doi.org/10.1016/j.asoc.2015.01.002
- Saremi, M., Mousavi, S. F., & Sanayei, A. (2009). TQM consultant selection in SMEs with TOPSIS under fuzzy environment. Expert Systems with Applications, 36(2), 2742–2749. https://doi.org/https://doi.org/10.1016/j.eswa.2008.01.034
- Schyns, B., & Croon, M. A. (2006). A model of task demands, social structure, and leader–member exchange and their relationship to job satisfaction. The International Journal of Human Resource Management, 17(4), 602–615. https://doi.org/https://doi.org/10.1080/09585190600581378
- Searle, R., & Skinner, D. (Eds.). (2011). Trust and human resource management. Edward Elgar Publishing.
- Selden, S. C., & Sowa, J. E. (2015). Voluntary turnover in nonprofit human service organizations: The impact of high performance work practices. Human Service Organizations: Management, Leadership & Governance, 39(3), 182–207.
- Shaw, J. D., Gupta, N., & Delery, J. E. (2005). Alternative conceptualisations of the relationship between voluntary turnover and organizational performance. Academy of Management Journal, 48(1), 50–68. https://doi.org/https://doi.org/10.5465/amj.2005.15993112
- Spreitzer, G. M. (1995). Psychological empowerment in the workplace: Dimensions, measurement, and validation. Academy of Management Journal, 38(5), 1442–1465.
- Stanujkic, D., Zavadskas, E. K., Brauers, W., & Karabasevic, D. (2015). An extension of the MULTIMOORA method for solving complex decision-making problems based on the use of interval-valued triangular fuzzy numbers. Transformations in Business & Economics, 14(2B), 355–375.
- Sturman, M. C., Shao, L., & Katz, J. H. (2012). The effect of culture on the curvilinear relationship between performance and turnover. The Journal of Applied Psychology, 97(1), 46–62. https://doi.org/https://doi.org/10.1037/a0024868
- Sun, L. Y., Aryee, S., & Law, K. S. (2007). High-performance human resource practices, citizenship behavior, and organizational performance: A relational perspective. Academy of Management Journal, 50(3), 558–577. https://doi.org/https://doi.org/10.5465/amj.2007.25525821
- Takeuchi, R., Lepak, D. P., Wang, H., & Takeuchi, K. (2007). An empirical examination of the mechanisms mediating between high-performance work systems and the performance of Japanese organizations. The Journal of Applied Psychology, 92(4), 1069–1083. https://doi.org/https://doi.org/10.1037/0021-9010.92.4.1069
- Tuana, N. A. (2018). Developing a generalized fuzzy multi-criteria decision making for personnel selection. Fuzzy Economic Review, 23(2), 27–41.
- Úbeda-García, M., Claver-Cortés, E., Marco-Lajara, B., Zaragoza-Sáez, P., & García-Lillo, F. (2018). High performance work system and performance: Opening the black box through the organizational ambidexterity and human resource flexibility. Journal of Business Research, 88, 397–406. https://doi.org/https://doi.org/10.1016/j.jbusres.2017.12.045
- Van De Voorde, K., & Beijer, S. (2015). The role of employee HR attributions in the relationship between high‐performance work systems and employee outcomes. Human Resource Management Journal, 25(1), 62–78. https://doi.org/https://doi.org/10.1111/1748-8583.12062
- Wang, Z., & Xu, H. (2017). How and when service-oriented high-performance work systems foster employee service performance: A test of mediating and moderating processes. Employee Relations, 39(4), 523–540. https://doi.org/https://doi.org/10.1108/ER-07-2016-0140
- Xian, H., Atkinson, C., & Meng-Lewis, Y. (2019). Guanxi and high performance work systems in China: Evidence from a state-owned enterprise. The International Journal of Human Resource Management, 30(19), 2685–2704. https://doi.org/https://doi.org/10.1080/09585192.2017.1332670
- Xu, Z., & Yager, R. R. (2008). Dynamic intuitionistic fuzzy multi-attribute decision making. International Journal of Approximate Reasoning, 48(1), 246–262. https://doi.org/https://doi.org/10.1016/j.ijar.2007.08.008
- Yang, J. T. (2010). Antecedents and consequences of job satisfaction in the hotel industry. International Journal of Hospitality Management, 29(4), 609–619. https://doi.org/https://doi.org/10.1016/j.ijhm.2009.11.002
- Yazdani, M., Wen, Z., Liao, H., Banaitis, A., & Turskis, Z. (2019). A grey combined compromise solution (CoCoSo-G) method for supplier selection in construction management. Journal of Civil Engineering and Management, 25(8), 858–874. https://doi.org/https://doi.org/10.3846/jcem.2019.11309
- Youndt, M. A., Snell, S. A., Dean, J. W., Jr., & Lepak, D. P. (1996). Human resource management, manufacturing strategy, and firm performance. Academy of Management Journal, 39(4), 836–866.
- Zacharatos, A., Barling, J., & Iverson, R. D. (2005). High-performance work systems and occupational safety. The Journal of Applied Psychology, 90(1), 77–93. https://doi.org/https://doi.org/10.1037/0021-9010.90.1.77
- Zavadskas, E. K., & Turskis, Z. (2010). A new additive ratio assessment (ARAS) method in multicriteria decision‐making. Technological and Economic Development of Economy, 16(2), 159–172. https://doi.org/https://doi.org/10.3846/tede.2010.10
- Zavadskas, E. K., Turskis, Z., Antucheviciene, J., & Zakarevicius, A. (2012). Optimization of weighted aggregated sum product assessment. Elektronika ir Elektrotechnika, 122(6), 3–6.
- Zavadskas, E. K., Turskis, Z., & Kildienė, S. (2014). State of art surveys of overviews on MCDM/MADM methods. Technological and Economic Development of Economy, 20(1), 165–179. https://doi.org/https://doi.org/10.3846/20294913.2014.892037
- Zhang, J., Bal, P. M., Akhtar, M. N., Long, L., Zhang, Y., & Ma, Z. (2019). High‐performance work system and employee performance: the mediating roles of social exchange and thriving and the moderating effect of employee proactive personality. Asia Pacific Journal of Human Resources, 57(3), 369–395. https://doi.org/https://doi.org/10.1111/1744-7941.12199
- Zhang, M., Di Fan, D., & Zhu, C. J. (2014). High-performance work systems, corporate social performance and employee outcomes: Exploring the missing links. Journal of Business Ethics, 120(3), 423–435. https://doi.org/https://doi.org/10.1007/s10551-013-1672-8
- Zhang, S., & Liu, S. (2011). A GRA-based intuitionistic fuzzy multi-criteria group decision making method for personnel selection. Expert Systems with Applications, 38(9), 11401–11405. https://doi.org/https://doi.org/10.1016/j.eswa.2011.03.012
- Zhao, S., Zhang, J., Zhao, W., & Poon, T. S. C. (2012). Changing employment relations in China: A comparative study of the auto and banking industries. The International Journal of Human Resource Management, 23(10), 2051–2064. https://doi.org/https://doi.org/10.1080/09585192.2012.668364
- Zopiatis, A., Constanti, P., & Theocharous, A. L. (2014). Job involvement, commitment, satisfaction and turnover: Evidence from hotel employees in Cyprus. Tourism Management, 41, 129–140. https://doi.org/https://doi.org/10.1016/j.tourman.2013.09.013
- Zungbey, O. D. D., Bonsu, N. O., Ntow, M. A. O., & Sokro, E. (2019). High performance work systems and employee turnover intentions: Moderating effect of psychological attachment. In J. Kantola, & S. Nazir (Eds.), International Conference on Applied Human Factors and Ergonomics (pp. 240–250). Springer.