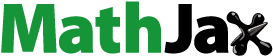
Abstract
Even though trade tariffs have generally fallen since the GATT agreement, non-tariff trade barriers still exist and show an upward trend. An important type of non-tariff trade barrier is logistics service related to the transport of goods to foreign markets. Efficient logistics is of great importance for small and open economies such as the Central and Eastern European EU member countries that became EU members in 2004 or later and are in the process of economic convergence with the old EU member countries, mostly through trade. On the other hand, logistics is important for old EU member countries because it influences competitiveness in global supply chains. The aim of this paper is to examine the homogeneity of the two blocks of EU countries in terms of logistics performance, i.e. to examine the impact of logistics performance on the international bilateral trade of the EU15 and CEMS with the rest of the world in the period 2010–2018. We develop and estimate a structural gravity model with Poisson pseudo-maximum probability estimator, using the LPI and its sub-indices as the main independent variables of interest. Our results show that differences in LPI values have heterogenous impact on bilateral trade, especially when considering trade in different classes of goods and different groups of country pairs.
1. Introduction
Logistics is strongly connected to trade and its contribution to the trade competitiveness of countries is growing, especially since the trade tariffs have largely declined due to the trade liberalization process than began after General Agreement on Tariffs and Trade came into force in 1948. Efficient transport and logistics can either boost trade or become a barrier to it, therefore we consider it as a non-tariff barrier. Nords and Piermartini (Citation2004) argue that the cost of transporting goods to foreign markets presents a good example of non-tariff barriers. However, it is not just the transport costs that matter; security, quality of infrastructure, customs procedures and the length of time that it takes for goods to be shipped matter as well. Hummels (Citation2001) states that the time that it takes for goods to be shipped and the unpredictability related to time are also costly to traders. ‘International trade calls for flows to be organised and synchronised through strategic nodes and networks that facilitate storage, conservation and any other value-added service required due to the very characteristics of the goods being transported’ (Puertas et al., Citation2014, p. 468). The World Bank has developed the logistics performance index (hereinafter: LPI) as a benchmarking tool to help countries identify the challenges and opportunities they face with respect to logistics performance. LPI is used to analyse differences between countries in terms of logistics costs and the quality of the infrastructure for overland and maritime transport (Marti et al., Citation2014b).
Numerous papers in this field have found the positive links between trade facilitation and/or logistics performance and trade, such as Wilson et al., Citation2003; Behar & Manners, Citation2008; Marti et al., Citation2014a; Marti et al., Citation2014b etc. However, their investigations only considered the links between absolute value of logistics performance index (or its sub-indices) and total/aggregate trade (or specific product groups), but there was no investigations how particular LPI sub-index affect particular product groups. This paper investigates the impact of the difference of logistics performance sub-indices (hereinafter LPI) values between trading partners on their bilateral trade covering the biennial period from 2010 to 2018. We investigate if the difference in LPI matters and does it affect bilateral trade. Our paper extends the existing literature in a way that we classify trading goods using Broad Economic Categories (BEC) classification, that we then aggregate to the three basic System of National Accounts (SNA) classes of goods: intermediate, capital and consumption goods. In that way we also investigate for potential heterogeneous impact of LPI differences with respect to different classes of goods. There is a scarce literature on the product or product groups-specific research in this research field, and there is lack of empirical findings about the effects of improvements in trade logistics on trade in specific product groups. Our assumption is that different logistics functions do not matter equally to different products, for example the perishable nature of food products or the sensitivity of chemical products makes them more vulnerable to delays in trade (Liu & Yue, Citation2013). Therefore, this paper attempts to detect possible differences in trade of different classes of goods. In order to estimate the effects of logistics performance on international trade we use augmented gravity model. As a proxy variable for logistics performance we use LPI, that is, its six sub-indices, namely ‘the efficiency of customs and border management clearance, the quality of trade and transport infrastructure, the ease of arranging competitively priced shipments, the competence and quality of logistics services, the ability to track and trace consignments, and finally, the frequency with which shipments reach consignees within scheduled or expected delivery times’ (Arvis et al., Citation2018).
Our paper also addresses the gap in the literature investigating how trade patterns vary across different groups of countries. Our focus are European Union member countries, where we distinguish between old EU member countries (hereinafter EU15) and new EU member countries or so-called Central and Eastern EU member countries (hereinafter CEMS). We compare effects of the difference in LPI on bilateral trade between these two groups of countries and Rest of the World (ROW) countries. There is a clear lack of research of the differences in logistics performance within economic integrations and its impact on trade flows. We emphasise the importance of this research for CEMS countries, well known for relatively complicated transition from planned to market economy, outdated transport infrastructure, but also good geographical position and membership in the EU. According to the Mordor Intelligence, Citation2018 Report, CEMS, such as Czech Republic, Hungary, Poland, and Slovakia, are among the fastest growing economies in the EU. However, their logistics market is still in infant phase, and undeveloped in comparison with the logistics markets of old EU member countries. CEMS need to address poor infrastructure, especially railways, political corruption, lack of competitiveness etc. Despite the current issues, the CEMS are an attractive location for the investments in logistics. Therefore, from the macroeconomic point of view, it is important to take into consideration the potentials of CEMS logistics market and its impact on international trade. On the other hand, we have EU15, EU core group of countries that we basically use as a benchmark for CEMS.
This paper contributes to the existing literature in several ways, covering the connection between logistics performance and international trade in different classes of goods. It evaluates the importance of the logistics services in international trade studies, and it analyses how differences in LPI sub-indices levels between trading countries impact bilateral trade flows, differentiating between two groups of countries within economic integration, namely EU. As such, our research follows recent work on the effects of trade costs, where logistics performance plays a significant role (Saslavsky & Shepherd, Citation2014).
The remainder of the paper is structured as follows: Section 2 reviews the literature related to trade logistics research. Section 3 presents the methodology used in the empirical part of the paper. Section 4 explains the data and variables included in the analysis. Section 5 discusses the results and policy implications, and finally, Section 6 presents concluding remarks.
2. Literature review
Trade liberalization and the tariff reductions motivated researchers to start investigating the effects of non-tariff barriers on international trade, respectively the effects of reductions of transport costs and other barriers to trade, so called ‘trade facilitation’, on international trade (Anderson & van Wincoop, Citation2004; Baier & Bergstrand, Citation2001; Brun et al., Citation2005; Clark et al., Citation2004; Hummels, Citation2001, Citation2007; Limao & Venables, Citation2001). As many authors note, improvements in trade facilitation leads to international trade growth. In their seminal paper, Wilson et al. (Citation2003) estimate the effects of trade facilitation on trade in APEC countries. To measure the effects of trade facilitation, they employed the gravity model where port infrastructure, customs environment, regulatory environment, and e-business infrastructure were used as proxy variables for trade facilitation measures. In 2005, same authors expand their research; they estimate the effects of trade facilitation on trade in manufactured products for 75 countries in the period from 2000 to 2001. The results of both studies suggest that improvements in all four trade facilitation measures lead to increase in international trade. Soloaga et al. (Citation2006) study the effects of changes in trade facilitation on trade in Mexican main industrial sectors and their results suggest that trade facilitation measures should be taken seriously when creating trade policies since the improvement in trade facilitation could increase export by approximately 20% and imports by 11%. Iwanow and Kirkpatrick (Citation2007) investigate the impact of regulatory quality and trade facilitation on export. The results of their gravity model suggest that all trade facilitation measures, including improved transport and communications infrastructure increase exports. Portugal-Perez and Wilson (Citation2010) use physical infrastructure, information and communications technology, border and transport efficiency, and business and regulatory environment as trade facilitation proxy variables in order to detect their effects on trade volume and the results also support aforementioned findings. According to Djankov et al. (Citation2010), each additional day that a product is not being dispatched, reduces trade by more than 1% and that percentage is even higher in case of perishable products, such as agricultural products, meaning that perishable products are more time sensitive. For the first time, in 2007, the World Bank published the Logistics Performance Index which includes all above mentioned trade facilitation measures i.e. customs clearance, transport infrastructure, quality of logistics service, timeliness and the ability to track the shipment. LPI received significant attention in the international trade literature and public policy discourse and researchers started to use it as a proxy variable for trade facilitation and include it in the international trade analysis. Behar and Manners (Citation2008) incorporate LPI 2007 for the first time in their gravity framework in order to investigate the effects of the logistics of source and destination country on bilateral exports and the effects of logistics of countries’ neighbours on exports. They use aggregate LPI as a proxy variable for logistics of source and destination country and their findings show that logistics positively affect exports, however bordering countries’ logistics matters only for the landlocked exporters. Moreover, the results also show that the destination country’s neighbours’ logistics is negatively related to exports to that country. They explain those findings as a matter of choice. Namely, exporting countries choose between numerous disembark places and mostly send their goods to the ‘relatively well-equipped countries before allowing regional distributors to take over’.
Hertel and Mirza (Citation2009) and Felipe and Kumar (Citation2012) contribute to the literature by including LPI index and its sub-indices as trade facilitation measures in order to estimate the effects of trade facilitation on trade in Asian countries by using gravity model approach. While Hertel and Mirza use only one LPI sub-index in each regression, Felipe and Kumar incorporate all the LPI sub-indices in one equation. Both analyses conclude that trade facilitation positively affect trade and that infrastructure is the most important LPI sub-index. According to Felipe and Kumar’ estimation, the gains in trade vary from 28% in case of Azerbaijan to 63% in case of Tajikistan. Their results also suggest that from the exporter point of view, infrastructure has the greatest impact on trade while from the importer side, customs efficiency has the greatest impact on trade.
Puertas et al. (Citation2014) and Marti et al. (Citation2014a, Citation2014b) also estimate the effects of logistics performance on international trade. All three studies base research on gravity model and use LPI as proxy variable for logistics performance and as in Behar and Manners (Citation2008) their results show significant positive effects of logistics performance of trade, implying that logistics is more important to the exporting than to the importing countries. Furthermore, authors recommend the enhancement of exporter-oriented policies and interventions. Puertas et al. (Citation2014) focus research on 26 EU countries in the period from 2005 to 2010. In the case of EU countries, the competence and quality of logistics services record the highest score, followed by the ability to track and trace consignments and the quality of customs and infrastructure. This results actually show better performance of the EU private sector in case of trade facilitation since components with the highest impact are in reliability of the private sector. Marti et al. (Citation2014a, Citation2014b) estimate the effects of logistics performance on trade flows in emerging countries grouped in five regions, Africa, Eastern Europe, Far East, South America and Middle East. They control for the trade between different groups of products in accordance to their logistics complexity and their findings show that the more difficult is to transport goods, the more important becomes logistics performance. Similarly, Saslavsky and Shepherd (Citation2014) investigate the effects of logistics performance on trade in parts and components within international production networks and their main conclusion is that trade in parts and components is more sensitive to logistics performance than trade in final goods. Bresslein and Huber (Citation2016) analyse trade patterns of EU countries distinguishing between trade in parts, components and final goods using Eurostat COMEXT database at 8-digit level. Their findings confirm that trade patterns differ for different types of products, namely parts, components, and final goods and that all EU countries are active through all supply chain, however while developed countries trade mostly with other developed countries, less developed EU countries trade with more developed countries. Latest findings of Gani (Citation2017) and Host et al. (Citation2019) estimate the effects of logistics performance on international trade using cross-country data for a large sample of countries and both agree that logistics performance have statistically significant and positive effect on trade flows, particularly on exports. In addition, Bugarčić et al. (Citation2020) analyse the impact of logistics performance on trade volume within two groups of countries, Central and Eastern European and Western Balkan countries, and conclude that sub-indices international shipments, logistic quality and competence and tracking and tracing have the highest effects on trade volume in observed year 2018.
Finally, the majority of empirical studies agree that logistics performance and trade facilitation, in general, play an important role in international trade. The findings reveal that logistics and transport is increasingly important for trade across supply chains and therefore is necessary to investigate and better understand how trade patterns vary across different groups of countries within economic integration and how logistics performance and its sub-indices affects trade in different groups of products. Participation in regional and global supply chains, especially for new EU countries is significant for their competitiveness and therefore our aim is to detect the effects of logistics performance on EU trade in specific group of products across and offer suggestions for further trade and logistics policies.
3. Methodology
In our research, the theoretical framework to investigate the effects of logistics service performance on international trade is based on the gravity model theory of international trade. Since the pioneer work of Tinbergen (Citation1962), the gravity equations have been frequently used in many trade related research papers during decades (Anderson & van Wincoop, Citation2004; Behar & Manners, Citation2008; Bergstrand, Citation1985, Citation1989; Frede & Yetkiner, Citation2017; Host et al., Citation2019; Krugman, Citation1991; Zajc Kejžar et al., Citation2016). We develop the following structural gravity model to estimate the effects of logistics performance differences between trading partners on bilateral trade:
(1)
(1)
where
is the value of bilateral trade in U.S. dollars between reporting country i and partner country j in year t (since LPI data is published biennially, t represents years 2010, 2012, 2014, 2016 and 2018). For reporting countries, we have EU28 member countries (so i goes from 1 to 28), while j includes EU28 member countries as well as ROW j goes from 1 to 157; for years 2010 and 2012 our sample has 152 partner countries). This difference of 5 countries is due to the fact that in 2014 five new countries began publishing LPI scores).
presents absolute difference of the GDP (in current US dollars) between country i and country j in year t.
represents distance between capital cities of trading partners.
is a dummy variable with the value one if trading partners share land border, zero if not and
is dummy variable with the value one if countries have common official primary language, zero if not. We note that our approach, using differences of trading partners variables as regressors is not new it this type of research. Gravity models employed to test Linder’s theory/hypothesis use absolute difference between GDP per capita of the importing and the exporting country as one of the regressors (Arnon & Weinblatt, Citation1998; Atabay, Citation2015, Jošić & Metelko, Citation2018). Furthermore, this approach is used also in other research fields, like fiscal policy, which include bilateral trade data in their analysis (see Holzner et al., Citation2018).
Terms and
represent exporters and importers fixed effects, respectively. Use of exporter and importer fixed effects has become standard since Anderson and van Wincoop (Citation2004) and Baldwin and Taglioni (Citation2006), because it solves potential biases in estimation results due to different price levels between countries and other country-fixed, country-pair fixed and time-fixed characteristics, depending of the type of data (cross-sectional or panel data).
Main variable(s) of interest are presented with and is calculated as absolute difference in one of the six LPI sub-indices between trading partners. The six LPI sub-indices are the following:
efficiency of clearance process (hereinafter Customs)
quality of trade and transport infrastructure (hereinafter Infrastructure)
ease of arranging competitively priced shipments (hereinafter International)
competence and quality of logistic services (hereinafter Logistics)
ability to track and trade consignments (hereinafter Tracking)
timeliness of shipments with the expected delivery time (hereinafter Timeliness).
The LPI is based on worldwide survey carried out across more than 5000 freight forwarders and logistics firms who operate internationally. Each respondent rates their trade logistics experience (in six above mentioned dimensions/sub-indices) with the eight countries they trade the most. Further details of the construction of each sub-index are available in Arvis et al. (Citation2018). The descriptive statistics of the (summary for biennially data from 2010 to 2018) for the full sample is given in . In and we present results for two sub-samples, for the cases when reporting countries are EU15 countries and partner countries are ROW countries, and when reporting countries are CEMS countries and partner countries are ROW countries.
We estimate Model (1) for each LPI sub-index. We couldn't estimate the model with all six LPI sub-indices together due to a high degree of correlation between sub-indices, resulting in high VIF for some LPI coefficients (higher than 10). In order to find out whether trade in different product classes is more sensitive to different logistics performance sub-indices, we estimate our gravity model separately for each class of SNA: intermediate, capital and consumption goods.
When choosing the estimator for our model, we had different possibilities, like standard ordinary least squares, fixed effects estimator or Poisson estimator. We chose and estimated Model 1 with Poisson Pseudo-Maximum-Likelihood Estimator (PPML), first introduced in gravity model setting by Silva and Tenreyro (Citation2006). We also used multilateral resistance terms introduced through fixed reporter and partner effects, thus following one of the seminal paper in this field of research, that of Anderson and van Wincoop (Citation2004). That way we obtain consistent estimates of the gravity model variables, that are robust to different patterns of heteroskedasticity. Furthermore, by using PPML we are able to include zero trade flows, thus avoiding a source of bias. In our data, there are 5.2% observations at country pair level with zero trade.
4. Data
Our data consists of bilateral trade data between EU28 member countries and their trading partners, 157 countries in total. We distinguish between two groups within EU-28 countries: new EU member countries, that is all countries that became EU members since 2004 (CEMS) and old EU member countries (EU15). Source of bilateral trade data is UN Comtrade database. We obtained GDP data from World Bank Open Data, while the data for other standard other standard gravity variables we obtained from CEPII database. Data for our main variable of interest, LPI sub-indices, came from World Bank. presents descriptive statistics for standard gravity model variables, while as explained in Methodology section, shows descriptive statistics of absolute differences of LPI sub-indices between trading partners for the full sample.
Table 1. Summary statistics.
Table 2. Summary statistics of the variable lpisub for the full sample.
shows average trade value between trading dyads for three groups of countries that we define in our paper and from where we can observe the following: 1) EU15 intraregional trade is by far more developed in comparison with CEMS intraregional trade; 2) CEMS trade more with EU15 (interregional trade) than with other countries in CEMS; 3) EU15 is far more oriented toward trade with ROW in comparison with CEMS.
Table 3. Summary statistics for average bilateral trade for three groups of countries.
If we compare results of descriptive statistics in with the results in , it is clear that EU15 have better logistics performance with respect to CEMS when comparing both groups with the ROW countries. We can use this difference as a proxy variable and argue that it reflects the difference between economic development levels between CEMS and EU15.
Table 4. Summary statistics of the variable lpisub for EU15-ROW sub-sample.
5. Results and discussion
Our results,Footnote1 presented in show that variation in LPI sub-indices help explain variation in total trade, that is, the bigger the difference in LPI sub-indices, the lower the trade between trading partners. What is even more important, we find that there is heterogeneous impact of LPI sub-indices on trade, that is noticeable both in EU15-ROW and CEMS-ROW sub-samples and across all three classes of goods. Most negative and significant impact of an increase in LPI difference between trading partner is evident in the case of trade in intermediate goods between EU15 and ROW. This finding is in line with our expectations since almost two-thirds of global trade is in intermediate goods and trade in intermediate goods is closely connected with regional and global value chains that shape regional and global trade and global economy.
Table 5. Summary statistics of the variable lpisub for CEMS-ROW sub-sample.
Table 6. Estimation results of Model 1 for intermediate goods.
Table 7. Estimation results of Model 1 for capital goods.
Table 8. Estimation results of Model 1 for consumption goods.
The results show that in the case of EU15-ROW trade, sub-indices Timelines, Tracking and International seems to have the highest negative effects on trade. Namely, the score gap in the sub-index the ease of arranging competitively priced shipments between trading partners (International) has the highest negative effect on trade on average, with its peak in 2016. Furthermore, the score gaps in the ability to track and trade consignments (Tracking) and timeliness of shipments with the expected delivery time (Timeliness) also affect trade significantly negative, especially for the period 2010 to 2014. The score gap in the quality of trade and transport infrastructure is not significant through the whole examined period, which is opposite to the economic literature where transport infrastructure is one of the most important trade promotors. The situation is slightly different in the case of trade in intermediate goods between CEMS countries and ROW. The results of trade in intermediate goods are in line with the results of the previous works showing that most of sub-indices have significant effect on trade (Felipe & Kumar, Citation2012; Marti et al., Citation2014a; Puertas et al., Citation2014) while the results of trade in capital and consumption goods are inconclusive. These results lead us to the conclusion that logistics might be more important in trade in intermediate goods than in trade with capital and consumption goods.
In the case of trade in capital and consumption goods the results are quite ambiguous. In the case of trade in capital goods, the sub-indices Customs, International, Logistics and Tracking show the highest negative effects on trade in case of both EU15 and CEMS trade with the ROW. Unlike in the case of trade in intermediate goods, Timeliness is less important when it comes to the trade in capital goods, since the nature of trade in capital goods is different due to several reasons. Supply of capital goods is limited due to the fact that 10 countries account for 80% of world capital goods production (Mutreja et al., Citation2014). Also, demand is less elastic when compared with intermediate and consumption goods since buying capital goods is considered as a long-term investment. In the case of trade in consumption goods, there is a clear distinction between estimations results for EU15-ROW vs CEMS-ROW. Namely, in the case of CEMS-ROW, the significance and the signs of the estimated coefficient are in line with our expectations, that is, the larger the LPI gap between trading partners, the lower the trade in consumption goods. On the other side, the significance and the signs of the coefficients for the EU15-ROW case is counterintuitive; the coefficients are mostly not significant and are in some cases even positive, especially for the year 2018. We find the reason for these results in the economic development gap between EU15 and CEMS countries and that trade in consumption goods is mainly demand driven, while LPI sub-indices are supply-side oriented. Furthermore, we tested our model without GDP variable included and we obtained results that show that GDP differences between EU15 and CEMS with respect to their trading partners from the ROW countries could explain differences in results when it comes to trade in consumption goods.
Finally, since LPI index and its sub-indices are focused on supply chains, that is, they represent ‘simple comparators of how efficiently supply chains connect firms to markets or logistics performance’ (Arvis et al., Citation2018), we look at the EU15-ROW vs CEMS-ROW difference from regional and global supply chain perspective. We would like to stress out that in our paper we focus only on EU15 and CEMS trade with ROW, fully noting that regional supply chains within EU15 and between EU15 and CEMS have bigger importance to EU as a whole. Since it would be difficult to disentangle effects of LPI differences on intra-EU trade from other factors that affect strongly trade within EU, we focused only on trade of EU members with Third countries (ROW).
When we compare effects of LPI differences on trade in the case of EU15-ROW vs CEMS-ROW trade, is seems that these differences had more negative impact on EU15-ROW trade in the aftermath of the Great Recession and particularly for trade in intermediates, where we observe significant negative effects across almost all sub-indices in 2010. As the time passed, situation changed and in 2018 we observe that CEMS-ROW trade is highly affected by differences in logistics performance (). This can be attributed to the fact that throughout the observed period, considerable gap between LPI index and its sub-indices of CEMS countries with respect to EU15 did not diminish. Moreover, based on previous findings (Baldwin & Lopez-Gonzalez, Citation2015; Lejour et al., Citation2017) in this field as well as statistical data of average bilateral trade flows of EU15-ROW vs CEMS-ROW showed in , we argue that EU15 countries are more immersed in global supply chains and that this is the reason why they experienced larger negative effects of LPI differences in the aftermath of Global Recession as opposed to CEMS countries which are more immersed in regional supply chains and trade with EU15 and other countries of CEMS group of countries. Results for most recent available year (2018) show that CEMS countries are not converging to EU15 when it comes to trade with ROW and that are probably still oriented toward regional supply chain, that can limit their competitiveness in globalized market of 21th century.
6. Conclusion
The aim of this paper was to investigate the effects of logistics performance, specifically different logistics functions on international trade in different classes of goods and to investigate possible differences within EU member countries. By incorporating LPI sub-indices, as proxy variables for logistics performance in the gravity model, we tried to investigate which of the logistical functions should be treated with priority and how the improvements in trade logistics affect specific product groups. Furthermore, we were interested about the effects of the difference in LPI on bilateral trade between two groups of countries, EU15 and CEMS countries with the rest of the world countries. We used EU15 as a benchmark for CEMS.
Our results support previously mentioned findings where logistics performance has statistically significant impact on bilateral trade flows. The results also show that difference in LPI sub-indices score between trading partners negatively affects trade, however this effect is different for different classes of goods. Namely, biggest negative effect of difference in the levels of LPI sub-indices between trading partners is noted in the case of trade in intermediate goods, meaning that intermediate goods are more sensitive to trade than capital or consumption goods, where it plays less prominent role. It is also quite interesting to observe that sub-indices like timeliness, tracking and international, which are in the domain of the private sector are more significant for trade in intermediate goods, while customs and infrastructure are more relevant to trade in capital goods. Our findings also show that LPI differences between trading partners for both groups of EU countries affect trade in intermediate goods more strongly, but it varies through different years. Global recession from 2008, had negative effect on global trade that hit harder EU15 countries, which are more oriented to global supply chains in comparison to CEMS. In the long term, in our case, from 2010 to 2018, EU15 stabilised trade flows with ROW, while CEMS are still very much oriented toward regional trade and regional supply chains, with LPI being significant hurdle in trade with ROW.
Several important policy implications flow from our results. We argue that CEMS countries need to put more effort in the development of trade logistics to converge with EU15 in the development of logistics services because it will remove bottlenecks, provide better transport corridors for trade, help reduce trading time, and increase the competitiveness of the shipment prices. Above all, logistics performance is a gather work for both, public and private sector and in order to improve logistics performance, countries and integrations must simultaneously work on changes in many areas, namely infrastructure, border procedures and regulatory environment, transport regulation and private sector development. Private sector development should be one of the priorities for CEMS, considering that its components affect trade in intermediate goods. That way, CEMS countries will have more chances of increasing participation in global supply chains.
We acknowledge that an important limitation of our research is estimations of our model on cross-section data. Although we had at our disposal biennial panel data, we decided to go along with cross-section estimation since, in our opinion, time period is too short for robust estimation results. As for the future research, with more LPI data available over the years, focus should shift on panel data analysis. Another possible contribution to this field of research is to downsize the analysis on sectoral or firm level. Finally, more thorough research of different product groups withing different classes of goods (for example, within intermediate goods) could shed more light into relationship between trade and logistics performance.
Disclosure statement
No potential conflict of interest was reported by the author.
Additional information
Funding
Notes
1 In results we present only estimates of the coefficients of LPI-subindices. Size and significance of other gravity model variables included in Model 1 are mostly significant and have signs in line with theoretical predictions. Estimates of these coefficients are available upon request. Also, since presented results are obtained after estimating 180 models, we didn't show any goodness of fit statistics. Average coefficient of determination across all estimations, calculated as square value of the correlation between the predicted and the observed values of the dependent variable, is around 93%.
References
- Anderson, J. E., & van Wincoop, E. (2004). Trade costs. Journal of Economic Literature, 42(3), 691–751. https://doi.org/https://doi.org/10.1257/0022051042177649.
- Arvis, J.-F., Ojala, L., Wiederer, C., Shepard, B., Raj, A., Dairabayeva, K., Kiiski, T. (2018). Connecting to compete: Trade logistics in the global economy. https://openknowledge.worldbank.org/bitstream/handle/10986/29971/LPI2018.pdf.
- Arnon, A., & Weinblatt, J. (1998). Linder's hypothesis revisited: Income similarity effects for low income countries. Applied Economics Letters, 5(10), 607–611.
- Atabay, R. (2015). Validity of linder hypothesis in BRIC countries. Journal of International Trade, Logistics and Law, 1(2), 85–92.
- Baier, S. L., & Bergstrand, J. H. (2001). The growth of world trade: Tariffs, transport costs, and income similarity. Journal of International Economics, 53(1), 1–27. https://doi.org/https://doi.org/10.1016/S0022-1996(00)00060-X
- Baldwin, R., & Lopez-Gonzalez, J. (2015). Supply-chain trade: A portrait of global patterns and several testable hypotheses. The World Economy, 38(11), 1682–1721. https://doi.org/https://doi.org/10.1111/twec.12189
- Baldwin, R., Taglioni, D. (2006). Gravity for dummies and dummies for gravity equations. National Bureau of Economic Research Working Paper 12516.
- Behar, A., Manners, P. (2008). Logistics and exports. CSAE Working Paper Series 2008-13, Centre for the Study of African Economies, University of Oxford. https://ideas.repec.org/p/csa/wpaper/2008-13.html.
- Bergstrand, J. (1989). The generalized gravity equation, monopolistic competition, and the factor-proportions theory in international trade. The Review of Economics and Statistics, 71(1), 143–153.
- Bergstrand, J. H. (1985). The gravity equation in international trade: Some microeconomic foundations and empirical evidence. The Review of Economics and Statistics, 67(3), 474–481.
- Bresslein, M., Huber, S. (2016). International trade in parts, components, and final goods: Who trades what with whom? https://www.uni-regensburg.de/wirtschaftswissenschaften/vwl-moeller/medien/huber/pcf_online.pdf.
- Brun, J.-F., Carrere, C., Guillaumont, P., & de Melo, J. (2005). Has distance died? Evidence from a panel gravity model. World Bank Economic Review, 19(1), 99–119.
- Bugarčić, F. Ž., Skvarciany, V., & Stanišić, N. (2020). Logistics performance index in international trade: Case of Central and Eastern European and Western Balkans countries. Business: Theory and Practice, 21(2), 452–459. https://doi.org/https://doi.org/10.3846/btp.2020.12802.
- Clark, X., Dollar, D., & Micco, A. (2004). Port efficiency, maritime transport costs, and bilateral trade. Journal of Development Economics, 75(2), 417–450. https://doi.org/https://doi.org/10.1016/j.jdeveco.2004.06.005
- Djankov, S., Freund, C., & Pham, C. S. (2010). Trading on time. Review of Economics and Statistics, 92(1), 166–173. https://doi.org/https://doi.org/10.1596/1813-9450-3909. https://doi.org/https://doi.org/10.1162/rest.2009.11498
- Felipe, J., & Kumar, U. (2012). The role of trade facilitation in Central Asia. Eastern European Economics, 50(4), 5–20. https://doi.org/https://doi.org/10.2753/EEE0012-8775500401
- Frede, J., & Yetkiner, H. (2017). The regional trade dynamics of Turkey: A panel data gravity model. The Journal of International Trade & Economic Development, 26(6), 633–648.
- Gani, A. (2017). The logistics performance effect in international trade. The Asian Journal of Shipping and Logistics, 33(4), 279–288.
- Hertel, T., Mirza, T. (2009). The role of trade facilitation in South Asian economic integration. Study on intraregional trade and investment in South Asia. ADB. https://www.researchgate.net/publication/255626961_The_Role_of_Trade_Facilitation_in_South_Asian_Economic_Integration.
- Holzner, M., Tkalec, M., Vizek, M., & Vukšić, G. (2018). Fiscal devaluations: Evidence using bilateral trade balance data. Review of World Economics, 154(2), 247–275. https://doi.org/https://doi.org/10.1007/s10290-018-0309-5
- Host, A., Pavlić Skender, H., & Zaninović, P. A. (2019). Trade logistics – the gravity model approach. Journal Zbornik Radova Ekonomskog Fakulteta u Rijeci/Proceedings of Rijeka Faculty of Economics, 37(1), 327–342.
- Hummels, D. (2001). Time as a trade barrier (GTAP Working Papers). Center for Global Trade Analysis, Department of Agricultural Economics, Purdue University. [Retrieved from https://EconPapers.repec.org/RePEc:gta:workpp:1152].
- Hummels, D. (2007). Transportation costs and international trade in the second era of globalization. Journal of Economic Perspectives, 21(3), 131–154.
- Iwanow, T., & Kirkpatrick, C. (2007). Trade facilitation, regulatory quality and export performance. Journal of International Development, 19(6), 735–753. https://doi.org/https://doi.org/10.1002/jid.1399
- Krugman, P. (1991). Increasing returns and economic geography. Journal of Political Economy, 99(3), 483–499.
- Jošić, H., & Metelko, M. (2018). Testing the validity of Linder hypothesis for Croatia. Croatian Review of Economic, Business and Social Statistics, 4(1), 62–73. https://doi.org/https://doi.org/10.2478/crebss-2018-0006
- Lejour, A., Rojas-Romagosa, H., & Veenendaal, P. (2017). Identifying hubs and spokes in global supply chains using redirected trade in value added. Economic Systems Research, 29(1), 66–81.
- Limao, N., & Venables, A. J. (2001). Infrastructure, geographical disadvantage, transport costs, and trade. The World Bank Economic Review, 15(3), 451–479. https://doi.org/https://doi.org/10.1093/wber/15.3.451
- Liu, L., & Yue, C. (2013). Investigating the impacts of time delays on trade. Food Policy, 39(C), 108–114. https://doi.org/https://doi.org/10.1016/j.foodpol.2013.01.001
- Marti, L., Puertas, R., & Garcia, L. (2014a). Relevance of trade facilitation in emerging countries’ exports. The Journal of International Trade & Economic Development, 23(2), 202–222.
- Marti, L., Puertas, R., & Garcia, L. (2014b). The importance of the logistics performance index in international trade. Applied Economics, 46(24), 2982–2992.
- Mordor Intelligence. (2018). Freight and logistics market in Central and Eastern Europe (CEE)-growth, trends, and forecast (2020–2025). https://www.mordorintelligence.com/industry-reports/freight-and-logistics-market-in-central-and-eastern-europe.
- Mutreja, P., Ravikumar, B., Sposi, M. J. (2014). Capital goods trade and economic development. Working Paper No. 183. Federal Reserve Bank of Dallas Globalization and Monetary Policy Institute. https://econpapers.repec.org/paper/fipfeddgw/183.htm.
- Nords, H. K., Piermartini, R. (2004). Infrastructure and trade. WTO Staff Working Papers ERSD-2004-04, World Trade Organization (WTO), Economic Research and Statistics Division. https://ideas.repec.org/p/zbw/wtowps/ersd200404.html.
- Portugal-Perez, A., Wilson, J. (2010). Export performance and trade facilitation reform: Hard and soft infrastructure. Policy Research Working Paper Series 5261. The World Bank.
- Puertas, R., Marti, L., & Garcia, L. (2014). Logistics performance and export competitiveness: European experience. Empirica, 41(3), 467–480. https://doi.org/https://doi.org/10.1007/s10663-013-9241-z
- Saslavsky, D., & Shepherd, B. (2014). Facilitating international production networks: The role of trade logistics. The Journal of International Trade & Economic Development, 23(7), 979–999.
- Silva, J. M. C. S., & Tenreyro, S. (2006). The log of gravity. Review of Economics and Statistics, 88(4), 641–658. https://doi.org/https://doi.org/10.1162/rest.88.4.641
- Soloaga, I., Wilson, J. S. & Mejia, A. (2006). Moving forward faster:trade facilitation reform and Mexican competitiveness. Policy Research Working Paper Series 3953, The World Bank. https://ideas.repec.org/p/wbk/wbrwps/3953.html.
- Tinbergen, J. (1962). An analysis of world trade flows. In J. Tinbergen (Ed.), Shaping the world economy (pp. 27–30). The Twentieth Century Fund.
- Wilson, J., Mann, C., & Otsuki, T. (2003). Trade facilitation and economic development: A new approach to quantifying the impact. The World Bank Economic Review, 17(3), 367–389. https://doi.org/https://doi.org/10.1093/wber/lhg027
- Zajc Kejžar, K., Kostevc, Č., & Zaninović, V. (2016). The role of regional economic integrations for trade margins: A case of Croatia. Journal Zbornik Radova Ekonomskog Fakulteta u Rijeci/Proceedings of Rijeka Faculty of Economics, 34(1), 11–41. https://doi.org/https://doi.org/10.18045/zbefri.2016.1.11.