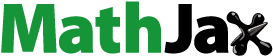
Abstract
World Tourism Organisation, declares the Tour Operators as tourism engine of strategically importance to support jobs and inclusive growth in all regions. Tour operators emerges following the 2008 crises, as a global job engine. Its atypical profile of highest human capital concentrator in tourism, attract and retain talents, works digital with a high-intensity information use. Is a rapid adopter of technological innovation, generate high value added in highly competitive global markets.
We look in this paper to understand why employment is growing or declining in a regional tourism tour operator sector during 2008–2018, in some EU28 regions? We use Exploratory Spatial Data Analysis to map the indicator ‘tour operator’s employment growth’ components decomposed by the Shift Share Analysis Method. Analysed Eurostat data for 266 regions (281 regions) indicates that for the average regional tour operators employment growth heterogeneity is driven almost at half by region-specific factors. The main contributions are: identifying this indicator as appropriate to be a core one in OECD (2013) tourism competitiveness framework & redefine tour operator sector as a core sector of tourism in the Global model of tourism of Harrison.
Introduction
In the last two decades, tourism become the world’s largest industry (Xin, Citation2015), acting globally it brings together ‘regions, tourists, business suppliers, economies, governments, communities and environments’. Tourism industry and traveling according to Turner (Citation2018) creates jobs, contributes substantially to prosperity and global development. Tribe (Citation1997) and Bhatia (2007) defined the tourism industry structure as being made from producers (carriers, accommodation and man-made attractions) and support service suppliers (private or public). Tour operators and travel agents are classified as mainly tourism industry by EUROSTAT and codified N79.Footnote1 Both categories have similar objective to create value for tourists.
Tour operators plays, recently the main role in maximise customer value and gain a competitive advantage in the global markets. Also, tourists, tour operators, and service suppliers’ frames a complete tourism supply chain. Shang-Yu Liu and Wei-Shuo Lo (Citation2016) and Dupeyras and MacCallum (Citation2013) link the operator’s presence with enhancing country competitiveness. Tour operators sector account 668.8 thousand persons employed in 2018, increasing with 70.8 thousand in the same period, having a growth of 11.8%, 4.6 higher than the total employment growing rate.
Tour operators sector is the highest human capital concentrator among all tourist industries. It attracts and retains talents, tertiary share in employment is 47.6%, classified as Knowledge Intensive Activities (KIA, over 33%). This sector is a fast integrator of technological innovation OECD (2007)Footnote2 classified by intensity of technology and knowledge usage as a Less Knowledge-Intensive Market Services (LKIMS). That means this sector share the KIS sectors characteristics: generate high value added (over 21% according to OECD 2007), have a high-intensity information use, based on ICT and digitisation adoption working in highly competitive markets. Tour operators, as KIS, presents a multidimensional profile: (i) knowledge – generate and exploit; (ii) innovation and (iii) spatial proximity/regional dimension (Muller & Doloreux, Citation2007). Also, is an innovation creator not only an innovation adopter (Bryson & Daniels, Citation2007, p.179). At the same time, it acts as a highly gender inclusive sector, women share is 63% from total employment (Eurostat, Citation2019).Footnote3
On the background that ‘Europe continues to stand as the most visited region, welcoming half of the world’s international tourist arrivals’,Footnote4 World Tourism Organisation (UNWTO, Citation2018) declares the Tour Operators as tourism engine of strategically importance to support jobs and inclusive growth in all regions.
Spain’s case, the global tourism leader, open the debates in regard the relationship between tourism and economic growth, when high shocks acts (i.e. 2008 Global Financial and Economic Crisis, the Arab Spring) (Perles-Ribes et al., Citation2017).
Dupeyras and MacCallum (Citation2013) creates a framework for comparative measurement of competitiveness in tourism and iterates as research gap the assessment of ability of a destination to deliver quality and competitive tourism services through employment growth. Other gaps highlighted by the literature are: assessing the structure of the tourism sector; the structure of tourism supply chains; and the use of e-tourism, innovative services, social media, etc.
The gap we are addressing by our study is the competiveness of tourism industry through employment growth using a multi-perspective spatial analysis of the tourism potential in EU in 28 countries at NUTS2 level for N79 (the national change effect, industrial mix effect, and regional competitiveness effect).
Starting from Moretti's (Citation2012) conclusion that the agglomerations of high human capital predict the success of locations and provide a multiplier effect, we selected the indicator ‘Employment growth in tour operators sector, in a region in a time period’ as a tool to measure the destination ability to generate competitive tourism services through employment growth.
Research question: What regions in EU, during 2008–2018, registered employment growth tourism industry (N79) and where is located a high potential of his sector?
Our research topic informs about the key theoretical perspective of global tourism competitiveness in the development paradigm. The key theoretical perspective is that talents changes the tourism structure adopting in a accelerate manner the digital transformation opportunities, increasing competitiveness of destination region. The global tourism paradox is that mass tourism push pressure for a tourist region, regardless development level, to be competitively at global scale. The optimal tourism structure is a mainly spatial analysis object.
This study introduction presents the tour operators broader framework, employment growth effect for tourism industry. Literature review looks for tourism theory and tourism operators, tourism competitiveness for a destination and tourism operator’s regional employment growth. The methodology is a mix of standardised methods: Exploratory Spatial Data Analysis (ESDA) as a tool for map the tour operator’s employment growth components at regional level, decomposed by the Shift Share Analysis (SSA)’s (National Share, Industrial Mix Share and Competitive Effect). Results and discussions picture the maps and details for regions with competitive advantage, relative specialisation and high endogenous effect on tour operator’s employment growth. Paper ends with the findings of the study, highlighting the theoretical and practical contributions and the limitations of the methods and indicators used in this study. Moreover, we articulate further developments in research development and guidelines for policy makers.
Literature review
Tourism theory and tourism operators
Cukier (Citation2006) recently consecrate tourism as an academic discipline and the tourism complexity needs interdisciplinary approach in its study (Tribe, Citation1997). According to Jennings (Citation2006) ‘epistemologies and hegemony no longer represent an accountable tourism research agenda in a twenty-first century world of flux and unpredictability’, while Xin (Citation2015) bring in the conceptual inquiry. Stergiou and Airey (Citation2018) conclude that the elusive and eclectic character of tourism theory make it difficult to delineate, reflecting its immaturity. Ritchie et al. (Citation2008) conceptualises the Core-foundational Model of Tourism as a result of interpretation of the interface between Tourism Theory and its Foundation Disciplines, emphasising its highly disciplinary pluralism. The economic and management stream brings the richest theoretical contributions and according to Stergiou and Airey (Citation2018) Smart Tourism emerges as new research field. Ye et al. (Citation2020) points, on the context of 5 G technology adoption, that ‘the prevalence of Internet of Things (IoT), mobile applications (apps), location-based services, geo-tag services, Virtual Reality (VR), Augmented Reality (AR), social media, and smart devices offers immense opportunities for tourism stakeholders to generate, store, and retrieve big data that serve various purposes’.
Tour operators could be reflected by the area of event tourism as field of study and area of professional practice of planned events (Getz & Page, Citation2016). Tour operators as KIS, should evolve towards ‘tour information service support system, core agents for smart tourism’ (Li et al., Citation2017).
Harrison (Citation2015) distinguish the Global model of tourism political economy that incorporates both developed and developing societies, under the development theory paradigm. Sharpley (Citation2009) identifies the evolution of Development Theory from 1950s in the following process stages: Modernisation theory (MT), MT& dependency theory, Neoliberalism, Alternative development, Sustainable development, Post-development and the demand for a New Paradigm.
Tourism competitiveness for a destination
One topic representative for tourism management perspective is the destination competitiveness. OECD build in 2013 a shared definition of tourism competitiveness for a destination: ‘is about the ability of the place to optimise its attractiveness for residents and non-residents, to deliver quality, innovative, and attractive (e.g. providing good value for money) tourism services to consumers and to gain market shares on the domestic and global market places, while ensuring that the available resources supporting tourism are used efficiently and in a sustainable way’ (Dupeyras & MacCallum, Citation2013).
Salinas-Fernández et al. (Citation2020) show that ‘tourism destination competitiveness is a multidimensional concept that is widely studied in the academic literature’, but difficult to measure. Travel & Tourism Competitiveness Index, is the most popular compound index designed by Word Economic forum since 2007, Guaita-Martinez et al. (Citation2019) are proposing a new methodology for TTCI to classify the 136 country.
Dupeyras and MacCallum (Citation2013) identifies two practical approaches: strong chains as potential for development or weakness in chains to improve competitiveness.
In 2013 the 31 OECD members and partner countries creates the Framework for comparative measurement of competitiveness in tourism (Dupeyras & MacCallum, Citation2013). The Framework comprises three types of indicator that can be applied to measure competitiveness in tourism − 11 core indicators, 5 supplementary indicators and 4 for future development indicators. The ‘Employment in tourism by age, education levels and type of contracts’ is an additional non-core indicators. This indicator measures ‘Assessment of Ability of a destination to deliver quality and competitive tourism services through employment growth’. Interpretation is seen as ‘a measure that would assess ability to attract, retain and develop talent in the industry to enable improved competitiveness’ (Dupeyras & MacCallum, Citation2013).
Tourism operators employment growth and regional competitiveness
The growth performance difference of the relative to national average is explained by Classical Shift Share Analysis (SSA) as the result its economic structure and/or the growing rate of its sectors. Sentz (Citation2011) describes SSA as ‘a standard regional analysis method that attempts to determine how much of regional job growth can be attributed to national trends and how much is due to unique regional factors’. Its research question is: Why employment is growing or declining in a regional industry, cluster, or occupation? According to Lailani (Citation2014) SSA is a relative simple analysis ‘especially useful for understanding what is happening in an industry that is growing locally but declining nationally (or the reverse)’. SSA and Local Quotient Techniques (LQT) are used also in tandem to analysis the behaviour of regional economies. Munawir (Citation2014) identify through SSA the Bandung sectors with a competitive advantage when compared to West-Java and Indonesia. Prats and Ramirez (Citation2018) asses using SSA the levels of efficiency in the regional structure in the state of Tabasco from 2003 to 2013.
Fuchs et al. (Citation2000) make with SSA the Asian regions profile by the relative competitive advantage in tourism. Yasin et al. (Citation2004) apply SSA to characterise the Portuguese tourism industry in the context of the challenges and opportunities of the global tourism.
Dogru and Sirakaya-Turk (Citation2017) improved the SSA method with the Shift-share regression for measuring the tourism industry's performance in a South Carolina in the USA. Shi et al. (Citation2007) in Jiangsu Province and Firgo and Fritz (Citation2017) in Australia applies a spatially extended SSA and a modified dynamic shift-share model to analyse the spatial competitiveness of international tourism in in comparison with its neighbours. Traistaru and Wolff (Citation2002) apply SSA on employment data at county level, counting 89 regions for Bulgaria, Hungary and Romania for the period 1990–1999. Their results confirm that the regional employment growth heterogeneity is driven almost entirely by region-specific factors, while the industry mix and regional competitiveness factors play only a minor role.
Capello and Fratesi (Citation2011) evidenced that the degree of participation of the local economy dimension next to the presence of mega cities is a measure of the degree of integration of a local economy in a global economy. Artige and Neuss (Citation2013) compare the two growth effects of a geographical unit with those of any other geographical unit without defining a reference territory, eliminating the flaws identified by Dunn (Citation2005) and Esteban-Marquillas (Citation1972).
You et al. (Citation2010) apply the spatial expansion model with SSA to the six provinces of central China. Authors concludes that ‘if the competition component difference is positive, it indicates that the neighbourhoods increase the competitiveness of that industry, if not, it means that it hinders the enhancement of the competitiveness of the industry, and so on’. Goschin (Citation2014) emphasise for Romania that that the developed regions recovers more easily from the crisis due to their economic potential, but appropriate regional policies are requested. Zaman et al. (Citation2015) show that tour operators employment growth could support the sustainable regional growth in the sense endogenous development.
Methodology
Classical SSA, is an old and ease of use method to study regional growth patterns. Was developed by Creamer since 1942 and formalised by Fuchs (Citation1962) and Ashby (Citation1964). Buck (Citation1970) examines empirically the usefulness of shift and share analysis as applied to regional employment growth.
Herzog and Olsen (Citation1977) points about the Problem of ‘Weights’ and The Problem of ‘Interwoven Effects’ of the SSA. The SSA in the Problem of ‘Weights’ do not take into account the ‘changes in regional industrial structure over the analysis period’ (Dunn, Citation2005; Fuchs, Citation1959; Klaassen & Paelinck, Citation1972) and for The Problem of ‘Interwoven Effects of regional structure determines the magnitude of both the industry-mix and competitive effects, therefore the competitive advantage/disadvantage is relative’.
Casler (Citation1989) propose a theoretical context for SSA, based on standard microeconomic theory. Graham Shaw and Spence (Citation1998) recommends modifications to the labour demand SSM, including input price and technological growth effects.
Argues among the advantages of deterministic SSM is the result to Blien et al. (Citation2014) recommend dynamic SSA to show the structural effect. O'Leary and Webber (Citation2015) apply for 181 European regions from 1980 to 2007 dynamic SSA shows the ‘importance of structural change for growth and convergence’.
Esteban-Marquillas (Citation1972) redefines the competitive position and creates a fourth shift-share component, the ‘allocation effect’.
Shift share equations
In 1992 Selting and Loveridge defines the ‘SSM as a method of decomposing employment patterns into expected (share) and differential (shift) components’. In this context, Selting and Loveridge (Citation1992) uses SSM for analysing: (a) spatial heterogeneities at national level and at regional level; (b) examining lateral variations in growth (the case of two regions with similar economic structure but with different growth performance); (c) the one industry performance levels differentiated by region. SSM, decompose the regional change in employment growth during a period of time, in the following effects (or components): the national growth effect, the industrial mix effect, and the competitive effect:
(1)
(1)
E = employment level
i = the number of sectors or industries in a region or nation (i = 1,2,…s)
j = the number of regions in an geographical area (j = 1,2,…r)
Eij = employment in the ith sector in the jth region
Change in Employment in a certain industry (i) from the region (j) during [t-1:t] period
= Employment in a certain industry (i) in the (j) region at the time (t)
= Change due to National Trends; National Growth Effect
= Change due to Industrial Mix; Industrial Mix Effect
= Change due to Regional shift or Competitive Effect
National share
The national growth effect is the ‘amount that total regional employment would have grown if it grew at precisely the same rate as total employment in the nation as a whole’ (Stilwell, 1969, p.163). Implicitly, the model asserts that the industries in a region will grow at approximately the rate of national industries unless the region has a comparative advantage or disadvantage (Bishop & Simpson, Citation1972; Knudsen & Barff, Citation1991; Loveridge Citation1995; Selting & Loveridge, Citation1992, p.4)
(2)
(2)
Employment in the region in that industry in the first year [t-1];
National employment growth rate in [t-1: t] period
= total national employment
at initial moment t-1
= total national employment
at final moment t
Industrial mix share
Regions differ by their economic structure and performance. Each region could be characterised by a sectorial pattern with different combinations of slow-growing sectors and specialised in sectors with high growth rates, both relative to the national average. In SSA the Industry Mix Effect dimension describes the regional variations in industrial composition. Selting and Loveridge (Citation1992) defines the industry mix as ‘the amount of growth attributable to differences in the sectorial makeup of the region versus that of the nation. The summation of the industry mix over each of the industries in the region, IM0, provides a total industry mix effect for all sectors in the region. A positive total industry mix implies the region is specialized in industries that, nationally, are experiencing greater growth than the overall national average. A negative total industry mix means that a region has higher than average proportions of people employed in industries that are sluggish relative to the average growth of all national industries’.
(3)
(3)
eio= the percentage change in nationwide employment for industry i
= national employment in the ith industry
at initial moment t-1
= national employment in the ith industry
at final moment t
Regional shift (Or local share)/competitive effect
‘If industry i in region j grows at anything other than the sum of the national growth effect and the industry mix (i.e. the region's share), the residual is ascribed to the competitive effect. The competitive effect as a “shift” from what would be expected if the region's industry grew at exactly the proportion of national growth and industry mix’ (Selting & Loveridge, Citation1992). The ‘implicit in shift-share analysis is the assumption that regional economies should grow at national growth rates unless there are comparative advantages or disadvantages operating at the regional level’ (Bishop and Simpson, Citation1972). In the classical SSA, is considered that ‘A positive competitive effect means that a region's industry is growing faster than the national average industry growth rate and a negative competitive effect implies that a region's industrial growth is lagging behind national industries manufacturing the same products’ (Stevens and Moore, Citation1980, cited by Selting & Loveridge, Citation1992). The growth attributed to the competitive effect is the value that is left after the national growth effect and industry mix are subtracted. This residual is inferred to result from factors that are unique to the region. The competitive effect arises ‘from interregional differences affecting a given area's attractiveness to the activity’ (James and Hughes, Citation1973, p.223). These differences are developed because of endogenous factors inherent to the region (Dawson, 1982). The competitive effect can be thought of as a measurement of a region's competitive edge or comparative advantage in the production of the goods in the ith industry.
While the shift-share competitive effect describes whether regional conditions favour or discourage growth, it does not provide answers as to why a strongly positive shift exists in one region but not in another. Reasons for differential growth arise from an amalgam of factors, which may include different levels of resource endowments, multiplier effects, agglomeration economies, or policy measures such as low business taxes or high investments in human capital formation. By itself, shift-share cannot ferret out which factors are at work in various regions. (Selting & Loveridge, Citation1992, p.4)
(4)
(4)
eij= the percentage of change in employment in industry i, region j relative to a base year
While the shift share competitive effect is known also as Endogenous versus Exogenous Growth Differentiation a definition from which shift-share derives part of its name is that of the regional share. The sum of the national growth effect and the industry mix (NE0j + IM0j) are together called the ‘region's proportion or share of growth’. Both, the national growth effect and the industry mix effect, are exogenous factors that are determined by national growth rates, not local or regional economic conditions. Together, they comprise the region's expected growth, or the growth that would occur in the region if each of the industries grew at the same rate as the nation as a whole (Selting & Loveridge, Citation1992).
Exploratory spatial data analysis (ESDA)
From the ESDA techniques we use the Choropleth Maps which represents ‘Counterpart of Histogram, where are values/attributes for discrete spatial units with associate colours palette (Anselin, Citation2002). The maps uses geocoded data and we represents the variables using 5 classes Natural Breaks (Jenks) Classification. This classification technique is an optimisation method for Choropleth Maps, minimises variation in each group, applied in Arc GIS desktop 9.3. This method allows identifying clusters where data values are ‘placed into a single class. Class breaks occur where there is a gap between clusters’. In this case, ‘data is unevenly distributed; that is, many features have the same or similar values and there are gaps between groups of values’ (ArcGIS 9.2. Desktop Help, Citation2008).
Data collection
Tour operators (N79) are fully included in the Eurostat (Citation2019) lately selection of NACE Rev.2 classes, at 4 digits. Eurostat methodologyFootnote5 reports in 2016 over 13.36 million persons employed in the EU28 tourism industries, from which 521.34 thousand persons in tour operators’ sector. Employment in tourism industries is concentrated, more than 2/3 in top five countries: Germany, United Kingdom, Spain, Italy and France. Tour operator’s employment is 3.9% in total employment for Spain and France, share equal with the EU28 mean. This share is over the Eu28 mean in Germany (4.2%) and United Kingdom (4%) and below in Italy (3.2%).
Employment data used to apply SSA standard at national level for each EU28 countries are provided by Eurostat: at national level [lfsa_egan2] and at regional (NUTS2) Footnote6 level for 266 from a total of 281 regions [sbs_r_nuts06_r2]. General time frame is 2008–2016.
Results and discussions
The classical SSA has some limits: cannot explain whether changes in employment are significant, economic structure dynamics effect is ignored, the absence of tests of the evaluations, etc. Also, economic growth is difficult to measure directly, changes in the level of regional employment (in our case) have become accepted surrogates for economic growth.
Our results are reliable as consistency or repeatability by the Eurostat indicator, fully comparable across time and space, used in the methodology.
National Growth Effect in EU28 during 2008--2016 period points that 173 regions from 12 countries present positive national share of tour operator’s employment. The illustrate the National Share component of N79 employment, calculated independently by each country, applying ESDA technique. These regions have a comparative advantage provided by studied sector, compared to all other economic sectors and present a Centre-Periphery pattern, across EU28 map.
Figure 1. Employment change in N79 due to National Trends; National Growth Effect in EU28 during 2008–2016 period at NUTS 2 level.
Source: Map made by authors, ESRI SHAPE file.
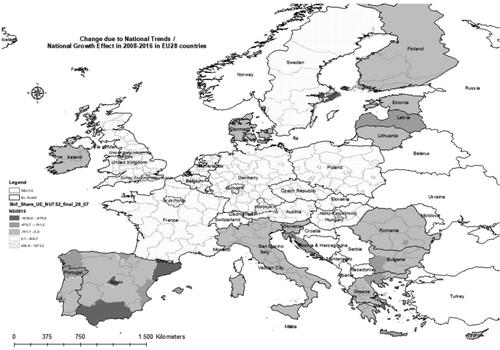
indicate that the regions with competitive advantage in tour operators are clustered in countries with 80% total regions with this characteristic. Regions with competitive advantage in tour operators sector covers in average 61.1% from total regions at EU28 level.
Table 1. Number of regions by countries with regional employment growth in N79 higher than total growth employment rate during 2008–2016 in EU28: number of regions with competitive advantage in the N79 sector [number of regions].
In are presented the selected regions by countries with N79 regional employment positive modification, higher than 406 employees, during 2008--2016 period, in EU28. These are the EU 28’s regions with competitive advantage a best employment performance [employed persons] with a threshold given by Jenks classification ESDA method. The map result is in line with (You et al., Citation2010) neighbourhood pattern – the best performance in employment growth regions are surrounded by regions with relative advantage in N79 employment relative to nationwide employment.
Table 2. Selected regions by countries with N79 regional employment positive modification, higher than 406 employees, during 2008–2016 period, in EU28: the regions with competitive advantage a best employment performance [employed persons].
Frankfurt, Paris, London, Budapest, Stockholm, Köln – metropolis are at the heart of these regions, tendency in line with (WTTC, Citation2019).
Industrial mix present in the 144 regions that are relatively specialised in tour operators sector that, nationally, are experiencing greater growth than the overall national average. In are presented the 14 countries which presents the shares of regions specialised in N79 over 66%, while the average share at EU28 is 51.2%.
Figure 2. Employment change in N79 due to Industrial Mix; Industrial Mix Effect in EU28 during 2008-–2016 period at NUTS 2 level.
Source: Map made by authors, ESRI SHAPE file.
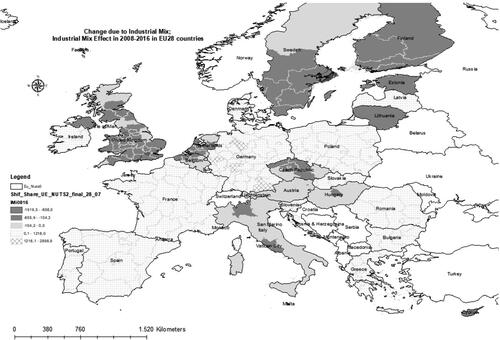
Table 3. Number of regions by countries specialised in N79 during 2008–2016 in EU28 [number of regions].
reveals one spatial pattern base on relative contiguity,Footnote7 from South West to North (Portugal, Spain, France, Germany and Poland) and down to South East (Poland, Slovenia, Romania, Bulgaria, Macedonia and Greece) in a U revers shape. In this pattern is connected also Denmark and Latvia, Ireland is an ‘island’ in terms of specialisation. The Specialisation effect is spread in a pattern of U reverse shape, indicating a contiguity & connectivity effect presence. High degree of specialisation indicate involvement in globalisation (Capello & Fratesi, Citation2011).
In are presented the 5 selected regions by countries with a positive total industry mix effect higher than 1216 employees. These are the EU 28’s regions with relative specialisation in tour operator’s profile, providing the best employment performance [employed persons] with a threshold given by Jenks classification ESDA method. Among the 5 leading regions 4 are from Germany, again Darmstadt on the first place and one is from Greece, Attiki having the second rank by employment growth. Frankfurt, the geographical centre of EU enlarged, ‘is an alpha world city and a global hub for commerce, culture, education, tourism and transportation’, major city from Darmstadt. Also, Attiki have Athens as capital, a capital with a Smart Specialisation Platform.Footnote8
Table 4. Selected regions by countries with a positive total industry mix effect higher than 1216 employees in N79 at NUTs 2 level, during 2008–2016 period, in EU28. The amount of growth regarded as being caused by the differences in sectoral employment in N79 and the employment growth at national level. [Employed persons].
present the 116 regions that have a positive competitive effect, respectively high endogenous growth effect, experiencing greater growth than the overall national average. The tour operator’s employment in these regions grows faster than the sector employment at national average growth rate. This local growth rate is specific for the region. () Supplementary we add the last overall rankings in 2019 and rank change since 2017 of the Travel & Tourism Competitiveness Index (TTCI). Calderwood et al. (Citation2019) Our results are convergent with TTCI ranking for the best performer. There are 4 countries (United Kingdom, Germany, Italy and France, exception for Spain) from the top 5 TTCI rank, with large numbers of regions (over 12) that have high endogenous growth effect for Employment growth in tour operators. But all these countries keep their rank in 2017 compared to 2019 (exception for United Kingdom that lose on place). In terms of progress of increasing competitiveness, among studied countries, Romania reached the highest score positive modification of 12 during 2017–2019 for TTCI driven by 2 regions. Denmark increases its rank with 10 places in 2 regions from total 5 regions. Slovenia (in 1 region from 2) and Finland (in all its 5 regions) also increases their rank with 5 places. Our indicator offers complementary information’s, at regional level for TTCI, allowing deep insights. Among best performers, Italy proves to be more competitive in 13 regions from its 21 regions with a regional coverage of 61.9%, closed followed by United Kingdom with 51.4% endogenous regional coverage. Finland, Austria, Belgium and Slovenia, are small countries with high coverage of endogenous competitive effect, with coverage also higher than 50% from all regions.
Figure 3. Employment change in N79 due to Regional shift or Competitive Effect in EU28 during 2008–2016 period at NUTS 2 level.
Source: Map made by authors, ESRI SHAPE file.
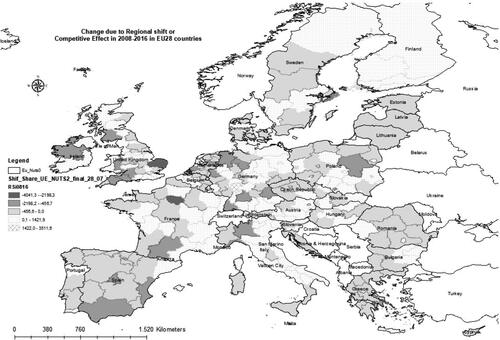
Table 5. Number of regions in which the N79 has a positive competitive effect – the employment in the N79 in these regions grows faster than the employment in the N79 sector at national average growth rate: the growth is given by the specific of the region [number of regions].
Among the 21 countries with selected 116 regions there are 11 countries counting 96 regions presents a Share of regions with positive competitive effect provided by N79 higher than EU28 average of 40% ().
Table 6. Selected regions by countries with a positive competitive effect higher than 1422 employees in N79 at NUTS 2 level, during 2016–2018 period, in EU28. Regions where the N79 employment is growing faster than the national average industry growth rate. [Employed persons].
Regional conditions favour in the highest level employment growth in Darmstadt and Nord-Holland hosting mega cities like Frankfurt and Amsterdam working as Global Hubs (Harrison, Citation2015). Here the employment increases in the mentioned period with over 3225 person. Regions with a good competitive effect (second class in ) could improve the tour operator’s sector performance more if connect to the Global Hubs. Germany proves to be highly global connected and with the highest number of regions competitive endogenous for tour operators growth performance.
indicates a randomised spatial profile of competitive effect, the region specific conditions to support tour operator’s employment growth, with small clusters national and cross border. This result is similar with Batista e Silva et al. (Citation2018) spatiotemporal patterns of tourism in Europe at high-resolution with conventional and big data sources.
Some of the results are simply snap shots that confirms the tourism megatrends announced by (WTTC, Citation2019) ‘today’s hyper-connected world, power and demographic shifts from West to East and nations to cities’.
Conclusion
In EU28 (266 from a total of 281 regions) during 2008–2016 period, the over 40% of the average regional tour operators employment growth heterogeneity is driven almost by region-specific factors. This finding is in line with Traistaru and Wolff (Citation2002)’s conclusion, at smaller scale. Following You et al. (Citation2010), these 116 regions with a positive competitive component increases the competitiveness of tour operators sector as well as tourism industry.
Our main contribution is the decomposing with SSA of the Employment growth in tour operator’s sector analysis at regional level during 2008–2016 period. This indicator is highly relevant for:
Theory: tour operator sector is a core sector of tourism in the Global model of tourism announced by Harrison (Citation2015). It plays the role of the ‘brain’ for the smart tourism (Stergiou & Airey, Citation2018), is not support service but creator for tourism products;
Practice: the identification of a core indicator appropriate for OECD (2013) tourism competitiveness framework.
This indicator allow deep analysis for endogenous competitive regions capacity to attract and retain talents, e-tourism and other innovative services proxy measure, insights regarding the structure of tourism supply chains, on innovation and use of social media in the tourism industry (Dupeyras & MacCallum, Citation2013) and a measure of industry thickness dynamics, clusters and competitiveness, existing/potential. Supplementary, we provide details regarding the spatial structure of agglomerations and clusters identified at regional level. Regions with high rates of tour operators indicates the Jacobian externalities agglomeration (Jacobs, Citation1961, Citation1969), acting as regional knowledge spill overs in a region, the variety of sectors are related or unrelated (Frenken et al., Citation2007). The Morettian Human capital spill over effect is tremendous important for education policy, for new talents attraction in the region (Moretti, Citation2004).
In short, the tour operator presence agglomeration increases the resilience of the hosted region and their cities.
Methodological limitations – SSA does not indicate why these industries are competitive, merely shows the sectors in which the region is outcompeting or under competing the nation (Sentz, Citation2011), considering that the competitive advantage is relative (Dunn, Citation2005; Fuchs, Citation1959; Klaassen & Paelinck, Citation1972).
Data and indicator limitations – Seasonality. Monthly frequency will be an improvement; activity structure of tour operators; employment contract type; county level are additional variables to be considered.
Further developments in research development in the field of tour operators are: the speed of digital transformation adoption assessment to fully beneficiate of the opportunities; platform employment (gig economy) from tourism impact on job creation; optimal tourism sector structure at the best spatial granularity; effects of global connectivity in networks and Global Hubs, relationship with smart cities for EU tourism network management.
The results of the present study offers guidelines for policy makers:
The map of regions with effect on tour operators competitiveness as input for tourism policy and entrepreneurship development;
Locations and inputs for priorities Human capital, active, investments in digital infrastructure policies;
Benchmarking of EU28 the regions by endogenous Competitive Effect on tour operators employment growth;
The growth of employment in tour operators sector is an indicator that allow to provide diverse and deeper insight, useful for tourism understanding and practice improvement.
Our results goes beyond the ‘common sense’ of the touristic countries. Some of the results are simply snap shots that confirms the tourism megatrends announced by (WTTC, Citation2019) ‘today’s hyper-connected world, power and demographic shifts from West to East and nations to cities are redefining centres of influence and reshaping global markets, while individuals increasingly mobilise and demand accountability’.
Disclosure statement
No potential conflict of interest was reported by the authors.
Additional information
Funding
Notes
2 OECD Science, Technology and Industry Scoreboard 2007, p2 10
3 Eurostat, 2018. Tourism industries – employment. Statistics Explained https://ec.europa.eu/eurostat/statistics-explained/pdfscache/46236.pdf
4 European Union Tourism Trends, https://www.e-unwto.org/doi/pdf/10.18111/9789284419470
6 Nomenclature of Territorial Units for Statistics (NUTS).https://ec.europa.eu/eurostat/statistics-explained/index.php/Glossary:Nomenclature_of_territorial_units_for_statistics_(NUTS)
7 Between Slovenia and Romania is a discontiguity, but in terms of distance is below 100 km. on the background of entire EU map we consider this situation as relative contiguity.
References
- Anselin, L. (2002). Mapping and analysis for spatial social science. http://www.csiss.org/events/conferences/2002/anselin_aaa.pdf
- ArcGIS 9.2. Desktop Help. (2008). Classification methods. http://webhelp.esri.com/arcgisdesktop/9.2/index.cfm?TopicName=Classification_methods.
- Artige, L., & Neuss, L.V. (2013). A new shift-share method (No. 1302), CREPP Working Papers. Centre de Recherche en Economie Publique et de la Population (CREPP) (Research Center on Public and Population Economics) HEC-Management School. University of Liège.
- Ashby, L. D. (1964). The geographical redistribution of employment: an examination of the elements of change. Survey of Current Business, 44(10), 13–20.
- Batista e Silva, F., Marín Herrera, M. A., Rosina, K., Ribeiro Barranco, R., Freire, S., & Schiavina, M. (2018). Analysing spatiotemporal patterns of tourism in Europe at high-resolution with conventional and big data sources. Tourism Management, 68, 101–115. https://doi.org/https://doi.org/10.1016/j.tourman.2018.02.020
- Bishop, K. C., & Simpson, C. E. (1972). Components of change analysis: problems of alternative approaches to industrial structure. Regional Studies, 6(1), 59–68.
- Blien, U., Eigenhüller, L., Promberger, M., & Schanne, N. (2014). The shift-share regression: An application to regional employment development in Bavaria. In K. Kourtit, P. Nijkamp, & R. Stimson (Eds.), Applied regional growth and innovation models. Springer Berlin Heidelberg, Berlin, Heidelberg. pp. 109–137. https://doi.org/https://doi.org/10.1007/978-3-642-37819-5_6
- Bryson, J., Daniels, P. (2007). The handbook of service industries [WWW Document]. https://www.e-elgar.com/shop/the-handbook-of-service-industries
- Buck, T. W. (1970). Shift and share analysis—A guide to regional policy? Regional Studies, 4(4), 445–450. https://doi.org/https://doi.org/10.1080/09595237000185441
- Calderwood, L. U., & Soshkin, M. (2019). The travel & tourism competitiveness report 2019. In World Economic Forum.
- Capello, R., & Fratesi, U. (2011). ERSA Conference Papers. Globalization and endogenous regional growth (No. ersa10p677). European Regional Science Association.
- Casler, S. D. (1989). A theoretical context for shift and share analysis. Regional Studies, 23(1), 43–48. https://doi.org/https://doi.org/10.1080/00343408912331345272
- Cukier, J. (2006). Tourism research: policy, planning and prospects. Department of Geography University of Waterloo.
- Dogru, T., & Sirakaya-Turk, E. (2017). Engines of tourism’s growth: An examination of efficacy of shift-share regression analysis in South Carolina. Tourism Management, 58, 205–214. https://doi.org/https://doi.org/10.1016/j.tourman.2016.10.021
- Dunn, E. S. (2005). A statistical and analytical technique for regional analysis. Papers in Regional Science, 6(1), 97–112. https://doi.org/https://doi.org/10.1111/j.1435-5597.1960.tb01705.x
- Dupeyras, A., & MacCallum, N. (2013). Indicators for measuring competitiveness in tourism: A guidance document (OECD Tourism Papers No. 2013/02; OECD Tourism Papers, Vol. 2013/02). https://doi.org/https://doi.org/10.1787/5k47t9q2t923-en
- Eurostat. (2019). Tourism industries – Employment. Statistics explained. https://ec.europa.eu/eurostat/statisticsexplained/
- Esteban-Marquillas, J. M. (1972). I. A reinterpretation of shift-share analysis. Regional and Urban Economics, 2(3), 249–255. https://doi.org/https://doi.org/10.1016/0034-3331(72)90033-4
- Firgo, M., & Fritz, O. (2017). Does having the right visitor mix do the job? Applying an econometric shift-share model to regional tourism developments. The Annals of Regional Science, 58(3), 469–490. https://doi.org/https://doi.org/10.1007/s00168-016-0803-4
- Frenken, K., Van Oort, F., & Verburg, T. (2007). Related variety, unrelated variety and regional economic growth. Regional Studies, 41(5), 685–697. https://doi.org/https://doi.org/10.1080/00343400601120296
- Fuchs, M., Rijken, L., Peters, M., & Weiermair, K. (2000). Modelling Asian incoming tourism: A shift‐share approach. Asia Pacific Journal of Tourism Research, 5(2), 1–10. https://doi.org/https://doi.org/10.1080/10941660008722067
- Fuchs, V. R. (1959). Changes in the location of U.S. manufacturing since 1929. Journal of Regional Science, 1(2), 1–18. https://doi.org/https://doi.org/10.1111/j.1467-9787.1959.tb01455.x
- Fuchs, V. R. (1962). Statistical explanations of the relative shift of manufacturing among regions of the united States. Papers of the Regional Science Association, 8, 1–5.
- Getz, D., & Page, S. J. (2016). Progress and prospects for event tourism research. Tourism Management, 52, 593–631. https://doi.org/https://doi.org/10.1016/j.tourman.2015.03.007
- Goschin, Z. (2014). Regional growth in romania after its accession to EU: A shift-share analysis approach. Procedia Economics and Finance, 15, 169–175. https://doi.org/https://doi.org/10.1016/S2212-5671(14)00471-7
- Graham Shaw, D. J., & Spence, N. (1998). A productivity growth interpretation of the labour demand shift‐share model. Regional Studies, 32(6), 515–525. https://doi.org/https://doi.org/10.1080/00343409850119085
- Guaita-Martinez, J. M., Martin-Martin, J. M., & Salinas-Fernandez, J. A. (2019). Innovation in the measurement of tourism competitiveness, Chapter 13. In M. A. Galindo-Martín, M. T. Mendez-Picazo, M. S. Castaño-Martínez, (Eds.), Analyzing the relationship between innovation, value creation, and entrepreneurship (pp. 268–288). IGI Global.
- Harrison, D. (2015). Development theory and tourism in developing countries: what has theory ever done for us? International Journal of Asia Pacific Studies, 11(S1), 53–82.
- Herzog, H., & Olsen, R. (1977). Shift-share analysis revisited: The allocation effect and the stability of regional structure. Reg. Urban Stud. Sect. Energy Div. Oak Ridge Natl. Lab. Oak Ridge Tenn, 37830.
- Jacobs, J. (1961). The death and life of great American cities. Vintage Books A Division of Random House.
- Jacobs, J. (1969). The city. The economy of the cities. National Civic Review, 58(9), 447–448. https://doi.org/https://doi.org/10.1002/ncr.4100580916
- James Jr, F., & Hughes, J. (1973). A test of shift and share analysis as a predictive device. Journal of Regional Science, 13(2), 223–231.
- Jennings, G. R. (2006). Advances in tourism research: Theoretical paradigms and accountability. In Á. Matias, P. Nijkamp, & P. Neto (Eds.), Advances in modern tourism research (pp. 9–35). Physica-Verlag HD. https://doi.org/https://doi.org/10.1007/978-3-7908-1718-8_2
- Klaassen, L. H., & Paelinck, J. H. P. (1972). II. Asymmetry in shift- and share analysis. Regional and Urban Economics, 2(3), 256–261. https://doi.org/https://doi.org/10.1016/0034-3331(72)90034-6
- Knudsen, D. C., & Barff, R. (1991). Shift-share analysis as a linear model. Environment and Planning A: Economy and Space, 23(3), 421–431. https://doi.org/https://doi.org/10.1068/a230421
- Lailani. (2014). Shift share analysis for calculating regional competitive advantage.id blog. https://blog.id.com.au/2014/how-to/advanced-users-shift-share-analysis-for-calculating-regional-competitive-advantage/
- Li, Y., Hu, C., Huang, C., & Duan, L. (2017). The concept of smart tourism in the context of tourism information services. Tourism Management, 58, 293–300. https://doi.org/https://doi.org/10.1016/j.tourman.2016.03.014
- Liu, S. Y., & Lo, W. S. (2016). The low-carbon operations in ecotourism service supply chain management. Journal of Tourism and Hospitality Management, 4(4), 147–159.
- Loveridge, S. (1995). A practical approach to shift-share analysis. Community Development Society. Journal, 26(1), 110–124. https://doi.org/https://doi.org/10.1080/15575339509490166
- Moretti, E. (2004). Workers’ education, spillovers, and productivity: Evidence from plant-level production functions. American Economic Review, 94(3), 656–690. https://doi.org/https://doi.org/10.1257/0002828041464623
- Moretti, E. (2012). The new geography of jobs. Houghton Mifflin Harcourt.
- Muller, E., & Doloreux, D. (2007). The key dimensions of knowledge-intensive business services (KIBS) analysis: a decade of evolution (No. U1/2007), Working Papers “Firms and Region.” Fraunhofer Institute for Systems and Innovation Research (ISI).
- Munawir, R. (2014). Analysis of Bandung’s competitive sectors through LQ & Shift Share.
- O'Leary, E., & Webber, D. J. (2015). The Role of Structural Change in European Regional Productivity Growth. Regional Studies, 49(9), 1548–1560. https://doi.org/https://doi.org/10.1080/00343404.2013.839868
- Perles-Ribes, J. F., Ramón-Rodríguez, A. B., Rubia, A., & Moreno-Izquierdo, L. (2017). Is the tourism-led growth hypothesis valid after the global economic and financial crisis? The case of Spain 1957–2014. Tourism Management, 61, 96–109. https://doi.org/https://doi.org/10.1016/j.tourman.2017.01.003
- Prats, G. M., & Ramirez, A. A. (2018). Analysis of the behavior of a regional economy through the shift share and location quotient techniques. Management Dynamics in the Knowledge Economy, 6, 553–568.
- Ritchie, B., Sheehan, L. R., & Timur, S. (2008). Tourism sciences or tourism studies?Implications for the design and content of tourism programming. Teoros. Revue de Recherche En Tourisme, 27(1). https://journals.openedition.org/teoros/1621
- Salinas-Fernández, J. A., Serdeira Azevedo, P., Martín Martín, J. M., & Rodríguez Martín, J. A. (2020). Determinants of tourism destination competitiveness in the countries most visited by international tourists: Proposal of a synthetic index. Tourism Management Perspectives, 33, 100582. https://doi.org/https://doi.org/10.1016/j.tmp.2019.100582
- Selting, A. C., & Loveridge, S. (1992). A summary of the literature on shift-share analysis. Staff Paper Series, Department of Agricultural and Applied Economics Staff Paper, P92–13.
- Sentz, R. (2011). Understanding shift share. Emsi. https://www.economicmodeling.com/2011/12/05/understanding-shift-share-2/.
- Sharpley. (2009). Tourism development and the environment: Beyond sustainability? Earthscan.
- Shi, C., Zhang, J., Yang, Y., & Zhou, Z. (2007). Shift-share analysis on international tourism competitiveness—A case of Jiangsu Province. Chinese Geographical Science, 17(2), 173–178. https://doi.org/https://doi.org/10.1007/s11769-007-0173-2
- Stergiou, D. P., & Airey, D. (2018). Understandings of tourism theory. Tourism Review, 73(2), 156–168. https://doi.org/https://doi.org/10.1108/TR-07-2017-0120
- Stevens, B. H., & Moore, C. L. (1980). A critical review of the literature on shift-share as a forecasting technique. Journal of Regional Science, 20(4), 419–437.
- Traistaru, I., & Wolff, G. B. (2002). Regional specialization and employment dynamics in transition countries (No. B 18-2002). ZEI - Center for European Integration Studies.
- Tribe, J. (1997). The indiscipline of tourism. Annals of Tourism Research, 24(3), 638–657. https://doi.org/https://doi.org/10.1016/S0160-7383(97)00020-0
- Turner, R. (2018). Travel & tourism economic impact 2018. Romania.
- World Tourism Organization (UNWTO). (2018). European Union tourism trends. World Tourism Organization (UNWTO). https://doi.org/https://doi.org/10.18111/9789284419470
- World Travel and Tourism Council: World, Transformed. (2019). World, Transformed: Megatrends and Their Implications for Travel and Tourism, in cooperation with Bloomberg Media Group. Retrieved July, 2019, from https://wttc.org/Research/Insights.
- Xin, S. (2015). The theory and practice of conceptual research in tourism. 277. http://epubs.surrey.ac.uk/814163/1/revised%20thesis%20%28Shuang%20Xin%206100038%29.pdf
- Yasin, M., Alavi, J., Sobral, F., & Lisboa, J. (2004). A shift-share analysis approach to understanding the dynamic of the portuguese tourism market. Journal of Travel & Tourism Marketing, 17(4), 11–22. https://doi.org/https://doi.org/10.1300/J073v17n04_02
- Ye, B. H., Ye, H., & Law, R. (2020). Systematic review of smart tourism research. Sustainability, 12(8), 3401. https://doi.org/https://doi.org/10.3390/su12083401
- You, S., Chen, Y., Yang, T., & Huang, B. (2010). Spatial shift-share method: A new method in the study of regional industrial structures. In Zhu, R., Zhang, Y., Liu, B., Liu, C. (Eds.), Information computing and applications. Springer Berlin Heidelberg, Berlin, Heidelberg. pp. 507–514. https://doi.org/https://doi.org/10.1007/978-3-642-16339-5_67
- Zaman, G., Georgescu, G., Goschin, Z., Antonescu, D., & Popa, F. (2015). Dezvoltarea economică endogenă la nivel regionaL. Cazul României. Editura Expert. https://doi.org/https://doi.org/10.13140/rg.2.1.2731.4000
Annex 1. NUTS 2 regions with data in other years than the reference interval 2008 and 2016.
Annex 2. National Share of N79 employment EU countries profiles at NUTS 2 level.
Annex 3. Industrial Mix Share over N79 employment EU countries profiles at NUTS 2 level.
Annex 4. Regional Shift (Or Local Share)/Competitive Effect of N79 employment EU countries profiles at NUTS 2 level.