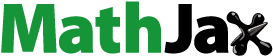
Abstract
COVID-19 pandemic has disrupted the economies around the world and the economic fallout from preventive measures such as lockdown is enormous. It has massive repercussions for the sharing economy as well. This research paper empirically analyses the impact of lockdown restrictions on five major sectors of the sharing economy such as ride-hailing, accommodation, freelance work, entertainment and delivery services. To evaluate this impact, we employed the difference-in-difference estimation technique using the Google trends data for selected countries. Daily search data of 2019 and 2020 is reindexed to examine the change in search patterns that occurred after lockdown. The empirical results show that transportation and accommodation sectors are negatively impacted by COVID-19 related lockdown while the other sectors of the sharing economy such as freelance work, streaming services and online deliveries are seeing a surge in searches. The findings of this study provide vital insights into the economic disruptions caused by COVID-19 related lockdown. We have highlighted the sectors that are booming during pandemic thus the sharing economy platforms and government have opportunities to invest in these sectors to jump-start the economy. The recently unemployed gig workers can also be employed in these sectors to address the problem of unemployment.
1. Introduction
COVID-19 is a novel Coronavirus that transfers from human to human and started spreading in late December 2019 in Wuhan, China. Within a month, the World Health Organization (WHO) declared COVID-19 a “public health emergency of international concern” (WHO, 2020b). On 11 March 2020, the WHO declared COVID-19 a global pandemic due to its worldwide spread. As of 22nd September 2020, there have been 3,11,32,906 confirmed cases of COVID-19, including 9,62,008 deaths (WHO, Citation2020a). China was the first country to place lockdown to contain the spread of coronavirus and that lockdown was proven successful in limiting the cases in China. Thus, other countries also followed suit and enforced lockdown. As most of the world is in lockdown, the demand for major sharing economy services such as Uber, Airbnb have plunged. We argue that the gig workers of these sharing economy platforms are facing massive income loss.
The sharing economy (SE) is a form of the new economic model that focuses on the peer-to-peer exchange of goods and services to increase the efficiency of underutilized resources. This model also goes by different terms such as platform markets, gig economy, collaborative consumption, etc. all these terms have some differences but the main essence is the same thus in this study all these terms are used interchangeably.
SE was growing at a fast pace before the pandemic. It was estimated that by 2025, the value of SE would be $335 billion in 2025 (Yaraghi & Ravi, Citation2017) and this forecast was on the track of becoming a reality. Moreover, Mehdiabadi et al. (Citation2020) suggested that currently, FinTech is seen as a good platform for implementing ideas based on the sharing economy and crowdfunding. But unfortunately, the COVID-19 outbreak happened and shattered the entire economic system. Some experts are speculating that this pandemic can end the SE (Conger & Griffith, Citation2020).
Sharing services related to hospitality and transportation are getting hammered due to travel restrictions. Due to the worldwide travel restrictions, Airbnb is suffering the most as its revenue has decreased by up to 50%. Rental booking data shows that Beijing has seen a 96% decrease in Airbnb booking from January to March 2020 (DuBois, Citation2020). Uber is the most used service of SE yet it is also facing a massive decline in demand for its services. Lyft and other ride-hailing applications are also facing the same downward spiral in the rides and revenues (O’Brien, Citation2020).
However, the COVID-19 pandemic has created some new opportunities for SE. As several countries are in lockdown so the use of online shopping and food delivery services has increased (Koetsier, Citation2020). The closure of offices has increased the demand for freelance work. Due to the closing of cinemas, video streaming services such as Netflix, Disney+, and Amazon Prime have become the key source of entertainment. All streaming services are seeing a gigantic increase in subscriptions and usage (Epstein, Citation2020). Thus, the conjecture that this pandemic will end the SE is baseless because the effects of COVID-19 related lockdown are not the same for all the sectors of SE.
In this study, we aim to investigate the changes that occurred in the search trends of SE services dueto the COVID-19. This is analyzed by collecting the Google trends data on 10 search terms across five sectors of SE. Our study also aims to assist the policymakers and platforms in identifying the potential opportunities created by COVID-19. This research paper fills the gap in the existing literature by empirically analysing the financial turmoil caused by this outbreakin different sectors of SE.
The rest of the paper is organized as follows. Section 2 discusses the literature review, while section 3 deals with the theoretical framework. Data and methodology are explained in section 4 and the results are explained in the 5th section. Conclusion and policy recommendations are in the last section.
2. Literature review
Sharing economy has grown exponentially in the past few years because it allows users to utilize their idle resources to earn additional income and make new social connections (Botsman & Rogers, Citation2010). This growth of SE can also be attributed to the popularity of sustainable growth. Spulbar et al. (Citation2019) suggested that sustainable development represents a great challenge for the global economy. Therefore, the sharing platforms claim to be the pathway to sustainability (Heinrichs, Citation2013). On the other hand, the governance quality should be strengthen that set the direction of change to achieve greater financial stability and growth (Zulfiqar et al., Citation2020). However, COVID-19 has exposed the risks involved in sharing with strangers and the social connections that used to be the driving force of SE have become the reason behind the lower demand for SE services. COVID-19 related lockdown has influenced the performance of all the sectors of the economy.
Many researchers are trying to check the impact of the pandemic on different sectors of the economy. According to Abiad et al. (Citation2020), even a contained outbreak of this magnitude will cost $156 billion to the global economy, most of which will be bear by the consumption and tourism sectors. Abodunrin et al. (Citation2020) critically examine the impact of COVID-19 on the global economy. They explain that the pandemic has tormented all sectors and suggest measures such as tax relief and work allowance for the recovery of different sectors. Another study by Hoque et al. (Citation2020) measures the impact of COVID-19 on the tourism industry in the case of China. They find that travel restrictions that have been placed to control the spread of Coronavirus from China to other countries impacted its tourism industry to a great extent. This pandemic has ramifications on the gig economy as well and has left the gig economy workers more vulnerable as they have inadequate social protection (Chohan, Citation2020). This leaves millions of gig economy workers unprotected during one of the biggest economic crises.
However, this is not the first time that a pandemic gloomed the global economy. Recent pandemics like SARS and MERS also bought economic misery for the effected nations (Keogh-Brown & Smith, Citation2008; Siu & Wong, Citation2004). The Spanish flu pandemic of 1918 is one of the deadliest pandemics in the recorded human history, it infected over 500 million people worldwide (Taubenberger and Morens, 2006). Karlsson et al. (Citation2014) explore the impact of this Spanish flu pandemic on the economic performance of Sweden. According to them, this pandemic has caused an increase in poverty and unemployment.
Beutels et al. (Citation2009) investigate the impact of the SARS outbreak on different economic and social indicators in Beijing using the cross-correlation function. This study concludes that leisure activities, transportation and tourism sectors suffered the most. Some other studies such as Chen et al. (Citation2007) and Mao et al. (Citation2010) conclude that the growth and tourism industries affected the most due to the SARS outbreak. According to the study of Chou et al. (Citation2004), the hotel occupancy rate also plunged by almost 50% in Taiwan. Thus, it can be seen that pandemics have always greatly affected the tourism and related industries and the recovery of Airbnb and Couchsurfing will take time.
A detailed review of the literature shows that this study fills the knowledge gap in many ways. It extends the literature by empirically exploring the impact of COVID-19 on SE. To the best of researcher’s knowledge, no study has used Google trends data to empirically analyse the effect of COVID-19 related lockdown on SE services. This study will help future researchers in understanding the economic disruptions caused by COVID-19 and lockdown.
3. Data and research methodology
One-third of the world’s population is under lockdown currently as the World Health Organization (WHO) advised social distancing as the safest measures to prevent the spread of the coronavirus. The world is changing and SE is also experiencing a change from this pandemic. To analyse the impact of lockdown on the use of SE service, we use Google trends data. It is a new source of big data. Literature shows that Google trends data is effective in predicting the tourism decision, financial market fluctuations, and even disease outbreak (see for details: Carneiro & Mylonakis,Citation2009; Önder, 2017).
3.1. Google trends data
Google Trends is a search trend feature that shows how frequently a given search item is entered into Google’s search engine relative to the site’s total search volume over a given period. Google Trends provides an index of the relative volume of search requests performed through Google. The index values range from 0 to 100 where 100 represents the day when the highest daily searches were done during the selected time period and 0 represents the day when no or insufficient searches on that search term were performed. It is a vital source of high-frequency data because it provides data on a hourly, daily, weekly, and monthly basis. Due to the increasing popularity of internet usage, most of the people certainly use web search engines to collect relevant information for goods they aim to buy. In this study, we use Google Trends data to show the changing demands for various sharing economy services after the COVID-19 outbreak.
For our analysis, we used a total of 10 search terms of different services that belong to 5 sectors of SE. The detail of services included can be seen in the table below:
Data on these search terms were collected for 9 countries, namely, Australia, Brazil, France, Germany, India, Italy, Mexico, Spain, and United Kingdom. These countries are selected because they have high users of sharing economy and enforced a nation-wide lockdown.
For the analysis, we used daily search data of the above terms from 1st January 2019 to 10th May 2019 and compare it with the data of the same dates in 2020. This time period is used because the COVID-19 started spreading globally from January 2020 and the countries started enforcing lockdown. Since May the lockdown restrictions started to relax in several countries including in the model, therefore, the data till the 10th of May 2020 is used only.
However, this daily data is not directly comparable because both data files come from a different search query and the data is not in absolute terms rather it is in a 0 to 100 index. Therefore, directly comparing the files can result in incorrect estimation because a daily value of 50 in 2020 might represent lower daily searches than a value of 60 in 2019 as their denominator (maximum searches) is different.Another issue is that daily Google trends data is not available for a period longer than 9 months. To tackle this, we downloaded the available weekly data from 1st January 2019 to 10th May 2020 and followed the rescaling technique used by Brodeur et al. (Citation2020).
3.2. Data re-scaling
Re-scaling the daily data by respective weekly data can be done in the following steps:
Denote the daily data of 2019 as Di,c2019and calculate the weekly average of 2019:
Denote the daily data of 2020 as Di,c2020 and calculate the weekly average of 2020:
From the weekly data, separate the data of desired weeks i.e. from 1st January to 10th May for 2019 and 2020:
Calculate the weights for 2019 and 2020 using the following formulas:
Multiply the daily data of 2019 with the corresponding weekly weight from weightc,2019, do the same for 2020 to get the rescaled data:
Normalize the rescaled data by dividing the daily values by the maximum value of Di,c2019-2020and multiply by 100. It will bring the daily data under the range of between 0 to 100 which is the original format of Google Trends data.
3.3. Sample selection
We selected those countries that enforced nation-wide lockdown during our selected period because lockdown is the treatment in our difference-in-difference estimation. The list of countries included in the model and the lockdown dates can be seen in the :
Some countries such as China and Russia were excluded from the model because they either do not have the services included in the model or their number of users is very low.
3.4. Theoretical framework
Sharing has always been a part of society though it used to be limited to close relatives and neighbours. However, the development of Information Communication Technology (ICT) has helped in creating an environment of trust by providing users and service providers with necessary information about other party. For example, the two-way rating system of Uber makes the driver and customer create a positive experience for each other in order to receive a positive rating, as it is described in the social exchange theory. Social exchange theory (SET) has been used widely in the literature to study social interactions. This theory states that people do a cost and benefit analysis when interacting with each other and they prefer the relationship that maximizes rewards and minimises the cost (Boateng et al., Citation2019; Emerson, Citation1976). This theory effectively addressed the change in the use of SE services during COVID-19.
The lockdown has increased the cost of sharing vehicle and house due to the possibility of contracting virus therefore the use of SE in these sectors have reduced. However, getting food delivered and online shopping, watching Netflix instead of going to the theatres have become more beneficial as they allow consumers to safely enjoy the services. Freelance work is also becoming more popular because working from home minimized the risk of contracting the infection.
From SET, it can be argued that lockdown has made some services more convenient and some riskier. Therefore, we see a difference in the demand for SE in different sectors.
3.5. Methodology
The difference-in-difference (DID) estimator is one of the most popular tools in applied research for checking the impact of policy intervention on economic variables. Many researchers are using this technique for understanding the effect of COVID-19 on a variety of variables (Brodeur et al., Citation2020; Chen et al., Citation2020; Lyu & Wehby, Citation2020).
A key assumption of DID is the parallel trend assumption that means in the absence of intervention, the control and treatment group would have followed parallel paths (Abadie, Citation2005). Therefore, DID is used in this study because in the absence of COVID-19 and lockdowns the SE would have followed the same trend as in 2019.
In this research study, we want to check the impact of COVID-19 related lockdown on the use of SE services. We do so by comparing the pre- and post-lockdown daily searches in 2020 with daily searches on the same dates of 2019 and the use of DiD helps us in controlling for the seasonal and country-specific shocks.
Following Brodeur et al. (Citation2020), we formed the following model:
Where, Di,cis the daily searches for a service of SE. Lockdowni,c is the dummy variable that takes the value of 0 in the dates when no lockdown was enforced and 1 from the date of lockdown implementation and afterward. Yeari is also a dummy variable that takes the value of 1 in 2020 and 0 in 2019. DPMi-1,c represents the lagged value of new Covid-19 related deaths per million.
4. Empirical results
4.1. Difference-in-difference estimation results
shows difference-in-difference estimates of lockdown effects for five main services sectors of SE.
Table 3. Results of difference-in- difference estimation.
The worldwide travel restrictions that have been put to minimize the spread of coronavirus have knocked down the performance of ride-hailing and accommodation services of SE. Our results also indicate the negative and significant impact of lockdown on the search intensity for both ride-hailing (Uber and Lyft) and accommodation (Airbnb and Couchsurfing) services. There is a significant decrease in the search volume for Uber (b = −22.79, p <.05) and Lyft (b = −14.11, p <.01). But the effect size of lockdown for Uber is large than Lyft as evident by the standard deviation. This decrease in demand means that millions of driver-partners of ride-hailing applications have become unemployed and they require financial support to weather the storm. Platforms should hire these workers for delivery services as the demand for grocery and food delivery has peaked.
The same is the case of accommodation services, from we also see obvious drops in the search intensity for both Airbnb (b = −43.36, p <.01) and Couchsurfing (b = −16.89, p <.01). A recent study by (Abodunrin et al., Citation2020) has also indicated the similar consequences of COVID-19 on the travel industry. Dolnicar and Zare (Citation2020) fear that this pandemic may have a long-term consequence on the growth of Airbnb. However, Airbnb has set up a $250 million fund to mitigate the financial losses incurred by hosts due to booking cancellations (Airbnb, Citation2020).
Table 1. Summary of the detail of services.
Table 2. The lockdown dates.
The stay-at-home orders have a positive impact on freelance, entertainment and online delivery services. The closure of workplaces has resulted in outsourcing the work to freelancers. Many unemployed workers also searching for gigs in freelance marketplaces that caused an increase in daily searches for freelance services. This research study also reports that lockdown has a significant and positive effect on Upwork (b = 10.20, p <.05) and Fiverr (b = 28.17, p <.01).
From , we observe that Stay at home orders are associated with a significant increase in searches for entertainment services. The lockdown has a positive effect on the search intensity for both Netflix (b = 25.69, p <.01) and Amazon (b = 37.86, p <.01), but the effect size is large for Amazon searches. This positive impact of lockdown is also evident by the increased revenue and subscribers of streaming services reported by Epstein (Citation2020).
The public is currently relying on gig workers for supplying the medication and groceries thus pandemic has increased the demand for gig workers. Recently unemployed gig workers in other fields can find employment opportunities in the delivery sector. Our empirical results also confirm the positive and significant effect of lockdown on the search intensity for the search term Food Delivery (b = 8.63, p <.10) however there has not been a significant change in the searches for the online shopping.
4.2. Robustness check
We have checked the stability of our model by different robustness checks. The robustness is checked by using the lockdown announcement date instead of the implementation date, the first case reported date, first death reported date and by splitting the models into early and late lockdown countries. The results are stable for these robustness checks. The following reports the result of DID using the first death reported date as the treatment instead of the lockdown enforcement date.
Table 4. DID estimation robustness check.
4.3. Difference-in-difference estimation: graphical analysis
We compare the raw data of pre and post lockdown searches in 2020 to the pre and post lockdown searches the same dates in 2019. We use the data of raw averages for all the search topics included in our study. These average searches range from 0 to 100. We set the day at which lockdown was announced equal to zero in 2020. The red dots show the average searches before and after the lockdown in 2020 and the grey dots are representing the average searches before and after the stay at home orders in 2019.
plots the daily search activity for the services included in the model. It is evident that before the lockdown, the average searches for Uber and Lyft are following a parallel trend in 2019 and 2020. However, after the lockdown, the average searches for both platforms decreased abruptly in 2020 (red dots). In the case of Airbnb and Couchsurfing, the decline is evident even before lockdown due to travel restrictions that were placed before lockdown. Graphs of Netflix and Amazon Prime start showing the upward trend before the nation-wide lockdown and that trend is constant even 50 days after the lockdown. In the case of freelance markets, the searches of Fiverr show a greater increase in 2020 as compared to Upwork. Lastly, the searches for online shopping and food delivery can be seen sky rocketing during the first week of lockdown but that the trend begins to come back to the 2019 position.
5. Conclusions and policy recommendation
Pandemics are known to bring economic misery and the current pandemic is no different in this respect. Countries are compelled to place lockdowns due to the high infection rate of COVID-19 despite the enormous economic cost associated with it. These preventive measures have impinged all sectors of SE, it has doubled the use of some services and slashed the demand of other sectors. In this study, we have empirically analyzed the gains and losses faced by SE due to months-long lockdown. DID estimation shows a massive decline in the transportation and accommodation sector while an upsurge is observed in the platforms associated with freelance, delivery and entertainment sectors.
Transportation and accommodation are two of the biggest sectors of SE in terms of daily users and revenue and these two are most adversely affected by lockdown restrictions. This situation has left millions of workers of these sectors out of jobs and they are not even entitled to basic labour protections because they are considered independent contractors instead of workers. Based on these findings, we suggest that all platforms should compensate their service providers for the losses incurred by them during the lockdown. Governments should also include gig workers in their relief programs and provide them unemployment benefits. Governments and platforms should work together to restart tourism and to ensure the safety of users and service providers.
Countries should fully utilize the rapid digitization that occurred due to COVID-19 related lockdown and should invest more in these sectors of SE to boost their economies and create jobs for recently unemployed workers. As Buheji and Ahmed (Citation2020) states that the enhancement of the sharing economy can be one of the hidden economic opportunity of COVID-19. Thus, it is safe to say that SE will thrive after these gloomy times.
Disclosure statement
No potential conflict of interest was reported by the authors.
Correction Statement
This article has been republished with minor changes. These changes do not impact the academic content of the article.
References
- Abadie, A. (2005). Semiparametric difference-in-differences estimators. The Review of Economic Studies, 72(1), 1–19. https://doi.org/https://doi.org/10.1111/0034-6527.00321
- Abiad, A., Arao, R. M., & Dagli, S. (2020). The economic impact of the COVID-19 outbreak on developing Asia. Asian Development Bank, 128, 1–14. https://doi.org/https://doi.org/10.22617/BRF200096
- Abodunrin, O., Oloye, G., & Adesola, B. (2020). Coronavirus Pandemic and its implication on global economy. International Journal of Arts, Languages and Business Studies (IJALBS), 4, 13–23.
- Airbnb. (2020). $250M to support hosts impacted by cancellations - Resource Center. https://www.airbnb.com/resources/hosting-homes/a/250m-to-support-hosts-impacted-by-cancellations-165
- Beutels, P., Jia, N., Zhou, Q. Y., Smith, R., Cao, W. C., & De Vlas, S. J. (2009). The economic impact of SARS in Beijing. Tropical Medicine & International Health, 14(SUPPL. 1), 85–91. https://doi.org/https://doi.org/10.1111/j.1365-3156.2008.02210.x
- Boateng, H., Kosiba, J. P. B., & Okoe, A. F. (2019). Determinants of consumers’ participation in the sharing economy: A social exchange perspective within an emerging economy context. International Journal of Contemporary Hospitality Management, 31(2), 718–733. https://doi.org/https://doi.org/10.1108/IJCHM-11-2017-0731
- Botsman, R., & Rogers, R. (2010). How collaborative consumption is changing the way we live (pp. 1–304). New York, NY: Harper Collins Publishers.
- Brodeur, A., Clark, A. E., Fleche, S., & Powdthavee, N. (2020). Assessing the impact of the coronavirus lockdown on unhappiness, loneliness, and boredom using Google Trends. arXiv preprint arXiv:2004.12129.
- Buheji, M., & Ahmed, D. (2020). Foresight of Coronavirus (COVID-19) opportunities for a better world. American Journal of Economics, 10(2), 97–108. https://doi.org/https://doi.org/10.5923/j.economics.20201002.05
- Carneiro, H. A., & Mylonakis, E. (2009). Google trends: A web-based tool for real-time surveillance of disease outbreaks. Clinical Infectious Diseases: An Official Publication of the Infectious Diseases Society of America, 49(10), 1557–1564. https://doi.org/https://doi.org/10.1086/630200
- Chen, M. H., Jang, S. S., & Kim, W. G. (2007). The impact of the SARS outbreak on Taiwanese hotel stock performance: an event-study approach. International Journal of Hospitality Management, 26(1), 200–212. https://doi.org/https://doi.org/10.1016/j.ijhm.2005.11.004
- Chen, H., Qian, W., & Wen, Q. (2020). The impact of the COVID-19 pandemic on consumption: Learning from high frequency transaction data. Available at SSRN 3568574.
- Chohan, U. W. (2020). A post-coronavirus world: 7 points of discussion for a new political economy. SSRN Electronic Journal. https://doi.org/https://doi.org/10.2139/ssrn.3557738
- Chou, J., Kuo, N.-F., & Peng, S.-L. (2004). Potential impacts of the SARS outbreak on Taiwan’s. Asian Economic Papers, 3(1), 84–99. https://doi.org/https://doi.org/10.1162/1535351041747969
- Conger, K., Griffith, E. (2020). The results are in for the sharing economy. They are ugly. The New York Times. https://www.nytimes.com/2020/05/07/technology/the-results-are-in-for-the-sharing-economy-they-are-ugly.html
- Dolnicar, S., & Zare, S. (2020). Coronavirus and Airbnb – Disrupting the Disruptor. March, 18. https://doi.org/https://doi.org/10.31235/osf.io/t9n6q
- DuBois, D. (2020). Impact of the coronavirus on global short-term rental markets - AirDNA - Short-term vacation rental data and analytics. https://www.airdna.co/blog/coronavirus-impact-on-global-short-term-rental-markets
- Emerson, R. M. (1976). Social exchange theory. Annual Review of Sociology, 2(1), 335–362. https://doi.org/https://doi.org/10.1146/annurev.so.02.080176.002003
- Epstein, A. (2020). Netflix had its best quarter ever because of coronavirus — Quartz. https://qz.com/18,42,471/netflix-had-its-best-quarter-ever-because-of-coronavirus/
- Heinrichs, H. (2013). Sharing economy: a potential new pathway to sustainability. Gaia - Ecological Perspectives for Science and Society, 22(4), 228–231. https://doi.org/https://doi.org/10.14512/gaia.22.4.5
- Hoque, A., Shikha, F. A., Hasanat, M. W., Arif, I., & Hamid, A. B. A. (2020). The Effect of Coronavirus (COVID-19) in the tourism industry. Asian Journal of Multidisciplinary Studies, 3(1), 52–58.
- Karlsson, M., Nilsson, T., & Pichler, S. (2014). The impact of the 1918 Spanish flu epidemic on economic performance in Sweden: An investigation into the consequences of an extraordinary mortality shock. Journal of Health Economics, 36(1), 1–19. https://doi.org/https://doi.org/10.1016/j.jhealeco.2014.03.005
- Keogh-Brown, M. R., & Smith, R. D. (2008). The economic impact of SARS: How does the reality match the predictions? Health Policy (Amsterdam, Netherlands)), 88(1), 110–120. https://doi.org/https://doi.org/10.1016/j.healthpol.2008.03.003
- Koetsier, J. (2020). COVID-19 app economy: Instacart, Walmart, Costco Way Up, Uber and Lyft drop like rocks. https://www.forbes.com/sites/johnkoetsier/2020/03/24/covid-19-app-economy-instacart-walmart-costco-way-up-uber-and-lyft-drop-like-rocks/#234234f5551c
- Lyu, W., & Wehby, G. L. (2020). Comparison of estimated rates of coronavirus disease 2019 (COVID-19) in border counties in Iowa without a stay-at-home order and border counties in Illinois With a stay-at-home order. JAMA Network Open, 3(5), e2011102-e2011102. https://doi.org/https://doi.org/10.1001/jamanetworkopen.2020.11102
- Mao, C. K., Ding, C. G., & Lee, H. Y. (2010). Post-SARS tourist arrival recovery patterns: An analysis based on a catastrophe theory. Tourism Management, 31(6), 855–861. https://doi.org/https://doi.org/10.1016/j.tourman.2009.09.003
- Mehdiabadi, A., Tabatabeinasab, M., Spulbar, C., Karbassi Yazdi, A., & Birau, R. (2020). Are we ready for the challenge of banks 4.0? Designing a roadmap for banking systems in industry 4.0. International Journal of Financial Studies, 8(2), 32. https://doi.org/https://doi.org/10.3390/ijfs8020032
- O’Brien, S. A. (2020). The ride hailing industry is getting turned on its head by coronavirus - CNN. https://edition.cnn.com/2020/03/27/tech/drivers-delivery-workers/index.html
- Önder, I. (2017). Forecasting tourism demand with Google trends: Accuracy comparison of countries versus cities. International Journal of Tourism Research, 19(6), 648–660. https://doi.org/https://doi.org/10.1002/jtr.2137
- Spulbar, C., Ejaz, A., Birau, R., & Trivedi, J. (2019). Sustainable investing based on momentum strategies in emerging stock markets: A case study for Bombay Stock Exchange (BSE) of India. Scientific Annals of Economics and Business, 66(3), 351–311. https://doi.org/https://doi.org/10.2478/saeb-2019-0029
- Siu, A., & Wong, Y. C. R. (2004). Economic impact of SARS: The case of Hong Kong. Asian Economic Papers, 3(1), 62–83. https://doi.org/https://doi.org/10.1162/1535351041747996
- Taubenberger, J. K., & Morens, D. M. (2006). 1918 Influenza: the mother of all pandemics. Revista Biomedica, 17(1), 69–79.
- Yaraghi, N., & Ravi, S. (2017). The current and future state of the sharing economy. Brookings India IMPACT Series No. 032017, 1-38. https://doi.org/https://doi.org/10.2139/ssrn.3041207
- WHO. (2020a). Coronavirus disease (COVID-19) pandemic. World Health Organization https://www.who.int/emergencies/diseases/novel-coronavirus-2019
- WHO. (2020b). Statement on the second meeting of the International Health Regulations (2005) Emergency Committee regarding the outbreak of novel coronavirus (2019-nCoV). Geneva, Switzerland: World Health Organization. https://www.who.int/news-room/detail/30-01-2020-statement-on-the-second-meeting-of-the-international-health-regulations-(2005)emergency-committee-regarding-the-outbreak-of-novel-coronavirus-(2019-ncov)
- Zulfiqar, A. I., Ejaz, A., Spulbar, C., Birau, R., & Nethravathi, P. S. R. (2020). Measuring the impact of governance quality on stock market performance in developed countries. Economic Research-Ekonomska Istraživanja, 33(1), 3406–3426. https://doi.org/https://doi.org/10.1080/1331677X.2020.1774789