Abstract
Nowadays, the role of the sharing economy in tourism increases, the number of people involved as guests or hosts rising day by day. This dynamic generates a viable alternative to the traditional services, allowing tourists to customise their trips and enrich their experiences. This paper focuses on accommodation services, investigating the factors influencing the prices established by Airbnb hosts. Using structural equation modelling, the authors analyse the influence of different categories of factors (listing’s characteristics, hosts’ involvement, listing’s reputation, listing’s location, and rental policies) on the average daily rate. The results emphasise that the hosts establish the listing’s price based on listing’s characteristics and on their involvement – the owners managing only one listing and the ones charging a security deposit value more their involvement, while the other hosts focus more on the listings’ characteristics. The location of the listing counts for experienced owners, while for opportunist owners it has no importance. The listings’ reputation has a negative impact on the price, contrary to the conclusions achieved in other studies, an aspect that supports the idea that price determinants differ across regions.
1. Introduction
Currently, the new socio-economic system of sharing economy represents a habitual element in the market, recognised and embraced by an increasing number of individuals. It has emerged due to ICT innovations in different areas like transportation, accommodation, food, and skills (Tussyadiah & Pesonen, Citation2016). The higher level of spread encountered in the hospitality industry led to the development of platforms like Airbnb, 9Flats, Expedia, Fairbnb. Among these, Airbnb, a pioneer in peer-to-peer accommodation, represents the most important player at the global level with a substantial influence in the market.
According to a recent report, Airbnb guests can book from more than 6 million rooms, flats, and houses, in around 81.000 cities all over the world. There are more than 150 million hosts enrolled in the system which provided accommodation services in 2018 for 260 million guests (Sherwood, Citation2019). Airbnb successfully provided a lot of competitive advantages and financial resources for launching it into the hotel business. Thus, for 2020, through a partnership with an accommodation developer to convert some commercial proprieties in New York into high-end apartment-style suites, Airbnb will enter the lodging market beside traditional players. The phenomenon of Airbnb development has changed the traveller’s consumption patterns, on one hand, and the structure of the lodging sector, on the other hand (Wang et al., Citation2019). For example, due to the important presence of Airbnb supply in some areas, the hotels had to respond by lowering their prices (Zervas et al., Citation2017), reducing in this way their profitability. Consumers benefited from this strategy, even those who were not customers for the Airbnb platform, and much more from the growth of goods and services diversity provided through peer-to-peer platforms. After the economic recession, the impact was enhanced also by the fact that people paid more attention to their spending and tried to use more opportunities for preserving their resources (Guttentag et al., Citation2018).
The boost of share economy system popularity attracted the attention of practitioners and academics, thus in the literature exist a lot of studies (Hamari et al., Citation2016; Sigala, Citation2017) which try to explain the motives of people to share and provide services (especially accommodation) through commercial sharing platforms, the way they establish the prices, the influence upon the consumer behaviour, and the impact on travel and tourism industry. Focussing on pricing strategies, there are still aspects that have to be explored, like: what are the differences in owners’ strategies, what is the impact of their goals, what are the criteria used to establish the price, or why the impact of factors differs from region to region.
Using structural equation modelling, the purpose of the present study is to develop a formative model that enables the identification of factors that influence Airbnb listing prices. This paper aims to enrich the literature by concentrating the analysis on hosts’ price setting behaviour. Starting from the characteristics of the different categories of owners, the paper will explore the impact of these differences on price determinants, this approach representing a new contribution to the literature. Moreover, the authors propose and test the role of a new variable, host involvement, which upgrades the host’s attributes by including the behaviour the owners have in relation to their guests and the Airbnb platform.
To achieve the paper’s goals, a multigroup analysis is implemented based on the following four criteria: the owners’ reasons to share their homes, the number of listings, the requirement of a security deposit, and the existence of the superhost status. The official data for 881 Airbnb listings in the city of Cluj-Napoca (Romania), during the time span of April 2017 – March 2018, were used to apply the model and to implement the analyses.
The remainder of this paper is organised as follows: section two presents the literature, section three describes the model proposed, section four presents the variables used and the methodology applied to collect and process the data, section five discusses the results. The last section is dedicated to conclusions.
2. Theoretical framework
The Airbnb platform differs from traditional accommodation units in terms of facilities, customer service, website design, and booking systems. The lodging model specific for Airbnb favours a closer social interaction between residents and tourists (Tussyadiah & Pesonen, Citation2016), to create a sense of place as a competitive advantage compared with conventional lodging proprietaries (Cheng, Citation2016). In addition, the literature suggests that this social appeal of peer-to-peer accommodation contributes to several outcomes like a longer length of stay, higher frequency of travel, and a bigger range of activities. On the other hand, Farmaki and Stergiou (Citation2019) highlighted that among the hosts’ motives to share their homes, social interactions with guests are equally important as economic reasons. Furthermore, accordingly with the need for intensity of social interaction, different types of behaviour were illustrated (Farmaki & Stergiou, Citation2019).
Many studies have focussed on Airbnb’s advantages, threats (Fang et al., Citation2016; Lampinen & Cheshire, Citation2016; Meleo et al., Citation2016), legal issues (Edelman & Geradin, Citation2015), impacts on the hotel industry and tourism industry employment (Fang et al., Citation2016; Neeser et al., Citation2015; Zervas et al., Citation2017) or revenues (Frenken & Schor, Citation2017; Gibbs, Guttentag, Gretzel, Yao, et al., Citation2018; Gunter & Onder, Citation2018; Zhang et al., Citation2018). For instance, Guttentag (Citation2015) emphasised that the strengths of Airbnb are related to lower prices and the socio-cultural benefits resulted from staying in a local residence. On the other side, the lack of legislation to regulate the activity and the safety issues may represent possible threats to Airbnb’s future growth. The regulatory framework is mandatory to allow peer-to-peer platforms to operate legally and to allow all the stakeholders to enjoy the advantages created by these platforms. Zervas et al. (Citation2017) reported that a 1% increase in Airbnb listings in Texas resulted in a 0.05% decrease in quarterly hotel revenues. This result supports the idea that the Airbnb development may impact negatively the financial results of traditional accommodation units. Fang et al. examined the effect of Airbnb on the tourism industry in Idaho, USA. Their results emphasise that the sharing economy can generate employment, especially in small to medium markets, the additional impact being less significant as the market grows (Fang et al., Citation2016).
The exponential growth of the Airbnb platform raised the interest in studies trying to highlight the price determinants of Airbnb listings (Wang & Nicolau, Citation2017). Since the price influences clients’ choice of accommodation and hosts’ profits, identifying the factors determining the price can help hosts establish an equitable price so that both the owners and guests benefit from being part of the sharing economy.
Until now, different studies have been focussed on price determinants of Airbnb listings, their results emphasising the complexity of the relationship between pricing and its determinants. Moreover, different authors suggest that the relationship between price and its determinants differ undoubtedly across cities/countries/regions due to the variation in city types, city economics, and Airbnb development in the region (Gibbs, Guttentag, Gretzel, Morton, et al., Citation2018; Lorde et al., Citation2019).
For instance, racial discrimination creates an unfortunate impact on prices, the Afro-American, Asian and Hispanic owners being obliged to charge lower prices for similar listings than white hosts (Edelman & Luca, Citation2014; Kakar et al., Citation2016). Gutt and Herrmann (Citation2015) identify that once the minimum number of reviews is obtained and the rating becomes visible, the price increase by €2.69. Moreover, higher ratings determine hosts to charge higher prices.
Prices are also correlated with reputation and trustworthiness. Ikkala and Lampinen (Citation2014), Gutt and Herrmann (Citation2015), Kakar et al. (Citation2016), Brochado et al. (Citation2017), and Mody et al. (Citation2017) emphasise the impact of different components of reputation on prices, most of the elements having a positive effect. Ert et al. (Citation2016) highlighted that visual-based trustworthiness, created through the users’ photographs in the case of Airbnb listings in Stockholm, determines the listing prices. Li et al. (Citation2016) and Zhang et al. (Citation2017) reported that the distance to the nearest landmark and facility is correlated with Airbnb listing prices. Dudás et al. (Citation2017) underline that, in the case of Budapest, distance to the city centre is a weak price determinant.
Summarizing the findings from previous studies on Airbnb pricing, five groups of variables were identified:
Listing/home attributes include the following variables:
Accommodation type and room type, number of bedrooms, number of bathrooms, facilities (car parking, swimming pool, and wireless Internet) have a positive effect on prices (Chen & Xie, Citation2017; Gibbs, Guttentag, Gretzel, Morton, et al., Citation2018; Wang & Nicolau, Citation2017);
The number of accommodation photos has a positive effect on prices (Ert et al., Citation2016; Gibbs, Guttentag, Gretzel, Morton, et al., Citation2018);
Free breakfast has a negative impact on prices (Gibbs, Guttentag, Gretzel, Morton, et al., Citation2018; Wang & Nicolau, Citation2017).
Listing location includes the following variables:
Neighbourhood average rental price, number of other Airbnb listings, price of similar Airbnb listings or price of hotels, availability of sightseeing, eating or shopping areas, or other attractions have a positive effect on prices (Chen & Xie, Citation2017; Kakar et al., Citation2016; Li et al., Citation2016);
The distance to the city centre has different effects on prices, ranging from weak (Dudás et al., Citation2017) to a significant negative effect (Li et al., Citation2016).
Listing reputation includes the following variables:
Ratings on cleanliness and location have a positive effect on prices (Chen & Xie, Citation2017; Kakar et al., Citation2016);
The reviews’ number and scores have a negative effect on prices (Gibbs, Guttentag, Gretzel, Morton, et al., Citation2018; Kakar et al., Citation2016; Wang & Nicolau, Citation2017). A higher number of reviews is the result of a higher number of bookings, which is, usually, a characteristic of the cheaper listings;
Average review score has a positive effect on prices (Gutt & Herrmann, Citation2015; Ikkala & Lampinen, Citation2014);
Ratings on accuracy and check-in do not have a significant effect on prices (Chen & Xie, Citation2017).
Host attributes include the following variables:
Hosts’ listing count, host verification, host profile picture, and response time have a positive impact on the price (Chen & Xie, Citation2017; Wang & Nicolau, Citation2017);
Race has a negative effect on prices (Edelman & Geradin, Citation2015; Kakar et al., Citation2016);
Gender, marital status, and sexual orientation did not have a significant effect on price (Chen & Xie, Citation2017; Kakar et al., Citation2016);
Superhost status has mixed effects on prices (Chen & Xie, Citation2017; Gibbs et al., 2018; Kakar et al. Citation2016, Liang et al., Citation2017; Wang & Nicolau, Citation2017).
Rental policies describe the Airbnb policies the owner may implement:
Strict cancellation policy and guests’ phone verification required has a positive effect on prices (Chen & Xie, Citation2017; Wang & Nicolau, Citation2017);
Smoking allowed has a negative effect on prices (Gibbs, Guttentag, Gretzel, Morton, et al., Citation2018; Wang & Nicolau, Citation2017).
Based on these conclusions, the following hypotheses will be tested:
H1: Better listing characteristics will determine the hosts to charge a higher price.
H2: A farther listing location from the city centre will determine the hosts to charge a lower price.
H3: A better listing reputation will determine the hosts to charge a higher price.
H4: Hosts willing to involve more in managing Airbnb listings charge a higher price.
H5: The stricter the rental policies, the higher will be the price charged.
3. The model
The formative model developed to investigate the factors influencing the hosts' behaviour in setting rental prices has the average daily rate (measured in USD) as a dependent variable and the five latent variables, described in the literature section, as independent variables. When the owners establish the price, they take into consideration different aspects that influence the value of their listing for tourists: their listings’ characteristics, their rental policies, their involvement, the reputation of their listing, and the distance to the city centre. The host involvement construct represents an upgrade of the host’s characteristics including the owner’s attributes and behaviour in managing their listing on the platform. The effects of attributes on prices are mixed (Kakar et al., Citation2016; Wang & Nicolau, Citation2017), while the effects of owner’s behaviour were not analysed yet in the literature.
Based on the previous findings, and considering reputation as an important factor in this process, being the results of the interaction between other categories of factors (host involvement, listing characteristics, rental policies, location), the authors have developed the model described in .
4. Methodology
4.1. Description of variables
Browsing the literature focussed on pricing strategies in the sharing economy and tourism industry, several categories of factors were identified. Starting from this information, research hypotheses were developed, taking into consideration the specific aspects of sharing economy accommodation services. One of the most important issues that should be addressed is the flexibility of supply – compared to traditional accommodation units, the sharing economy owners are able at any moment to start (be active) or stop (be inactive) providing their services – aspect which determines fluctuations in supply from one month to another. This situation is the result of having at least three different categories of owners: 1) the experienced (professional) host who is providing services throughout the entire year, possibly operating more than one listing; 2) the stable income seeker who wants to obtain extra income by renting regularly its space, but not on a full-time basis; and 3) the opportunist who is renting its space occasionally just to obtain an extra income in a specific period, usually when the level of incomes they could obtain is higher due to seasonality of tourists flows.
The listing's characteristics, location, reputation, and rental policies latent variables have similar content to the studies presented in the literature section, while the host's involvement latent variable represents a new approach proposed by the authors. Previous studies analysed the influence of the owner’s characteristics/attributes on prices (Ert et al., Citation2016; Lorde et al., Citation2019), emphasising that the number of host’s photos and their facial expression represent sources of trustworthiness, which is associated with higher prices. Furthermore, the owner’s behaviour and actions in managing the listing’s presence on the platform and the communication with potential customers represent other important sources to build trust. These elements describe, in the authors' view, the host's involvement/effort in making their listing(s) more attractive for potential customers, a latent variable whose influence on the price will be investigated. In this direction, the authors consider that an owner will be more involved if he/she is willing to:
fulfil the criteria imposed by Airbnb to become a superhost
respond fast, and to all inquiries received: each host has 24 hours to respond to any inquiry, but some of them answer very fast, others very late, and some hosts do not answer at all. The hosts who are more involved and who want to have better performance will answer fast, so faster answers mean higher involvement. Furthermore, answering all inquiries represents a sign of involvement.
upload a bigger number of photos for each listing to allow the potential customers to have a better image of the location's characteristics. Each owner decides how many photos to upload to the Airbnb platform. The bigger the number of photos available, the higher will be the chance to attract bookings, so a host interested to represent an option for potential customers will upload more photos. Therefore, a bigger number of listing photos represent a sign of deeper involvement.
manage many listings on the Airbnb platform
implement a flexible pricing strategy according to the changes in the market supply and demand. If the host has a very low involvement in the pricing strategy, it will charge approximately the same price no matter the moment of the year, the variance of the average daily rate being small. On the other side, an owner who is very involved in the process of pricing will try to adjust the price according to the changes in the market, the variance of the average daily rate being higher.
4.2. Data collection and processing
To study the research hypothesis, yearly and monthly data of all Airbnb listings from Cluj-Napoca were obtained from AIRDNA (airdna.co). The main purpose of data selection was to identify and use only the active listings in the analysis. The following criteria were used:
The monthly reported average daily rate should be higher than 0
Only the listings with reviews were selected
The shared rooms listings were eliminated due to their small number (they were only 10 listings)
During the timespan, April 2017 – March 2018, a maximum of 952 listings were available for booking on the platform (see ). Applying the previous criteria, 881 listings were used in the analysis. Due to the tourism specificity of the city, the number of listings available fluctuates significantly from month to month, this aspect allowing the accommodation supply to adjust easily to tourism demand. The events organized in the city, some of them being well known at the national and international level, generate significant fluctuations both in the size of tourism flows and in the price of accommodation services.
Figure 2. Number of monthly Airbnb listings active in Cluj-Napoca, Romania.
Source: Authors’ calculation
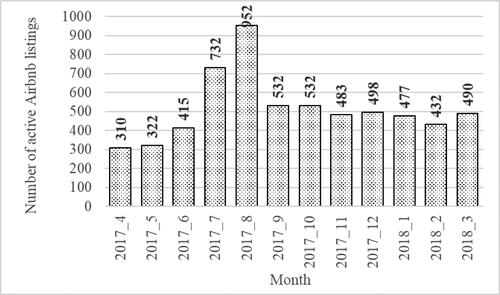
Most of the listings are small apartments (1-2 bedrooms), rented entirely to guests, without asking for a security deposit. Approximately 20% of the hosts were involved in the renting phenomenon for at least 10 months, 25% of them had the superhost status, while approximately 50% of the hosts were managing only one listing.
Structural equation modelling and multigroup analysis were conducted using Smart PLS 3. To implement the multigroup analysis, different categories of owners were created, based on the following criteria:
Starting from the number of months the hosts were active (their location was listed) on the platform during the analysed period: experienced (their listing was available for at least 10 months), stable income seekers (their listing was available between 5 and 9 months) and opportunists (their listing was available maximum 4 months);
Starting from the number of listings they managed on the platform: one listing and many listings;
Starting from the requirement of security deposit: hosts who ask for a security deposit and who do not ask for a security deposit;
Starting from superhost status: hosts who have the superhost status and who do not have the superhost status.
4.3. The model validation
To validate the model described in Section 3, the statistical significance of indicators’ effect on the latent variables was performed through a bootstrapping procedure. Since this is a formative model, a more liberal approach was adopted, considering the effect statistically significant if the p-value is less than 0.1 (see ).
Table 1. Description of variables used in the model.
Table 2. The listing’s characteristics.
Table 3. Outer weights.
The second step performed to validate the model was the collinearity diagnostic. Especially in the case of a formative model, multicollinearity must be avoided, the regression estimates being unstable and having high standard errors. The variance inflation factors (VIFs) were assessed and all the values were below 3 (see ), which means there is no problem with multicollinearity in the proposed model.
Table 4. Outer VIF values.
Kock (Citation2015) emphasised that a VIF greater than 3.3 is a sign of pathological collinearity and an indicator that the model may be contaminated by common method bias. In the case of the model proposed, since all VIFs resulting from a full collinearity test are lower than 3.3, the model is not affected by the common method bias.
To identify if there are significant differences between hosts concerning the factors that influence their price decision, a multigroup analysis was performed. The model proposed was applied to the categories of owners described in the data collection and processing section, to identify significant differences between the path coefficients.
5. Results and discussions
To evaluate the research hypotheses, the model presented in the methodology section was developed and applied in the case of Cluj-Napoca, Romania. The variables described previously were taken initially into consideration in the process of model creation, while in the final model only the variables and relationships having statistical significance have been kept. According to Sarstedt et al. (Citation2017), the strength of the relationship between latent variables is represented by path coefficients.
A path coefficient of 0.441 between the listing’s characteristics latent variable and the average daily rate implies that owners considering their listing as providing better amenities, will charge higher prices; for each additional standard deviation unit, the price construct will increase by 0.441 standard deviation units when keeping all other independent constructs constant. Moreover, analysing the path coefficients, it can be observed that the listing’s characteristics registered the strongest impact on price, followed by the host involvement latent variable, these two aspects being taken into consideration most often by the hosts.
Analysing the listing’s characteristics, only the number of bedrooms, the listing type, and the maximum number of guests were kept. Among these variables, the most important for hosts is the maximum number of guests, a bigger accommodation capacity determining the host to charge a higher price. The owners renting the entire location charge a higher price than the owners renting private rooms.
In conclusion, the H1 research hypothesis is being validated, the hosts considering the listing’s characteristics as one of the most important aspects for pricing, this result being in line with the previous studies presented in the literature section.
Regarding the H1 hypothesis, the multigroup analysis brings important contributions to the literature. The analysis focussed on hosts managing one listing compared to hosts managing many listings showed that the latter grant higher importance to the listing’s characteristics when they establish the price – a statistically significant difference of 0.192 (p-value = 0.998) being identified between the path coefficients. Correlating this result with the path coefficients between the host’s involvement and price (the involvement is the most important aspect in the pricing process in the case of owners managing one listing), we can suppose that the hosts managing many listings cannot be very involved in managing all of them, so they prefer to focus more on the listing’s characteristics when they fix the price.
Another difference in pricing behaviour is identified in the case of hosts asking for a security deposit, who are valuing more their involvement than the listing’s characteristics. Since only approximately 10% of owners are doing this, the security deposits may hurt their revenues, as many hosts confirmed on Airbnb forums. Being aware of this risk, they grant bigger importance to their involvement in hosting when they fix the price to generate an added value for the customers. Having the guarantee of being able to cover the unexpected damages, they try to compensate through their involvement the potential negative impact of their requirement – a statistically significant difference of 0.353 (p-value = 0.008) were identified between the path coefficients.
According to , the higher the distance to the city centre, the lower will be the price - the path coefficient of −0.078 between DistanceCityCentre and price latent variables implies that owners considering their listing as being located further will charge lower prices – for each additional standard deviation unit of the distance variable, the price construct will decrease by 0.078 standard deviation units, when keeping all other independent constructs constant. Therefore, the H2 hypothesis is validated.
The multigroup analysis confirms the mixed effect of the location described in the literature, providing valuable insights regarding the reasons for these effects. As the hosts gain more experience on Airbnb, they give higher importance to distance from the city centre: a statistically significant difference of 0.168 (p-value = 0.000) was identified between the path coefficients of experienced and opportunist hosts and a statistically significant difference of 0.090 (p-value = 0.032) was identified between the path coefficients of opportunists and stable income seeker hosts. In their long-run strategy, the experienced hosts are aware of the negative impact the distance may have on their listing reputation and they will try to compensate the distance with a price decrease. On the other side, the opportunists are renting usually in periods when the tourist flows are high, or when a specific opportunity appears, their short-run strategy is not influenced too much by the listing’s location.
Out of all variables tested in the final model, the listing’s reputation is evaluated through the monthly number of booked days and the number of reviews. Analysing the results from , it can be concluded that the H3 hypothesis is not validated - the path coefficient of −0.187 between the listing’s reputation and price implies that the owners who consider their listing having a good reputation, will charge lower prices – for each additional standard deviation unit of the listing’s reputation variable, the price construct will decrease by 0.187 standard deviation units when keeping all other independent constructs constant.
A better reputation does not determine the owner to increase the price, but to lower it. This result contradicts the previous literature, one possible explanation can be the host’s strategy to increase the occupancy rate by lowering the price; like this, the number of reviews is rising also. It seems that this approach is characteristic to all categories of owners in the analysed market, no significant statistical difference being identified between the path coefficients of any host categories. This strategy may be characteristic to new/incipient/immature markets, like Cluj-Napoca's market (the first apartment listed on Airbnb was in 2015), where the hosts, being aware of the increased competition they will have in the future, are interested to build a good reputation to achieve a long-run competitive advantage.
The hosts' involvement in managing their listing may be characterised through different items. In the final model, only the number of photos and the variance of the average daily rate were statistically relevant. As it can be noticed in the model (), a path coefficient of 0.369 between host involvement and the average daily rate implies that more involved owners will charge higher prices – for each additional standard deviation unit, the price construct will increase by 0.369 standard deviation units when keeping all other independent constructs constant, the H4 hypothesis is validated. Besides confirming the role of host characteristics described in the literature, the result develops the literature highlighting the significant role of the owner’s behaviour. Rational hosts, focussed on maximising their listing value in the long run, will be willing to allocate more resources and to get more involved in developing a pricing policy adapted to changes in market demand.
The rental policies with statistically significant influence in the model are the value of cleaning fee, extra people fee, minimum stay, and instant booking enabled. Any additional fee the host charges will increase the final price, while the minimum stay and instant book policies facilitate a decrease of price. Overall, the H5 hypothesis was validated - the path coefficient of 0.160 between rental policies and price implies that hosts who impose rental policies will tend to charge higher prices – for each additional standard deviation unit of the rental policies variable, the price construct will increase by 0.160 standard deviation units, when keeping all other independent constructs constant.
The multigroup analysis showed that the owners having the superhosts status consider the impact of rental policies on price less significant than other hosts - a statistically significant difference of 0.128 (p-value = 0.030) was identified between the path coefficients. One possible explanation for this difference is the fact that superhosts prefer to have instant booking enabled and to establish more than one night for the minimum stay, aspects which compensate for the increase of price due to other policies.
6. Conclusions
The findings of this study highlighted different degrees of influence for a set of 5 categories of variables upon the host’s behaviour on the Airbnb platform in the process of setting the rental prices. It has been considered a total of 24 variables classified in the following categories: listing’s characteristics (7 variables), listing’s reputation (4 variables), distance to the city centre (1 variable), host’s involvement (6 variables), and rental policies (6 variables).
6.1. Theoretical implications
Following previous studies done in the same area of research, the greater impact on price decisions is from the listing’s characteristics, especially the maximum number of guests, number of bedrooms, and type of listing. A novel contribution in this regard is the finding that owners who are managing many listings are influenced more significantly by listings’ characteristics in establishing rental prices in comparison to those who are managing only one listing.
The study confirms that the location of the listing represents another important variable in setting the price. Like in the case of hotels, a better location established as a shorter distance to the city centre or closer to a tourist attraction is used by hosts to increase their rental prices. Previous studies emphasised that the distance to the city centre may have both positive and negative effects on prices, the results of this study bringing a new perspective on this situation. According to their strategic thinking, the owners perceive differently the importance of location in their pricing strategies. The opportunist hosts value less the role of the location, the main reason being their short-run strategy focussed on revenues maximisation, compared to experienced owners who are focussed to achieve long-run success.
This study adds new knowledge to the existing literature by defining a new category of variables in the analysis of price determinants – the hosts' involvement in managing their listing(s). Previous studies focussed on hosts’ characteristics/attributes assessed mostly the impact of hosts’ attributes on their pricing decisions, while, in this paper, the authors focus on the hosts’ behaviour in the usage of the revenue management principles and pricing policies to generate additional value for them and their customers. This behaviour represents a strong evidence regarding the raising of a new host segment, more entrepreneurial, paying more attention to the market environment, with more knowledge regarding accommodation business activities and appropriate marketing and pricing strategies.
6.2. Practical implications
In a platform where the reviews count, both guests and owners adjust their behaviour to build confidence and to co-create value for both. As a result, most of the hosts with good reviews, follow in their pricing strategy an approach that allows them to create a good quality-cost experience for their guests. For example, the hosts who impose more complex rental policies tend to use higher prices in the rental process, but at the same time, being aware that the rental policies bring them additional benefits, they are willing to involve more to generate additional value also for their guests. Thus, through increased interaction with the guest and Airbnb platform, the owners improve the quality of the services they provide, enriching the guests’ experience, better quality is associated with higher prices. Furthermore, the hosts asking for a security deposit are characterised by a higher level of involvement, this aspect being more important in their pricing strategy than the listings’ characteristics.
Superhosts represent another category of owners who provide high quality services to their guests. Contrary to the previous approach, the higher quality they provide results from their higher involvement and flexibility (most of them allow instant booking) and from their willingness to provide better listing amenities, the rental policies being less significant.
Contrary to the conclusions of the studies described in the literature section, the listing's reputation has a negative impact on the price established by hosts. This result supports the idea that the price determinants differ across regions, two aspects being relevant for this situation - the level of the market development and the approach the host has in building the listing’s reputation. Usually, an incipient market is registering a high dynamic in terms of listings available from year to year, some of the hosts adopting long-run strategies to build their reputation. Also, no matter the level of market development, there will always be new hosts joining the platform, aiming to achieve notoriety rapidly. In both cases, these hosts are willing to charge lower prices to receive more bookings, increase the occupancy rate, and receive more reviews. Moreover, correlating these aspects with a high level of involvement, the owners will be able to achieve both very good review scores and a high number of reviews, a proof of the high-quality experience they provide.
Thus, the results obtained may represent appropriate guidelines for the persons willing to rent their apartments/homes through a sharing economy platform in choosing the best strategy. The “right price” must be established starting from the home’s characteristics and facilities provided to clients. Then, according to their objectives (short-run or long-run), they will have to adjust the price in relation to the distance to the city centre and their involvement.
6.3. Limitations of the study
The price’s determinants differ undoubtedly across regions due to the regions’ characteristics and economics and due to Airbnb development. As a result, testing the proposed model on a developing market may represent a limitation of the study. Comparing with previous studies, most of the price determinants have a similar influence on pricing decision, but there are also other factors with a different impact, creating new opportunities for further studies.
The influence of market development on the relationship between a listing’s reputation and the price represents one of the aspects that will be studied in the future. In mature markets, owners who achieve reputation use this asset to obtain higher revenues, while in the case of incipient markets, the goal of achieving reputation may be associated with lower prices.
Disclosure statement
No potential conflict of interest was reported by the author(s).
References
- Brochado, A., Troilo, M., & Shah, A. (2017). Airbnb customer experience: Evidence of convergence across three countries. Annals of Tourism Research, 63, 210–212. https://doi.org/10.1016/j.annals.2017.01.001
- Chen, Y., & Xie, K. (2017). Consumer valuation of Airbnb listings: A hedonic pricing approach. International Journal of Contemporary Hospitality Management, 29(9), 2405–2424. https://doi.org/10.1108/IJCHM-10-2016-0606
- Cheng, M. (2016). Sharing economy: a review and agenda for future research. International Journal of Hospitality Management, 57, 60–70. https://doi.org/10.1016/j.ijhm.2016.06.003
- Dudás, G., Boros, L., Kovalcsik, T., & Kovalcsik, B. (2017). The visualisation of the spatiality of Airbnb in Budapest using 3-band raster representation. Geographia Technica, 12(1), 23–30. https://doi.org/10.21163/GT_2017.121.03
- Edelman, B., & Geradin, D. (2015). Efficiencies and regulatory shortcuts: How should we regulate companies like Airbnb and Uber? Stanford Technology Law Review, 19, 293–328.
- Edelman, B., Luca, M. (2014). Digital Discrimination: The Case of Airbnb.com. Harvard Business School Working Paper. Retrieved October 10, 2019, from https://www.hbs.edu/faculty/Publication%20Files/Airbnb_92dd6086-6e46-4eaf-9cea-60fe5ba3c596.pdf
- Ert, E., Fleischer, A., & Magen, N. (2016). Trust and reputation in the sharing economy: The role of personal photos on Airbnb. Tourism Management, 55, 62–73. https://doi.org/10.1016/j.tourman.2016.01.013
- Fang, B., Ye, Q., & Law, R. (2016). Effect of sharing economy on tourism industry employment. Annals of Tourism Research, 57, 264–267. https://doi.org/10.1016/j.annals.2015.11.018
- Farmaki, A., & Stergiou, D. P. (2019). Escaping loneliness through Airbnb host-guest interactions. Tourism Management, 74, 331–333. https://doi.org/10.1016/j.tourman.2019.04.006
- Frenken, K., & Schor, J. (2017). Putting the sharing economy into perspective. Environmental Innovation and Societal Transitions, 23, 3–10. https://doi.org/10.1016/j.eist.2017.01.003
- Gibbs, C., Guttentag, D., Gretzel, U., Morton, J., & Goodwill, A. (2018). Pricing in the sharing economy: A hedonic pricing model applied to Airbnb listings. Journal of Travel & Tourism Marketing, 35(1), 46–56. https://doi.org/10.1080/10548408.2017.1308292
- Gibbs, C., Guttentag, D., Gretzel, U., Yao, L., & Morton, J. (2018). Use of dynamic pricing strategies by Airbnb hosts. International Journal of Contemporary Hospitality Management, 30(1), 2–20. https://doi.org/10.1108/IJCHM-09-2016-0540
- Gunter, U., & Onder, I. (2018). Determinants of Airbnb demand in Vienna and their implications for the traditional accommodation industry. Tourism Economics, 24(3), 270–224. https://doi.org/10.1177/1354816617731196
- Gutt, D., Herrmann, P. (2015). Sharing means caring? Hosts’ price reaction to rating visibility. European Conference on Information Systems. Münster. Retrieved October 5, 2019, from http://aisel.aisnet.org/cgi/viewcontent.cgi?article=1053&context=ecis2015_rip
- Guttentag, D. (2015). Airbnb: Disruptive innovation and the rise of an informal tourism accommodation sector. Current Issues in Tourism, 18(12), 1192–1217. https://doi.org/10.1080/13683500.2013.827159
- Guttentag, D., Smith, S., Potwarka, L., & Havitz, M. (2018). Why tourists choose Airbnb: A motivation-based segmentation study. Journal of Travel Research, 57(3), 342–359. https://doi.org/10.1177/0047287517696980
- Hamari, J., Sjöklint, M., & Ukkonen, A. (2016). The sharing economy: Why people participate in collaborative consumption. Journal of the Association for Information Science and Technology, 67 (9), 2047–2059. https://doi.org/10.1002/asi.23552
- Ikkala, T., Lampinen, A. (2014). Defining the price of hospitality: networked hospitality exchange via Airbnb. In Proceedings of the Companion Publication of the 17th ACM Conference on Computer Supported Cooperative Work & Social Computing (pp. 173–176).
- Kakar, V., Franco, J., Voelz, J., Wu, J. (2016). Effects of host race information on Airbnb listing prices in San Francisco. Retrieved from https://mpra.ub.uni-muenchen.de/69974/1/
- Kock, N. (2015). Common method bias in PLS-SEM: A full collinearity assessment approach. International Journal of e-Collaboration, 11(4), 1–10. https://doi.org/10.4018/ijec.2015100101
- Lampinen, A., & Cheshire, C. (2016). Hosting via Airbnb: Motivations and financial assurances in monetized network hospitality. In Proceedings of the 2016 CHI Conference on Human Factors in Computing Systems, San Jose, CA, USA. (pp. 1669–1680). Retrieved September 20, 2019
- Li, Y., Pan, Q., Yang, T., Guo, L. (2016). Reasonable price recommendation on Airbnb using Multi-Scale clustering. In Proceedings of the 35th Control Conference (CCC) (pp. 7038–7041). Retrieved September 15, 2019
- Liang, S., Schuckert, M., Law, R., & Chen, C.-C. (2017). Be a “Superhost”: The importance of badge systems for peer-to-peer rental accommodations. Tourism Management, 60, 454–465. https://doi.org/10.1016/j.tourman.2017.01.007
- Lorde, T., Jacob, J., & Weekes, Q. (2019). Price-setting behavior in a tourism sharing economy accommodation market: A hedonic price analysis of AirBnB hosts in the Caribbean. Tourism Management Perspectives, 30, 251–261. https://doi.org/10.1016/j.tmp.2019.03.006
- Meleo, L., Romolini, A., & de Marco, M. (2016). The sharing economy revolution and peer-to-peer online platforms. The case of Airbnb. In Proceedings of the International Conference on Exploring Services Science, Bucharest, Romania (Vol. 247, pp. 561–570). Retrieved September 12, 2019
- Mody, M., Suess, C., & Lehto, X. (2017). The accommodation experiencescape: A comparative assessment of hotels and Airbnb. International Journal of Contemporary Hospitality Management, 29(9), 2377–2404. https://doi.org/10.1108/IJCHM-09-2016-0501
- Neeser, D., Peitz, M., & Stuhler, J. (2015). Does Airbnb hurt hotel business: Evidence from the nordic countries. https://doi.org/10.13140/RG.2.1.4939.8248
- Sarstedt, M., Ringle, C. M., & Hair, J. F. (2017). Partial least squares structural equation modeling. In Handbook of market research (pp. 1–40). Springer International Publishing AG 2017.
- Sherwood, H. (2019, May 5). How Airbnb took over the world. The Guardian https://www.theguardian.com/technology/2019/may/05/airbnb-homelessness-renting-housing-accommodation-social-policy-cities-travel-leisure.
- Sigala, M. (2017). Collaborative commerce in Tourism: Implications for research and industry. Current Issues in Tourism, 20 (4), 346–355. https://doi.org/10.1080/13683500.2014.982522
- Tussyadiah, I., & Pesonen, J. (2016). Impacts of peer-to-peer accommodation use on travel patterns. Journal of Travel Research, 55 (8), 1022–1040. https://doi.org/10.1177/0047287515608505
- Wang, Y., Asaad, Y., & Filieri, R. (2019). What makes hosts trust Airbnb? Antecedents of hosts’ trust toward Airbnb and its impact on continuance intention. Journal of Travel Research, 59(4), 686–703. https://doi.org/10.1177/0047287519855135
- Wang, D., & Nicolau, J. (2017). Price determinants of sharing economy based accommodation rental: A study of listings from 33 cities on Airbnb.com. International Journal of Hospitality Management, 62, 120–131. https://doi.org/10.1016/j.ijhm.2016.12.007
- Zervas, G., Proserpio, D., & Byers, J. (2017). The rise of the sharing economy: Estimating the impact of Airbnb on the hotel industry. Journal of Marketing Research, 54(5), 687–705. https://doi.org/10.1509/jmr.15.0204
- Zhang, Z., Chen, R. J., Han, L. D., & Yang, L. (2017, September). Key factors affecting the price of Airbnb listings: A geographically weighted approach. Sustainability, 9(9), 1635. https://doi.org/10.3390/su9091635
- Zhang, T., Jahromi, M., & Kizildag, M. (2018). Value co-creation in a sharing economy: The end of price wars? International Journal of Hospitality Management, 71, 51–58. https://doi.org/10.1016/j.ijhm.2017.11.010