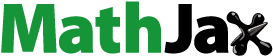
Abstract
In the post-industrial period, traditional Chinese enterprises are facing the awkward situation of being ‘big but not strong’, with their core technologies being controlled by others. The digital transformation of enterprises has not only reshaped business models and industrial boundaries, but also boosted the high-quality development of China’s economy. This paper reviews the existing literature and discovers that digital technology promotes enterprise production efficiency through cost reduction, efficiency improvement, and innovation. Based on the data of listed manufacturing companies in the Shanghai and Shenzhen stock exchanges from 2009 to 2017, this paper constructs a differences in differences (DID) model to empirically study the relationship between digital transformation and production efficiency. The results revealed that the implementation of digital transformation plays a significant role in promoting economic benefits and the results of the lag regression method are still robust. Based on this, combined with the actual situation of Chinese enterprises, this paper proposes countermeasures and suggestions to promote the development of enterprise digital transformation. The conclusion is of great significance for Chinese enterprises to occupy a dominant position in the new wave of global industrial revolution.
1. Introduction
The integration of digital technology and traditional industry innovation is a new force driving China’s rapid economic growth. The digitisation of the external environment paves the way for the digital transformation of traditional enterprises. In recent years, the rapid development of digital technology represented by 5 G, cloud computing, artificial intelligence, and so on has continuously spawned new forms and models that meet the needs of the market, promoting the digital transformation of entity enterprises. A White Paper on China’s Digital Economy (2020) holds that digital technology is the core driving force of the digital economy The integration of digital technology with the real economy not only improves the level of digitisation, networking, and intelligence of enterprises, but also accelerates the reconstruction of economic development and governance model. Statistics reveal that industrial digitisation has become an important supporting force for the development of the national economy. In 2019, China’s industrial digital added value was 28.8 trillion yuan, accounting for 29% of its GDP.
China has gradually transitioned to the late stage of industrialisation (Huang, Citation2018). However, in the face of a new wave of industrial change, the problems of traditional enterprises being ‘big but not strong’ and having their core technology controlled by others have been highlighted, which has seriously affected the high-quality development of China’s economy. Research has shown that the rapid growth of information technology can effectively promote rapid economic growth (David & Grobler, Citation2020), with digital technology invading all areas of business including retail, advertising, healthcare, finance, manufacturing, and education (Matyushok et al., 2020). As early as 2012, China’s government began to attach importance to the layout of digital technology-related industries, promulgating the ‘12th Five-Year Plan’ on the issuance of the National Strategic Emerging Industries Development Plan and other documents to promote not only research on big data, cloud computing, Internet of things, and other digital technologies, but also the integration of information and industrialisation. Subsequently, the government launched a series of policy documents related to the ‘Internet’, further promoting the digital transformation of traditional enterprises. For the first time, in 2016, the Hangzhou G20 Summit passed the Digital Economic Development and Cooperation Initiative, which marked a new chapter for the development of China’s digital economy. In recent years, advancements in artificial intelligence and other digital technologies have strengthened the pathway towards the digital transformation of traditional enterprises. The occurrence of black swan events, such as the novel coronavirus pandemic, further spawned new products and forms suited to current consumer demands, subverted traditional industries, and forced the digital transformation of traditional enterprises. There is no doubt that traditional enterprises are reshaping their business models and industrial boundaries through digital transformation to promote the high-quality development of China’s economy.
This digital transformation of traditional enterprises is important to enhance supply-side structural reform. It is of great significance to clarify the mechanism and key problems of digital technology driving the digital transformation of traditional enterprises in China’s economy. Based on the present situation of the digital transformation of traditional enterprises, this paper combs the relevant literature, analyses the mechanism of digital technology driving the digital transformation of traditional enterprises, and explores the bottleneck problems encountered in transformation and upgrading enterprises. This will help provide a reference for relevant government departments and enterprise digital transformation.
2. Mechanism analysis of enterprise digital transformation and production efficiency
In the digital age, rapid changes in the consumer market have led to the active digital transformation of traditional industries. Digital transformation of enterprises includes the overall transformation of enterprise strategy, marketing, commodities, business model, management, corporate culture, and thinking mode (Ma et al., Citation2017). The research literature on digital transformation from the perspective of enterprises has been scarce in recent years, with some scholars failing to clearly explain the forms of digital transformation in enterprises. Therefore, this paper combs the existing literature from the perspective of cost reduction, efficiency improvement, and innovation to analyse the internal mechanism of enterprise digital transformation and production efficiency.
The characteristics of zero cost replication and transmission of knowledge, information, and experience in digital technology greatly weaken the costs of enterprise production, transaction, and management, thus promoting the digital transformation of traditional enterprises. A study by Abouzeedan et al. (Citation2013) revealed that enterprise digitalisation is conducive to strengthening the ability to acquire resources required for operations, production, innovation, and other activities and to save related expenditures. Njihia and Merali (Citation2013) research revealed that digital technology development promotes the flow of factor resources and reduces the cost of access to financial and human resources for poor people. Studies by Lerner et al. (Citation2006), Agrawal and Goldfarb (Citation2008), Brynjolfsson and Saunders (2009), Dana and Orlov (Citation2014), Goldfarb and Tucker (Citation2019), and others have shown that the application of digital technology in enterprises can reduce the following five types of costs: search, copy, transportation, tracking, and verification costs. Xiao et al. (Citation2015) and other studies revealed that the application of digital technology in the production and operation of enterprises such as procurement, sales, and distribution can effectively improve the allocation of resources, reduce the transaction costs caused by information asymmetry, and meet consumers’ personalised needs on time. A study by He (Citation2018) revealed that the interconnection of human body data, sample version data, process data, and production data through digital platforms makes large-scale, intelligent, and customised production possible in the garment industry and promotes its transformation. Lin and Lv (2019) used case studies to show that because digital technology presents all aspects of enterprise production, sales, and consumption in the digital form, transparent information results in personalised, customised, and experiential service consumption. Moreover, traditional business models do not break, consumers change to ‘productive consumers’, and sales channels of enterprises gradually shift from offline to online. Li and Zhang (Citation2019) revealed that big data technology can optimise the internal structure of traditional industries and reconfigure inter-industry essential resources, thus driving the transformation and upgrading of traditional industries. Qi et al. (Citation2020) revealed that digital technology has opened up the information barriers of both supply and demand markets, accelerated the circulation of market information, and effectively solved the problem of oversupply in traditional industries.
A study by Gölzer and Fritzsche (Citation2017) showed that digital technology can be used precisely in business processes. Because the intelligent decision-making system and control system improve the operation and management efficiency of enterprises, their production performance is improved. Li’s (2020) research revealed that digital technology can effectively drive the intelligent transformation of enterprise products and services and the creation of new marketing operations, which, consequently, can help improve enterprise market share and performance. Vial (Citation2019) revealed that digital technology can effectively promote organisational change in enterprises and enhance enterprise operation performance through value creation. Zhu et al. (Citation2021) pointed out that investments in R&D and information communications technology (ICT) increase the probability of product and process innovation, which increase the firm’s productivity. An empirical study by Qi and Cai (Citation2020) showed that the digital transformation of China’s non-high-tech manufacturing industry is in its infancy and the positive impact of its digitisation on enterprise performance is offset by management disorders. However, digital transformation effectively affects business model innovation. Zhou et al. (Citation2020) conducted an empirical study from a regional perspective, finding that although regional digitisation level had a significant positive impact on regional innovation performance, very high regional digital application and platform and equipment construction would inhibit regional innovation performance.
If the level of use of digital channels is high, it could change company communication and transform basic marketing and business concepts (Stankovska et al., Citation2016), with information exchange leading to more innovativeness in high-tech small and medium-size enterprises (SMEs) (Pustovrh et al., Citation2017). A study by Srinivasan and Venkatraman (2018) showed that enterprises connect producers, service providers, traders, and consumers through digital platforms, creating a new community market and promoting the digital transformation of the enterprise business model. Kong et al. (Citation2018) studied the impact of Google’s withdrawal from the Chinese search service market on the innovation of Chinese companies that rely on foreign technology; they discovered that the availability of information is a decisive factor for corporate innovation. Gil-Gomez et al. (Citation2020) revealed that customer relationship management (CRM) can be regarded as a kind of green IT, oriented towards digital transformation and sustainable business model innovation. Li and Qiao (Citation2019) demonstrated that digital technology drives enterprise business model innovation from the four aspects of resources, value, business, and distribution. A study by Shi (Citation2019) revealed that digital technologies such as artificial intelligence can effectively solve the personalised customisation needs of the consumer market, promote not only the new models and formats with high efficiency and fine service, but also the transformation and upgrading of traditional industries. Studies by Xing et al. (Citation2019) and others have revealed that digital technology interacts with business model innovation, promoting inclusive market construction through innovative modes such as intelligent content creation and sharing and accurate content push. An empirical study by Shen and Yuan (Citation2020) revealed that the transformation of enterprises to the Internet has significantly improved their independent innovation capabilities.
Studies by Koellinger (Citation2008) and Forman and Zeebroeck (Citation2012) found that innovation based on digital technology can result in better effects on corporate performance. Empirical research conducted by Graetz and Michaels (2015) revealed that emerging production methods such as industrial robots, automation, and artificial intelligence have increased the economic growth rate by 0.37%. Acemoglu and Restrepo (Citation2018) use the task model to theoretically describe that total factor productivity (TFP) is an important transmission mechanism for industrial robots to affect economic growth. Based on the Acemoglu and Restrepo (Citation2018) task model, Yang and Hou (2020) proved that industrial robots can affect economic growth not only directly, but also through TFP. He and Liu (Citation2019) used empirical research of entity enterprise data to show that the digital transformation of enterprises can improve the efficiency of capital use and the innovation ability of enterprises, thereby significantly improving the production performance of enterprises. Chen et al. (Citation2019) revealed that the application of artificial intelligence can improve the intelligence and automation of production activities, increase the rate of return on capital, and increase TFP. According to Wang et al. (Citation2020), the digital economy gave rise to new industries, business formats, and business models, which have improved the efficiency of social production. Research by Zhao et al. (Citation2020) revealed that the development of the digital economy can promote high-quality economic development by promoting enterprise innovation.
3. Measurement model construction, variables, and data description
3.1. Construction of the measurement model
In 2012, China enacted the ‘12th Five Year’ National Strategic Emerging Industry Development Plan, focusing on the R&D of new generation information technology such as the Internet of Things and cloud computing and clarifying the development strategy of informatisation and digitisation. Affected by this policy, some companies proposed digital transformation development strategies to intensify the application of a new generation of digital technology. Based on the proposed implementation of digital transformation development by listed companies in temporary or regular announcements in 2012 as a natural experiment, the double difference method was used to estimate the impact of corporate digital transformation on production efficiency. By controlling other variables, this method examines whether there is a significant difference in production efficiency between the treatment and control groups before and after the implementation of digital transformation in listed companies. The model setting is as shown in formula (1):
(1)
(1)
Here, i is the listed company and t is the year; Y refers to the production efficiency of listed companies; is the core explanatory variable; and
In the sample period, if listed company i implements digital transformation strategy,
otherwise it is 0; if
otherwise it is 0. In this paper, the processing group comprises the manufacturing listed companies that implement digital transformation, and the control group comprises other manufacturing listed companies.
is the control variable;
represents the unobservable regional individual effect; and
represents the time effect;
is a random interference term, which obeys the normal distribution.
3.2. Data source and variable description
Because China is currently in a critical period of manufacturing transformation and upgrading, and the digital transformation of enterprises is of great significance to the manufacturing industry, in this article, listed manufacturing companies in Shanghai and Shenzhen from 2009 to 2017 were selected as the research object, drawing on the method followed by He and Liu (Citation2019) based on the Cathay Pacific database, China Stock Market & Accounting Research (CSMAR), and Wind database. Companies that proposed digital transformation and other related information in the 2012 listed company disclosure report were collected and counted, resulting in 116 selected industry manufacturing companies.
The explanatory variable in this article is the return on net assets (Roe) that measures the production efficiency of listed companies; the core explanatory variable is the corporate digital transformation (Treatment). The data is based on the disclosure reports of listed companies. Taking into account the research needs, this article sets the control variables: asset-liability ratio (Alr), company size (Size), property rights (Soe) and duality (Duality). The definition of each variable and descriptive statistics are shown in .
Table 1. Descriptive statistics of variables.
4. Empirical results and analysis
4.1. Balanced trend hypothesis test
indicates that before 2012, the treatment and control groups showed similar trends during the period from 2009 to 2012 but showed differences after 2012. The Roe of the treatment group was significantly better than that of the control group. After 2016, the control group has shown an upward trend perhaps due to the integration of 5 G, AI, and other technologies with the real economy.
4.2. Benchmark regression results
In order to clearly understand the economic benefits brought about by the digital transformation of enterprises, this paper considers Roe as the dependent variable, the implementation of digital transformation as the core explanatory variable, and adds control variables such as company scale characteristics, property rights, and governance characteristics for regression estimation. According to the setting of the difference in differences (DID) model, the estimated results are shown in .
Table 2. DID model estimation results.
Model (1) is a benchmark regression, with the results revealing that compared to not implementing digital transformation, its implementation by enterprises has a significant promotion effect on economic benefits. Considering that the digital transformation of enterprises is a slow process, models (2–4), and (5) all use the lag phase of the digital transformation of enterprises as the core explanatory variable, which can better explain real problems. The positive effect of digital transformation on the economic benefits of its implementation of digital transformation is relatively stable compared with that of enterprises not implementing digital transformation; industry and regional fixed effects are added separately, and the conclusion remains unchanged. This shows that the digital transformation produces an obvious performance improvement effect and can promote the high-quality development of Chinese enterprises.
5. Conclusions and suggestions
Digital technology represented by 5 G, artificial intelligence, big data, Internet of Things, and so on has promoted a new wave of industrial transformation in the world. The digital transformation of enterprises is the only way for China to enhance its industrial competitiveness and promote high-quality economic development. In this regard, countries have proposed digital economy strategies one after another, aiming to take the lead in the new wave of industrial transformation. Compared with developed countries, China's informatisation began late, and the joint advancement of informatisation and digitisation has brought challenges to the digital transformation of enterprises. Based on the actual situation of traditional Chinese enterprises, this paper analyses the internal mechanism of enterprise digital transformation and production efficiency, proposing relevant suggestions based on the practical experience of Chinese enterprises.
The first suggestion relates to improving the digital economy legal system and clarifying data use system norms. At present, there are lagging laws and regulations related to the digital transformation of Chinese enterprises, and digital intellectual property rights are not clear. National laws, regulations, and policies are different for issues such as data ownership, management, use, and transactions; moreover, many digital technology-related laws and regulations in China need to be improved. The lack of standards and systems is another problem. To address this, the government should construct a strategic system of the digital economy, clarify the task list of each department, coordinate as a whole, and form the resultant force of the digital transformation of enterprises. Digital property rights, data security, and other digital economy-related laws and regulations need to be constantly improved and information security standards and management norms should be established in the digital environment. Rules and regulations for data opening, management, trading, and so on should be formulated to protect enterprises from jointly developing data and to actively connect with international digital trade, investment, and judicial rules. This will encourage enterprises to digitise transnational development.
The construction of a new generation of information technology infrastructure such as 5 G, which provides basic support for the digital transformation and development of enterprises should be accelerated. Comprehensive network coverage and smooth broadband speed are important guarantees for enterprises to obtain product information at all times. However, China’s 5 G infrastructure construction has just begun, and its 61.2% network coverage rate is far lower than the 87.3% and 84.6% coverage rates of the United States and Japan, respectively. Moreover, the uneven distribution of regional network resources has hampered the digital transformation of enterprises in some remote areas. Inadequate infrastructure support for digital technology has restricted the digital transformation of Chinese companies. Therefore, the government should accelerate the 5 G, artificial Internet of Things, Internet Data Center(IDC), and other intelligent information infrastructure forms that integrate perception, transmission, storage, calculation, and processing and adapt them to the enhanced application broadband of enterprise digital transformation. In order to build a distributed resource sharing information resource bank, a cross-industry, cross-manufacturer integrated Internet of Things management platform should be developed to create a safe, controllable, internationally competitive innovation service system.
It is also important to build a digital technology innovation platform and encourage school-enterprise cooperation to study the neck jamming technique. Due to the weak R&D capabilities of most companies and the lack of common technology platforms in the industry, many companies mainly rely on the purchase of foreign advanced equipment and the introduction of production capabilities to achieve technological transformation, resulting in the lack of core technologies and poor independent innovation capabilities, resulting in the digital development of Chinese manufacturing companies. To address the issues of unbalanced, inadequate problems and insufficient mastery of core technologies such as industrial chips, smart sensors, industrial robots, and computerized numerical control machine tools, the government should encourage leading enterprises to undertake major national, provincial, and municipal science and technology projects and support cooperation among enterprises, universities, and scientific research institutes to build joint laboratories and research centres for artificial intelligence, big data, and other technologies. Cloud computing, quantum communications, information security, intelligent robots, and other key areas should also be prioritized to build open and innovative R&D platforms and jointly tackle key problems with a major leading role in the neck jamming technique.
The whole chain of digital transformation services should be actively deployed. Most of the enterprises in China do not have a high level of informatisation; their understanding of digital transformation reflects the level of introducing digital technology; they, especially SMEs, which do not systematically promote enterprise production, lack basic understanding of industrial Internet, big data, cloud computing, etc. Digital transformation at all levels, including operations, management, and culture, is the need of the hour. In addition, the market lacks professional third-party organisations that serve the digital transformation of enterprises, and enterprises do not have the ability to deploy digital design and operation technologies, which hinder their digital transformation. The government should support the classification of sub-database construction covering enterprises, industries, products, markets, R&D, and other data resources of the industry database system, right from product design, production, and manufacturing to after-sales service. Through the organisation of industry competitions, such as summit forums and international conferences, horizontal and vertical industrial chain exchanges, cooperation, and demand docking can be enhanced, and the gathering of high-end innovation elements in the field of global digital technology can be accelerated.
The training mechanism of digital talents should be examined and the digital literacy of the entire population must be enhanced. The digital transformation of enterprises urgently needs a large number of interdisciplinary talents fluent in both digital technology and professional knowledge. However, because China’s digital technology development began relatively late, universities train fewer corresponding talents, making it difficult to find leading talents in the field of digital technology. There is also a large talent gap in ordinary digital technology positions, which hinders the digital transformation of enterprises. Comprehensively strengthening the construction of basic, professional, and practical courses on digital technology and strengthening students' digital ability are effective ways to overcome the shortage of talents. Google’s ‘Open Roberta’ cloud platform could be referenced to encourage teenagers to use ‘Lego brainstorming’ to program ideas. Schools should also take into account the interests of different students in terms of the digital technology platform’s tutoring content. Besides, the improvement of the cooperative education model combining production, education, and research, should be accelerated and the experience of the German dual system of vocational education and training should be followed.
A digital platform enabling the coordinated development of large, medium, and small enterprises should be constructed. At present, the digital transformation of Chinese enterprises is facing the problem of the imperfect industrial ecological chain. Due to the needs of digital transformation, many companies have built sharable cloud computing, big data, and industrial Internet platforms; however the number of companies that actually participate is very small because the ecosystems of each company are self-oriented, which will inevitably lead to conflicts between different companies. The ability of large and small-scale enterprises to develop collaboratively is weak; there is no digital industrial pattern in which large and small-scale enterprises have their own advantages, compete for innovation, and develop in cascade. The industrial ecological chain based on digital technology is not yet fully formed. In the long run, building a stable product ecosystem and a complete product ecological chain will help enterprises to take root and develop further in advantageous areas. Therefore, the government urgently needs to build a digital sharing platform based on large manufacturing enterprises and a digital enabling platform for SMEs. The government also needs to provide support for all kinds of innovation to build industry, public service, and docking exchange platforms; build R&D service platforms for SMEs; and carry out systematic enterprise digital transformation services, such as production management, achievement transfer transformation, and inspection.
Disclosure statement
No potential conflict of interest was reported by the authors.
Additional information
Funding
References
- Abouzeedan, A., Klofsten, M., & Hedner, T. (2013). Internetization management as a facilitator for managing innovation in high-technology smaller firms. Global Business Review, 14(1), 121–136. https://doi.org/10.1177/0972150912466462
- Acemoglu, D., & Restrepo, P. (2018, May). Modeling automation. Aea Papers and Proceedings, 108, 48–53. https://doi.org/10.1257/pandp.20181020
- Agrawal, A., & Goldfarb, A. (2008). Restructuring research: Communication costs and the democratization of university innovation. American Economic Review, 98(4), 1578–1590. https://doi.org/10.1257/aer.98.4.1578
- Brynjolfsson, E., & Saunders, A. (2009). Wired for innovation: How information technology is reshaping the economy. Mit Press.
- CAICT. (2020). White paper on China's digital economy. http://www.caict.ac.cn/kxyj/qwfb/bps/202007/t20200702_285535.htm.
- Chen, Y. B., Lin, C., & Chen, X. L. (2019). Artificial intelligence, aging and economic growth. Economic Research Journal, 54(07), 47–63.
- Dana, J. D., Jr, & Orlov, E. (2014). Internet penetration and capacity utilization in the US airline industry. American Economic Journal: Microeconomics, 6(4), 106–137.
- David, O. O., & Grobler, W. (2020). Information and communication technology penetration level as an impetus for economic growth and development in Africa. Economic research-Ekonomska Istraživanja, 33(1), 1394–1418. https://doi.org/10.1080/1331677X.2020.1745661
- Forman, C., & Zeebroeck, N. V. (2012). From wires to partners: How the Internet has fostered R&D collaborations within firms. Management Science, 58(8), 1549–1568. https://doi.org/10.1287/mnsc.1110.1505
- Gil-Gomez, H., Guerola-Navarro, V., Oltra-Badenes, R., & Lozano-Quilis, J. A. (2020). Customer relationship management: Digital transformation and sustainable business model innovation. Economic research-Ekonomska Istraživanja, 33(1), 2733–2750. https://doi.org/10.1080/1331677X.2019.1676283
- Goldfarb, A., & Tucker, C. (2019). Digital economics. Journal of Economic Literature, 57(1), 3–43. https://doi.org/10.1257/jel.20171452
- Gölzer, P., & Fritzsche, A. (2017). Data-driven operations management: Organisational implications of the digital transformation in industrial practice. Production Planning & Control, 28(16), 1332–1343. https://doi.org/10.1080/09537287.2017.1375148
- Graetz, G., & Michaels, G. (2015). Robots at work: The impact on productivity and jobs (No. 447). Centre for Economic Performance, LSE.
- He, X. T. (2018). Digital technology drives customized transformation of garment industry. Journal of Basic Science of Textile University, 31(01), 19–24. https://doi.org/10.13338/j.issn.1006-8341.2018.01.004
- He, F., & Liu, H. X. (2019). The performance improvement effect of digital transformation enter prises from the digital economy perspective. Reform, 4, 137–148.
- Huang, Q. H. (2018). China’s industrial development and industrialization process during the 40 years of reform and opening-up. China Industrial Economics, 9, 5–23. https://doi.org/10.19581/j.cnki.ciejournal.2018.09.011
- Koellinger, P. (2008). The relationship between technology, innovation, and firm performance—Empirical evidence from e-business in Europe. Research Policy, 37(8), 1317–1328. https://doi.org/10.1016/j.respol.2008.04.024
- Kong, D., Lin, C., Wei, L., & Zhang, J. (2018). Information accessibility and corporate innovation. Management Science, forthcoming. http://dx.doi.org/10.2139/ssrn.3291811
- Lerner, J., Pathak, P. A., & Tirole, J. (2006). The dynamics of open-source contributors. American Economic Review, 96(2), 114–118. https://doi.org/10.1257/000282806777211874
- Li, F. (2020). The digital transformation of business models in the creative industries: A holistic framework and emerging trends. Technovation, 92–93, 102012. https://doi.org/10.1016/j.technovation.2017.12.004
- Lin, L., & Lv, W. D. (2019). The impact of digital transformation on management reform of manufacturing enterprises: A case study based on Kutesmart and Haier. Scientific Decision Making, 25(01), 85–98.
- Li, F., & Qiao, H. (2019). Research on the business model evolution of digital technology driven industrial service—A case study of Goldwind. Management Review, 31(08), 295–304. https://doi.org/10.14120/j.cnki.cn11-5057/f.2019.08.025
- Li, Y. H., & Zhang, S. W. (2019). Path of Big Data driving the transformation and upgrading of traditional industry: Based on Big Data value chain perspective. Science and Technology Management Research, 39(07), 156–162. https://doi.org/10.3969/j.issn.1000-7695.2019.07.022
- Lv, T. (2019). Trend and path of digitalized transformation of the traditional industries. People’s ForumAcademic Frontier, 8(18), 13–19. https://doi.org/10.16619/j.cnki.rmltxsqy.2019.18.002
- Ma, H. T., Meng, Z. L., Yan, D. L., & Wang, H. L. (2017). Digital economy: A new driving force for China's innovation and growth. CITIC Publishing Group.
- Matyushok, V., Krasavina, V., Berezin, A., & García, J. S. (2021). The global economy in technological transformation conditions: A review of modern trends. Economic Research-Ekonomska Istraživanja, 34(1), 1471–1497. https://doi.org/10.1080/1331677X.2020.1844030
- Njihia, J. M., & Merali, Y. (2013). The broader context for ICT4D projects: A morphogenetic analysis. MIS Quarterly, 37(3), 881–905. https://doi.org/10.25300/MISQ/2013/37.3.10
- Pustovrh, A., Jaklič, M., Martin, S. A., & Rašković, M. (2017). Antecedents and determinants of high-tech SMEs’ commercialisation enablers: Opening the black box of open innovation practices. Economic research-Ekonomska Istraživanja, 30(1), 1033–1056. https://doi.org/10.1080/1331677X.2017.1305795
- Qi, Y. D., & Cai, C. W. (2020). Multiple effects of digitalization on the performance of manufacturing enterprises and its mechanism. Study & Exploration, 42(07), 108–119.
- Qi, Y. D., Xiao, X., & Cai, C. W. (2020). Digital reconstruction of industrial organization. Journal of Beijing Normal University(Social Sciences), 57(02), 130–147.
- Shen, G. B., & Yuan, Z. Y. (2020). The effect of enterprise internetization on the innovation and export of Chinese enterprises. Economic Research Journal, 55(01), 33–48.
- Shi, Z. Z. (2019). Integration of artificial intelligence and traditional industries—Analyze the intelligent development mode of enterprises with a focus on Ping’an technology. People’s ForumAcademic Frontier, 8(18), 20–27. https://doi.org/10.16619/j.cnki.rmltxsqy.2019.18.003
- Srinivasan, A., & Venkatraman, N. (2018). Entrepreneurship in digital platforms: A network‐centric view. Strategic Entrepreneurship Journal, 12(1), 54–71. https://doi.org/10.1002/sej.1272
- Stankovska, I., Josimovski, S., & Edwards, C. (2016). Digital channels diminish SME barriers: The case of the UK. Economic research-Ekonomska Istraživanja, 29(1), 217–232. https://doi.org/10.1080/1331677X.2016.1164926
- Vial, G. (2019). Understanding digital transformation: A review and a research agenda. The Journal of Strategic Information Systems, 28(2), 118–144. https://doi.org/10.1016/j.jsis.2019.01.003
- Wang, K. K., Wu, G. B., & Zhang, G. J. (2020). Has the development of the digital economy improved production efficiency? The Economist, 32(10), 24–34. https://doi.org/10.16158/j.cnki.51-1312/f.2020.10.004
- Xiao, J. H., Xie, K., Wu, Y., & Liao, X. H. (2015). The supply chain transformation from being partner from being partner-oriented to being customer-oriented: a double-case study on the supply chains in e-commerce enterprises. Management World, 31(4), 137–154. https://doi.org/10.19744/j.cnki.11-1235/f.2015.04.013
- Xing, X. Q., Zhou, P. L., Zhang, Z., & Tang, X. H. (2019). Digital technology, BOP business model innovation and inclusive market construction. Management World, 35(12), 116–136. https://doi.org/10.19744/j.cnki.11-1235/f.2019.0167
- Yang, G., & Hou, Y. (2020). The usage of industry robots, technology upgrade and economic growth. China Industrial Economics, 37(10), 2–20. https://doi.org/10.19581/j.cnki.ciejournal.2020.10.004
- Zhao, T., Zhang, Z., & Liang, S. K. (2020). Digital economy, entrepreneurship, and high-quality economic development: Empirical evidence from urban China. Management World, 36(10), 65–76. https://doi.org/10.19744/j.cnki.11-1235/f.2020.0154
- Zhou, Q., Wang, Y. L., & Yang, W. (2020). An empirical study of the impact of digital level on innovation performance—A study based on the panel data of 73 counties (districts, cities) of Zhejiang Province. Science Research Management, 41(07), 120–129. https://doi.org/10.19571/j.cnki.1000-2995.202007.013
- Zhu, F., Li, Q., Yang, S., & Balezentis, T. (2021). How ICT and R&D affect productivity? Firm level evidence for China. Economic Research-Ekonomska Istraživanja, 1–19. https://doi.org/10.1080/1331677X.2021.1875861