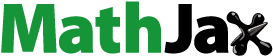
Abstract
This study proposes a simple two-period model to consider consumers’ borrowing behaviour in a decentralised consensus and information distribution platform. Based on this model, we develop a bank risk mitigation framework and find that decentralised digital identity and encryption technology are the most important factors for attaining market equilibrium between decentralised consensus and information distribution. Specifically, the greater the scope of digital identity construction and the more blockchain consensus records there are, the less likely the borrower will default. Our study provides meaningful practical implications for bankers and policy regulators to help them better understand consumers’ borrowing behaviour and decisions to default.
1. Introduction
Financial turmoil in the last two decades has revealed that much work remains to better understand the sources of bank risk and improve our monitoring tools (Li & Zinna, Citation2014). Nijskens and Wagner (Citation2011) argue that credit risk transfer affects bank risk, regardless of whether the increase in bank risk is due to higher individual bank risk or higher systemic risk. Additionally, information asymmetry between borrowing and lending parties plays a vital role in credit risk transfer and bank credit management. In this case, the opacity of commercial banks' operations amplifies banks’ risk transmission mechanisms (Clair, Citation1992). The rapid expansion of credit becomes a precursor to the banking crisis, which, to a certain extent, leads to a decline in asset quality and further triggers the reallocation of assets (Reinhart & Rogoff, Citation2009).
Several studies have investigated the association between opacity and bank risk. Zheng (Citation2020) shows that opacity has a negative effect on bank loan growth, with this effect being more pronounced for banks that are more reliant on wholesale funds. Other studies have attempted to address the issue of adverse selection and information asymmetry by examining the deposit insurance mechanism (Hou et al., Citation2016) and the social credit rating of borrowers and lenders (Hasan et al., Citation2013). Along with enhancing information disclosure, these measures could alleviate the problem and lessen adverse selection and information asymmetry. Nevertheless, the adverse selection and information asymmetry problem that induces bank opacity and bank risk cannot be completely solved within the present theoretical framework provided by banking studies.
Recently, researchers have developed theoretical models to study blockchain-based applications, such as cryptocurrencies and smart contracts, based on economics and finance theory (Cong et al., Citation2021; Cong & He, Citation2019). Cong and He (Citation2019) study the market equilibria between decentralised consensus and information distribution and posit that smart contracts based on blockchain technology can mitigate information asymmetry and improve welfare and consumer surplus, while distributing information during consensus generation may encourage greater collusion. However, the decentralised consensus and information distribution trade-off has not been fully considered in existing bank risk studies.
To fill this gap in the literature, we propose a simple two-period model to extend Drozd and Serrano-Padial (Citation2017) consumer default model and Cong and He (Citation2019) decentralised consensus and information model to consider consumers’ borrowing behaviours in a decentralised consensus platform. We make the following contributions:
First, this study is among the first to investigate consumers’ borrowing behaviour and their default decisions in a decentralised consensus platform. Without decentralised consensus, banks that provide centralised consensus often enjoy huge market power. Traditional bank credit is granted when the borrower provides certain collateral (secured debt) or obtains credit ratings from credit rating agencies (unsecured debt). Both collateral and credit ratings involve high degrees of human intervention and potentially lead to greater uncertainty and cost. Smart contracts may increase contractibility and enforceability on certain contingencies if decentralised digital identity and encryption technology are in place.
Second, scholars have examined and provided important insights into risk mitigation in the financial sector over the past few decades. However, they have attempted to provide solutions from a macro perspective (Eisenberg & Noe, Citation2001). For instance, Capponi and Chen (Citation2015) propose a multiperiod clearing framework and argue that the level of systemic risk is mitigated through the provision of liquidity assistance. Conversely, in this study, we investigate bank risk mitigation from a micro perspective. We divide consumers into consumers with digital identity and those without digital identity to investigate the relationship between digital identity and the opportunity cost of digital scene construction. We also explore the importance of social capital and credit prior probability by considering the trade-off between decentralised consensus and information distribution featured by blockchain applications.
The main findings of our study are as follows. In a two-period consumer default model, based on the bank risk mitigation framework, we find decentralised digital identity and encryption technology to be the most important factors to attain market equilibria between decentralised consensus and information distribution. Specifically, the greater the scope of digital identity construction and the more blockchain consensus records there are, the less likely it is that the borrower will default in terms of the impact of having a digital identity on bank credit risk. Owing to the information disclosure of their digital identity in a decentralised consensus platform, digital identity owners must bear greater costs if they want to collude with the recorder. Therefore, if the owner of a digital identity wants to default, he or she must bear a higher cost, with a corresponding smaller default risk.
The remainder of this paper is structured as follows. Section 2 reviews the existing literature on bank risk mitigation, blockchain architecture, technology development, and banking. Section 3 describes the two-period consumer default model of the bank risk mitigation framework. Section 4 explores two important factors of the bank risk mitigation framework: decentralised digital identity and encryption technology. Section 5 presents some applications of blockchain technology in the banking sector. Section 6 summarises the conclusions of the study.
2. Literature review
2.1. The bank risk mitigation literature
The use of information technology (IT) facilitates bank lending by mitigating adverse selection and informational asymmetries (Dashottar & Srivastava, Citation2021; Osmani et al., Citation2021). Livshits et al. (Citation2016) argue that financial innovations based on improved IT were a critical factor in the rise in aggregate borrowing and defaults in the US market during the 1980s and the 1990s. They find that financial innovations lead to more lending contracts, with each contract targeting small and niche groups and extending credit to riskier borrowers.
Several studies have examined the use of IT to mitigate adverse selection and information asymmetry and its effect on the provision of unsecured consumer credit. Narajabad (Citation2012) provides an informational explanation for the rise in household bankruptcy, the increase in unsecured consumer debt, and the rise in the availability of unsecured consumer credit in the US market. It is reported that credit availability and borrowing increase when creditors have more accurate information on assessing borrowers’ default costs with the help of IT development. Athreya et al. (Citation2012) find that asymmetric information is damaging to the functioning of the unsecured credit market in general. Sanchez (Citation2010) incorporates asymmetric information and costly screening into a model of consumer debt and bankruptcy to study the role of the IT revolution in the transformation of the unsecured credit market. These studies, however, focus only on the lending stage when investigating the effect of the extensive use of IT on adverse selection and information asymmetry.
By developing a novel model of consumer default in which debt collection plays a vital role in sustaining risky lending, Drozd and Serrano-Padial (Citation2017) explore the effects of IT progress on the collection of delinquent consumer debt. Their model links IT improvements to debt collection and the supply of consumer credit. They argue that IT mitigates information asymmetry because it allows creditors to concentrate collection efforts on delinquent borrowers who are more likely to repay.
Now, the blockchain technology represents the latest developments in IT and associated financial innovations (Thakor, Citation2020). It provides a decentralised consensus and enlarges the contracting space through smart contracts. Cong and He (Citation2019) posit that smart contracts can mitigate information asymmetry and improve welfare and consumer surplus through enhanced entry and competition, while distributing information during consensus generation may encourage greater collusion.
2.2. The blockchain architecture literature
The blockchain technology broadly includes four aspects: peer-to-peer (P2P) network design (Li et al., Citation2018), encryption algorithms (Singh & Singh, Citation2016), distributed ledger technology (DLT) (Dai et al., Citation2018), and decentralised data storage (Ali et al., Citation2018). It may also involve distributed storage, machine learning, virtual reality (VR), and the Internet of things (IoT). However, the narrowly defined blockchain technology only involves data storage technology, databases, or file operations. From the perspective of architecture design (Gudgeon et al., Citation2020; Zhao et al., Citation2020), the blockchain can be divided into three levels: the protocol layer, the extension layer, and the application layer, which perform the functions of data verification, data dissemination, and data representation at the bottom of the blockchain, respectively ().
The protocol layer consists of storage and network layers, offering network programming, distributed algorithms, encryption signatures, and data storage. A blockchain-based data storage and access framework can remove the total dependence on a centralised repository. The files' metadata are stored in the blockchain, whereas the actual files are stored off-chain through distributed hash tables (DHTs) at multiple locations using a peer-to-peer network in this framework (Ali et al., Citation2018). Recognition of the unique challenges of blockchain programming has inspired developers to create languages to encapsulate domain-specific script codes (Coblenz, Citation2017), which is the basis for blockchain platforms, such as Ethereum, to facilitate transactions on a decentralised computing platform among parties that have not established trust.
The consensus and distributed algorithm play a crucial role in maintaining the safety and efficiency of the blockchain (Mingxiao et al., Citation2017). This idea comes is based on business logic, as it can greatly reduce the hardware circuit scale, easily implement pipeline processing, and improve the execution speed of the circuit. Using the right algorithm may significantly increase the performance of blockchain applications in the banking industry. In addition to decentralised ledgers and strong security, nonrepudiation is another important property of information security in blockchains (Fang et al., Citation2020). A digitally encrypted signature scheme effectively achieves nonrepudiation and plays a vital role in the bank risk mitigation framework.
The extension layer is responsible for the product development of the actual application of the blockchain technology to drive economic and social development. It consists of two categories based on different product lines. The first is intelligence technology that facilitates business transactions in various trading markets, such as smart contracts and tokens, and is an important channel for both fiat and crypto currencies. The second is used for processing literal data forms, such as documents, pictures, e-books, and videos.
The application layer produces new financial formats or service models to upgrade the financial system and promote the efficiency and quality of financial operations and services (Zhang et al., Citation2020). The blockchain technology enriches financial scenes through e-wallets, transaction URLs, major financial APIs, and the integration of online and offline channels. The ecosystem and financial service system based on blockchain applications can fully tap the potential needs of consumers and effectively alleviate traditional financial risk issues, such as information asymmetry.
2.3. The technology development and banking literature
With the integration of big data and the financial industry, the analysis of borrowers' historical transaction data by the banking industry has, to some extent, alleviated the problem of incomplete information endowment in investment decisions caused by information asymmetry. Further, the digital economy has reshaped the economic and social forms globally based on its characteristics of dataisation, intelligence, and platformisation, with the use of artificial intelligence (AI) data processing technology to predict economic and financial risks proving to be better than traditional economic intuition (Beutel et al., Citation2019). Moreover, recently, the growth rate of economic and social data penetration into bank credit and financial supervision have been gradually accelerating. Coupled with the catalytic effect of derivatives such as ‘financial accelerators’, the systemic and bank individual risks caused by improper processing of bank credit data cannot be underestimated.
As the core of AI and cloud computing, data are shaping new industrial developments, that is, the fourth industrial revolution. This trend is gradually accelerating with the deep integration of the IT and financial industries. While data bring opportunities to human society, they also pose risks. Issues surrounding data property rights, data security, and privacy protection have become increasingly prominent. The management differences in each data processing link have exposed issues at different levels, such as corporate management and social systems. Therefore, data processing technology has become the key to competition among major internet giants and has given birth to new proposition-data governance (Abraham et al., Citation2019). With the rapid accumulation of data capacity in the financial industry, the requirements for data quality and computing capabilities continue to increase, and the importance of data governance in the development of the banking industry has increased significantly.
As a new technology spawned by global technological innovation and industrial transformation, the blockchain technology has the potential to be adopted by financial organisations and banks for various applications (Syed et al., Citation2019). For instance, blockchain applications can help alleviate individual bank risk as well as systemic risk by providing industrial digital passes (Savelyev, Citation2018), smart contracts (Cong & He, Citation2019), and blockchain embedded credit systems for SMEs (Wang et al., Citation2019).
Wang et al. (Citation2019) argue that the alleviation of information asymmetry and credit rationing problems can be achieved through decentralised consensus and information distribution among all participants. In addition, many studies suggest that the integrated application of blockchain technology can play an important role in developing the digital economy, regulatory technology, and data security (Kaal, Citation2020).
However, some scholars worry that the blockchain technology may cause market anomalies and thus pose risks to the banking system (Cahill et al., Citation2020). This brings to our attention that the use of the blockchain technology in bank risk mitigation has yet to mature in concept and technology, as its development to be applied to the bank risk mitigation model is restricted by (1) transaction speed and (2) energy consumption. The transaction speed of a blockchain is one of the prime parameters through which the viability of a blockchain is gauged in the bank risk mitigation framework. A well-designed consensus mechanism needs to be efficient in meeting the high frequency of online transactions. For instance, existing electronic payment systems can handle over 50,000 transactions per second (TPS), while Bitcoin can only handle an average of approximately three TPS (Sun et al., Citation2021). One major concern that may inhibit or delay the widespread adoption of the blockchain technology is energy consumption (Truby, Citation2018).
3. Theoretical framework
We propose a simple two-period model to extend Drozd and Serrano-Padial (Citation2017) consumer default model and Cong and He (Citation2019) decentralised consensus and information model to consider consumers’ borrowing behaviour in a decentralised consensus platform.
The economy is populated by numerous banks and a continuum of consumers. Banks provide unlimited credit to consumers and divide consumers into two groups: consumers with digital identities and those without. Banks lend money directly to consumers through digital identities. For consumers without a digital identity, bank lending is a prior probability π(R)∈ (0,1), which is proportional to the consumers' social reputation capital R.
Consumers have the option of borrowing from banks to smooth their consumption. In period one, they use an exogenous income stream for consumption purposes and decide whether they will borrow from the bank to fill the gap between their consumption and exogenous consumption.
represents the debt amount and δ denotes the decision made by consumers to borrow (δ = 1) or not to borrow (δ = 0). In period two, consumers use an exogenous income stream
for consumption purposes. If they have borrowed from the bank in period one, the repayment amount is
where
is the debt interest. They need to decide whether to default (
or not to default (
Following the above settings, a typical consumer’s two-period utility function is:
(1)
(1)
where
and
represent the consumption of this typical consumer in the first and second periods, respectively.
is a discount factor. We assume that
is strictly increasing, strictly concave, and differentiable for all
The consumers’ decision to borrow
and the decision to default
maximise their utility:
(2)
(2)
In the first period, banks make lending decisions based on the characteristics of consumers without a digital identity. A smart contract for this debt contract is then automatically generated. In the second period, consumers decide to repay the debt of the previous period and form a real delivery status which is transmitted to a group of potential recorders associated with the blockchain protocol through the IoT sensor, where
indicates default. According to their own information, recorders
report
These reports form a compilation,
The recorder may choose to misreport information to maximise his or her own interest (i.e.,
). In this case, the consensus function is
(3)
(3)
where
is the probability of the recorder reporting the true information, which is nonnegative, and
If the borrower chooses to borrow in the first phase, the borrower will make the decision
of whether to repay the loan in the second phase, and a distributed consensus delivery state will be formed according to the blockchain protocol and the report of each recorder, that is:
(4)
(4)
The constraint condition of the objective function of consumer with digital identity is:
(5)
(5)
where
is the scope of digital identity establishment and
represents the opportunity cost of having a digital identity, which is a positive linear function with
Its value also includes the cost of digital identity establishment and the opportunity cost of the digital alliance chain in terms of the social reputation cost; its value is greater than the reputation capital
of borrowers without digital identity. The constraint conditions of the objective function of consumer
without digital identity are as follows:
(6)
(6)
where
represents the measurable function. Since
is a strictly increasing linear function, the utility situations faced by consumer
with digital identity and consumer
without digital identity under two different decisions can be expressed as
(7)
(7)
The effects of the three cases in EquationEquation (7)(7)
(7) are denoted as
and
respectively.
(8)
(8)
The effects of the three cases in EquationEquation (8)(8)
(8) are denoted as
and
respectively. We attempt to analyze the risk difference of having a digital identity in the case of successful loans and how to construct a digital identity chain to reduce default risk.
When and
and
the consumer will choose to repay the debt on time and vice versa.
represents the cost of the consumer with digital identity colluding with the recorder to change the consensus, which is in a linearly increasing relationship with the number of recorders
It can be concluded that if the opportunity cost of digital identity and the cost of changing the consensus are sufficiently high, the conditions of the consumer's repayment will not be difficult to meet. In other words, the greater the scope of digital identity construction and the more blockchain consensus records there are, the less likely the borrower will default. When the digital identity range
or recorder
is sufficiently large, borrowers rarely default. Therefore, the amount that the borrower needs to repay for the loan in the second phase is the critical value for the construction of the digital identity penalty mechanism and the blockchain consensus mechanism.
We also consider the impact of having a digital identity on bank credit risk in the theoretical framework. When and
and
the borrower chooses to repay the loan, and vice versa.
denotes the cost of colluding with the recorder to change the consensus about the consumer without digital identity. Owing to the openness of their digital identity information in the blockchain consensus chain, digital identity owners must bear greater costs if they want to collude with the recorder. Therefore, if the owner of digital identity wants to default, he or she must bear a higher cost, and his or her default risk is correspondingly smaller.
shows the default risk of borrowers with digital identities and those without in the second period. The exiting evidence suggests that borrowers with digital identities have a relatively low probability of default (Allen et al., Citation2020). Simultaneously, if the cost of defaulting or changing the agreement is less than the amount to be repaid in the second instalment, the probability of default rises sharply. Based on the bank risk mitigation framework, two important factors (i.e. decentralised digital identity and encryption technology) are further explored in the next section.
4. Decentralised digital identity and encryption technology
4.1. Decentralised digital identity
In the era of big data, digitisation continues to penetrate our lives. Private firms, government agencies, and institutions routinely gather vast amounts of digitised personal information about their customers and clients (Sarwate & Chaudhuri, Citation2013).
Much of this information is private or sensitive, particularly in the financial industry. For instance, due to the difference in data origin and dissemination, various false and deceptive information is flooded, which makes banks encounter serious interference in the process of customer credit evaluation. Additionally, the proliferation and manipulability of data undermine data trust. Banks may incur huge costs due to the value screening of massive data, while data disasters may hinder bank customer credit evaluation.
Compared to traditional identity systems, the digitisation and networking of personal information have greatly improved the overall social efficiency and maximised the release of user value so that the government, service providers, users, and other parties can benefit from it. However, the development of digital identity is still subject to many constraints. A key technological challenge for the future is how to design systems and processing techniques for drawing inferences from these large-scale data while maintaining the privacy and security of private data and individual identities.
If digital identities are placed on the underlying blockchain, it can effectively realise digital identity using authority management and identity verification (Bakre et al., Citation2017). Encryption protection can be carried out using asymmetric encryption keys to realise private key control ownership. Users can provide public keys for the authorisation of major applications while preventing privacy leakage. In other words, while the user has the right to control personal information, it also protects the security of the user data.
A decentralised digital identity is a basic capability and a precondition for business development (Goodell & Aste, Citation2019)). We attempt to elaborate the effects of decentralised digital identities on bank credit risk mitigation from the three dimensions of ‘length’, ‘depth’, and ‘breadth’. The basic framework of content bearing, technical system, and scene coverage is shown in .
The ‘length’ of distributed digital identities refers to the common superposition of digital identities, protocols, roles, data, and scenarios. The use of distributed digital identities to establish secure communication channels is a string of characters defined by the W3C Decentralized Identifier (DID) specification organisation, which is the starting point of distributed digital identities. Based on DID, the International Organization for Standardization has designed a series of protocols with complete functions and clear semantics, defining online identity description documents (Document), and the process of generating, presenting, verifying, and destroying credentials (Credential), covering identity, and the complete life cycle of credential management. Three important roles are defined in the agreement: authenticator, user, and verifier, which constitute the core ternary relationships. Finally, distributed data identities can be used in business, social governance, and digital life. In the banking scenario, the user encrypts copyright information such as ‘applicant + release time + release content’ and uploads it. The copyright information is used for the unique blockchain ID, which is equivalent to having an electronic ID card.
The ‘depth’ of distributed digital identity refers to the technical support that can be provided in terms of user control, distributed verification, and privacy protection. Compared to traditional identity systems, distributed digital identities do not control data islands but give the users the control of data. The users choose different scenarios according to their wishes in terminal tools, data trust management, and secure exchange mechanisms. Second, the distributed technology system includes the distributed public key infrastructure (DPKI) system, DLT represented by blockchain, and distributed storage technology. Finally, based on an in-depth algorithm support, the distributed digital identity system uses distributed consensus algorithms, cutting-edge cryptographic algorithms, polymorphic signatures, and other multiple interactive algorithms.
The application and satisfaction of bank blockchain scenarios are the footholds and the ‘breadth’ of decentralised digital identities. As individuals, with different scenarios and regions of economic activities, people’s identities, attributes, and credentials are different, and the credit and value of social people are replanned. The judgment standards of certifiers and verifiers of different functional departments strengthen the globalisation and decentralisation of social credit networks. The distributed digital identity contributes to the construction of the credit system, the value of data, the cost of circulation, and privacy protection.
Based on open-source interoperability and data security, it effectively realises interregional, intersubject, and intermarket interconnections. Second, the establishment of environmental frameworks such as now your customer (KYC) and anti-money laundering (AML) protocols and the DID alliance is conducive to the efficient operation of the banking business, enabling the on-chain and off-chain data to interact well. In particular, decentralised intelligent KYC information security distributed storage is conducive to the optimisation of the customer credit management system by banks, allowing participants to effectively use existing relationships and necessary data through various API authentications to create a secure ‘trust network’ to avoid disclosing sensitive data, thereby achieving data governance from the perspective of data authorisation.
4.2. Encryption technology
The encryption technology is a process in which plain-text information is converted into cipher-text information through an algorithm. The recipient of the information can decrypt the cipher-text information with a key to obtain the plain-text information. The key is divided into public and private keys, which appear in pairs (Wang et al., Citation2018). For instance, the public key is used to confirm the receipt of the transaction, which is similar to the ‘receiver’ in the banking transaction, while the private key is used for digital signatures to confirm the ownership of the transaction, similar to the ‘signature’ in the banking transaction. The basic encryption algorithm is mainly divided into power encryption and elliptic curve encryption; Bitcoin adopts the latter encryption principle. This type of encryption algorithm is to realize the transaction from one party to the other and vice versa.
Simultaneously, according to whether the encryption and decryption keys are the same, the algorithm can be divided into symmetric, asymmetric, and a combination of symmetric and asymmetric encryption (Li et al., Citation2019). First, symmetric encryption means that the keys for encryption and decryption are the same. Second, asymmetric encryption uses a key pair: a public key and a private key. The private key can calculate the public key; however, the public key cannot obtain the private key. The private key encrypts information, whereas the corresponding public key can decrypt it; conversely, the public key encrypts information, and the corresponding private key can also be unlocked. Notice that digital signatures and data encryption technologies are two different uses of asymmetric encryption. Finally, symmetric and asymmetric encryption can be divided into two stages: stage one which uses asymmetric encryption to encrypt the key and pass it to the receiver and stage two which uses symmetric encryption to encrypt and decrypt the plain-text information. The process is illustrated in .
The blockchain application of encryption technology in bank risk mitigation effectively prevents operational risks from data tampering, sharing digital fingerprints, and sharing original data. In terms of preventing data tampering, asymmetric encryption is technically more appropriate for practical purposes. For example, in Ethereum, users need to obtain the private key to encrypt the transaction data to generate a signature, and then send the transaction data with the signature. Owing to the inefficiency of asymmetric encryption, this process is not suitable for encrypting large amounts of data.
The system generally uses the information digestion algorithm to generate information fingerprints, and then generates digital signatures based on shorter information fingerprints (Aune et al., Citation2017). Shared digital fingerprints are digital fingerprints that use information digestion algorithms to generate shared data and upload them to the chain. All parties in the chain use traditional methods to obtain shared data, and finally, each party uses the digital fingerprint on the chain to verify whether the data are correct. The shared digital fingerprint mechanism ensures on-chain storage and efficient reading and writing, and effectively guarantees the processing of big data for bank customer transactions from the perspective of data governance and supervision functions to prevent customer transaction risks.
Given the characteristics that original data can be shared with multiple visitors and the low efficiency of directly using asymmetric encryption, symmetric encryption of data information is adopted to transmit symmetric keys. The data issuer uses symmetric encryption to encrypt the original data. When it needs to be shared with a recipient, the symmetric encryption key is encrypted with the recipient’s public key, and then the data cipher-text and the symmetric key cipher-text are sent to the recipient. The person first uses his or her private key to solve the symmetric key, and then uses the symmetric key to solve the original data.
5. Applications
Through the certification of the program code, the blockchain technology effectively incorporates the operational risk and credit risk assessment and decision-making of the banking industry in the transaction process into a specific implementation process. Recently, the individual credit evaluation of the information society has transformed into a shared credit evaluation model based on technology and platform resources. This section discusses how the blockchain technology helps prevent bank risks in the banking sector from two aspects: payment system and digital currency.
5.1. Blockchain payment system
As economic behaviours that frequently appear in the market, consumption and investment play an important role in bank payment and settlement services. The blockchain technology can help reduce the cost of reconciliation and dispute resolution between financial institutions due to its own characteristics of nontamperability and traceability (Zhong et al., Citation2019). Additionally, it can realise the decentralisation of settlement business in the form of a bank settlement business. Moreover, as it uses a distributed accounting method to make the digital transaction structure immutable, it can greatly increase the processing speed of the bank settlement business and reduce transaction costs and credit risks (Wang et al., Citation2018).
In terms of bank payment risks, the blockchain technology uses encryption to protect transaction records, with the ledger being difficult for hackers to invade, thus effectively preventing the data risk of authority intrusion. Furthermore, the distributed structure makes the risks divergent, that is, when one of the intermediate links has a problem, the others are not affected and can operate normally, thereby effectively preventing the spread of risks and the possibility of further evolving into a banking crisis.
Blockchain payments have an important application in interbank and cross-border payments in the banking field. On the one hand, in traditional interbank payments, each bank has its own independent account book, and each link must be calculated and checked separately. On the other hand, Blockchain payments establish a consortium chain, and each bank only needs to prepare a reserve account, which can save intermediate transaction links. Thereby, more funds can be freed up for other transactions, and the risk of bank system paralysis caused by cumbersome links and huge workload can be further reduced.
Further, traditional cross-border payments can cause bank payment risks in two ways. First, banknotes must be exchanged. However, the banknotes of both parties to the transaction may not have a strong credit value as they depend on national credit and the internal political environment. Differentiation, in turn, causes information asymmetry in microbank cross-border payments and further strengthens the transmission of financial crises from channels such as supply chain finance and international trade relations. Second, the intermediate settlement link is complicated, with the information and data of both parties in the transaction having their own blind spots, which lengthens the transaction. This cycle can disturb the balance of the bank's capital chain. Conversely, since blockchain payments in cross-border payment scenarios use digital currencies for payment, this peer-to-peer payment mechanism completely solves the problem of legal currency swaps and long settlement cycles, thus effectively preventing the bank risks.
5.2. Digital currency
Blockchain-based digital asset issuance includes government- and nongovernment-led issuances, with the specific methods comprising centralisation and disintermediation. Among them, the government-led digital source chain represents a certain country's public service digital assets, while the nongovernment-issued digital source chain represents a specific application of digital assets. Banks are the main channel for the government's macro monetary policy regulation, while asset blockchains essentially monitor financial risks, thereby reducing the probability of bank risks. Digital currency is an encrypted digital string representing a specific amount issued by the central bank guaranteed and signed, including the most basic number, amount, signature of the owner and issuer.
On the one hand, according to the classic financial theory, currency is an important tool for regulating the economy. Since it is currently realised through the issuance of paper currency and paper currency exists physically, it is difficult for a central bank to grasp the specific form, scale, and use of the paper currency issued by it and can only have a very rough estimate. Such estimates often lead to errors in the monetary policy.
On the other hand, the issuance of digital currency must be authenticated by the registration centre. The serial number is the unique identifier of digital currency and is used as a digital currency index. The registration centre not only records the digital currency and the corresponding user identity and completes the ownership registration, but also records the flow and completes the registration of the entire process of digital currency generation, circulation, inventory verification, and demise. The registration centre is constructed based on the traditional centralised method, that is, a digital mining centre with a new concept, while the authentication centre is an important part of the controllable and anonymous design of digital currencies. The authentication of financial institutions or high-end users can use public key infrastructure (PKI), whereas the authentication of low-end users can use identity-based cryptography (IBC). The real-time situation of currency can be accurately tracked according to the digital currency operating mechanism, thus developing a more accurate understanding of monetary policy.
6. Conclusions
6.1. Theoretical contributions
The primary theoretical contribution of this study is the development of a simple two-period model to consider consumers’ borrowing behaviour in a decentralised consensus and information distribution platform.
First, within the bank risk mitigation framework based on this model, decentralised digital identities and encryption technology are the most important factors for attaining market equilibria between decentralised consensus and information distribution. Regarding the impact of having a digital identity on bank credit risk, the greater the scope of digital identity construction and the more blockchain consensus records there are, the less likely that the borrower will default. Owing to the information disclosure of their digital identity in a decentralised consensus platform, digital identity owners must bear greater costs if they want to collude with the recorder.
Second, we shed new light on bank risk mitigation from a micro perspective. We divide consumers into consumers with digital identity and those without digital identity, then investigate the relationship between digital identity and the opportunity cost of digital scene construction. We also explore the importance of social capital and credit prior probability by considering the trade-off between decentralised consensus and information distribution featured by blockchain applications.
6.2. Managerial implications
Our study provides meaningful practical implications for bankers and policy regulators. The findings that decentralised digital identity and encryption technology are the most important factors in a blockchain-based bank risk mitigation framework provide particularly useful and practical insights to bankers to help them better understand consumers’ borrowing behaviour and decisions to default. Thus, bankers should consider increasing investment in the blockchain payment system and focus on the construction of digital identity and improving encryption technology.
These results also suggest that if policy regulators want to strengthen risk mitigation in the banking system, they should be more acute in regulating blockchain-based applications, such as cryptocurrencies, tokens, and smart contracts.
6.3. Limitations and future research
Despite the aforementioned significant theoretical contributions, this study has the following limitations. First, the model proposed in the present study is a simple two-period model that considers only banks and consumers. Although it derives some meaningful results, it may not serve well in the more complex financial market. Thus, a multiperiod model that considers more market participants will be our future research agenda.
Second, while the theoretical model effectively illustrates the consumer’s borrowing behaviour within a decentralised consensus and information distribution framework, conducting empirical analysis using data of consumers with and without digital identity could help us better understand the effect. Thereby, future research can build on this study and extend our model.
Disclosure statement
No potential conflict of interest was reported by the authors.
Additional information
Funding
References
- Abraham, R., Schneider, J., & Vom Brocke, J. (2019). Data governance: A conceptual framework, structured review, and research agenda. International Journal of Information Management, 49, 424–438. https://doi.org/10.1016/j.ijinfomgt.2019.07.008
- Allen, F., Gu, X., & Jagtiani, J. (2020). A survey of fintech research and policy discussion [FRB of Philadelphia Working Paper No. 20–21]. https://ssrn.com/abstract=3622468
- Ali, S., Wang, G., White, B., & Cottrell, R. L. (2018). A blockchain-based decentralized data storage and access framework for pinger [Paper presentation]. Paper Presented at the 2018 17th IEEE International Conference on Trust, Security and Privacy in Computing and Communications/12th IEEE International Conference on Big Data Science and Engineering (TrustCom/BigDataSE).
- Athreya, K., Tam, X. S., & Young, E. R. (2012). A quantitative theory of information and unsecured credit. American Economic Journal: Macroeconomics, 4(3), 153–183.
- Aune, R. T., Krellenstein, A., O’Hara, M., & Slama, O. (2017). Footprints on a blockchain: Trading and information leakage in distributed ledgers. The Journal of Trading, 12(3), 5–13. https://doi.org/10.3905/jot.2017.12.3.005
- Bakre, A., Patil, N., & Gupta, S. (2017). Implementing decentralized digital identity using blockchain. International Journal of Engineering Technology Science and Research, 4(10), 379–385.
- Beutel, J., List, S., & von Schweinitz, G. (2019). Does machine learning help us predict banking crises? Journal of Financial Stability, 45, 100693. https://doi.org/10.1016/j.jfs.2019.100693
- Cahill, D., G. Baur, D., (Frank) Liu, Z., & W. Yang, J. (2020). I am a blockchain too: How does the market respond to companies’ interest in blockchain? Journal of Banking & Finance, 113, 105740. https://doi.org/10.1016/j.jbankfin.2020.105740
- Capponi, A., & Chen, P. C. (2015). Systemic risk mitigation in financial networks. Journal of Economic Dynamics and Control, 58, 152–166. https://doi.org/10.1016/j.jedc.2015.06.008
- Clair, R. T. (1992). Loan growth and loan quality: Some preliminary evidence from Texas banks. Economic Review, Federal Reserve Bank of Dallas, Third Quarter, 1992, 9–22.
- Coblenz, M. (2017). Obsidian: A safer blockchain programming language [Paper presentation]. Paper Presented at the 2017 IEEE/ACM 39th International Conference on Software Engineering Companion (ICSE-C). https://doi.org/10.1109/ICSE-C.2017.150
- Cong, L. W., & He, Z. (2019). Blockchain disruption and smart contracts. The Review of Financial Studies, 32(5), 1754–1797. https://doi.org/10.1093/rfs/hhz007
- Cong, L. W., Li, Y., & Wang, N. (2021). Tokenomics: Dynamic adoption and valuation. The Review of Financial Studies, 34(3), 1105–1155. https://doi.org/10.1093/rfs/hhaa089
- Dai, M., Zhang, S., Wang, H., & Jin, S. (2018). A low storage room requirement framework for distributed ledger in blockchain. IEEE Access, 6, 22970–22975. https://doi.org/10.1109/ACCESS.2018.2814624
- Dashottar, S., & Srivastava, V. (2021). Corporate banking – Risk management, regulatory and reporting framework in India: A blockchain application-based approach. Journal of Banking Regulation, 22(1), 39–51. https://doi.org/10.1057/s41261-020-00127-z
- Drozd, L. A., & Serrano-Padial, R. (2017). Modeling the revolving revolution: The debt collection channel. American Economic Review, 107(3), 897–930. https://doi.org/10.1257/aer.20131029
- Eisenberg, L., & Noe, T. H. (2001). Systemic risk in financial systems. Management Science, 47(2), 236–249. https://doi.org/10.1287/mnsc.47.2.236.9835
- Fang, W., Chen, W., Zhang, W., Pei, J., Gao, W., & Wang, G. (2020). Digital signature scheme for information non-repudiation in blockchain: A state of the art review. EURASIP Journal on Wireless Communications and Networking, 2020(1), 1–15. https://doi.org/10.1186/s13638-020-01665-w
- Goodell, G., & Aste, T. (2019). A decentralized digital identity architecture. Frontiers in Blockchain, 2, 17. https://doi.org/10.3389/fbloc.2019.00017
- Gudgeon, L., Moreno-Sanchez, P., Roos, S., McCorry, P., & Gervais, A. (2020). SoK: Layer-two blockchain protocols [Paper presentation]. Paper Presented at the International Conference on Financial Cryptography and Data Security.
- Hasan, I., Jackowicz, K., Kowalewski, O., & Kozłowski, Ł. (2013). Market discipline during crisis: Evidence from bank depositors in transition countries. Journal of Banking & Finance, 37(12), 5436–5451. https://doi.org/10.1016/j.jbankfin.2013.06.007
- Hou, X., Gao, Z., & Wang, Q. (2016). Internet finance development and banking market discipline: Evidence from China. Journal of Financial Stability, 22, 88–100. https://doi.org/10.1016/j.jfs.2016.01.001
- Kaal, W. A. (2020). Blockchain solutions for agency problems in corporate governance. In Balachandran, K. R. (ed.), Information for Efficient Decision Making Big Data, Blockchain and Relevance (pp. 313–329). World Scientific Publishing Co.
- Li, H., Tian, H., Zhang, F., & He, J. (2019). Blockchain-based searchable symmetric encryption scheme. Computers & Electrical Engineering, 73, 32–45. https://doi.org/10.1016/j.compeleceng.2018.10.015
- Li, J., & Zinna, G. (2014). On bank credit risk: Systemic or bank specific? Evidence for the United States and United Kingdom. Journal of Financial and Quantitative Analysis, 49(5–6), 1403–1442. https://doi.org/10.1017/S0022109015000022
- Li, Z., Barenji, A. V., & Huang, G. Q. (2018). Toward a blockchain cloud manufacturing system as a peer to peer distributed network platform. Robotics and Computer-Integrated Manufacturing, 54, 133–144. https://doi.org/10.1016/j.rcim.2018.05.011
- Livshits, I., Mac Gee, J. C., & Tertilt, M. (2016). The democratization of credit and the rise in consumer bankruptcies. The Review of Economic Studies, 83(4), 1673–1710. https://doi.org/10.1093/restud/rdw011
- Mingxiao, D., Xiaofeng, M., Zhe, Z., Xiangwei, W., & Qijun, C. (2017). A review on consensus algorithm of blockchain [Paper presentation]. IEEE International Conference on Systems, Man, and Cybernetics (SMC) (pp. 2567–2572). IEEE.
- Narajabad, B. N. (2012). Information technology and the rise of household bankruptcy. Review of Economic Dynamics, 15(4), 526–550. https://doi.org/10.1016/j.red.2012.06.002
- Nijskens, R., & Wagner, W. (2011). Credit risk transfer activities and systemic risk: How banks became less risky individually but posed greater risks to the financial system at the same time. Journal of Banking & Finance, 35(6), 1391–1398. https://doi.org/10.1016/j.jbankfin.2010.10.001
- Osmani, M., El-Haddadeh, R., Hindi, N., Janssen, M., & Weerakkody, V. (2021). Blockchain for next generation services in banking and finance: Cost, benefit, risk and opportunity analysis. Journal of Enterprise Information Management, 34(3), 884–899. https://doi.org/10.1108/JEIM-02-2020-0044
- Reinhart, C. M., & Rogoff, K. S. (2009). This time is different: Eight centuries of financial folly. Princeton University Press.
- Sanchez, J. M. (2010). The IT revolution and the unsecured credit market [Federal Reserve Bank of St. Louis Working Paper No. 2010-022A].
- Sarwate, A. D., & Chaudhuri, K. (2013). Signal processing and machine learning with differential privacy: Algorithms and challenges for continuous data. IEEE Signal Processing Magazine, 30(5), 86–94.
- Savelyev, A. (2018). Some risks of tokenization and blockchainizaition of private law. Computer Law & Security Review, 34(4), 863–869. https://doi.org/10.1016/j.clsr.2018.05.010
- Singh, S., & Singh, N. (2016). Blockchain: Future of financial and cyber security [Paper presentation]. Paper presented at the 2016 2nd International Conference on Contemporary Computing and Informatics (IC3I). https://doi.org/10.1109/IC3I.2016.7918009
- Sun, Y., Xue, R., Zhang, R., Su, Q., & Gao, S. (2021). RTChain: A reputation system with transaction and consensus incentives for e-commerce blockchain. ACM Transactions on Internet Technology, 21(1), 1–24. https://doi.org/10.1145/3430502
- Syed, T. A., Alzahrani, A., Jan, S., Siddiqui, M. S., Nadeem, A., & Alghamdi, T. (2019). A comparative analysis of blockchain architecture and its applications: Problems and recommendations. IEEE Access., 7, 176838–176869. https://doi.org/10.1109/ACCESS.2019.2957660
- Thakor, A. V. (2020). Fintech and banking: What do we know? Journal of Financial Intermediation, 41, 100833. https://doi.org/10.1016/j.jfi.2019.100833
- Truby, J. (2018). Decarbonizing Bitcoin: Law and policy choices for reducing the energy consumption of blockchain technologies and digital currencies. Energy Research & Social Science, 44, 399–410. https://doi.org/10.1016/j.erss.2018.06.009
- Wang, B., Sun, J., He, Y., Pang, D., & Lu, N. (2018). Large-scale election based on blockchain. Procedia Computer Science, 129, 234–237. https://doi.org/10.1016/j.procs.2018.03.063
- Wang, R., Lin, Z., & Luo, H. (2019). Blockchain, bank credit and SME financing. Quality & Quantity, 53(3), 1127–1140. https://doi.org/10.1007/s11135-018-0806-6
- Wang, X., Xu, X., Feagan, L., Huang, S., Jiao, L., & Zhao, W. (2018). Inter-bank payment system on enterprise blockchain platform [Paper presentation]. 11th International Conference on Cloud Computing (CLOUD) (pp. 614–621). IEEE.
- Zhang, L., Xie, Y., Zheng, Y., Xue, W., Zheng, X., & Xu, X. (2020). The challenges and countermeasures of blockchain in finance and economics. Systems Research and Behavioral Science, 37(4), 691–698. https://doi.org/10.1002/sres.2710
- Zhao, H., Zhang, M., Wang, S., Li, E., Guo, Z., & Sun, D. (2020). Security risk and response analysis of typical application architecture of information and communication blockchain. Neural Computing and Applications, 33(13), 7661–7671.
- Zheng, Y. (2020). Does bank opacity affect lending? Journal of Banking & Finance, 119, 105900. https://doi.org/10.1016/j.jbankfin.2020.105900
- Zhong, L., Wu, Q., Xie, J., Li, J., & Qin, B. (2019). A secure versatile light payment system based on blockchain. Future Generation Computer Systems, 93, 327–337. https://doi.org/10.1016/j.future.2018.10.012