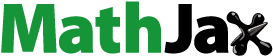
Abstract
Freight and passenger transportation profoundly impacts environmental degradation due to the higher fossil fuel consumption and respective emissions. Therefore, this study explores the impact of freight and passenger transportation on environmental pollution (particulate matter 2.5(PM 2.5)) in BRICS countries using annual data from 1990 to 2018. We employed various advanced econometric approaches to handle issues arising from panel data, such as the presence of unit root, cross-sectional dependency, structural break, and parameters heterogeneity. The overall results show that freight and passenger transportation significantly contribute to a higher concentration of PM 2.5; however, the impact of freight transportation is almost double that of passenger transportation. Similarly, economic growth also caused higher environmental pollution. Moreover, this study confirms the presence of the Environmental Kuznets Curve hypothesis in the long run. These results provide valuable suggestions to achieve a sustainable environment.
1. Introduction
The transportation sector is considered as an important segment of the economy, and it impacts all aspects of human life, research, education, entertainment, culture, trade, defense, and so on (Baloch & Suad, Citation2018; Maparu & Mazumder, Citation2017; Sohail et al., Citation2021; Sun et al., Citation2021). This driving sector plays an imperative part in the economic growth of the nations, but unfortunately, it is also the main source of energy ingesting and contributes to lower environmental quality (Raza et al., Citation2019; Sharif et al., Citation2020; Van Fan et al., Citation2018). The rapid development of the transport sector has contributed to the socio-economic improvement of the nation, and demand for transport services is anticipated to rise, leading to higher emissions and causing global warming (Liu et al., Citation2020; Mustapa & Bekhet, Citation2016). The transport industry is the world’s second-largest energy consumer after the industrial sector, and it consumes more than 30% of the world’s total energy consumption ( Danish, Baloch, & Suad, Citation2018; Giannakis et al., Citation2020; Liang et al., Citation2017; An et al., Citation2021a ).
The transport sector mainly depends on fossil fuels, and as a result of the increase in atmospheric temperature, green transportation is considered one of the main alternatives for countries around the world (Chien et al., Citation2021; Danish et al., 2018; Razzaq et al., Citation2021b). Worldwide carbon dioxide emissions (CO2 emanations) have increased quickly over a long time due to increasing energy utilization boosted by fast economic growth in global economies. This growth leads to substantial changes in temperature and precipitation designs and has had deliberate environmental consequences, such as the increased occurrence of droughts, floods, and storms and rise in worldwide temperature and sea level (Safaai et al., Citation2011; Stern, Citation2009). The continuous growth of transport sector and its effects on the environment attract the attention of policymakers in sustainable transportation (Amin et al., Citation2020).
The transportation sector in any country leads to increase the economic growth, due to rise in economic progress the countries around the globe upsurges their trade as a result of which there will be more use of freight and passenger transportation modes (Godil et al., Citation2020; Sobrino & Monzon, Citation2014). The freight segment is an enormous supporter of discharges of greenhouse gas emissions (GHG), relieving its adverse consequence on the climate, which is imperative for sustainable development (Jofred & Öster, Citation2011). Freight transportation and logistics act as the artery of the national economy (Luo et al., Citation2016). Freight is transported nowadays by planes, trucks, ships, rail lines, predominately fueled by petroleum-driven powers (Muratori et al., Citation2017). Among various vehicle modes, road transportation has alluded as a significant supporter of emissions. Talbi (Citation2017) contends that road transport primarily depends on non-renewable energy sources, which is the main supporter of the transport area's CO2 emanations (Sharif et al., Citation2019).
According to Air Transportation Action Group (Citation2019), after road transportation, the aviation segment is the significant sector for releasing gasses into the atmosphere. The gases released in the atmosphere from the aeronautics sector are more dangerous because the quality of air will be affected at the surface. Most gasses are released near the surface environment, whereas the other half is released over 6000 m (Balkanski et al., Citation2010). The transport division assumes a central part in a globalized economy as it permits goods to be exchanged, mobility for individuals, social and economic development and is one of the reasons for suburbanization among urban areas (Berg & Ihlström, Citation2019; Danish et al., 2018; García-Olivares et al., Citation2018; Krautzberger & Wetzel, Citation2012). The carbon emanation emerges from different methods of transportation; for example, road transportation, rail transportation, inland delivery, freight frameworks, and aviation (Aggarwal & Jain, Citation2016; Hoffrichter et al., Citation2012; Kim & Van Wee, Citation2014; Šimenc, Citation2016; Zhou et al., Citation2016). Energy utilization unfavorably influences the climate and hence brings about expanding CO2 emissions (Talbi, Citation2017).
At the point when ecological contamination has become a major issue and fundamentally adds to a worldwide temperature alteration, a practical zero-emission transportation framework becomes important (Amin et al., Citation2020). As of late, public concern over ozone-depleting substance emanations from the transportation sector has increased (Cui & Li, Citation2015). Controlling the carbon dioxide emanations from transportation is significant for sustainable development. Numerous nations and regions have forced CO2 emissions caps on the transportation division. The fundamental methodologies have been depicted as follows: energy and innovation development, policy execution, transportation structure adjustment, and different techniques (Abrell, Citation2010; Banister et al., Citation2011; Grahn et al., Citation2009; Knittel, Citation2012; Waisman et al., Citation2013).
In the last 30-year time period, the amount of dangerous industrial pollutants gasses released in the environment are less in the developed countries, but they are increased in the developing world (Barbier, Citation1997). This type of association is called the Environmental Kuznets Curve (EKC); the KEC hypothesis revealed an inverse U-shaped relationship between numerous indicators of environmental degradation and the amount of per capita income (Dinda, Citation2004). In 1990s, the KEC became a reference to describe the association of how various forms of environmental pollutants are released in the atmosphere due to the economic growth of a country (Beckerman, Citation1992). Most of the developing countries are unable to protect their environment from these dangerous pollutants, but they are trying to determine the level of their income at which they are capable of bearing the production methods that respect the environment (Cherniwchan, Citation2012). Therefore, pollution is observed as a byproduct of industrial production activities.
In this era, the notion of BRICS (Brazil, Russia, India, China, and South Africa) countries is becoming popular more and more in academia, and public media and their importance in the global economy continue to increase (Liu et al., Citation2020; Scerri & Lastres, Citation2020). The BRICS counties are referred to as five major emerging economies, occupying 26% of the total world land and 42% of the world population (He et al., Citation2020). The BRICS countries represent a combination of developing economies with a large population and a significant share of economic output globally (Chen & De Lombaerde, Citation2014). The total GDP growth of the BRICS counties was U.S. dollars 188.76 billion in 2017, equal to 23.3% of the world total (He et al., Citation2020). Goldman (Citation2003) determined that in less than 40 years at one end, the BRICS countries played a more important role in improving the world economy than G6 (United Kingdom, United States, France, Japan, Italy, and Germany). On the other hand, the size of their economy may account for more than half of G6 countries by 2025.
The World Bank statistics show that from 2008 to 2018, the nominal GDP of the BRICS countries increases from 11.8% to 22.3% of the world’s total. As a result, the proposed share of the BRICS countries will rise to 37.7% in world GDP, respectively (He et al., Citation2020). BRICS countries are playing an essential role in increasing the world economy, but at the same time, they have received global attention due to their greater use of energy. The resulting pollution problems, likewise CO2 emissions and the other dangerous gasses, causes global warming and increased atmospheric temperature. Arrow et al. (Citation1996) contended that a country would achieve sustainability only if its economic growth is attuned to environmental quality. In 2013 during the 5th BRICS summit, the BRICS countries signed an agreement on climate cooperation and the green economy in order to ensure the technical exchange to overcome the negative effect of climate change on developing countires and financial support as well (Cowan et al., Citation2014; Lingyan et al., Citation2021; Razzaq et al., Citation2021a). The relationship between economic growth and air pollution in BRICS countries is debatable due to their rapid economic growth (Liu et al., Citation2020). The various gasses that cause air pollution are carbon dioxide (CO2), sulfur dioxide (S2O), suspended particulate matter (PM2.5 or PM10, with diameters of 2.5 or 10 μm, respectively), Nitrous oxides (NOx) (Bagoulla & Guillotreau, Citation2020; Ling et al., Citation2021; Shahzad et al., Citation2021).
This study contributes to the empirical literature by exploring the impact of freight and passenger transportation on air pollution in BRICS countries. Prior studies mainly draw the influence of transportation sector and CO2 emissions as a determinant for air pollution while ignoring particulate matter (PM2.5), which is the most relevant measure for air pollution. Moreover, the BRICS countries are showing exponential growth in transport services and environmental issues, which is not yet explored in the recent scenario. Apart from that, existing studies mainly rely on traditional panel estimators, which fail to address complexities arising from multiple structure breaks and parameter heterogeneity. To overcome these issues, this study applied contemporary empirical estimation techniques to produce reliable outcomes.
2. Literature review
The transport sector is the second-largest energy consumer sector globally and profoundly contributes to the economy more explicitly in developing nations (He et al., Citation2021; Ozturk & Acaravci, Citation2013; Sharif et al., Citation2020). Though, in spite of the part of the transport sector in economic growth and development, it may be a threat to global warming (Danish et al., 2018; Solaymani, Citation2019). The persistent development of transport sector around the world also leads to increase the release of dangerous gases like CO2 emissions in the air and this will be give an alarming signal to the policy makers in order to make transportation sector more sustainable (Amin et al., Citation2020). Analysts have appeared that there’s a solid connection between economic growth and CO2 emissions (Begum et al., Citation2015).
Raza et al. (Citation2019) investigated a positive and significant association of energy consumption and economic growth on CO2 emissions. Some earlier studies revealed that the transportation sector affects the environment differently at different places, and it all depends upon the procedures and the methods used in that sector. Consequently, these research studies do not give clear and definite associations (Ahmed et al., Citation2020; Alshehry & Belloumi, Citation2017; Du et al., Citation2019; Kharbach & Chfadi, Citation2017; Sohail et al., Citation2019). Chandran and Tang (Citation2013) propose that energy consumption in transport sector and economic growth significantly affect CO2 emissions over the long run in ASEAN-5 nations. Shahbaz et al. (Citation2015) investigated that both transport energy utilization and road infrastructure cause to upsurge pollution in Tunisia. Moreover, a bidirectional causality has been investigated between transport-value added, transport energy utilization with CO2 emanations.
Most of the prior researchers used various econometric models and techniques to analyze energy planning. Likewise, Marrero (Citation2010) affirmed that the emission of dangerous gasses like CO2 may be decreased and that all depends upon the type of fuel used in the vehicles and moreover, if we use a large amount of renewable energy sources, this will also help in the more significant reductions of dangerous emissions. Pongthanaisawan and Sorapipatana (Citation2013) developed an econometric model to determine the impact of energy demand in the transport sector and its impact on CO2 emissions. The findings of the study revealed that fuel-changing alternatives are the influential source for reducing CO2 emissions. Utilizing a comparative model, Klier and Linn (Citation2013) determined that the cost of the fuel act as an effective gadget for reducing CO2 emanations in some European countries. Timilsina and Shrestha (Citation2009) examine the impact of energy consumption, population growth, and CO2 emanation in the transport sector for some Asian economies. The study used annual data from 1980 to 2005 and concluded that economic growth, energy consumption, and population growth cause CO2 emanation. Ahmed et al. (Citation2020) used to determine the impact of CO2 emanations in the Indian economy for the year 1980–2015. The study's outcomes revealed that economic growth and the road sector energy utilization are the main reason for increasing CO2 emanations. Liddle (Citation2015) used to check the EKC hypothesis on panel data of developing and developed countries. The study used urban transport-related GHG emanations per capita income and used control variables like fuel prices and urban intensity for CO2 emissions function. The finding of the study support EKC theory for CO2 emanations. Likewise, Andrés and Padilla (Citation2018) examined the impact of GHG emissions in the transportation sector of some European countries. The study used annual panel data for the period 1980 to 2014, the findings of the study revealed that transport energy intensity and the volume of the transport significantly positively associated with GHG emissions in these countries.
Chandran and Tang (Citation2013) investigated the impacts of foreign direct investment, transport sector, economic growth, and CO2 emanations by employing a panel of ASEAN-5 countries. The finding of the research study revealed that there is positive effect of the transport sector and economic growth on CO2. Mustapa and Bekhet (Citation2016), in the Malaysian context, affirm that transportation sector represents 28% of the CO2 outflows and on aggregate out of that 85% of the emissions are due to road transport in Malaysia. Saboori et al. (Citation2014) examined the long run connections of transport division and CO2 emanations in “Organization for Economic Cooperation and Development (OECD)” countries by using panel data for the period 1960– 2008. Their study findings show that there is a bidirectional causality found between transport division energy utilization and CO2 emissions. Erdogan et al. (Citation2020) examined the impact of air transportation and the rail transportation on environmental pollution. The study applied robust panel estimation technique on a panel data of top 10 air transportation countries from the time period 1995 to 2014. The study’s findings show that air transportation leads to increase emanations, whereas railway transport decreases emissions in selected countries.
Furthermore, Ratanavaraha and Jomnonkwao (Citation2015) used to determine the impact of energy use for reducing CO2 emissions, specifically in the transportation sector in Thailand. The findings of the study showed a significant association between population, GDP and CO2 emissions. Godil et al. (Citation2020) investigated the relationship between freight transportation and passenger transportation with CO2 emissions for the US economy. The study used monthly data from 2000 to 2019 and applied QADRL approach. The study's findings revealed a negative relationship of both freight and passenger transportation with CO2 emissions and indicated that the transportation system in the USA helps in reducing CO2 emissions, respectively.
Danish et al. (2018) examined the association between GDP, energy use, and CO2 emissions in the transport sector. The findings demonstrate a strong connection between selected variables in the transportation sector. Greening et al. (Citation1999) analyze the emanations of CO2 in 10 OECD countries due to freight transport. The study used data from 1970 to 1993 and discovered that the flow of CO2 increases due to freight transport in selected OECD nations. Hu et al. (Citation2019) investigated the release of CO2 gas in the atmosphere due to the transportation sector in Sweden. The result of the study revealed that 14% of the gases released are in the form of CO2 emissions from the transportation sector in Sweden. B. Wang et al. (Citation2018) used to determine the influential factors for CO2 emanations because of China's freight transportation and passenger transportation. The study used data from 1990 to 2015, and the findings showed that population, per capita GDP, and the structure of the transport system are the main factor for increasing CO2 emanations in China. Luo et al. (Citation2016) determined the impact of freight transportation on CO2 emanations on the data of 31 provinces of China from 1990 to 2007. Their results revealed that the freight transport segment is the influential factor in increasing CO2 emanation in China, approximately it expanded 68 to 436 Mt CO2 in china form 1990 to 2007. In another study on time-series data of the USA, Mishra et al. (Citation2020) examined the association between transportation services and CO2 emissions. The study used partial and multiple wavelet coherence techniques on the monthly time-series data from 2001 to 2017. The findings of the study show a long-run association between transportation services and CO2 emissions in the USA.
To examine the Environmental Kuznets hypothesis (EKC), Alshehry and Belloumi (Citation2017) investigated the impact of transport-emission nexus in the context of EKC for Saudi Arabia. The study applied ARDL bound approach in order to investigate the relationship between economic growth, and CO2 emissions in transport sector. The findings of the study rejected the validity of EKC hypothesis for transportation sector emissions in Saudi Arabia. Likewise, Xu and Lin (Citation2015) used to determine the main factors responsible for reducing CO2 emissions in China. The study was also used to check the EKC hypothesis. The findings show an inverted U-shaped association to CO2 emissions; this is due to an increase in private vehicle ownership at early stages and expansion in using the electric or hybrid vehicles at the later stage of development. Liddle's Liddle (Citation2015) findings also validate EKC hypothesis on the panel data of developing and developed economies. Go et al. (Citation2021) never find any evidence for EKC for the Malaysian context from 1990-2017. On the other hand, Kharbach and Chfadi (Citation2017) investigated a significant long-run relationship between the transport sector, economic growth, number of vehicles, and CO2 emanations. Therefore, supporting the concept of EKC hypothesis for Morocco.
The above mention studies confirmed that the transportation sector significantly influences environmental quality by releasing some dangerous gasses into the atmosphere. These studies showed that measures and modes of transportation indeed determine the amount of pollutants released into the air. More specifically, the gasses released in the air have their own property; most of the prior studies use CO2 emissions as a proxy for air pollution, however, particular matter 2.5 (PM2.5) has greater relevance due to its scientific nature and correlation with transport emissions.
3. Material and methods
In light of the hypothetical structure, this research study utilizes particulate matter 2.5 as the dependent variable and uses four independent variables such as passenger transportation, freight transportation, economic growth, and square of economic growth (EKC). The data for all variables are acquired from the World Development Index (WDI). The overall detail for the proposed model is specified underneath as:
(1)
(1)
In Equationequation (1)(1)
(1) the cross-section “i” represents selected BRICS countries, whereas “t” is the time period, which is from 1990-2018. The regression equation for the model from Equationequation (1)
(1)
(1) is given as:
(2)
(2)
where PM2.5it is particulate matter 2.5 is used as the dependent variable for this study and is defined as the air pollutant which has a diameter of less than 2.5 micrometers. PM2.5 is generated due to chemical reactions and the burning of fossils fuels in the atmosphere. Passenger transport PTRit is one of the independent variables and is defined as the total movement of passengers from one place to another by using inland transport on a given selected network (Ammirato et al., Citation2020; Godil et al., Citation2020). FTRit, is freight transportation which means shipping of transporting commodities, goods and cargo by land, sea or air (Godil et al., Citation2020; SteadieSeifi et al., Citation2014). GDPit is gross domestic product, and it measures economic growth in terms of the total worth of goods produced and total services provided in a country during one year (Roy & Das, Citation2020). GDP2it gross domestic product square representing EKC hypothesis (Ali et al., Citation2020). The term αi is used for cross-section, and the error term is represented by φit.
3.1. Estimation strategy
3.1.1. Unit root testing
In this research study, data analysis has been started by checking the units' cross-sectional dependence (CD). CD testing is necessary before starting the unit root test process because it helps us to select specific unit root tests from the set of 1st, 2nd and 3rd generation tests to manage CD. The issue of CD should be handled during estimations; it might prompt size distortion, misleading outcomes, and bias stationarity (Salim et al., Citation2017; Westerlund, Citation2007). To determine the presence of CD issues, this study used Pesaran (Citation2015) test. After obtaining the results from the CD test, the subsequent step is to check the stationarity of selected panel data. Prior literature shows that to overcome the issue of non-stationarity in the panel data, there are three types of unit root tests for example, first generation panel unit root test, second generation panel unit root test, and the third generation panel unit root test, respectively. The issue of non-stationarity in the data with homogeneous panel was resolved by Levin et al. (Citation2002), Choi (Citation2001), and Maddala and Wu (Citation1999). The issue of non-stationarity with heterogeneous panels was fixed by Im et al. (Citation2003). Likewise, Lluís Carrion‐I‐Silvestre et al. (Citation2005) manage the issue of multiple structural breaks; nevertheless, it additionally does not overcome the issue of CD. The second-generation panel unit root tests were created by Choi (Citation2006), Pesaran (Citation2007), and Moon and Perron (Citation2012), and this test has overcome the issue of CD and heterogeneity between the units. Likewise, the problem of structural breaks remains present in the data after applying the first and second-generation unit root test; it implies that these tests lose their power and are unable to perform well. At this point, the researchers recommended a third-generation panel unit root test to overcome the issue of structural breaks in the data and overcome the issue of heterogeneity and CD problems (Bai & Carrion-I-Silvestre, Citation2009). This research study applied Bai & Carrion-I-Silvestre (Citation2009), and Pesaran (2007) tests to overcome the issue of non-stationarity with CD, as the presence of CD does not allow to apply first-generation panel unit root tests (Jalil, Citation2014). Moreover, the study used Lluís Carrion‐I‐Silvestre et al. (Citation2005) test in order to overcome the issue of structural breaks for each cross-section in the data.
3.1.2. Co-integration testing
After confirming stationarity properties of data, it is imperious to check slope homogeneity or heterogeneity. For that, we employ Swamy (Citation1970) test. The study shows homogeneous assumptions for the null hypothesis and heterogeneous slope parameters for the alternative hypothesis. In the presence of CD, the first-generation co-integration test by Westerlund (Citation2005), and Larsson et al. (Citation2001) neglect to offer worthy estimates due to the size properties distortion. To overcome the issue of non-stationarity, heterogeneity, and CD, this study used Banerjee & Carrion‐I‐Silvestre (Citation2017) test. This method overcomes all the issues mentioned above as well as recognize the structural breaks in the presence of co-integration. Westerlund (Citation2007), at one end, integrates the issue of CD and heterogeneous slopes parameters, but this test negates to determine the impact of conceivable structural breaks; as a result, this test is unable to reject the null hypothesis of no co-integration in the data. Later on, the study used Westerlund and Edgerton (Citation2008) approach to deal with CD, serially correlated errors, and heterogeneous slops, and sequentially handle structural breaks which are present at various locations for each cross section. The study also used Banerjee and Carrion‐I‐Silvestre (Citation2017) approach to determine the co-integrating relationship between selected variables. This method is based on Common Correlated Effects Mean Group (CCEMG) and overcome the issues of non-stationarity, heterogeneity, and CD.
3.1.3. Cross-sectionally augmented autoregressive distributed lags (CS-ARDL)
According to the literature, several factors cause the issue of CD. This problem can mislead the results if we cannot control unobservable common factors correlated with the regressors in the model. The problem of CD and slope heterogeneity can efficiently be handled by CS-ARDL approach. This technique can overcome the above-said issues by using a dynamic common correlated estimator (Çoban & Topcu, Citation2013; Yao et al., Citation2019). The preliminary form of CS-ARDL is presented in Equationequation (2)(2)
(2) as taking after:
(3)
(3)
The detail of the Autoregressive Distributed Lags (ARDL) model is presented in Equationequation (3)(3)
(3) . On the off chance that we follow Equationequation (7)
(7)
(7) , this equation gives us distorted results due to the presence of CD. EquationEquation (4)
(4)
(4) is the all-inclusive type of Equationequation (3)
(3)
(3) by taking cross-sections averages of each regressor, as this will help us to cope up with inappropriate inference due to the presence of the threshold effect instigated because of CD (Chudik & Pesaran, Citation2015).
(4)
(4)
where,
= (
) are the midpoints of both the regressed and regressor under investigation, the lag indicators for each of the variable is represented as pw, pz, px. Additionally, Wit is the representation for the dependent variable, for example, particulate matter 2.5 and Zit is used as a symbol for all the selected independent variables, for example, passenger transportation, freight transportation, economic growth, and economic growth square. Likewise, X indicates cross-section averages to avoid CD caused by spillover effects (Liddle, Citation2018). The CS-ARDL is used to determine the long-run coefficients from short-run coefficients, respectively. EquationEquation (5)
(5)
(5) represents the mean group estimator and the long-run coefficient for this research study as follows:
(5)
(5)
The mean group is given as follows:
(6)
(6)
Short-run Coefficients are estimated as follows:
(7)
(7)
where
(8)
(8)
(9)
(9)
(10)
(10)
For CS-ARDL, like pooled mean group (PMG), the term Error Correction Mechanism (ECM (−1) shows the speed of adjustment towards equilibrium or the time it takes for an economy to reach equilibrium point.
3.2. Robustness tests
According to Yao et al. (Citation2019), traditional approaches give biasness in the results because of slope heterogeneity and CD. Therefore, this study used Augmented Mean Group (AGM) by Eberhardt and Teal (Citation2010) and Common Correlated Effect Mean Group (CCEMG) by Pesaran (Citation2006) to overcome the issue of non-stationarity, slope heterogeneity, structural breaks, and CD. The CCEMG takes averages of both dependent and independent variables to remove the spillover effects arises due to CD by including time dummies (Liddle, Citation2018). The AMG is an alternate way to deal with CCEMG, which caters to structural breaks, CD, and heterogeneity and additionally incorporate dummy variables and deals with unobservable factor (Eberhardt & Teal, Citation2010).
4. Empirical results and discussion
The results from Pesaran (Citation2015) CD test are presented in . The finding confirms the rejection of null hypothesis of no CD for all the selected variables such as PM2.5it, PTRit, FRTit, GDPit, and GDP2it at a 1 percent level of significance. The empirical findings highlight the presence of CD in the panel data.
Table 1. Results of cross-sectional dependence (CD) analysis.
shows the empirical findings of Pesaran (2007), Bai & Carrion-I-Silvestre (Citation2009) unit root tests when CD, heterogeneity, and structural breaks are present in the data. The findings of the study indicate the rejection of null hypothesis of unit root at the level. Even after considering the issue of conceivable structural breaks, the results failed to reject null hypothesis of unit root in the panel data only in Bai & Carrion-I-Silvestre (Citation2009) test. Though, for Pesaran (2007), the dependent and the independent variables are stationary at level. The empirical findings of these tests confirm that all the selected variables such as PM2.5it, PTRit, FRTit, GDPit, and GDP2it are all stationary at first difference or integrated at I (1).
Table 2. Results of unit root test with and without structural break Pesaran (2007).
After applying CD and the unit root tests, the study applied Swamy (Citation1970) slope homogeneity tests which were suggested by Pesaran and Yamagata (Citation2008). The primary purpose of this technique is to check the slope homogeneity and heterogeneity across panels (Alam et al., Citation2018). A homogenous slop coefficient is expected for the case of null hypothesis, whereas some other estimates will be recommended for the alternative hypothesis. shows the result of slope homogeneity by rejecting the null hypothesis at a 1 percent level of significance.
Table 3. Results of slope heterogeneity analysis.
The empirical findings of Wasteland and Edgerton’s (2008) test are presented in . The test statistics reject the null hypothesis of “no co-integration”, implying that the variable are co-integrated in the long-run. This test accounts for structural breaks, serial correlation, CD, and heterogeneity while estimation, thus considered superior to traditional co-integration tests. The test outcome rejected the null hypothesis for all options i.e., structural breaks, regime shift, and the mean shift. It confirms the presence of a co-integration relationship among variables in the long run.
Table 4. Results of Westerlund and Edgerton (Citation2008) panel co-integration analysis.
shows the outcome of Banerjee and Carrion‐I‐Silvestre (Citation2017) co-integration approach and affirm the presence of a co-integrating association between PM2.5it, PTRit, FRTit, GDPit, and GDP2it at 1 percent level of significance for the overall sample and each selected country such as Brazil, Russia, India, China, and South Africa. As the co-integrating association between all the variables is affirmed, therefore we will go for determining the short-run and the long-run association between particulate matter 2.5, passenger transportation, freight transportation, and economic growth, respectively.
Table 5. Results of Banerjee and Carrion‐I‐Silvestre (Citation2017) co-integration analysis.
The empirical outcomes of the cross-sectional augmented autoregressive distributed lags model (CS-ARDL) are presented in . The empirical findings show that passenger transportation positively affects particulate matter 2.5 with a coefficient of 0.111%, which is significant at 1%. It notes that a 1% rise in passenger transportation causes to increase in particulate matter 2.5 by 0.111%. The results are supported by (Ahmed et al., Citation2020; Andrés & Padilla, Citation2018; Chandran & Tang, Citation2013). Similarly, freight transportation is also positively linked with particulate matter 2.5. The study discovers that a 1% increase in freight transportation causes particulate matter 2.5 to rise by 0.284%. These findings echoed recent literature ( Greening et al., Citation1999; Luo et al., Citation2016; B. Wang et al., Citation2018 ). Likewise, the results show that economic growth is positively associated with particulate matter 2.5, which is significant at 1%, with a coefficient of 0.253%. We find that a 1% increase in gross domestic product (GDP) causes to increase in particulate matter 2.5 by 0.253%. These results adhered the findings of ( Ahmed et al., Citation2020; Chandran & Tang, Citation2013; Ratanavaraha & Jomnonkwao, Citation2015; Raza et al., Citation2019; B. Wang et al., Citation2018 ). Similarly, economic growth produces a positive impact on particulate matter 2.5 with a coefficient of 0.253. It shows that a 1 percent increase in economic growth leads to increased particulate matter 2.5 by 0.253%. The square of GDP represents the presence of EKC curve, which shows that after reaching a certain level, the impact of income on particulate matter 2.5 turns negative. These results are largely supported by extensive literature, where it is argued that higher income improves the country’s efficiency, leading to lower residuals (An et al., Citation2021; Razzaq et al., Citation2021).
Table 6. Results of CS-ARDL analysis (Long run CS-ARDL results).
reported the short-run results, and the study finds a significant positive association between passenger transportation, freight transportation, economic growth, and particulate matter 2.5. In contrast, economic growth square negatively affects particulate matter 2.5.
Table 7. Results of CS-ARDL analysis (Short-run CS-ARDL results).
The ECM (−1) indicates the speed of adjustment, which is −0.112%, confirming the conversion towards long-run equilibrium in case of any shock in the short-run. It also validates a stable long-run association between model variables. The short-run coefficients for passenger transportation, freight transportation, economic growth and economic growth square are 0.097%, 0.239%, 0.271% and −0.073% respectively.
shows the robustness results obtained from AMG and CCEMG approaches. Both approaches represent a negative relationship between the square of economic growth and particulate matter 2.5 with coefficients of −0.300 and −0.441%, individually. Contrarily, there exists a positive association between passenger transportation and particulate matter 2.5 with coefficients of 0.2647% and 0.303% for AMG and CCEMG, respectively. Likewise, freight transportation is positively associated with particulate matter 2.5 with coefficients of 0.301% and 0.510%, separately. Additionally, gross domestic product (GDP) is related positively with particulate matter 2.5 with coefficients of 0.406% and 0.3008%. The results obtained from the robustness check support our outcomes obtained from cross-sectionally augmented autoregressive distributed lags model (CS-ARDL).
Table 8. Results of AMG and CCEMG for robustness check.
5. Conclusion and policy implications
This study aims to analyze the impact of freight transportation and passenger transportation on air pollution in the case of BRICS countries. The study used particulate matter 2.5 as the proxy for air pollution. We used Pesaran (Citation2015) test to determine the CD, and later, the study applied different unit root tests, for example, Lluís Carrion-I-Silvestre et al. (2005), Pesaran (2007), and Bai & Carrion-I-Silvestre (Citation2009) to check the stationarity in the panel data. Moreover, the study investigated the slope heterogeneity applying Swamy (Citation1970) and Pesaran and Yamagata (Citation2008) tests. In addition, the long-run co-integration relationship among the variables is determined using Westerlund and Edgerton (Citation2008), and Banerjee and Carrion‐I‐Silvestre (Citation2017) approach. The empirical findings show that passenger transportation, freight transportation, and economic growth positively affect particulate matter 2.5. Whereas square of economic growth is negatively associated with particulate matter 2.5, confirming the presence of EKC hypothesis.
Based on the above findings, some polices implications are suggested for BRICS economies. First, green transportation networks should be introduced to reduce environmental pollution. The logistics activities in these countries must be environmental friendly, and awareness must be created among the public to become aware of green logistics and adopt sustainable alternatives. Second, there is a need to improve fuel efficiency and introduce pollution-free vehicles to cope with the dangerous gasses released from freight and passenger transportation. The BRICS countries are developing countries, and their growth rate is fast compared to other developing countries, so the authorities in these countries should implement green transportation, green packaging, and green supply chain designs to promote green economic growth. For attaining long sustainable environment development, the government of BRICS countries should improve their physical infrastructure by introducing digital transportation system.
Disclosure statement
No potential conflict of interest was reported by the author(s).
References
- Abrell, J. (2010). Regulating CO2 emissions of transportation in Europe: A CGE-analysis using market-based instruments. Transportation Research Part D: Transport and Environment, 15(4), 235–239. https://doi.org/10.1016/j.trd.2010.02.002
- Aggarwal, P., & Jain, S. (2016). Energy demand and CO2 emissions from urban on-road transport in Delhi: current and future projections under various policy measures. Journal of Cleaner Production, 128, 48–61. https://doi.org/10.1016/j.jclepro.2014.12.012
- Ahmed, Z., Ali, S., Saud, S., & Shahzad, S. J. H. (2020). Transport CO2 emissions, drivers, and mitigation: An empirical investigation in India. Air Quality, Atmosphere & Health, 13(11), 1367–1374. https://doi.org/10.1007/s11869-020-00891-x
- Air Transportation Action Group. (2019). Retrieved 25 July, 2021, from https://www.atag.org/facts-figures.html.
- Alam, M., Hammoudeh, M. D., Tiwari, S., & Kumar, A. (2018). The nexus between access to electricity and labour productivity in developing countries. Energy Policy, 122, 715–726. https://doi.org/10.1016/j.enpol.2018.08.009
- Ali, M., Raza, S. A., & Khamis, B. (2020). Environmental degradation, economic growth, and energy innovation: Evidence from European countries. Environmental Science & Pollution Research, 27(22), 1–10.
- Alshehry, A. S., & Belloumi, M. (2017). Study of the environmental Kuznets curve for transport carbon dioxide emissions in Saudi Arabia. Renewable and Sustainable Energy Reviews, 75, 1339–1347. https://doi.org/10.1016/j.rser.2016.11.122
- Amin, A., Altinoz, B., & Dogan, E. (2020). Analyzing the determinants of carbon emissions from transportation in European countries: the role of renewable energy and urbanization. Clean Technologies and Environmental Policy, 22(8), 1725–1734. https://doi.org/10.1007/s10098-020-01910-2
- Ammirato, S., Felicetti, A. M., Linzalone, R., Volpentesta, A. P., & Schiuma, G. (2020). A systematic literature review of revenue management in passenger transportation. Measuring Business Excellence, 24(2), 223–242. https://doi.org/10.1108/MBE-09-2019-0096
- Andrés, L., & Padilla, E. (2018). Driving factors of GHG emissions in the EU transport activity. Transport Policy, 61, 60–74. https://doi.org/10.1016/j.tranpol.2017.10.008
- An, H., Razzaq, A., Haseeb, M., & Mihardjo, L. W. (2021). The role of technology innovation and people’s connectivity in testing environmental Kuznets curve and pollution heaven hypotheses across the Belt and Road host countries: New evidence from Method of Moments Quantile Regression. Environmental Science and Pollution Research International, 28(5), 5254–5270.
- An, H., Razzaq, A., Nawaz, A., Noman, S. M., & Khan, S. A. R. (2021a). Nexus between green logistic operations and triple bottom line: evidence from infrastructure-led Chinese outward foreign direct investment in Belt and Road host countries. Environmental Science and Pollution Research International, 28(37), 51022–51024. https://doi.org/10.1007/s11356-021-12470-3
- Arrow, K., Bolin, B., Costanza, R., Dasgupta, P., Folke, C., Holling, C. S., Jansson, B.-O., Levin, S., Mäler, K.-G., Perrings, C., & Pimentel, D. (1996). Economic growth, carrying capacity, and the environment. Environment and Development Economics, 1(1), 104–110. https://doi.org/10.1017/S1355770X00000413
- Bagoulla, C., & Guillotreau, P. (2020). Maritime transport in the French economy and its impact on air pollution: An input-output analysis. Marine Policy, 116, 103818. https://doi.org/10.1016/j.marpol.2020.103818
- Bai, J., & Carrion-I-Silvestre, J. L. (2009). Structural changes, common stochastic trends, and unit roots in panel data. Review of Economic Studies, 76(2), 471–501. https://doi.org/10.1111/j.1467-937X.2008.00530.x
- Balkanski, Y., Myhre, G., Gauss, M., Rädel, G., Highwood, E., & Shine, K. (2010). Direct radiative effect of aerosols emitted by transport: from road, shipping and aviation. Atmospheric Chemistry and Physics, 10(10), 4477–4489. https://doi.org/10.5194/acp-10-4477-2010
- Baloch, M. A., & Suad, S. (2018). Modeling the impact of transport energy consumption on CO 2 emission in Pakistan: Evidence from ARDL approach. Environmental Science and Pollution Research, 25(10), 9461–9473.
- Banerjee, A., & Carrion‐I‐Silvestre, J. L. (2017). Testing for panel co-integration using common correlated effects estimators. Journal of Time Series Analysis, 38(4), 610–636. https://doi.org/10.1111/jtsa.12234
- Banister, D., Anderton, K., Bonilla, D., Givoni, M., & Schwanen, T. (2011). Transportation and the environment. Annual Review of Environment and Resources, 36(1), 247–270. https://doi.org/10.1146/annurev-environ-032310-112100
- Barbier, E. B. (1997). Introduction to the environmental Kuznets curve special issue. Environment and Development Economics, 2(4), 369–381. https://doi.org/10.1017/S1355770X97000193
- Beckerman, W. (1992). Economic growth and the environment: Whose growth? Whose environment? World Development, 20(4), 481–496. https://doi.org/10.1016/0305-750X(92)90038-W
- Begum, R. A., Sohag, K., Abdullah, S. M. S., & Jaafar, M. (2015). CO2 emissions, energy consumption, economic and population growth in Malaysia. Renewable and Sustainable Energy Reviews, 41, 594–601. https://doi.org/10.1016/j.rser.2014.07.205
- Berg, J., & Ihlström, J. (2019). The importance of public transport for mobility and everyday activities among rural residents. Social Sciences, 8(2), 58. https://doi.org/10.3390/socsci8020058
- Chandran, V., & Tang, C. F. (2013). The impacts of transport energy consumption, foreign direct investment and income on CO2 emissions in ASEAN-5 economies. Renewable and Sustainable Energy Reviews, 24, 445–453. https://doi.org/10.1016/j.rser.2013.03.054
- Chen, L., & De Lombaerde, P. (2014). Testing the relationships between globalization, regionalization and the regional hubness of the BRICs. Journal of Policy Modeling, 36, S111–S131. https://doi.org/10.1016/j.jpolmod.2013.10.011
- Cherniwchan, J. (2012). Economic growth, industrialization, and the environment. Resource and Energy Economics, 34(4), 442–467. https://doi.org/10.1016/j.reseneeco.2012.04.004
- Chien, F., Anwar, A., Hsu, C. C., Sharif, A., Razzaq, A., & Sinha, A. (2021). The role of information and communication technology in encountering environmental degradation: Proposing an SDG framework for the BRICS countries. Technology in Society, 65, 101587. https://doi.org/10.1016/j.techsoc.2021.101587
- Choi, I. (2001). Unit root tests for panel data. Journal of International Money and Finance, 20(2), 249–272. https://doi.org/10.1016/S0261-5606(00)00048-6
- Choi, I. (2006). Combination unit root tests for cross-sectionally correlated panels. Econometric theory and practice: Frontiers of analysis and applied research: Essays in honor of Peter CB Phillips, Vol. 11. Cambridge University Press, 311–333.
- Chudik, A., & Pesaran, M. H. (2015). Common correlated effects estimation of heterogeneous dynamic panel data models with weakly exogenous regressors. Journal of Econometrics, 188(2), 393–420. https://doi.org/10.1016/j.jeconom.2015.03.007
- Çoban, S., & Topcu, M. (2013). The nexus between financial development and energy consumption in the EU: A dynamic panel data analysis. Energy Economics, 39, 81–88. https://doi.org/10.1016/j.eneco.2013.04.001
- Cowan, W. N., Chang, T., Inglesi-Lotz, R., & Gupta, R. (2014). The nexus of electricity consumption, economic growth and CO2 emissions in the BRICS countries. Energy Policy, 66, 359–368. [Database] https://doi.org/10.1016/j.enpol.2013.10.081
- Cui, Q., & Li, Y. (2015). An empirical study on the influencing factors of transportation carbon efficiency: Evidences from fifteen countries. Applied Energy, 141, 209–217. https://doi.org/10.1016/j.apenergy.2014.12.040
- Baloch, M. A., & Suad, S. (2018). Modeling the impact of transport energy consumption on CO2 emission in Pakistan: evidence from ARDL approach. Environmental Science and Pollution Research, 25(10), 9461–9473.
- Dinda, S. (2004). Environmental Kuznets curve hypothesis: A survey. Ecological Economics, 49(4), 431–455. https://doi.org/10.1016/j.ecolecon.2004.02.011
- Du, H., Chen, Z., Peng, B., Southworth, F., Ma, S., & Wang, Y. (2019). What drives CO2 emissions from the transport sector? A linkage analysis. Energy, 175, 195–204. https://doi.org/10.1016/j.energy.2019.03.052
- Eberhardt, M., & Teal, F. (2010). Productivity analysis in global manufacturing production. DEGIT, Dynamics, Economic Growth, and International Trade, 59.
- Erdogan, S., Adedoyin, F. F., Bekun, F. V., & Sarkodie, S. A. (2020). Testing the transport-induced environmental Kuznets curve hypothesis: The role of air and railway transport. Journal of Air Transport Management, 89, 101935. https://doi.org/10.1016/j.jairtraman.2020.101935
- García-Olivares, A., Solé, J., & Osychenko, O. (2018). Transportation in a 100% renewable energy system. Energy Conversion and Management, 158, 266–285. https://doi.org/10.1016/j.enconman.2017.12.053
- Giannakis, E., Serghides, D., Dimitriou, S., & Zittis, G. (2020). Land transport CO2 emissions and climate change: evidence from Cyprus. International Journal of Sustainable Energy, 39(7), 634–647. https://doi.org/10.1080/14786451.2020.1743704
- Go, Y.-H., Lau, L.-S., Liew, F.-M., & Senadjki, A. (2021). A transport environmental Kuznets curve analysis for Malaysia: Exploring the role of corruption. Environmental Science and Pollution Research International, 28(3), 3421–3433.
- Godil, D. I., Sharif, A., Afshan, S., Yousuf, A., & Khan, S. A. R. (2020). The asymmetric role of freight and passenger transportation in testing EKC in the US economy: Evidence from QARDL approach. Environmental Science and Pollution Research International, 27(24), 30108–30117.
- Goldman, S. (2003). Dreaming with BRICs: The path to 2050. Global Economics Paper, 99, 30108–30117.
- Grahn, M., Azar, C., & Lindgren, K. (2009). The role of biofuels for transportation in CO2 emission reduction scenarios with global versus regional carbon caps. Biomass and Bioenergy, 33(3), 360–371. https://doi.org/10.1016/j.biombioe.2008.08.019
- Greening, L. A., Ting, M., & Davis, W. B. (1999). Decomposition of aggregate carbon intensity for freight: Trends from 10 OECD countries for the period 1971–1993. Energy Economics, 21(4), 331–361. https://doi.org/10.1016/S0140-9883(99)00010-9
- He, F., Chang, K.-C., Li, M., Li, X., & Li, F. (2020). Bootstrap ARDL test on the relationship among trade, FDI, and CO2 emissions: based on the experience of BRICS countries. Sustainability, 12(3), 1060. https://doi.org/10.3390/su12031060
- He, X., Mishra, S., Aman, A., Shahbaz, M., Razzaq, A., & Sharif, A. (2021). The linkage between clean energy stocks and the fluctuations in oil price and financial stress in the US and Europe? Evidence from QARDL approach. Resources Policy, 72, 102021. https://doi.org/10.1016/j.resourpol.2021.102021
- Hoffrichter, A., Miller, A. R., Hillmansen, S., & Roberts, C. (2012). Well-to-wheel analysis for electric, diesel and hydrogen traction for railways. Transportation Research Part D: Transport and Environment, 17(1), 28–34. https://doi.org/10.1016/j.trd.2011.09.002
- Hu, J., Wood, R., Tukker, A., Boonman, H., & de Boer, B. (2019). Global transport emissions in the Swedish carbon footprint. Journal of Cleaner Production, 226, 210–220. https://doi.org/10.1016/j.jclepro.2019.03.263
- Im, K. S., Pesaran, M. H., & Shin, Y. (2003). Testing for unit roots in heterogeneous panels. Journal of Econometrics, 115(1), 53–74. https://doi.org/10.1016/S0304-4076(03)00092-7
- Jalil, A. (2014). Energy–growth conundrum in energy exporting and importing countries: Evidence from heterogeneous panel methods robust to cross-sectional dependence. Energy Economics, 44, 314–324. https://doi.org/10.1016/j.eneco.2014.04.015
- Jofred, P., & Öster, P. (2011). CO2 emissions from freight transport and the impact of supply chain management: A case study at Atlas Copco industrial technique.
- Kharbach, M., & Chfadi, T. (2017). CO2 emissions in Moroccan road transport sector: Divisia, Cointegration, and EKC analyses. Sustainable Cities and Society, 35, 396–401. https://doi.org/10.1016/j.scs.2017.08.016
- Kim, N. S., & Van Wee, B. (2014). Toward a better methodology for assessing CO2 emissions for intermodal and truck-only freight systems: A European case study. International Journal of Sustainable Transportation, 8(3), 177–201. https://doi.org/10.1080/15568318.2011.633689
- Klier, T., & Linn, J. (2013). Fuel prices and new vehicle fuel economy—comparing the United States and Western Europe. Journal of Environmental Economics and Management, 66(2), 280–300. https://doi.org/10.1016/j.jeem.2013.03.003
- Knittel, C. R. (2012). Reducing petroleum consumption from transportation. Journal of Economic Perspectives, 26(1), 93–118. https://doi.org/10.1257/jep.26.1.93
- Krautzberger, L., & Wetzel, H. (2012). Transport and CO2: Productivity growth and carbon dioxide emissions in the European commercial transport industry. Environmental and Resource Economics, 53(3), 435–454. https://doi.org/10.1007/s10640-012-9569-z
- Larsson, R., Lyhagen, J., & Löthgren, M. (2001). Likelihood‐based co-integration tests in heterogeneous panels. The Econometrics Journal, 4(1), 109–142. https://doi.org/10.1111/1368-423X.00059
- Levin, A., Lin, C.-F., & Chu, C.-S J. (2002). Unit root tests in panel data: Asymptotic and finite-sample properties. Journal of Econometrics, 108(1), 1–24. https://doi.org/10.1016/S0304-4076(01)00098-7
- Liang, Y., Niu, D., Wang, H., & Li, Y. (2017). Factors affecting transportation sector CO2 emissions growth in China: an LMDI decomposition analysis. Sustainability, 9(10), 1730. https://doi.org/10.3390/su9101730
- Liddle, B. (2015). Urban transport pollution: Revisiting the environmental Kuznets curve. International Journal of Sustainable Transportation, 9(7), 502–508. https://doi.org/10.1080/15568318.2013.814077
- Liddle, B. (2018). Consumption-based accounting and the trade-carbon emissions nexus. Energy Economics, 69, 71–78. https://doi.org/10.1016/j.eneco.2017.11.004
- Ling, G., Razzaq, A., Guo, Y., Fatima, T., & Shahzad, F. (2021). Asymmetric and time-varying linkages between carbon emissions, globalization, natural resources and financial development in China. Environment, Development and Sustainability, 1–29.
- Lingyan, M., Zhao, Z., Malik, H. A., Razzaq, A., An, H., & Hassan, M. (2021). Asymmetric impact of fiscal decentralization and environmental innovation on carbon emissions: Evidence from highly decentralized countries. Energy & Environment, 30108–30117. https://doi.org/10.1177/0958305X211018453
- Liu, Y., Huang, L., & Onstein, E. (2020). How do age structure and urban form influence household CO2 emissions in road transport? Evidence from municipalities in Norway in 2009, 2011 and 2013. Journal of Cleaner Production, 265, 121771. https://doi.org/10.1016/j.jclepro.2020.121771
- Liu, J.-L., Ma, C.-Q., Ren, Y.-S., & Zhao, X.-W. (2020). Do real output and renewable energy consumption affect CO2 emissions? Evidence for selected BRICS countries. Energies, 13(4), 960. https://doi.org/10.3390/en13040960
- Lluís Carrion‐I‐Silvestre, J., D., Barrio, ‐Castro, T., & López-Bazo, E. (2005). Breaking the panels: an application to the GDP per capita. The Econometrics Journal, 8(2), 159–175. https://doi.org/10.1111/j.1368-423X.2005.00158.x
- Luo, X., Dong, L., Dou, Y., Liang, H., Ren, J., & Fang, K. (2016). Regional disparity analysis of Chinese freight transport CO2 emissions from 1990 to 2007: Driving forces and policy challenges. Journal of Transport Geography, 56, 1–14. https://doi.org/10.1016/j.jtrangeo.2016.08.010
- Maddala, G. S., & Wu, S. (1999). A comparative study of unit root tests with panel data and a new simple test. Oxford Bulletin of Economics and Statistics, 61(S1), 631–652. https://doi.org/10.1111/1468-0084.0610s1631
- Maparu, T. S., & Mazumder, T. N. (2017). Transport infrastructure, economic development and urbanization in India (1990–2011): Is there any causal relationship? Transportation Research Part A: Policy and Practice, 100, 319–336. https://doi.org/10.1016/j.tra.2017.04.033
- Marrero, G. A. (2010). Greenhouse gases emissions, growth and the energy mix in Europe. Energy Economics, 32(6), 1356–1363. https://doi.org/10.1016/j.eneco.2010.09.007
- Mishra, S., Sinha, A., Sharif, A., & Suki, N. M. (2020). Dynamic linkages between tourism, transportation, growth and carbon emission in the USA: Evidence from partial and multiple wavelet coherence. Current Issues in Tourism, 23(21), 2733–2755. https://doi.org/10.1080/13683500.2019.1667965
- Moon, H. R., & Perron, B. (2012). Beyond panel unit root tests: Using multiple testing to determine the nonstationarity properties of individual series in a panel. Journal of Econometrics, 169(1), 29–33. https://doi.org/10.1016/j.jeconom.2012.01.008
- Muratori, M., Smith, S. J., Kyle, P., Link, R., Mignone, B. K., & Kheshgi, H. S. (2017). Role of the freight sector in future climate change mitigation scenarios. Environmental Science & Technology, 51(6), 3526–3533. https://doi.org/10.1021/acs.est.6b04515
- Mustapa, S. I., & Bekhet, H. A. (2016). Analysis of CO2 emissions reduction in the Malaysian transportation sector: An optimisation approach. Energy Policy, 89, 171–183. https://doi.org/10.1016/j.enpol.2015.11.016
- Ozturk, I., & Acaravci, A. (2013). The long-run and causal analysis of energy, growth, openness and financial development on carbon emissions in Turkey. Energy Economics, 36, 262–267. https://doi.org/10.1016/j.eneco.2012.08.025
- Pesaran, M. H. (2006). Estimation and inference in large heterogeneous panels with a multifactor error structure. Econometrica, 74(4), 967–1012. https://doi.org/10.1111/j.1468-0262.2006.00692.x
- Pesaran, M. H. (2007). A simple panel unit root test in the presence of cross‐section dependence. Journal of Applied Econometrics, 22(2), 265–312. https://doi.org/10.1002/jae.951
- Pesaran, M. H. (2015). Testing weak cross-sectional dependence in large panels. Econometric Reviews, 34(6–10), 1089–1117. https://doi.org/10.1080/07474938.2014.956623
- Pesaran, M. H., & Yamagata, T. (2008). Testing slope homogeneity in large panels. Journal of Econometrics, 142(1), 50–93. https://doi.org/10.1016/j.jeconom.2007.05.010
- Pongthanaisawan, J., & Sorapipatana, C. (2013). Greenhouse gas emissions from Thailand’s transport sector: Trends and mitigation options. Applied Energy, 101, 288–298. https://doi.org/10.1016/j.apenergy.2011.09.026
- Ratanavaraha, V., & Jomnonkwao, S. (2015). Trends in Thailand CO2 emissions in the transportation sector and Policy Mitigation. Transport Policy, 41, 136–146. https://doi.org/10.1016/j.tranpol.2015.01.007
- Raza, S. A., Shah, N., & Sharif, A. (2019). Time frequency relationship between energy consumption, economic growth and environmental degradation in the United States: Evidence from transportation sector. Energy, 173, 706–720. https://doi.org/10.1016/j.energy.2019.01.077
- Razzaq, A., Ajaz, T., Li, J. C., Irfan, M., & Suksatan, W. (2021b). Investigating the asymmetric linkages between infrastructure development, green innovation, and consumption-based material footprint: Novel empirical estimations from highly resource-consuming economies. Resources Policy, 74, 102302. https://doi.org/10.1016/j.resourpol.2021.102302
- Razzaq, A., Sharif, A., Ahmad, P., & Jermsittiparsert, K. (2021). Asymmetric role of tourism development and technology innovation on carbon dioxide emission reduction in the Chinese economy: Fresh insights from QARDL approach. Sustainable Development, 29(1), 176–193. https://doi.org/10.1002/sd.2139
- Razzaq, A., Wang, Y., Chupradit, S., Suksatan, W., & Shahzad, F. (2021a). Asymmetric inter-linkages between green technology innovation and consumption-based carbon emissions in BRICS countries using quantile-on-quantile framework. Technology in Society, 66, 101656. https://doi.org/10.1016/j.techsoc.2021.101656
- Roy, S., & Das, S. (2020). Impact of environmental elements on sustainable economic growth: Evidence from BIMSTEC. Wealth: International Journal of Money, Banking & Finance, 9(1), 46–51.
- Saboori, B., Sapri, M., & bin Baba, M. (2014). Economic growth, energy consumption and CO2 emissions in OECD (Organization for Economic Co-operation and Development)'s transport sector: A fully modified bi-directional relationship approach. Energy, 66, 150–161. https://doi.org/10.1016/j.energy.2013.12.048
- Safaai, N. S. M., Noor, Z. Z., Hashim, H., Ujang, Z., & Talib, J. (2011). Projection of CO2 emissions in Malaysia).Environmental Progress & Sustainable Energy, 30(4), 658–665. https://doi.org/10.1002/ep.10512
- Salim, R., Yao, Y., & Chen, G. S. (2017). Does human capital matter for energy consumption in China? Energy Economics, 67, 49–59. https://doi.org/10.1016/j.eneco.2017.05.016
- Scerri, M., & Lastres, H. M. (2020). The role of the state: BRICS National Systems of Innovation. Taylor & Francis.
- Shahbaz, M., Khraief, N., & Jemaa, M. M. B. (2015). On the causal nexus of road transport CO2 emissions and macroeconomic variables in Tunisia: Evidence from combined co-integration tests. Renewable and Sustainable Energy Reviews, 51, 89–100. https://doi.org/10.1016/j.rser.2015.06.014
- Shahzad, F., Yannan, D., Kamran, H. W., Suksatan, W., Nik Hashim, N. A. A., & Razzaq, A. (2021). Outbreak of epidemic diseases and stock returns: An event study of emerging economy. Economic Research-Ekonomska Istraživanja, 1–20. https://doi.org/10.1080/1331677X.2021.1941179
- Sharif, A., Baris-Tuzemen, O., Uzuner, G., Ozturk, I., & Sinha, A. (2020). Revisiting the role of renewable and non-renewable energy consumption on Turkey’s ecological footprint: Evidence from Quantile ARDL approach. Sustainable Cities and Society, 57, 102138. https://doi.org/10.1016/j.scs.2020.102138
- Sharif, A., Raza, S. A., Ozturk, I., & Afshan, S. (2019). The dynamic relationship of renewable and nonrenewable energy consumption with carbon emission: A global study with the application of heterogeneous panel estimations. Renewable Energy., 133, 685–691. https://doi.org/10.1016/j.renene.2018.10.052
- Šimenc, M. (2016). Overview and comparative analysis of emission calculators for inland shipping. International Journal of Sustainable Transportation, 10(7), 627–637. https://doi.org/10.1080/15568318.2015.1079753
- Sobrino, N., & Monzon, A. (2014). The impact of the economic crisis and policy actions on GHG emissions from road transport in Spain. Energy Policy, 74, 486–498. https://doi.org/10.1016/j.enpol.2014.07.020
- Sohail, M. T., Mahfooz, Y., Azam, K., Yen, Y., Genfu, L., & Fahad, S. (2019). Impacts of urbanization and land cover dynamics on underground water in Islamabad. Pakistan. Desal. Wat. Treat, 159, 402–411.
- Sohail, M. T., Ullah, S., Majeed, M. T., & Usman, A. (2021). Pakistan management of green transportation and environmental pollution: A nonlinear ARDL analysis. Environmental Science and Pollution Research, 1–10.
- Solaymani, S. (2019). CO2 emissions patterns in 7 top carbon emitter economies: the case of transport sector. Energy, 168, 989–1001. https://doi.org/10.1016/j.energy.2018.11.145
- SteadieSeifi, M., Dellaert, N. P., Nuijten, W., Van Woensel, T., & Raoufi, R. (2014). Multimodal freight transportation planning: A literature review. European Journal of Operational Research, 233(1), 1–15. https://doi.org/10.1016/j.ejor.2013.06.055
- Stern, N. (2009). A blueprint for a safer planet: How to manage climate change and create a new era of progress and prosperity: Random House.
- Sun, Y., Duru, O. A., Razzaq, A., & Dinca, M. S. (2021). The asymmetric effect eco-innovation and tourism towards carbon neutrality target in Turkey. Journal of Environmental Management, 299, 113653.
- Swamy, P. A. (1970). Efficient inference in a random coefficient regression model. Econometrica, 38(2), 311–323. https://doi.org/10.2307/1913012
- Talbi, B. (2017). CO2 emissions reduction in road transport sector in Tunisia. Renewable and Sustainable Energy Reviews, 69, 232–238. https://doi.org/10.1016/j.rser.2016.11.208
- Timilsina, G. R., & Shrestha, A. (2009). Transport sector CO2 emissions growth in Asia: Underlying factors and policy options. Energy Policy, 37(11), 4523–4539. https://doi.org/10.1016/j.enpol.2009.06.009
- Van Fan, Y., Perry, S., Klemeš, J. J., & Lee, C. T. (2018). A review on air emissions assessment. Journal of Cleaner Production, 194, 673–684. https://doi.org/10.1016/j.jclepro.2018.05.151
- Waisman, H.-D., Guivarch, C., & Lecocq, F. (2013). The transportation sector and low-carbon growth pathways: modelling urban, infrastructure, and spatial determinants of mobility. Climate Policy, 13(sup. 1), 106–129. https://doi.org/10.1080/14693062.2012.735916
- Wang, B., Sun, Y., Chen, Q., & Wang, Z. (2018). Determinants analysis of carbon dioxide emissions in passenger and freight transportation sectors in China. Structural Change and Economic Dynamics, 47, 127–132. https://doi.org/10.1016/j.strueco.2018.08.003
- Westerlund, J. (2005). New simple tests for panel co-integration. Econometric Reviews, 24(3), 297–316. https://doi.org/10.1080/07474930500243019
- Westerlund, J. (2007). Testing for error correction in panel data. Oxford Bulletin of Economics and Statistics, 69(6), 709–748. https://doi.org/10.1111/j.1468-0084.2007.00477.x
- Westerlund, J., & Edgerton, D. L. (2008). A simple test for co-integration in dependent panels with structural breaks. Oxford Bulletin of Economics and Statistics, 70(5), 665–704. https://doi.org/10.1111/j.1468-0084.2008.00513.x
- Xu, B., & Lin, B. (2015). Factors affecting carbon dioxide (CO2) emissions in China's transport sector: A dynamic nonparametric additive regression model. Journal of Cleaner Production, 101, 311–322. https://doi.org/10.1016/j.jclepro.2015.03.088
- Yao, Y., Ivanovski, K., Inekwe, J., & Smyth, R. (2019). Human capital and energy consumption: Evidence from OECD countries. Energy Economics, 84, 104534. https://doi.org/10.1016/j.eneco.2019.104534
- Zhou, W., Wang, T., Yu, Y., Chen, D., & Zhu, B. (2016). Scenario analysis of CO2 emissions from China’s civil aviation industry through 2030. Applied Energy, 175, 100–108. https://doi.org/10.1016/j.apenergy.2016.05.004