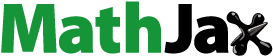
Abstract
This study assesses the impact of corruption on Nigeria’s domestic savings. To this end, an ARDL technique was employed to analyze quarterly data for the 1996–2019 period. We find evidence of a long-term relationship between domestic savings and corruption (along with income growth, income level, inflation rate, deposit interest rate, unemployment rate including oil price). The empirical results indicate that lowering corruption level raises domestic savings over the long-term. Other important factors that drive domestic savings over the long-run in Nigeria are income level, deposit interest rate, inflation rate, unemployment rate as well as oil price. Having established the major factors that affect domestic savings, some recommendations are offered to encourage saving mobilization in Nigeria.
Keywords:
1. Introduction
Early studies have assigned an important role to domestic saving mobilization in economic development process (Lucas, Citation1988; Romer, Citation1986; Solow, Citation1956). These studies opined that rising savings makes investment funds easily accessible to businesses, leading to an expansion of output, and ultimately higher levels of economic development. However, inadequate savings reduces investment spending and output, resulting in sluggish growth and development. In spite of the significance of high savings rates, Nigeria has been less successful in domestic saving mobilization (required to drive sustainable economic development) compared to selected developing economies over the years (see ). For instance, Nigeria’s gross/aggregate domestic savings (share of GDP) was lower than this group of countries except Morocco during the 2009–2011 period. Similarly, countries including Algeria, Gabon, Indonesia and Malaysia recorded savings rates which were higher than those of Nigeria in the year 2012, while the average savings rates of Nigeria fell below those of all nations in the group between 2013 and 2019.
Figure 1. Plots of domestic savings in selected developing countries including Nigeria. Source: Authors’ compilation based on data obtained from the World Development Indicators.
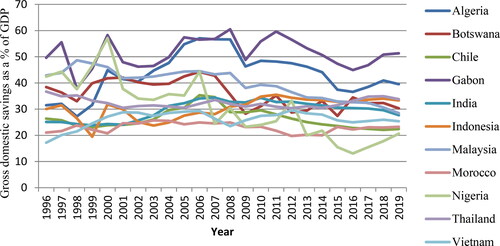
It is not surprising that low levels of economic development have accompanied inadequate savings in Nigeria with many living in poor economic conditions. Some of the factors blamed for Nigeria’s low domestic savings include low levels of income, deposit (interest) rate, economic growth, financial deepening/development, broad money supply, and high rate of inflation, high debt service, unfavourable terms of trade, amongst others (Abu et al., Citation2019; Adelakun, Citation2015; Antai et al., Citation2015; Bamidele et al., Citation2015; Chete, Citation1999; Imoisi et al., Citation2018; Mojekwu & Ogbulu, Citation2017; Nwachukwu & Egwaikhide, Citation2007; Nwachukwu & Odigie, Citation2011; Olusoji, Citation2003; Otiwu et al., Citation2018; Ozioma et al., Citation2016; Uremadu, Citation2007; Wafure & Abu, Citation2009).
But the studies didn’t consider corruption as an important factor that can affect domestic savings. Interestingly, some researchers have emphasized that corruption adversely affects domestic savings (Abu et al., Citation2015; Swaleheen, Citation2008). They argued that corruption encourages capital flight which consequently results in a decline in aggregate savings in a country. Also, reducing corruption is one major step to put a country on the path of sustainable development (Das & Drine, Citation2020).
Nigeria continues to rank high among countries considered to be corrupt (Abu & Karim, Citation2021; Abu & Staniewski, Citation2019), and the creation of anti-graft bodies like Economic and Financial Crimes Commission (EFCC) and Independent Corrupt Practices Commission (ICPC) has done little to lower corruption level in the country. This claim coincides with views of the Transparency International (TI) which publishes data on perceived corruption across countries. The TI’s corruption perception index (CPI) shows the level to which a nation is viewed to be corrupt. The index ranges from 0 (very corrupt) to 100 (not corrupt). Nigeria’s CPI has consistently been less than 40 out of the maximum value of 100 during the 1996–2019 period, thus, signalling that corruption level is high in the nation.
Whereas high corruption and low domestic savings coexist in Nigeria, authors have paid less interest to the empirical linkage between them. Therefore, this study does not only add to existing literature, it is also the first (to our knowledge) to look at the role corruption plays in Nigeria’s saving mobilization. In addition, employing quarterly data makes it easier to have a larger amount of observations which can lead to the generation of more reliable results. Moreover, we believe that the outcomes of our study can guide policymakers and government on the steps to take in boosting Nigeria’s savings. Following the introduction, Section 2 is literature review on corruption-savings nexus (including other drivers of savings). Section 3 is theoretical framework and specification of the model. Section 4 consists of econometric techniques and data, while Section 5 contains discussion of the results. Conclusion comes up in the last section.
2. Review of literature on corruption and savings (including other determinants of savings)
Most studies on savings determinants focused on factors other than corruption as potential influencers of domestic savings. In addition, the few existing empirical studies on corruption-savings relationship looked at the connection between them using cross-section and/or panel data. For example, Dietz et al. (Citation2007) used generalized method of moments (GMM) and fixed effects (FE) estimators to investigate the impacts of three indicators of institutional quality (i.e., lack of corruption, rule of law including bureaucratic quality) in interaction with an indicator of resource abundance on genuine savings in a group consisting of 115 countries for 18 years. The findings imply that lowering corruption level has a significant positive influence on genuine savings in interaction with resource abundance, and the negative influence of resource abundance on genuine savings decreases as corruption reduces. In addition, Swaleheen (Citation2008) employed the GMM and FE methods in evaluating the empirical relationship between savings and corruption across countries. He found that rising corruption level impacts savings negatively. Also, Freytag and Voll (Citation2013) examined the response of savings to institutions in emerging and developing nations by using both system-GMM and FE estimation techniques. The evidence demonstrates that institutions (inclusive of corruption) are important drivers of savings.
Furthermore, Abu et al. (Citation2015) used the ordinary least squares (OLS) and two-stage least squares (TSLS) techniques, which take into consideration random effects (RE) to assess the impact of corruption in interaction with income level on domestic savings in the Economic Community of West African States (ECOWAS) over the 1996–2012 period. The results illustrate that less corruption promote domestic savings, while the negative influence of corruption reduces as income level increases. Also, Boateng et al. (Citation2019) employed the two-step instrumental variable GMM (2S-IV-GMM) method to assess the impact of the quality of institutions on the saving behaviour in Sub-Saharan Africa (SSA) during 1980–2015 period. The results reveal that the quality of institutions (control of corruption inclusive) positively affects savings in SSA.
Studies have also examined the impact of income inequality, consumer confidence, tax-favoured retirement plans or pension schemes, including demographic factors on savings (Alvarez-Cuadrado & Vilalta, Citation2018; Kłopocka, Citation2017; Paiella & Tiseno, Citation2014; Tobing, Citation2012). For instance, Alvarez-Cuadrado and Vilalta (Citation2018) used FE and GMM estimators to evaluate how domestic savings respond to income inequality for a sample consisting of Canada, France, Germany, Japan, the United Kingdom and the United States from 1954 to 2007. The results point to age dependency and income inequality as factors that have a significant negative influence on savings, while GDP growth, interest rates and financial development have a positive influence on savings. The authors also discovered a negative influence of inequality in interaction with financial development on aggregate savings. Moreover, Kłopocka (Citation2017) employed OLS regression method to assess whether consumer confidence affects the saving and borrowing behaviour of households in the Polish economy during the 2002:Q1–2014:Q3 period. The results suggest that consumer confidence is a significant driver of households’ saving and borrowing behaviour. In Italy, Paiella and Tiseno (Citation2014) explored the influence of tax-favoured retirement plans on savings using the OLS and TSLS methods of analysis. The results demonstrate that tax-favoured retirement schemes have not had any significant impact on households’ savings. Tobing (Citation2012) studied the role of demographic variables on savings rates in a sample of 109 high and low income countries. The author’s findings illustrate that demographic variables (such as fertility and survival rates) are important factors that affect savings rates.
Although many studies exist on savings determinants in Nigeria, they concentrated on the impact of non-corruption factors on savings. For example, Imoisi et al. (Citation2018) used the OLS and cointegration methods and found national income and deposit interest rate as the major influencers of domestic savings in the Nigerian economy from 1982 to 2016. Also, Otiwu et al. (Citation2018) employed vector error correction method (VECM) and discovered that the level of income and financial inclusion were the major determinants of savings in Nigeria during the 1981–2015 period. On their part, Mojekwu and Ogbulu (Citation2017) used multiple regression technique to investigate the factors that affect national savings, and confirmed that financial deepening promoted aggregate savings during the 1981–2015 period in Nigeria. Similarly, Ozioma et al. (Citation2016) employed cointegration test and VECM, and established that the level of income and rate of interest have a significant influence on Nigeria’s savings over the 1980–2015 period. In the same vein, Adelakun (Citation2015) used the VECM to determine the core drivers of Nigeria’s savings, and concluded that the rate of inflation and interest rate are significant factors that influence saving behaviour in Nigeria. Also, using the vector error correction technique, Antai et al. (Citation2015) discovered that bank density, per capita income, economic growth, and financial sector development, were the core drivers of savings rates during the 1970–2008 period in Nigeria.
Furthermore, Bamidele et al. (Citation2015) employed a dynamic error correction technique, and found the main factors that impact savings in Nigeria to include current account balance (CAB), per capita income, financial intermediation, rate of real interest, rate of inflation, and financial reforms from 1960 to 2013. In addition, Nwachukwu and Odigie (Citation2011) applied the error correction method to unravel the major factors that explain saving behaviour in Nigeria during 1970–2007. The results demonstrate that disposable income growth rate and real interest rate are the major drivers of savings in Nigeria. Moreover, Wafure and Abu (Citation2009) employed error correction method to assess the main factors that impact domestic savings in Nigeria over the 1981–2007 period. The results confirmed that financial deepening, the number of bank branches, real interest rate, inflation rate, and real per capita income, were the core factors affecting Nigerians’ saving behaviour during the period.
Uremadu (Citation2007) used the OLS method to evaluate the main determinants of Nigeria’s savings from 1980 to 2001, and found growth of income per capita, interest rate spread, broad money supply, and debt service to positively affect savings. But inflation rate and real interest rate are significantly and negatively related to savings. In addition, Nwachukwu and Egwaikhide (Citation2007) employed error correction method, and observed that disposable income, rate of inflation, terms of trade, and foreign debt are significantly and positively related to private savings in Nigeria during 1970–2005. However, real interest rate and disposable income growth are significantly and negatively related to savings over the same period. Similarly, Chete (Citation1999) found that savings rises with per capita income, rate of inflation, real interest rate, and terms of trade growth, but falls with broad money supply-GDP ratio and external debt during 1973–1993 period. A summary of the corruption-savings relationship, including other factors that affect savings is presented in .
Table 1. Summary of empirical literature on corruption and savings relationship (and other determinants of savings).
A survey of the literature indicates that only a few empirical studies have been conducted on corruption-savings relationship at cross-country level, while research focusing on the empirical linkage between the variables in Nigeria is almost non-existent. Thus, the present study extends the literature by evaluating the impact of corruption on Nigeria’s savings using quarterly data for the 1996–2019 period.
3. Theoretical framework and specification of model
In building the savings model authors have relied on the life-cycle theory of Modigliani and Brumberg (Citation1954) which was further popularized by Ando and Modigliani (Citation1963). The theory suggests that income growth and income level including interest rate play important role in savings mobilization (Adewuyi et al., Citation2010; Deaton, Citation1992; Dirschmid & Glatzer, Citation2004; Modigliani, Citation1986), and any model built thereof can be extended to accommodate other factors which might influence savings (Browning & Crossley, Citation2001).
Following the discussion above, some authors submitted that income level captured by GDP per capita (Railaite & Čiutienė, Citation2020) and growth of GDP which is a measure of income or economic growth (Liu & Fan, Citation2020; Roszko-Wójtowicz & Grzelak, Citation2020; Santos-Arteaga et al., Citation2020), account for movements in savings (Athukorala & Sen, Citation2004; Athukorala & Tsai, Citation2003; Metin-Ozcan et al., Citation2003). These studies opined that at higher levels of income level (GDPC), countries save more, while savings decline at low income levels. Similarly, the life-cycle hypothesis argues that growth rate of income (GDPG) has a positive influence on saving behaviour because it (growth rate of income) raises the savings of the working/productive population more than the dissaving of the non-working/unproductive population (Metin-Ozcan et al., Citation2003). However, if the wealth impact from the increase in the level of income encourages consumption rather than savings, then income growth will reduce savings (Boateng et al., Citation2019).
Moreover, within the life-cycle framework, an increase in the interest (deposit) rate (DEPR) raises the opportunity cost of consumption in the current period, leading to a reduction in consumer’s current consumption and an increase in savings (i.e., substitution effect). However, if consumer is a net-lender, the increase in the rate of interest raises consumer’s income and consumption, and consequently, leads to a decline in savings (i.e., income effect). Thus, whether the interest rate will boost or reduce savings depends on the interplay between substitution and income effects (Athukorala & Sen, Citation2004). Recent studies have included the DEPR in their savings models (Antai et al., Citation2015; Boateng et al., Citation2019; Eze & Okpala, Citation2014; Imoisi et al., Citation2018; Otiwu et al., Citation2018; Ozioma et al., Citation2016).
Other variables that are important in explaining savings include inflation rate (Athukorala & Sen, Citation2004; Athukorala & Tsai, Citation2003; Hondroyiannis, Citation2006; Metin-Ozcan et al., Citation2003), and unemployment rate (Abu et al., Citation2019; Athukorala & Tsai, Citation2003). For example, inflation rate (INF) increases the uncertainty of future income streams leading to a rise in savings on precautionary grounds (Athukorala & Sen, Citation2004). Also, rising unemployment (UNEM) comes with socioeconomic costs (Gedek et al., Citation2017), and it captures precautionary savings effect that is related to uncertainty in the economy (Athukorala & Tsai, Citation2003). High unemployment is also a sign that the entire economy and production sector have become more uncertain and riskier. This compels households/individuals to increase their savings for precautionary motive. On the contrary, increases in unemployment can result in a reduction in savings because households/individuals draw on their savings with a view to smoothen their consumption (Athukorala & Tsai, Citation2003).
Furthermore, in line with the view of Bilan et al. (Citation2018) and Yiew et al. (Citation2019) on the importance of changing oil prices in an oil-dependent economy, Abu (Citation2017) and Abu and Gamal (Citation2020) have assigned a significant role to Nigeria’s oil sector when modeling the economy or economic variables therein. They argued that since the economy and government including its citizens depend largely on proceeds from oil exports/sales, changes in oil prices (OILP) can leave a lasting impact on the whole economy as well as its economic variables. For instance, increases in oil prices raise the nation’s income and enhances its citizens’ capacity in financing their consumption and ability to save. Thus, it is expected that an economic variable such as domestic savings will respond to changing oil prices.
In explaining the link between corruption and savings, researchers have relied on the capital flight theory (Abu et al., Citation2015; Swaleheen, Citation2008). In particular, Swaleheen (Citation2008) opined that, unlike private property which is protected by laws, proceeds from corrupt activity are likely to be seized and culprits punished if detected by the state/country. Thus, corrupt public officials usually transfer illegally acquired assets/monies overseas (i.e., capital flight) to avoid been caught and punished (Hutchcroft, Citation1997; Rose-Ackerman, Citation1999). In the same vein, Jain (Citation1988) viewed capital flight within the agency problem context in a political set-up. The author asserted that the privileges bestowed on the agent (leadership) gives him/them the opportunity to borrow funds/monies over the required amount from abroad (for domestic investment), and consequently appropriate a part of these funds (an act of corruption). If the domestic economy’s absorptive capacity is so low that it cannot accommodate the monies borrowed from overseas, it will lower the returns accruing to investment, thus, contributing to capital flight (Swaleheen, Citation2008). As capital flight takes place, measured national (aggregate) savings is reduced by the equivalent amount (Lessard & Williamson, Citation1987; Rodriguez, Citation1987; Swaleheen, Citation2008).
Corruption can also affect savings negatively by reducing public expenditure on health and education; discouraging private sector investment, foreign investment and international capital inflows; lowering the amount of socioeconomic services provided by the state including transfers and subsidies; slowing down growth of the economy; as well as raising unemployment, poverty and inequality levels (Abu et al., Citation2015). Based on the foregoing discussion, this study hypothesizes that domestic savings (SAV) is also affected by the level of corruption (COR). Therefore, the savings model is specified to include corruption and other potential determinants of savings as follows:
(1)
(1)
where
denotes the logarithm of variables taken to reduce skewness. SAV is domestic savings, COR is corruption, GDPC is income level, GDPG is income growth, INF is inflation rate, DEPR is deposit interest rate, OILP is oil price, and UNEM is unemployment rate.
4. Econometric methods and data
This study uses time series (data) from 1996 to 2019. All the data (except corruption index, rate of unemployment including oil price) were gathered from the World Development Indicators. The data include SAV, GDPC, GDPG, INF and DEPR. In addition, data on COR was obtained from the Transparency International, UNEM from the Central Bank of Nigeria and National Bureau of Statistics of Nigeria, and OILP from the International Energy Association and the Organization of Petroleum Exporting Countries databases.
The measurement of the variables goes as follows. SAV is gross or national savings measured in local currency, GDPC is GDP per capita in local currency, and GDPG is real GDP growth. INF is annual percentage change in general prices, and DEPR is the deposit interest rate in percentage. UNEM refers to the share of labour force which is available for and searching for employment but do not have work in percentage, and OILP is the average price of crude oil in international markets. COR is proxied by the TI’s corruption index which is available from the year 1996 only, and this makes it (corruption index) less than the minimum observations required to analyze time series data (i.e., n = 30 or above).
To get around the problem of short data (particularly the index of corruption), the Gandolfo (Citation1981) data interpolation approach was used to convert annual data on all the variables (from 1996 to 2019) to quarterly data. Therefore, we have a higher number of observations, that is, quarterly data from 1997:1–2018:4 (n = 88) since both first and last years of the series/data are eliminated during interpolation. The Gandolfo’s procedure has been employed in previous empirical research (Abu et al., Citation2019; Abu & Karim, Citation2021; Baharumshah et al., Citation2006; Baharumshah & Rashid, Citation1999). Abu et al. (Citation2019) and Abu and Karim (Citation2021) echoed the view of Smith (Citation1998) that interpolated series do not result in bias in estimates generated from cointegrating vectors even when the sample size is small.
4.1. Unit root tests
Given that annual data are employed in this study, it is necessary to ascertain the stationarity status of the series (Ghauri et al., Citation2020). This step is needed to avoid using series that are of order two [I(2)] in estimation exercise and generate misleading/spurious results (Yameogo & Omojolaibi, Citation2021; Zhou et al., Citation2019). To this end, the Augmented Dickey-Fuller (ADF) and Philips-Perron (PP) tests commonly used (Badulescu et al., Citation2020; Dias et al., Citation2020; Qin et al., Citation2021), were applied to ascertain the stationarity status of the series. The ADF equation to be estimated can be specified as:
is the series and
the error term. The ADF equation tests null hypothesis (H0) that the series possesses a unit root (or non-stationary). That is
H0: = 0 (non-stationary)
Against,
An alternative hypothesis (H1) that the series does not have a unit root (stationary). That is:
H1: < 0 (stationary)
As a complementary test, the PP test is also conducted to determine the stationarity status of the series. If the t-statistic from these tests is less compared to the critical value at 10%, 5% or 1%, then the H0 is accepted. On the contrary, if the calculated statistic is higher compared to the critical value, the H0 is not accepted.
4.2. ARDL-bounds test to cointegration
If it is confirmed that the series is I(0) and I(1) mixture, the ARDL-bounds testing to cointegration (Pesaran et al., Citation2001; Pesaran & Shin, Citation1999) will be applied to determine if cointegration exists between corruption and domestic savings, along with other potential determinants of domestic savings.
The justification for applying the ARDL method and its preference over the conventional methods of cointegration of Engle and Granger (Citation1987), Johansen (Citation1988; Citation1991), and Johansen and Juselius (Citation2009), has been explained by several studies (Abu et al., Citation2021; Abu & Staniewski, Citation2019; Nyasha & Odhiambo, Citation2015; Vlahinic & Jakovac, Citation2014). For example, the ARDL method can be used to assess the existence of cointegration whether the series are integrated to order one (i.e., I(1)), or a combination of I(1) and order zero (i.e., I(0)). In addition, the method can be employed to analyze small sample, but this is not the case with the conventional methods of cointegration because they require a relatively large sample size to be valid. Furthermore, whereas the conventional techniques of cointegration do not permit for different lags, the ARDL method allows the variables to possess varying lags. Finally, whereas the conventional methods of cointegration employ a system of equations, the ARDL technique uses a single equation in estimating both the short-run and long-run coefficients simultaneously. The ARDL model () is written as:
(2)
(2)
The first step is to conduct the bounds test on the H0 that there is no cointegration among the variables. That is,
Against the H1 which states that cointegration exists among the variables. That is,
Next, is to compare the calculated F-statistic with both the lower and upper critical bounds values, [I(0)] and [I(1)], respectively. If the F-statistic is larger than the (I(1)), it is concluded that cointegration exists between the variables. But if the computed statistic is lower than the (I(0)), it is concluded that cointegration does not exist between them. Moreover, if the F-statistic lies between the (I(1)) and (I(0)), then the inference will be inconclusive.
Once cointegration has been established, both long-run and short-run parameters can be estimated from the short-run and long-run models that are expressed as EquationEquations (3)(3)
(3) and Equation(4)
(4)
(4) as follows:
(3)
(3)
and
(4)
(4)
represents error correction variable, and
denotes the speed at which equilibrium is restored in case of any disturbance in the short-term.
4.3. Diagnostic tests
After the model estimation, diagnostic tests will be performed to evaluate the validity of generated results. This is achieved by using the Breusch-Godfrey serial-correlation test (Breusch, Citation1978; Godfrey, Citation1978a) to check for the existence of serial-correlation. The Breusch-Pagan-Godfrey heteroscedasticity test (Breusch & Pagan, Citation1979; Godfrey, Citation1978b) is used to ascertain the presence of heteroscedasticity. Lastly, Ramsey test (Ramsey, Citation1969) is applied to evaluate whether the model is well specified.
4.4. Stability tests
In evaluating the model and parameters stability, two tests namely - the cumulative sum of squares of recursive residuals (CUSUMS) and cumulative sum of recursive residuals (CUSUM) tests will be employed. If the CUSUMS plots break out of the upper bound or lower bound, it will be inferred that both the model and parameters are not stable in the long-term (see Greene, Citation2003).
5. Results and discussion
5.1. Descriptive statistics
Prior to estimation of a model, it is important to compute the descriptive statistics for the variables employed (Zhang et al., Citation2021). The results in illustrate that the mean log of domestic savings (LSAV) is 12.31, and the average corruption index is 2.94. In addition, the mean log of income level measured by GDP per capita (LGDPC) and income growth (GDPG) are 4.86 and 1.33, respectively.
Table 2. Descriptive statistics.
Also, the average inflation rate is 2.89, while the mean deposit rate is 2.62. Lastly, average log of oil price (LOILP) and mean unemployment rate (UNEM) are 1.06 and 3.74, respectively.
5.2. Results of unit root tests
shows the results of unit root tests. The results suggest that INF is stationary at level, while the remaining variables including LSAV, COR, LGDPC, GDPG, DEPR, LOILP and UNEM are stationary only after taking their first difference.
Table 3. Results of unit root tests.
Thus, the series are integrated to order I(0) and I(1), and this provides justification for the bounds test to cointegration approach.
5.3. Results of ARDL-bounds test to cointegration
The bounds test to cointegration results in reveal that F-statistic (4.59) is higher than the upper critical bound (i.e., 3.90) at the 1% level.
Table 4. Results of bounds test to cointegration.
This confirmation of cointegration demonstrates the existence of a long-term relationship among the variables.
5.4. Results of estimation of ARDL model
In estimation exercise the optimal lag-length chosen by the Akaike Information Criterion (AIC) is 4,3,1,0,1,3,2,4. The AIC was applied because it is adjudged to be superior to other lag selection criteria in small sample (Liew, Citation2004). The results of estimation () show that a reduction in the level of corruption (COR) leads to higher domestic savings (LSAV) both in the long-run and short-run. An increase in corruption index (i.e., lowering corruption level) by 1 point results in a 0.05% and a 0.03% increase in domestic savings in the long-run and short-run, at 5% level and 1% level, respectively. This finding supports the ones reported by early studies (Abu et al., Citation2015; Swaleheen, Citation2008). For example, Abu et al. (Citation2015) found that lowering corruption level results to an increase in domestic savings in ECOWAS countries. In the same vein, Swaleheen (Citation2008) confirmed that reducing corruption promotes savings across countries. Similarly, Boateng et al. (Citation2019) discovered that the quality of institutions (corruption inclusive) is a strong determinant of savings in SSA (including Nigeria). Also, the work of Freytag and Voll (Citation2013) suggests that institutions (inclusive of corruption) matter in the mobilization of savings.
Table 5. Results of estimation of ARDL model.
Income level measured by per capita GDP (LGDPC) is positive and significantly related to savings over the long-run. An increase in income level by 1% causes domestic savings to rise by a 1.15% over the long-run at the 5% level. This finding is line with the outcome of past studies (Abu et al., Citation2015; Athukorala & Sen, Citation2004; Athukorala & Tsai, Citation2003). It also supports the works of Olusoji (Citation2003) and Nwachukwu and Egwaikhide (Citation2007) that savings rises with the level of income in Nigeria. However, income growth (GDPG) is insignificant in explaining savings even in the long-term, and it is consistent with prior research on Nigeria (Mojekwu & Ogbulu, Citation2017; Ogbuabor et al., Citation2013).
Inflation (INF) has a negative and significant effect on savings in the short-run, while its impact is positive in the long-run. A 1% rise in inflation results in a 0.02% decrease and a 0.04% increase in savings in the short-run and the long-run, at 1% level and 5% level, respectively. The negative impact of inflation on domestic savings is consistent with the ones reported by prior studies (Adewuyi et al., Citation2010; Uremadu, Citation2007). For instance, Uremadu (Citation2007) confirmed that inflation has a significant negative influence on domestic savings in Nigeria, and Adewuyi et al. (Citation2010) discovered a negative impact of inflation on domestic savings in the case of ECOWAS countries (Nigeria inclusive). However, the positive impact of inflation lends support to prior research (Athukorala & Sen, Citation2004; Freytag & Voll, Citation2013; Nwachukwu & Egwaikhide, Citation2007). For example, Nwachukwu and Egwaikhide (Citation2007) reported that inflation promotes domestic savings in the Nigerian economy, while Athukorala and Sen (Citation2004) discovered a similar result for India.
Deposit interest rate (DEPR) has a significant positive influence on domestic savings. A 1% rise in deposit rate results in a 0.11% and a 0.10% increase in domestic savings in both the short-term and long-term, respectively, at the 1% level. This empirical finding is consistent with similar studies on Nigeria (Eze & Okpala, Citation2014; Imoisi et al., Citation2018; Ozioma et al., Citation2016).
Also, oil price (LOILP) does have a significant positive impact on domestic savings in both the short-term and long-term. If oil price increases by 1%, it will result in a 0.89% and a 0.90% increase in savings in the short-term and long-term, respectively, at 1% level.
Unemployment (UNEM) is negatively and significantly related to savings in both the long-term and the short-term. A 1% rise in unemployment causes savings to decrease by a 0.02% and a 0.06% in the short-term and long-term, at the 1% level and the 5% level, respectively. This outcome is consistent with related studies on Nigeria (Abu et al., Citation2019). The error correction term is significant and its coefficient is correctly signed (i.e., −0.31), indicating that approximately 31% of the deviations from equilibrium would be corrected during the 4th quarter of the year.
These empirical outcomes have some implications. The positive impact of reducing the level of corruption on domestic savings suggests that lowering corruption lessens capital flight and consequently raise Nigeria’s domestic savings. It also implies that rising corruption promotes capital flight which consequently lowers national savings. This finding portrays the Nigeria’s situation where massive corruption went unabated particularly in the public sector under various military administrations. For example, during the late General Sani Abacha’s led government several billions of Naira (local currency) were stolen from the nation’s coffers by top military and government officials and stashed away in foreign banks (capital flight). Efforts by former President (Olusegun Obasanjo) and the incumbent President (Muhammadu Buhari) to tackle corruption in collaboration with the international community have led to millions of dollars repatriated to Nigeria.
The positive influence of the level of income on domestic savings supports the claim that high income countries save more compared to their low income counterparts (Athukorala & Sen, Citation2004). This evidence provides support for Nigeria where many earn less than $2 per day. This low level of income implies that the average Nigerian cannot finance his/her consumption adequately and is unable to save. Moreover, the insignificant influence of growth rate of income on domestic savings suggests that the wealth impact of any increase in the level of income of Nigerians largely encourages consumption rather than promoting savings.
In addition, the positive impact of deposit interest rate on domestic savings implies that rising deposit rate promotes saving behaviour. The deposit rate in Nigeria has remained very low over the years, and it might have discouraged many to save in the face of high inflation rate.
The negative effect of inflation demonstrates that the high inflation in Nigeria raises uncertainty in respect of future value of income, and therefore discourages savings. Also, rising inflation lowers people’s purchasing power including their ability to save (Freytag & Voll, Citation2013). However, the positive influence of inflation on domestic savings supports the view that high inflation (rising macroeconomic uncertainty) compels people to save a portion of their income for precautionary reasons. This is the case with individuals in developing economies Nigeria inclusive whose income streams are more uncertain compared to their counterparts in wealthy nations (Athukorala & Sen, Citation2004; Deaton, Citation1989; Hondroyiannis, Citation2006; Kimball, Citation1990).
The positive sign of oil price coefficient suggests that higher price of oil raises the nation’s overall income levels including citizens’ ability to meet their consumption needs and consequently save the remainder. The negative relationship between unemployment and domestic savings illustrates that high unemployment (which has always been the case in Nigeria) forces individuals to draw down savings in an attempt to smoothen their consumption (Athukorala & Sen, Citation2004). In addition, when people are unemployed they do not earn income which further reduces their ability to save.
5.5. Results of diagnostic tests
presents the diagnostic tests results. The Breusch-Godfrey serial-correlation test result implies that the chi-square is 2.6030 and its probability is 0.2721. The Breusch-Pagan-Godfrey heteroscedasticity test result illustrates that the chi-square is 19.3690 with a probability value of 0.7791. Lastly, the Ramsey test result demonstrates that the F-statistic is 0.0518 and its probability is 0.8206.
Table 6. Results of diagnostic tests.
These findings reveal that the estimated model is well specified, and it is free from serial-correlation and heteroscedasticity problems.
5.6. Results of stability tests
The stability test results for the model using the CUSUMS and CUSUM tests are shown in and .
The CUSUM () and CUSUMS () plots lie within the boundaries, thus, confirming the stability of the model and the long-run coefficients of the regressors.
6. Conclusion
This study assesses the influence of corruption on Nigeria’s domestic savings from 1996 to 2019. Using the ARDL method to analyze quarterly data during this period, we discover that a long-term relationship exists among corruption and domestic savings along with other control variables. The results show that lowering the level of corruption raises domestic savings in Nigeria over the long-term. This finding supports the claim that high corruption induces capital flight, leading to a reduction in domestic savings, and vice versa. Other long-term significant drivers of domestic savings in Nigeria are income level, interest rate, oil price, rate of inflation, and unemployment rate. Having established that corruption discourages domestic savings, we offer some recommendations.
First, government is advised to sustain the ongoing war against corruption by increasing the funding of the anti-graft bodies (i.e., EFCC and ICPC). Coupled with this is the urgent need for proper monitoring and evaluation of the operations/activities of anti-corruption agencies so that recovered funds and assets are not re-looted or mismanaged by those saddled with the responsibility of apprehending, prosecuting and recovering of proceeds of corruption. Second, the judiciary should be strengthened and repositioned to be more effective and efficient to complement the fight against corruption. This will ensure that justice is dispensed without delay, and those found to be corrupt are punished or sanctioned accordingly. Third, government is advised to seek international community’s support so that corrupt individuals don’t have hidden place to keep stolen public funds, and monies illegally acquired and stashed away in foreign banks are repatriated to Nigeria. Fourth, government is encouraged to promote enlightenment/awareness campaign on the adverse impact of corruption on the economy. These measures can be complemented with policies to raise the levels of income, deposit interest rate, oil production, including reducing unemployment and inflation rates.
Lastly, we believe that our study has contributed to the literature on savings because it is the first (to our knowledge) to uncover the significant role corruption plays in savings mobilization in Nigeria. However, there are certain limitations inherent in this study which future research should make efforts to address. Future studies can employ other robust estimation methods including the bootstrap ARDL approach and/or threshold regression analysis. Also, aggregate institutions or its components (other than corruption) can be considered as potential drivers of domestic savings by future research as it may provide additional information on the role institutions (and its components) play in savings mobilization in Nigeria.
Disclosure statement
No potential conflict of interest was reported by the authors.
References
- Abu, N. (2017). Does Okun’s law exist in Nigeria? Evidence from the ARDL bounds test approach. Contemporary Economics, 11(2), 131–144. https://doi.org/10.5709/ce.1897-9254.232
- Abu, N., & Gamal, A. A. M. (2020). An empirical investigation of the twin deficits hypothesis in Nigeria: Evidence from cointegration techniques. Contemporary Economics, 14(3), 285–305. https://doi.org/10.5709/ce.1897-9254.405
- Abu, N., Gamal, A. A. M., Sakanko, M. A., Mateen, A., Joseph, D., & Amaechi, B. O. O. (2021). How have COVID-19 confirmed cases and deaths affected stock markets? Evidence from Nigeria. Contemporary Economics, 15(1), 76–100. https://doi.org/10.5709/ce.1897-9254.437
- Abu, N., Kadandani, B., Obi, B., & Modibbo, M. (2019). How does pensions affect savings in Nigeria? Evidence from quarterly data. Scientific Annals of Economics and Business, 66(4), 541–558. https://doi.org/10.2478/saeb-2019-0038
- Abu, N., & Karim, M. Z. A. (2021). Is the relationship between corruption and domestic investment non-linear in Nigeria? Empirical evidence from quarterly data. Estudios de Economia Aplicada, 39(3), 1–18. https://doi.org/10.25115/eea.v39i3.3953
- Abu, N., Karim, M. Z. A., & Aziz, M. I. A. (2015). Low savings rates in the Economic Community of West African States (ECOWAS): The role of corruption. Journal of Economic Cooperation and Development, 36(2), 63–90.
- Abu, N., & Staniewski, M. W. (2019). Determinants of corruption in Nigeria: Evidence from various estimation techniques. Economic Research-Ekonomska Istraživanja, 32(1), 3058–3076. https://doi.org/10.1080/1331677X.2019.1655467
- Adelakun, O. J. (2015). An investigation of the determinants of savings and investment in Nigeria. International Economics and Business, 1(2), 1–16. https://doi.org/10.5296/ieb.v1i2.8688
- Adewuyi, A. O., Bankole, A. S., & Arawomo, D. F. (2010). What determines saving in the Economic Community of West African States (ECOWAS)? Journal of Monetary and Economic Integration, 10(2), 71–99.
- Alvarez-Cuadrado, F., & Vilalta, M. (2018). Income inequality and saving. Oxford Bulletin of Economics and Statistics, 80(6), 1029–1061. https://doi.org/10.1111/obes.12236
- Ando, A., & Modigliani, F. (1963). The ‘life cycle’ hypothesis of saving: Aggregate implications and tests. American Economic Review, 53(1), 55–84.
- Antai, A., Sylvester, I. J. J., & Anam, B. E. (2015). Estimation of the short run and long run determinants of domestic savings rate in Nigeria (1970–2008). International Journal of Innovative Research in Technology, Basic and Applied Sciences, 1(1), 40–58.
- Athukorala, P., & Sen, K. (2004). The determinants of private saving in India. World Development, 32(3), 491–503. https://doi.org/10.1016/j.worlddev.2003.07.008
- Athukorala, P., & Tsai, P. (2003). Determinants of household saving in Taiwan: Growth, demography and public policy. Journal of Development Studies, 39(5), 65–88. https://doi.org/10.1080/00220380412331333149
- Badulescu, A., Badulescu, D., Simut, R., & Dzitac, S. (2020). Tourism – Economic growth nexus. Technological and Economic Development of Economy, 26(4), 867–884. https://doi.org/10.3846/tede.2020.12532
- Baharumshah, A. Z., Lau, E., & Khalid, A. M. (2006). Testing twin deficits hypothesis using VARs and variance decomposition. Journal of the Asia Pacific Economy, 11(3), 331–354. https://doi.org/10.1080/13547860600764245
- Baharumshah, A. Z., & Rashid, S. (1999). Export, imports and economic growth in Malaysia: Empirical evidence based on multivariate time series. Asian Economic Journal, 13(4), 389–406. https://doi.org/10.1111/1467-8381.00092
- Bamidele, A., Jibrin, M., & Isa, A. (2015). Determinants of domestic gross savings in Nigeria: Lessons for policy. CBN Economic and Financial Review, 53(3), 16–37.
- Bilan, Y., Gedek, S., & Mentel, G. (2018). The analysis of oil price and Ruble exchange rate. Transformations in Business & Economics, 17(3), 195–205.
- Boateng, E., Agbola, F. W., & Mahmood, A. (2019). Does the quality of institutions enhance savings? The case of Sub-Saharan Africa. Applied Economics, 51(58), 6235–6263. https://doi.org/10.1080/00036846.2019.1616066
- Breusch, T. S. (1978). Testing for autocorrelation in dynamic linear models. Australian Economic Papers, 17(31), 334–355. https://doi.org/10.1111/j.1467-8454.1978.tb00635.x
- Breusch, T. S., & Pagan, A. R. (1979). A simple test for heteroscedasticity and random coefficient variation. Econometrica, 47(5), 1287–1294. https://doi.org/10.2307/1911963
- Browning, M., & Crossley, T. F. (2001). The life cycle model of consumption and saving. Journal of Economic Perspectives, 15(3), 3–22. https://doi.org/10.1257/jep.15.3.3
- Chete, L. N. (1999). Macroeconomic determinants of private savings in Nigeria. NISER Monograph Series, 7.
- Das, G. G., & Drine, I. (2020). Distance from the technology frontier: How could Africa catch-up via socio-institutional factors and human capital? Technological Forecasting and Social Change, 150, 119755. https://doi.org/10.1016/j.techfore.2019.119755
- Deaton, A. (1989). Saving in developing countries: Theory and review. The World Bank Economic Review, 3(suppl 1), 61–108. https://doi.org/10.1093/wber/3.suppl_1.61
- Deaton, A. (1992). Understanding consumption. Oxford University Press.
- Dias, R., Teixeira, N., Machova, V., Pardal, P., Horak, J., & Vochozka, M. (2020). Random walks and market efficiency tests: Evidence on US, Chinese and European capital markets within the context of the global Covid-19 pandemic. Oeconomia Copernicana, 11(4), 585–608. https://doi.org/10.24136/oc.2020.024
- Dietz, S., Neumayer, E., & De Soysa, I. (2007). Corruption, the resource curse and genuine saving. Environment and Development Economics, 12(1), 33–53. https://doi.org/10.1017/S1355770X06003378
- Dirschmid, W., & Glatzer, E. (2004). Determinants of the household saving rate in Austria. Monetary Policy & the Economy, Q4, 25–38.
- Engle, R. F., & Granger, C. W. J. (1987). Co-integration and error correction: Representation, estimation and testing. Econometrica, 55(2), 251–276. https://doi.org/10.2307/1913236
- Eze, T. C., & Okpala, C. S. (2014). Determinants of private domestic savings in Nigeria (1970–2010). Journal of Economics and Sustainable Development, 5(8), 146–155.
- Freytag, A., & Voll, S. (2013). Institutions and savings in developing and emerging economies. Public Choice, 157(3-4), 475–509. https://doi.org/10.1007/s11127-013-0121-7
- Gandolfo, G. (1981). Quantitative analysis and econometric estimation of continuous time dynamic. North-Holland.
- Gedek, S., Misiak, T., & Mentei, G. (2017). Changes in GDP and the employment and unemployment in the European Union. Transformations in Business & Economics, 16(3C), 42–59.
- Ghauri, S. P., Ahmed, R. R., Streimikiene, D., & Streimikis, J. (2020). Forecasting exports and imports by using autoregressive (AR) with seasonal dummies and Box-Jenkins approaches: A case of Pakistan. Engineering Economics, 31(3), 291–301. https://doi.org/10.5755/j01.ee.31.3.25323
- Godfrey, L. G. (1978a). Testing for higher order serial correlation in regression equations when the regressors include lagged dependent variables. Econometrica, 46(6), 1303–1310. https://doi.org/10.2307/1913830
- Godfrey, L. G. (1978b). Testing for multiplicative heteroscedasticity. Journal of Econometrics, 8(2), 227–236. https://doi.org/10.1016/0304-4076(78)90031-3
- Greene, W. (2003). Econometric Analysis (5th ed.). Prentice Hall.
- Hondroyiannis, G. (2006). Private saving determinants in European countries: A panel co-integration approach. The Social Science Journal, 43(4), 553–569. https://doi.org/10.1016/j.soscij.2006.08.004
- Hutchcroft, P. D. (1997). The politics of privilege: Assesing the impact of rents, corruption, and clientelism on third world debt. Political Studies, 45(3), 639–658. https://doi.org/10.1111/1467-9248.00100
- Imoisi, A. I., Iyafekhe, C., & Ezeibekwe, F. O. (2018). Determinants of aggregate savings in Nigeria. Accounting & Taxation Review, 2(1), 186–198.
- Jain, A. K. (1988). An agency theoretic explanation of capital flight. Economics Letters, 28(1), 41–45. https://doi.org/10.1016/0165-1765(88)90069-9
- Johansen, S. (1988). Statistical analysis of cointegration vectors. Journal of Economic Dynamics and Control, 12(2-3), 231–254. https://doi.org/10.1016/0165-1889(88)90041-3
- Johansen, S. (1991). Estimation and hypothesis testing of cointegration vectors in Gaussian vector autoregressive models. Econometrica, 59(6), 1551–1580. https://doi.org/10.2307/2938278
- Johansen, S., & Juselius, K. (2009). Maximum likelihood estimation and inference on cointegration-with applications to the demand for money. Oxford Bulletin of Economics and Statistics, 52(2), 169–210. https://doi.org/10.1111/j.1468-0084.1990.mp52002003.x
- Kimball, M. (1990). Precautionary saving in the small and the large. Econometrica, 58(1), 53–73. https://doi.org/10.2307/2938334
- Kłopocka, A. M. (2017). Does consumer confidence forecast household saving and borrowing behavior? Evidence for Poland. Social Indicators Research, 133(2), 693–717. https://doi.org/10.1007/s11205-016-1376-4
- Lessard, D. R., & Williamson, J. (1987). The problem and policy responses. In D. R. Lessard, and J. Williamson (Eds.), Capital flight and third world debt (pp. 201–252). Institute for International Economics.
- Liew, V. K-S. (2004). Which lag length selection criteria should we employ?. Economics Bulletin, 3(33), 1–9.
- Liu, N., & Fan, F. (2020). Threshold effect of international technology spillovers on China's regional economic growth. Technology Analysis & Strategic Management, 32(8), 923–935. https://doi.org/10.1080/095375.2020.1729977
- Lucas, R. (1988). On the mechanics of economic development. Journal of Monetary Economics, 22(1), 3–42. https://doi.org/10.1016/0304-3932(88)90168-7
- Metin-Ozcan, K., Gunay, A., & Ertac, S. (2003). Determinants of private savings behaviour in Turkey. Applied Economics, 35(12), 1405–1416. https://doi.org/10.1080/0003684032000100373
- Modigliani, F. (1986). Life cycle, individual thrift, and the wealth of nations. American Economic Review, 76, 297–313.
- Modigliani, F., & Brumberg, R. (1954). Utility analysis and the consumption function: An interpretation of cross-section data. Franco Modigliani, 1(1), 388–436.
- Mojekwu, R. O., & Ogbulu, O. M. (2017). Determinants of national savings in Nigeria: A multivariate analysis (1981–2015). Journal of Finance, Banking and Investment, 4(1), 112–132.
- Nwachukwu, T. E., & Odigie, P. (2011). What drives private saving in Nigeria. AERC Research Paper, 212, 1–32.
- Nwachukwu, T. E., & Egwaikhide, F. O. (2007). An error-correction model of the determinants of private saving in Nigeria [Paper presentation]. 12th African Econometrics Society Annual Conference, South Africa.
- Nyasha, S., & Odhiambo, N. M. (2015). The impact of banks and stock market development on economic growth in South Africa: An ARDL-bounds testing approach. Contemporary Economics, 9(1), 93–108. https://doi.org/10.5709/ce.1897-9254.161
- Ogbuabor, J. E., Malaolu, V. A., & Mba, I. C. (2013). Informality and domestic savings in Nigeria: Lessons from time series analysis. International Journal of Development and Economic Sustainability, 1(3), 24–32.
- Olusoji, M. O. (2003). Determinants of private savings in Nigeria: An error correction approach. NDIC Quarterly, 13, 85–96.
- Otiwu, K., Okere, P. A., & Uzowuru, L. N. (2018). Determinants of private domestic savings in Nigeria (1981–2015). International Journal for Innovation Education and Research, 6(2), 21–40. https://doi.org/10.31686/ijier.vol6.iss2.938
- Ozioma, E.-O. F., Idenyi, O. S., Chinyere, U. C., & Eze, A. O. (2016). The determinants of domestic private savings in Nigeria. British Journal of Economics, Management & Trade, 15(2), 1–16. https://doi.org/10.9734/BJEMT/2016/27712
- Paiella, M., & Tiseno, A. (2014). Evaluating the impact on saving of tax- favored retirement plans. Journal of Pension Economics and Finance, 13(1), 62–87. https://doi.org/10.1017/S147474721300019X
- Pesaran, M. H., & Shin, Y. (1999). An autoregressive distributed lag modeling approach to cointegration analysis. In S. Strom (Ed.), Econometrics and economic theory in the 20th century. The Ragnar Frisch Centennial Symposium Econometric Society monographs, 31 (pp. 371–413). Cambridge University Press.
- Pesaran, M. H., Shin, Y., & Smith, R. (2001). Bound testing approaches to the analysis of level relationship. Journal of Applied Econometrics, 16(3), 289–326. https://doi.org/10.1002/jae.616
- Qin, Y., Wang, X., Xu, Z., & Škare, M. (2021). The impact of poverty cycles on economic research: Evidence from econometric analysis. Economic Research-Ekonomska Istraživanja, 34(1), 152–171. https://doi.org/10.1080/1331677X.2020.1780144
- Railaite, R., & Čiutienė, R. (2020). The impact of public health expenditure on health component of human capital. Engineering Economics, 31(3), 371–379. https://doi.org/10.5755/j01.ee.31.3.25158
- Ramsey, J. B. (1969). Tests for specification errors in classical linear least‐squares regression analysis. Journal of the Royal Statistical Society: Series B (Methodological), 31(2), 350–371.
- Rodriguez, F. M. A. (1987). Consequences of capital flight for Latin American debtor countries. In D. R. Lessard & J. Williamson (Eds.), Capital flight and third world debt (pp. 129–151). Institute for International Economics.
- Romer, P. (1986). Increasing returns and long-run growth. Journal of Political Economy, 94(5), 1002–1037. https://doi.org/10.1086/261420
- Rose-Ackerman, S. (1999). Corruption and government: Causes, consequences and reform. Cambridge University Press.
- Roszko-Wójtowicz, E., & Grzelak, M. M. (2020). Macroeconomic stability and the level of competitiveness in EU member states: a comparative dynamic approach. Oeconomia Copernicana, 11(4), 657–688. https://doi.org/10.24136/oc.2020.027
- Santos-Arteaga, F. J., Tavana, M., Torrecillas, C., & Di Caprio, D. (2020). Innovation dynamics and financial stability: A European Union perspective. Technological and Economic Development of Economy, 26(6), 1366–1398. https://doi.org/10.3846/tede.2020.13521
- Smith, S. F. (1998). Cointegration tests when data are linearly interpolated. Unpublished paper. State University of New York at Albany.
- Solow, R. (1956). A contribution to the theory of economic growth. The Quarterly Journal of Economics, 70(1), 65–94. https://doi.org/10.2307/1884513
- Swaleheen, M. (2008). Corruption and savings in a panel of countries. Journal of Macroeconomics, 30, 1285–1301. https://doi.org/10.1016/j.jmacro.2007.05.002
- Tobing, E. (2012). Demography and cross-country differences in savings rates: A new approach and evidence. Journal of Population Economics, 25(3), 963–987. https://doi.org/10.1007/s00148-011-0386-4
- Uremadu, S. O. (2007). Core determinants of financial savings in Nigeria: An empirical analysis for national monetary policy formulation. International Review of Business Research Papers, 3, 356–367.
- Vlahinic, N., & Jakovac, P. (2014). Revisiting the energy consumption-growth nexus for Croatia: New evidence from a multivariate framework analysis. Contemporary Economics, 8(4), 435–452. https://doi.org/10.5709/ce.1897-9254.155
- Wafure, O. G., & Abu, N. (2009). Long-run determinants of savings in Nigeria, 1981–2007: Evidence from time series data. Nigerian Journal of Economics and Management, 4(1), 87–106.
- Yameogo, C. E. W., & Omojolaibi, J. A. (2021). Trade liberalisation, economic growth and poverty level in sub-Saharan Africa (SSA). Economic Research-Ekonomska Istraživanja, 34(1), 754–774. https://doi.org/10.1080/1331677X.2020.1804428
- Yiew, T. H., Yip, C. Y., Tan, Y. L., Habibullah, M. S., & Alih Khadijah, C. (2019). Can oil prices predict the direction of exchange rate movements? An empirical and economic analysis for the case of India. Economic Research-Ekonomska Istraživanja, 32(1), 812–823. https://doi.org/10.1080/1331677X.2018.1559746
- Zhang, N., Wang, A., Haq, N., & Nosheen, S. (2021). The impact of COVID-19 shocks on the volatility of stock markets in technologically advanced countries. Economic Research-Ekonomska Istraživanja, 1–26. https://doi.org/10.1080/1331677X.2021.1936112
- Zhou, C., Hong, J., Wu, Y., & Marinova, D. (2019). Outward foreign direct investment and domestic innovation performance: Evidence from China. Technology Analysis & Strategic Management, 31(1), 81–95. https://doi.org/10.1080/09537325.2018.1485890