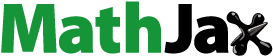
Abstract
The nexus between economic growth (EG) and carbon emission has been examined extensively, specifically in consumption-based CO2. However, the role of human capital, green energy, and sustainable economic growth in determining the carbon emission is yet to be explored specifically from emerging economies. This study aimed to examine the impact of human capital index, green energy, EG in terms of GDP, and square of GDP on carbon emission for long-short run with the help of CS-ARDL. The data for study variables was collected from 1995 to 2018. The study findings confirmed that there exists CSD, cointegration, and slope heterogeneity among the study variables. In contrast, the output through CS-ARDL indicated that the main reason for higher carbon emission in the targeted economies are economic growth under long-short run estimation. Additionally, the role of green energy and human capital index is also constructive in lowering the environmental degradation for both long-run and short-run estimation. Finally, some policy implications are also convassed at the end of the research.
1. Introduction
For achieving positive environmental outcomes, the titles like green growth and sustainable economic growth have gained much attention in recent years(Özer et al., Citation2020). Several scholars argued that attaining economic growth (EG) with environmental sustainability is also considered an important nexus in front of various policymakers, specifically in the field of sustainable environment (Chien et al., Citation2021; Corrás-Arias, Citation2020; Sadiq, Nonthapot et al., 2021). Having said that, to attain that economic growth with lower environmental impact, the dependability of conventional energy sources like fossil fuel is much needed, although adverse consequences for the environment can be seen over a longer time. Meanwhile, the promotion of green growth in any economy also depends on the utilisation of those energy sources which are clean or also known as renewable (Bruneckiene et al., Citation2020; Castillo-Vergara et al., Citation2021; W. Li et al., Citation2021). Renewable energy sources would provide the outcomes in the form of green energy, efficient generation and utilisation of different energy sources, conservation of natural resources like coal and oil, and finally lowering the amount of carbon emissions (Suki et al., Citation2022).Withal the ongoing demand for energy, specifically from traditional sources, has some serious environmental challenges. For this reason, the usage of clean technologies to combat such challenges needs some time for both developing and developed economies (Hao et al., Citation2021; Ključnikov et al., Citation2020; Tsunga et al., Citation2020).
Sharif et al. (Citation2020), Sadiq, Hsu, et al. (Citation2021) argued that the increased energy demand surely poses some serious threats and challenges (Sohag et al., Citation2019). Thereby, cleaner technologies are needed to combat the said challenges and promote CO2 emission reduction. A pile of studies suggest that cleaner energy should be promoted to reduce the dependence on non-renewable energy (Bhattacharya et al., Citation2016; Chien et al., Citation2021). In addition, using energy sources which are environment-friendly are crucial when it comes to CO2 emission (Danielle, Citation2020; Kirikkaleli & Sowah, Citation2020; Xiang et al., Citation2021). Furthermore, the use of sustainable and green energy sources goes side by side in economies with lower reliability on traditional energy sources. However, one of the key points that is widely ignored in the literature is that economic growth from a sustainable perspective is only possible if there is a regular checkup of environmental degradation. This would justify the sustainability of natural capital stock along with the usage of some renewable energy sources so that both economic growth and environmental sustainability side by side can provide positive outcomes (Dadelo, Citation2020; Daniel, Citation2021; Z. Liu et al., Citation2021; Shair et al., Citation2021; Sharif et al., Citation2021). Turning towards the energy consumption (EC) pattern in E7 economies, it was found that China has been on the top of the ranking for the last many decades. However, an increasing trend for energy consumption is also observed among other member states such as Russia, India, Mexico, Brazil, Indonesia, and Turkey, as shown in of the study.
Figure 1. Energy Consumption in E7 Economies from 1965 to 2017.
Source: Tong et al. (Citation2020)
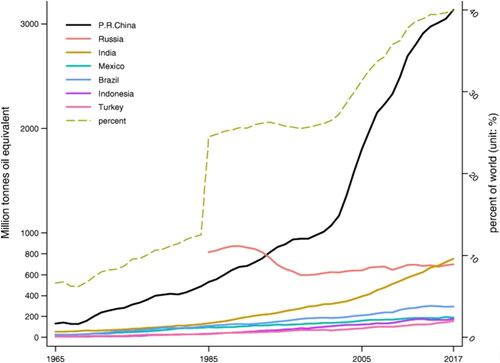
The prime purpose of the current study is to scrutinise the impact of EG, green energy utilisation, and human capital index on the carbon emission of E7 economies from 1995 to 2018. The reason to select the E7 economies sample is that they are among those emerging markets that have their growing economic growth in terms of GDP. Meanwhile, the consumption of energy in all these economies is showing an increasing trend over the last many decades, which specifies a higher level of environmental degradation. However, various steps have been taken by the government in these economies to control CO2 emission from different sources. More specifically, the growing trend of EC and greenhouse gas emissions in China is higher compared to the rest of the sample economies. These facts have provided a reasonable justification to conduct an empirical investigation. The study sample of E7 is observed as non-homogenous based on the EC, EG, ecological innovation, and, more specifically, the investment towards human capital. For this reason, the findings of this study may guide the stakeholders, specifically those responsible for the polices in terms of environmental sustainability.
The contribution of our study in the present body of literature can be viewed from a variety of perspectives. Firstly, the investigation was conducted while taking the role of green energy, human capital index, and EG in dealing with CO2 emissions, specifically from the context of E7 economies. Secondly, this study has considered a range of methodological contributions while analyzing the CSD, unit root properties, cointegration among the study variables, and checking for the heterogeneity. Furthermore, this research has implemented the CSR-ARDL as a methodological tool to explore whether long and short run are associated or not. The structure of the remaining paper is given as follows. Discussion related to theoretical and empirical literature is conducted under Section 2. A comprehensive description of the study variables along with the methods is provided under Section 3. The analysis and discussion part of the study is provided under Section 4. Finally, the 5th section covers the conclusion along with some policy implications.
2. Literature review
Earlier researchers presented both theoretical and empirical literature covering the various determinants of CO2 emissions in developed and emerging economies. For this purpose, different studies have used other variables such as environmental determinants and macroeconomics. At the same time, a range of measures has been presented to justify the title of environmental degradation. However, one of the most cited measured is known as CO2 emissions. For instance Hao et al. (Citation2021) and Sharif, Afshan, Chrea, Amel, and Khan et al. (Citation2020) have investigated green growth and low carbon emissions for the G7 economies. In addition, environmental taxes and human capital index role were also observed and the CS-ARDL approach was used. The finding of the study validated that elements like green growth, human capital, and energy from several renewable sources played a significant role in reducing carbon emissions in the natural surroundings. Bano et al. (Citation2018) and Sharif et al. (Citation2017) and have scrutinised the relationship of human capital with carbon emission in Pakistan’s economy from the period of 1971 to 2014. They have employed ARDL model to investigate the “cointegration effect” and causality association between the study variables. The authors’ study resulted in a long-run association between human capital and carbon emission. Furthermore, the improvement in the value of human capital will decrease the co2 Pakistan economy. The study also helped in policy formulation for dealing with environmental sustainability through human capital investment. Researches done by Sarkodie et al. (Citation2020) and Sharif et al. (Citation2019) considered the China economy for mitigating environmental degradation through human capital and renewable energy. It was stated that the complexity of the human capital in dealing with carbon emission and fossil fuel utilisation is underscored in China. For testing the correlation among chosen established constructs, dynamic ARDL simulation with support of some robust standard errors from 1961 to 2016 was performed. It was observed that EC, along with human capital, are playing their role as conducive catalysts for climate change. Furthermore, their study has also proved the presence of EKC.
Gu and Zhou (Citation2020) and Wu et al. (Citation2021) have stated that investment in such infrastructure where there is more achievement in the form of sustainable development are needed. In this regard, China has made a significant amount of investment in terms of Belt and Road Initiative and the development of renewable energy projects. Their study collected the data for renewable energy projects and examined the carbon emission generated through different sources. Based on the solar energy projects, it is believed that there is a reasonable decline in the value of carbon emission. K. H. Nguyen and Kakinaka (Citation2019) have tested the role of renewable EC, carbon emission along with the development stages by using panel cointegration analysis. They have collected data from 107 countries from the time period of 1990 to 2013. The study results validated the clear difference among low and high-income countries based on the EC and carbon emission. More specifically, mixed results have been found when we talk about EC and carbon emission. However, under high-income economies the said relation shows the negative impact. Shuai et al. (Citation2019) and Huang, Sadiq, and Chien et al. (Citation2021) have tested for EG decoupling from the carbon emission for 133 countries. To check for the carbon emission in targeted economies, they have considered carbon emission per capita, total carbon emission, and carbon intensity. Furthermore, their study has tested the global decoupling of economic growth, where the study findings confirmed that EG is decoupled from carbon intensity, carbon per capita, and total carbon.
A class of literature also indicated that human capital is an effective tool to cut down CO2 via increased efficiency of production process (Hsu et al., Citation2021; Huang et al., Citation2020; Sharif et al., Citation2017). Therefore, human resources are recommended to be employed so that new pollution free methods and technologies could be adopted in specific sector. Scholars argued that organisations that possess huge chunk of human capital, may have the tendency to decrease environmental costs in significant manner (Sharif et al., Citation2019).
Although there is a vast body of literature covering the title of carbon emission and its determinants like clean and renewable energy and EG (Chien et al., Citation2021; Nundkumar & Subban, Citation2020), however, one of the key gaps as observed in the present literature is in exploring the effect of the human capital index and related investment in combating environmental pollutions. Furthermore, studies examining the role of green energy in determining carbon emission are also scant. Finally, the literature work specifically from the context of E7 economies is also inadequate. Very few studies have been found covering the theoretical as well as empirical role of clean energy, economic growth, and human capital index in E7 countries.
3. Research methods
The starting method implemented under the present study dealt with the CSD, which is quite important while checking for the cointegration association between the variables of interest. Various authors have provided the significance for studying the CSD because of the significance of long and short run estimations. This would justify the argument that different factors which are normally macro have their direct and indirect role in determining the CSD between the constructs under observation. Meanwhile, if the issue of CSD is ignored, the findings through ARDL would no longer be acceptable due to biasness in the study coefficients. Additionally, spurious results may be experienced if the issue of CSD is ignored. Therefore, our study has applied the Chudik and Pesaran (Citation2015) test for checking the CSD under the first step of the analysis. Secondly, we have examined stationarity for the panel data which was collected for the E7 economies. Various researchers successfully managed to deal non-stationarity issues in the panel data (Garín-Mun, Citation2006; Kennedy et al., Citation2020; Pothen & Welsch, Citation2019). More specifically, the issue of non-stationarity in the panel is divided into three broad categories: 1st generation panel unit root, 2nd generation panel unit root, and finally 3rd generation panel unit root tests. This study followed Bai & Carrion-I-Silvestre (Citation2009) and Pesaran (Citation2007) methodologies in order to get rid of non-stationarity issues. After checking for the unit root, we applied the Swamy’s test, which will help us to check for the heterogeneity in the slope coefficients. This would justify that slope coefficients are not homogenous, which is assumed as null hypothesis under the present study. Due to non-stationarity and heterogeneity issues among study constructs, the study applied Westerlund and Edgerton (Citation2008) and Banerjee and Carrion‐i‐Silvestre (Citation2017) methodologies, which help to tackle the issue of structural breaks and existence of cointegration.
Chudik and Pesaran (Citation2015) argued that the ARDL model is one of the most “heterogeneous panel data estimators.” Still, the model typically did not find success to address CSD errors. In addition, various factors play a notable role in ascertaining the CSD, which may lead to some misleading output. In this regard, the methods entitled as CS-ARDL are feasible to apply whenever there is a presence of heterogeneity and the CSD among the variables of interest. Chudik and Pesaran (Citation2015) argued that CS-ARDL model basically grounds the ARDL model with the element of linear combination of average CSD. The reason is to capture CSD in error term. One of the key benefits in applying the CS-ARDL approach is that it deals with dynamic correlated effect estimator for various issues as expressed by Topcu and Çoban (Citation2017). The traditional approach to define CS-ARDL can be presented with the help of Equation 1 below.
Equation 1
Equation 1
The above Equation 1 can be modified in the following ways, as shown under Equation 2 of the study
Equation 2
Equation 2
The above Equation 2 is expressed as the ARDL model. To add more value to this model, we have taken into account Equation 3, an extended form of Equation 2 which considers the CS averages for each of the study explanatory variables.
Equation 3
Equation 3
Where, in the above Equation, different notations have been used. For example, indicates the average scores for both study dependent and independent variables, which are under consideration, whereas Pw, Pz, and Px are showing the lagged score for each of the study variables. Meanwhile, Wi,t shows the main dependent variable, which is entitled as carbon emission or CarEM, measured in terms of per capita during the study period. Additionally,
indicates the cross-sectional averages to overcome the spill-over effect. Meanwhile, Equation 3 can be expressed with the help of Equation 4 through CS-ARDL to monitor for the long-run estimation between the variables of interest.
Equation 4
Equation 4
The mean group is described with the help of following Equation 5
Equation 5
Equation 5
For the estimation of short-run coefficients, the following equation 5 is under observation
Equation 6
Equation 6
Where, in the above Equation the titles are
Equation 7
Equation 7
Equation 8
Equation 8
Equation 9
Equation 9
Furthermore, for CS-ARDL model, the title like ECM(-1) shows the “adjustment speed” taken up by any economy to hunt the equilibrium level. Finally, our study has also conducted a robustness analysis with slope heterogeneity and CSD presence. However, with CSD presence, the study has adopted AGM Eberhardt and Teal (Citation2010) and CCEMG Pesaran and Yamagata (Citation2008). However, AMG and CCEMG, both were predicted as a better estimator with the non-stationarity common factors and unobserved mean group analysis. Nevertheless, CCEMG is an alternative tool for the analysis compared to AMG, which caters heterogeneity issue, CSD, and structural breaks along with the dummies in the data set because it checks for unobserved factors. Besides, the literature justification for using AMG and CCEMG can be examined from the research contribution as provided by Paramati and Roca (Citation2019), Xia et al. (Citation2021), Nketia et al. (Citation2018), Atasoy (Citation2017), Gu and Zhou (Citation2020), and Sarkodie et al. (Citation2020).
4. Results and discussion
Under panel data setting, the titles like CSD and slope heterogeneity were the main problems that need to be addressed. For this purpose, reports the outcomes for the CSD for the study variables entitled (carbon emission, human capital index, green energy, GDP, and square of GDP (GDP2)). The null hypothesis under assumes “no CSD” among the study variables, whereas the alternative claims it is not. The findings validated CSD presence among the study variables as all the t-stats were significant at 1%.
Table 1. CSD analysis.
provides the outcome for the unit root test with and without structural break consideration (Pesaran, Citation2007). For a better understanding, Carrion‐i‐Silvestre et al. (2005) study finding helped in explaining the null and alternative hypotheses: supporting the non-stationarity and non-stationarity issue respectively. The findings under for CIPS and M-CIPS were found to be statistically significant at either 1% or 5% significance level. In addition, the empirical outcomes from the studies of Bai & Carrion-I-Silvestre (Citation2009) and also observed the problem of structural breaks and the CSD in the study data. The study’s findings of Bai & Carrion-I-Silvestre (Citation2009) and Chien et al. (Citation2021) have provided significant results for Z, Pm, and P as shown in . Our study findings have rejected the null and accepted the alternative hypothesis of the unit root or stationarity for all the variables when CSD, structural breaks, and heterogeneity. This findings would justify the argument that all the variables under the present study such as carbon emission, human capital index, green energy, GDP, and GDP2 are stationarity at first difference or integrated at I(1).
Table 2. URT Pesaran (Citation2007).
The findings under has provided the outcomes as generated through a modified version of Swamy (Citation1970) slope homogeneity test as suggested by Pesaran and Yamagata (Citation2008). One of the key benefits of applying this test is that it checks whether heterogenous or homogenous slope coefficients are present. This is because homogenous slope coefficients in the study coefficients may lead to some misleading results for which the inference will not be justified. For this purpose, both hypotheses (null and alternative) were tested where the null shows the presence of homogenous slope coefficients, and the alternative specifies heterogenous slope coefficients. The findings under suggest that both Delta tilde and Delta tilde adjusted were significant 1%. Therefore, the alternative hypothesis is justified under .
Table 3. SH Analysis.
presents the findings for Westerlund and Edgerton (Citation2008) panel cointegration. The null hypothesis stipulates no integration among the study variables when CSD, structural breaks, and slope heterogeneity are present, whereas the alternative specifies that cointegration existence among variables. The findings under confirmed the presence of cointegration between the study variables at no break, mean shift, and regime shift. This confirmed the cointegration association between carbon emission, human capital index, green energy, GDP, and GDP2.
Table 4. PC analysis.
presents the cointegration analysis for Banerjee and Carrion‐i‐Silvestre (2017), where the main dependent variable is carbon emission. The study findings have confirmed the existence of cointegration association between clean energy, human capital index, GDP, and GDP2. Furthermore, the findings for the selected economies like Brazil, China, India, Indonesia, Mexico, Russia, and Turkey also observe a cointegration association between the study variables. More specifically, the cointegration linkage by Westerlund and Edgerton (Citation2008) and Banerjee and Carrion‐i‐Silvestre (2017) have provided enough evidence for moving towards the long-run estimation while taking into account the study variables.
Table 5. Cointegration analysis.
Finally, the findings under provide evidence for the cross-sectional autoregressive distributed lags models for the long-run estimation. The empirical findings assured the claim with evidence that higher level of human capital index is considered to be crucial in reducing co2 emission. More specifically, for every single unit increase in HCI value, there is a downward shift of −0.310 in the value of carbon emission. Study done by Hao et al. (Citation2021) have also provided similar proposition, hence giving more authentication. This would justify the argument that improving the quality of human capital with the help of education and similar other investment may increase community awareness towards the usage of those technologies and energy sources that have lower environmental impact. Consequently, these technologies can help in reducing the CO2 emission. Various other studies like Bano et al. (Citation2018), Saleem & Shujah-Ur-Rahman (Citation2019), and Chien et al. (Citation2021) have also justified the above-stated impact of human capital index specifically from the context of Pakistan and for the BRICS economies.
Table 6. CS-Autoregressive distributed lag analysis for long-run.
In addition, the impact of GEN on CO2 emissions was negatively significant, which justified that the higher usage of green energy like those from renewable sources are very useful in combating environmental degradation. This relationship was confirmed as the beta coefficient is −0.679 and a t-value is −5.451. A bulk of empirical contribution is available to explain the constructive impact of green energy on co2. For example, F. Liu et al. (Citation2020), have supported the argument that higher usage of clean energy or environmentally friendly energy may provide fruitful results. This fact is also supported by Yi (Citation2015), who have confirmed that clean energy policies from the US government impact carbon emissions negatively. Moreover, depicts the long-run association between the GDP and carbon emission. The findings of CS-ARDL analysis confirmed that more economic growth in the targeted economies leads to higher carbon emissions. More specifically, every single unit increase in GDP value caused a shift of 0.214% in carbon emissions value for the selected economies. This relationship is widely supported in the recent and past literature by G. Li and Wei (Citation2021), Wang and Zhang (Citation2021), Yang et al. (Citation2021), and Apeaning (Citation2021). This is because for running any economy, the production of goods and services is an ongoing activity that depends significantly upon the energy sources, specifically from the non-renewable ones. In this regard, higher EC creates more environmental threats in the form of carbon emission and environmental degradation.
Besides, the findings for the long-run CS-ARDL also depict GDP2 influences carbon emission for the selected economies. The study finding confirms that GDP2 is significantly and negatively associated with CO2 emission in the selected economies. More specifically, a decline of −0.079 CO2 value was observed due to unit change in the GDP2 value. In the present literature, the association between GDP2 and carbon emission was also empirically tested. For example, Anser et al. (Citation2020) have tested the impact of GDP2 on carbon emission and found it negatively significant. Similarly, the research contribution as provided by Hao et al. (Citation2021) has also provided evidence that GDP2 is one of the crucial factors when it comes to determine carbon emission for G7 economies. Besides, some other studies had similar outputs, like Rabbi et al. (Citation2015), Dogan and Turkekul (Citation2016), and Nosheen et al. (Citation2021).
Finally, the findings in cover the short-run estimation through the CS-ARDL approach. It was observed that the human capital index is negatively and significantly impacting the carbon emission, hence justifying that more investment on the community members for better knowledge and education will increase awareness regarding carbon emissions. Under the long-run estimation, the impact of HCI on carbon emission is accepted towards reducing environmental degradation and dealing with sustainability issues. In this regard, research work conducted by Khan et al. (Citation2021) confirmed that there is a one-way effect of human capital on carbon emission. Sarkodie et al. (Citation2020) have also claimed that the increase in human capital value is conducive in dealing with environmental degradation and escalation of the emissions specifically from the context of China. Besides, some other studies like Bano et al. (Citation2018), Haini (Citation2021), Chien et al. (Citation2021) and Sheraz et al. (Citation2021) also probed the relation of human capital with carbon emission.
Table 7. CS-autoregressive distributed lag analysis for long-run.
In addition, it can be seen that green energy impacts carbon emission negatively under the short-run estimation. More specifically, for every single unit increase in renewable or green energy sources, there was a decline of 0.079% in CO2 emission. This relationship has been widely justified in the existing literature. In contrast, Nawaz et al. (Citation2021) have provided evidence that there is a huge impact of renewable energy production on environmental health. Research contributions by Dogan and Seker (Citation2016), Long et al. (Citation2015), Adams and Acheampong (Citation2019), and Khan et al. (Citation2020) have also justified the significant impact of renewable energy sources in dealing with environmental degradation. Furthermore, under short-run estimation, the GDP has shown significant and positive impact while GDP2 played a constructive role in reducing the carbon emission for the selected economies. This means that higher economic growth in the targeted economies is leading towards higher environmental degradation like carbon emission but not GDP2. In this regard, the nexus between GDP-carbon emission has been justified by earlier studies (Dong et al., Citation2020; Nathaniel et al., Citation2021; T. T. Nguyen et al., Citation2020; Saidi & Omri, Citation2020; Wang & Zhang, Citation2021).
Finally, the study findings in cover the robustness check through AMG and CCEMG while considering carbon emissions as the main dependent variable and the human capital index, green energy, GDP, and GDP2square as key explanatory variables. The study findings through AMG have confirmed that HCI, GEN, and GDP2 were significantly playing their role in reducing the carbon emission. In contrast, economic growth in terms of GDP was causing more emissions in selected countries. Similar output was observed under the common correlated effect mean group (CCEMC) where HCI, GEN, and GDP2 were reducing the environmental degradation except GDP.
Table 8. AMG and CCEMG for Robustness Check.
5. Conclusion and policy implications
The present study analyzed the impact of human capital index, green energy, economic growth through GDP, and GDP2 on the carbon emission in seven emerging countries. The study applied the Chudik and Pesaran (Citation2015) test to examine the CSD along with the cointegration analyses as suggested by Westerlund and Edgerton (Citation2008) and Banerjee and Carrion‐i‐Silvestre (2017). Furthermore, this study has focussed on checking for the slope heterogeneity analysis as suggested by Swamy (Citation1970) and Pesaran and Yamagata (Citation2008). Finally, the long-run and short-run cointegration relationship between the study variables were tested through the CS-ARDL approach. The study results confirmed that factors human capital index, green and clean energy, and square of economic growth are playing a significant role in combating environmental pollution like CO2 emission under long-run estimation. Additionally, the impact from GDP was found to be a core factor for increasing the carbon emissions under the long-run findings. For the short-run estimation, the study outcomes confirmed the findings, similar to the long-run estimation. Finally, the robust outcome under the present study has also justified the direct effect of economic growth for promoting natural degradation and constructive role of the human capital index and green energy along with the GDP2 in reducing environmental pollution (e.g., carbon emissions).
Based on the study findings, it is recommended that to reduce the impact of EG towards increasing carbon emission, domestic consumption level in all the targeted economies should be taken into consideration, specifically for those where there is a higher level of carbon emission because of traditional energy consumption and its usage for the production of goods and services. In this regard, emission-oriented consumption should be controlled through some immediate environmental reforms like carbon taxes and more eco-friendly innovations. Besides, the economic activities and transportation of goods in these economies are mainly depending upon consumption-based carbon emissions which needs to be replaced through hybrid technologies. Furthermore, more investment in the form of human capital for promoting awareness among general public can also generate some meaningful results as negative impact from HCI on carbon emission was found. This negative impact would justify the argument that community awareness is a key factor in reducing environmental pollutions among selected countries. Similar is suggested for the usage of green energy like those from renewable sources. This way, policies related to carbon emission and their nexus with governmental strategies also need a strong alignment as macro-level tactical planning can generate some fertile outcomes. Moreover, this study is also insightful for policy makers and environmentalists. After all, the revelation of study indicates that the green growth in energy and eco-innovation might shift the paradigm of industries from non-renewable to renewable resources, which further would be helpful to reduce CO2 emissions.
Disclosure statement
We declare that there is no competing interest.
Additional information
Funding
References
- Adams, S., & Acheampong, A. O. (2019). Reducing carbon emissions: the role of renewable energy and democracy. Journal of Cleaner Production, 240, 118245. https://doi.org/10.1016/j.jclepro.2019.118245
- Anser, M. K., Hanif, I., Alharthi, M., & Chaudhry, I. S. (2020). Impact of fossil fuels, renewable energy consumption and industrial growth on carbon emissions in Latin American and Caribbean economies. Atmósfera, 33(3), 201–213.
- Apeaning, R. W. (2021). Technological constraints to energy-related carbon emissions and economic growth decoupling: A retrospective and prospective analysis. Journal of Cleaner Production, 291, 125706. https://doi.org/10.1016/j.jclepro.2020.125706
- Atasoy, B. S. (2017). Testing the environmental Kuznets curve hypothesis across the US: Evidence from panel mean group estimators. Renewable Sustainable Energy Reviews, 77, 731–747.
- Bai, J., & Carrion-I-Silvestre, J. L. (2009). Structural changes, common stochastic trends, and unit roots in panel data. The Review of Economic Studies, 76(2), 471–501.
- Banerjee, A., & Carrion-i-Silvestre, J. L. (2017). Testing for panel cointegration using common correlated effects estimators. Journal of Time Series Analysis, 38(4), 610–636. https://doi.org/10.1111/jtsa.12234
- Bano, S., Zhao, Y., Ahmad, A., Wang, S., & Liu, Y. (2018). Identifying the impacts of human capital on carbon emissions in Pakistan. Journal of Cleaner Production, 183, 1082–1092. https://doi.org/10.1016/j.jclepro.2018.02.008
- Bhattacharya, M., Paramati, S. R., Ozturk, I., & Bhattacharya, S. (2016). The effect of renewable energy consumption on economic growth: Evidence from top 38 countries. Applied Energy, 162, 733–741. https://doi.org/10.1016/j.apenergy.2015.10.104
- Bruneckiene, J., Mikalonis, Š., & Rapsikevičius, J. (2020). The interaction between ownership concentration and market risk in European, American and Chinese markets: the effects of BREXIT. Engineering Economics, 31(5), 502–512. https://doi.org/10.5755/j01.ee.31.5.25997
- Castillo-Vergara, M., Quispe-Fuentes, I., & Poblete, J. (2021). Technological Innovation in the Food Industry: A Bibliometric Analysis. Engineering Economics, 32(3), 197–209. https://doi.org/10.5755/j01.ee.32.3.26000
- Chien, F., Ananzeh, M., Mirza, F., Bakar, A., Vu, H. M., & Ngo, T. Q. (2021). The effects of green growth, environmental-related tax, and eco-innovation towards carbon neutrality target in the US economy. Journal of Environmental Management, 299, 113633.
- Chien, F., Kamran, H. W., Albashar, G., & Iqbal, W. (2021). Dynamic planning, conversion, and management strategy of different renewable energy sources: a sustainable solution for severe energy crises in emerging economies. International Journal of Hydrogen Energy, 46(11), 7745–7758. https://doi.org/10.1016/j.ijhydene.2020.12.004
- Chien, F., Pantamee, A. A., Hussain, M. S., Chupradit, S., Nawaz, M. A., & Mohsin, M. (2021). Nexus between financial innovation and bankruptcy: Evidence from information, communication and technology (ICT) sector. The Singapore Economic Review, 1–22. https://doi.org/10.1142/S0217590821500181
- Chien, F., Sadiq, M., Kamran, H. W., Nawaz, M. A., Hussain, M. S., & Raza, M. (2021). Co-movement of energy prices and stock market return: environmental wavelet nexus of COVID-19 pandemic from the USA, Europe, and China. Environmental Science and Pollution Research. https://doi.org/10.1007/s11356-021-12938-2.
- Chien, F., Sadiq, M., Nawaz, M. A., Hussain, M. S., Tran, T. D., & Le Thanh, T. (2021). A step toward reducing air pollution in top Asian economies: The role of green energy, eco-innovation, and environmental taxes. Journal of Environmental Management, 297, 113420. https://doi.org/10.1016/j.jenvman.2021.113420
- Chien, F., Zhang, Y., Sadiq, M., & Hsu, C.-C. (2021). Financing for energy efficiency solutions to mitigate opportunity cost of coal consumption: an empirical analysis of Chinese industries. Environmental Science and Pollution Research. https://doi.org/10.1007/s11356-021-15701-9
- Chudik, A., & Pesaran, M. H. (2015). Common correlated effects estimation of heterogeneous dynamic panel data models with weakly exogenous regressors. Journal of Econometrics, 188(2), 393–420. https://doi.org/10.1016/j.jeconom.2015.03.007
- Corrás-Arias, M. Á. (2020). Determinantes de la banca extranjera en España. Un análisis por país de origen. Cuadernos de Economía, 43(121), 48–62.
- Dadelo, S. (2020). The analysis of sports and their communication in the context of creative industries. Creativity Studies, 13(2), 246–256. https://doi.org/10.3846/cs.2020.12206
- Daniel, R. (2021). Exploring creativity through artists’ reflections. Creativity Studies, 14(1), 1–17. https://doi.org/10.3846/cs.2021.11207
- Danielle, N. (2020). Allocation Of Risk In Public Private Partnerships In Information And Communications Technology. International Journal of Ebusiness and Egovernment Studies, 12(1), 17–32.
- Dogan, E., & Seker, F. (2016). The influence of real output, renewable and non-renewable energy, trade and financial development on carbon emissions in the top renewable energy countries. Renewable and Sustainable Energy Reviews, 60, 1074–1085. https://doi.org/10.1016/j.rser.2016.02.006
- Dogan, E., & Turkekul, B. (2016). CO 2 emissions, real output, energy consumption, trade, urbanization and financial development: testing the EKC hypothesis for the USA. Environmental Science and Pollution Research, 23(2), 1203–1213. https://doi.org/10.1007/s11356-015-5323-8
- Dong, B., Ma, X., Zhang, Z., Zhang, H., Chen, R., Song, Y., Shen, M., & Xiang, R. (2020). Carbon emissions, the industrial structure and economic growth: Evidence from heterogeneous industries in China. Environmental Pollution (Barking, Essex: 1987), 262, 114322. https://doi.org/10.1016/j.envpol.2020.114322
- Eberhardt, M., & Teal, F. (2010). Aggregation versus Heterogeneity in Cross-Country Growth Empirics.
- Garín-Mun, T. (2006). Inbound international tourism to Canary Islands: a dynamic panel data model. Tourism Management, 27(2), 281–291.
- Gu, A., & Zhou, X. (2020). Emission reduction effects of the green energy investment projects of China in belt and road initiative countries. Ecosystem Health and Sustainability, 6(1), 1747947. https://doi.org/10.1080/20964129.2020.1747947
- Haini, H. (2021). Examining the impact of ICT, human capital and carbon emissions: Evidence from the ASEAN economies. International Economics, 166, 116–125. https://doi.org/10.1016/j.inteco.2021.03.003
- Hao, L.-N., Umar, M., Khan, Z., & Ali, W. (2021). Green growth and low carbon emission in G7 countries: how critical the network of environmental taxes, renewable energy and human capital is? Science of the Total Environment, 752, 141853. https://doi.org/10.1016/j.scitotenv.2020.141853
- Hsu, C.-C., Quang-Thanh, N., Chien, F., Li, L., & Mohsin, M. (2021). Evaluating green innovation and performance of financial development: mediating concerns of environmental regulation. Environmental Science and Pollution Research. https://doi.org/10.1007/s11356-021-14499-w
- Huang, S.-Z., Chau, K. Y., Chien, F., & Shen, H. (2020). The Impact of Startups’ Dual Learning on Their Green Innovation Capability: The Effects of Business Executives’ Environmental Awareness and Environmental Regulations. Sustainability, 12(16), 6526. https://doi.org/10.3390/su12166526
- Huang, S.-Z., Sadiq, M., & Chien, F. (2021). The impact of natural resource rent, financial development, and urbanization on carbon emission. Environmental Science and Pollution Research. https://doi.org/10.1007/s11356-021-16818-7
- Kennedy, P. L., Schmitz, A., & DeLong, K. L. (2020). Biotechnology and demand concerns: the case of genetically modified US sugar beets.
- Khan, Z., Ali, S., Dong, K., & Li, R. Y. M. (2021). How does fiscal decentralization affect CO2 emissions? The roles of institutions and human capital. Energy Economics, 94, 105060. https://doi.org/10.1016/j.eneco.2020.105060
- Khan, Z., Ali, S., Umar, M., Kirikkaleli, D., & Jiao, Z. (2020). Consumption-based carbon emissions and international trade in G7 countries: the role of environmental innovation and renewable energy. Science of the Total Environment, 730, 138945. https://doi.org/10.1016/j.scitotenv.2020.138945
- Kirikkaleli, D., & Sowah, J. K. (2020). A wavelet coherence analysis: nexus between urbanization and environmental sustainability. Environmental Science and Pollution Research International, 27(24), 30295–30305.
- Ključnikov, A., Civelek, M., Polách, J., Mikoláš, Z., & Banot, M. (2020). How do security and benefits instill trustworthiness of a digital local currency? Oeconomia Copernicana, 11(3), 433–465. https://doi.org/10.24136/oc.2020.018
- Li, G., & Wei, W. (2021). Financial development, openness, innovation, carbon emissions, and economic growth in China. Energy Economics, 97, 105194. https://doi.org/10.1016/j.eneco.2021.105194
- Li, W., Chien, F., Kamran, H. W., Aldeehani, T. M., Sadiq, M., Nguyen, V. C., & Taghizadeh-Hesary, F. (2021). The nexus between COVID-19 fear and stock market volatility. Economic Research-Ekonomska Istraživanja. https://doi.org/10.1080/1331677X.2021.1914125
- Liu, F., Tait, S., Schellart, A., Mayfield, M., & Boxall, J. (2020). Reducing carbon emissions by integrating urban water systems and renewable energy sources at a community scale. Renewable and Sustainable Energy Reviews, 123, 109767. https://doi.org/10.1016/j.rser.2020.109767
- Liu, Z., Tang, Y. M., Chau, K. Y., Chien, F., Iqbal, W., & Sadiq, M. (2021). Incorporating strategic petroleum reserve and welfare losses: a way forward for the policy development of crude oil resources in South Asia. Resources Policy, 74, 102309. https://doi.org/10.1016/j.resourpol.2021.102309
- Carrion-i-Silvestre, J. L., Del Barrio-Castro, T. and López-Bazo, E. (2005). Breaking the panels: an application to the GDP per capita. The Econometrics Journal, 8(2), 159–175.
- Long, X., Naminse, E. Y., Du, J., & Zhuang, J. (2015). Nonrenewable energy, renewable energy, carbon dioxide emissions and economic growth in China from 1952 to 2012. Renewable and Sustainable Energy Reviews, 52, 680–688. https://doi.org/10.1016/j.rser.2015.07.176
- Nathaniel, S., Barua, S., Hussain, H., & Adeleye, N. (2021). The determinants and interrelationship of carbon emissions and economic growth in African economies: fresh insights from static and dynamic models. Journal of Public Affairs, 21(1), e2141. https://doi.org/10.1002/pa.2141
- Nawaz, M. A., Hussain, M. S., Kamran, H. W., Ehsanullah, S., Maheen, R., & Shair, F. (2021). Trilemma association of energy consumption, carbon emission, and economic growth of BRICS and OECD regions: quantile regression estimation. Environmental Science and Pollution Research International, 28(13), 16014–16028.
- Nguyen, K. H., & Kakinaka, M. (2019). Renewable energy consumption, carbon emissions, and development stages: Some evidence from panel cointegration analysis. Renewable Energy., 132, 1049–1057. https://doi.org/10.1016/j.renene.2018.08.069
- Nguyen, T. T., Pham, T. A. T., & Tram, H. T. X. (2020). Role of information and communication technologies and innovation in driving carbon emissions and economic growth in selected G-20 countries. Journal of Environmental Management, 261, 110162.
- Nketia, E. B., Kong, Y., & Korankye, B. (2018). Institutional Quality and Economic Growth Correlation in Africa; An Augmented Mean Group and Common Correlated Effects Mean Group Approach.
- Nosheen, F., Kouser, R., Anjum, S., & Akhter, M. J. (2021). Control of Carbon Emissions by Promoting Economic Growth and Renewable Energy in Newly Emerging Economic Block. Review of Education, Administration & LAW, 4(2), 409–420. https://doi.org/10.47067/real.v4i2.153
- Nundkumar, A., & Subban, M. (2020). Risk Management: A Strategic Approach To Enhance Tvet College Management. International Journal of Business & Management Studies, 12(2)
- Özer, M., Kamenković, S., & Grubišić, Z. (2020). Frequency domain causality analysis of intra-and inter-regional return and volatility spillovers of South-East European (SEE) stock markets. Economic Research-Ekonomska Istraživanja, 33(1), 1–25. https://doi.org/10.1080/1331677X.2019.1699138
- Paramati, S. R., & Roca, E. (2019). Does tourism drive house prices in the OECD economies? Evidence from augmented mean group estimator. Tourism Management, 74, 392–395. https://doi.org/10.1016/j.tourman.2019.04.023
- Pesaran, M. H. (2007). A simple panel unit root test in the presence of cross‐section dependence. Journal of Applied Econometrics, 22(2), 265–312. https://doi.org/10.1002/jae.951
- Pesaran, M. H., & Yamagata, T. (2008). Testing slope homogeneity in large panels. Journal of Econometrics, 142(1), 50–93. https://doi.org/10.1016/j.jeconom.2007.05.010
- Pothen, F., & Welsch, H. (2019). Economic development and material use. Evidence from international panel data. World Development, 115, 107–119. https://doi.org/10.1016/j.worlddev.2018.06.008
- Rabbi, F., Akbar, D., & Kabir, S. Z. (2015). Environment Kuznets curve for carbon emissions: A cointegration analysis for Bangladesh. International Journal of Energy Economics Policy, 5(1), 45.
- Sadiq, M., Hsu, C.-C., Zhang, Y., & Chien, F. (2021). COVID-19 fear and volatility index movements: empirical insights from ASEAN stock markets. Environmental Science and Pollution Research. https://doi.org/10.1007/s11356-021-15064-1
- Sadiq, M., Nonthapot, S., Mohamad, S., Chee Keong, O., Ehsanullah, S., & Iqbal, N. (2021). Does green finance matter for sustainable entrepreneurship and environmental corporate social responsibility during COVID-19? China Finance Review International, ahead-of-print(ahead-of-print) https://doi.org/10.1108/CFRI-02-2021-0038
- Saidi, K., & Omri, A. (2020). The impact of renewable energy on carbon emissions and economic growth in 15 major renewable energy-consuming countries. Environmental Research, 186, 109567. https://doi.org/10.1016/j.envres.2020.109567
- Saleem, N., & Shujah-Ur-Rahman, J. (2019). The impact of human capital and biocapacity on environment: environmental quality measure through ecological footprint and greenhouse gases. Pollut Eff Cont, 7, 237.
- Sarkodie, S. A., Adams, S., Owusu, P. A., Leirvik, T., & Ozturk, I. (2020). Mitigating degradation and emissions in China: the role of environmental sustainability, human capital and renewable energy. Science of the Total Environment, 719, 137530. https://doi.org/10.1016/j.scitotenv.2020.137530
- Shair, F., Shaorong, S., Kamran, H. W., Hussain, M. S., Nawaz, M. A., & Nguyen, V. C. (2021). Assessing the efficiency and total factor productivity growth of the banking industry: do environmental concerns matters? Environmental Science and Pollution Research International, 28(16), 20822–20838.
- Sharif, A., Afshan, S., Chrea, S., Amel, A., & Khan, S. A. R. (2020). The role of tourism, transportation and globalization in testing environmental Kuznets curve in Malaysia: new insights from quantile ARDL approach. Environmental Science and Pollution Research International, 27(20), 25494–25509.
- Sharif, A., Afshan, S., & Nisha, N. (2017). Impact of tourism on CO2 emission: evidence from Pakistan. Asia Pacific Journal of Tourism Research, 22(4), 408–421. https://doi.org/10.1080/10941665.2016.1273960
- Sharif, A., Afshan, S., & Qureshi, M. A. (2019). Idolization and ramification between globalization and ecological footprints: evidence from quantile-on-quantile approach. Environmental Science and Pollution Research International, 26(11), 11191–11211.
- Sharif, A., Bhattacharya, M., Afshan, S., & Shahbaz, M. (2021). Disaggregated renewable energy sources in mitigating CO2 emissions: new evidence from the USA using quantile regressions. Environmental Science and Pollution Research. https://doi.org/10.1007/s11356-021-13829-2
- Sharif, A., Mishra, S., Sinha, A., Jiao, Z., Shahbaz, M., & Afshan, S. (2020). The renewable energy consumption-environmental degradation nexus in Top-10 polluted countries: Fresh insights from quantile-on-quantile regression approach. Renewable Energy., 150, 670–690. https://doi.org/10.1016/j.renene.2019.12.149
- Sheraz, M., Deyi, X., Ahmed, J., Ullah, S., & Ullah, A. (2021). Moderating the effect of globalization on financial development, energy consumption, human capital, and carbon emissions: evidence from G20 countries. Environmental Science Pollution Research. https://doi.org/10.1007/s11356-021-13116-0
- Shuai, C., Chen, X., Wu, Y., Zhang, Y., & Tan, Y. (2019). A three-step strategy for decoupling economic growth from carbon emission: empirical evidences from 133 countries. Science of the Total Environment, 646, 524–543. https://doi.org/10.1016/j.scitotenv.2018.07.045
- Sohag, K., Kalugina, O., & Samargandi, N. (2019). Re-visiting environmental Kuznets curve: role of scale, composite, and technology factors in OECD countries. Environmental Science and Pollution Research International, 26(27), 27726–27737.
- Suki, N. M., Suki, N. M., Sharif, A., Afshan, S., & Jermsittiparsert, K. (2022). The role of technology innovation and renewable energy in reducing environmental degradation in Malaysia: A step towards sustainable environment. Renewable Energy., 182, 245–253. https://doi.org/10.1016/j.renene.2021.10.007
- Swamy, P. A. (1970). Efficient inference in a random coefficient regression model. Econometrica: Journal of the Econometric Society, 38(2), 311–323. https://doi.org/10.2307/1913012
- Tong, T., Ortiz, J., Xu, C., & Li, F. (2020). Economic growth, energy consumption, and carbon dioxide emissions in the E7 countries: a bootstrap ARDL bound test. Energy, Sustainability and Society, 10(1), 1–17. https://doi.org/10.1186/s13705-020-00253-6
- Topcu, M., & Çoban, S. (2017). Financial development and firm growth in Turkish manufacturing industry: evidence from heterogeneous panel based non-causality test. Economic Research-Ekonomska Istraživanja, 30(1), 1758–1769. https://doi.org/10.1080/1331677X.2017.1383179
- Tsunga, K., Moores-Pitt, P., & Mccullough, K. (2020). A Non-Linear Analysis Of South African Exports And Selected Macroeconomic Variables. International Journal of Economics and Finance, 12(2). https://doi.org/10.34109/ijefs.202012212
- Wang, Q., & Zhang, F. (2021). The effects of trade openness on decoupling carbon emissions from economic growth - Evidence from 182 countries. Journal of Cleaner Production, 279, 123838. https://doi.org/10.1016/j.jclepro.2020.123838
- Westerlund, J., & Edgerton, D. L. (2008). A simple test for cointegration in dependent panels with structural breaks. Oxford Bulletin of Economics and Statistics, 70(5), 665–704. https://doi.org/10.1111/j.1468-0084.2008.00513.x
- Wu, X., Sadiq, M., Chien, F., Ngo, Q.-T., & Nguyen, A.-T. (2021). Testing role of green financing on climate change mitigation: evidences from G7 and E7 countries. Environmental Science and Pollution Research. https://doi.org/10.1007/s11356-021-15023-w
- Xia, W., Doğan, B., Shahzad, U., Adedoyin, F. F., Popoola, A., & Bashir, M. A. (2021). An empirical investigation of tourism-led growth hypothesis in the european countries: evidence from augmented mean group estimator. Portuguese Economic Journal. https://doi.org/10.1007/s10258-021-00193-9
- Xiang, H., Ch, P., Nawaz, M. A., Chupradit, S., Fatima, A., & Sadiq, M. (2021). Integration and economic viability of fueling the future with green hydrogen: An integration of its determinants from renewable economics. International Journal of Hydrogen Energy, 46(77), 38145–38162. https://doi.org/10.1016/j.ijhydene.2021.09.067
- Yang, J., Hao, Y., & Feng, C. (2021). A race between economic growth and carbon emissions: What play important roles towards global low-carbon development? Energy Economics, 100, 105327. https://doi.org/10.1016/j.eneco.2021.105327
- Yi, H. (2015). Clean-energy policies and electricity sector carbon emissions in the U.S. states. Utilities Policy, 34, 19–29. https://doi.org/10.1016/j.jup.2015.04.001