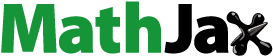
Abstract
First-year attrition and on-time graduation are key challenges for contemporary universities, which determine their efficiency. Based on the benefit of the doubt approach, this study reports the efficiency of the graduation process in 37 Australian public universities. The super-efficiency model extended by restrictions on virtual weights is used. The proposed model considers the attrition rate and the on-time graduation rate separately for domestic and overseas students and other variables, like student-staff ratio, the share of full-time students and the share of online students. Some additional factors are included, such as the university's affiliation with a grouping, the year of the university founding and basic data on the subject mix of universities courses, explaining the rankings created. The analysis indicates that research-oriented universities achieve better results and overseas students perform better than domestic ones. Also, it can be seen that the universities dealing with large-scale online learning are underperformed. The obtained results allow all stakeholders to understand better the efficiency of the graduation process. The main findings are consistent with research published elsewhere.
1. Introduction
Higher education policy has a clear trend towards massification for several decades. There are many reasons for this. The observed increase in the number of students is mainly due to political decisions. The World Declaration on Higher Education for the Twenty-First Century positioned ‘higher education as a fundamental pillar of human rights, democracy, sustainable development and peace' (UNESCO, Citation1998). One of the European Union strategic goals (EU, Citation2010) declares ‘increasing the share of the population aged 30-34 having completed tertiary education to at least 40% in 2020'. Such regulations shape national policies that increase access to higher education. A completely different view on the massification of higher education is presented by Marginson (Citation2016). In his opinion, ‘expansion of higher education is primarily powered not by economic growth but by the ambitions of families to advance or maintain social position'. The increase in the scale of this phenomenon is global. Between 1970 and 2016, the gross enrolment rate increased worldwide from 9.7% to 37.4%; in the Organization for Economic Co-Operation and Development (OECD), it increased from 22.1% to 74.6%; and in the European Union (EU), it increased from 17.3% to 68.0% (World Bank, Citation2018). This trend has many positive implications, such as the provision of high-skilled graduates for the knowledge economy. However, there are also adverse effects, such as first-year attrition and graduation beyond the nominal duration of studies.
Multiple factors can influence the non-completion of studies, such as the wrong choice of programme or study subject or insufficient preparation to meet the requirements of the curriculum. Besides, favourable labour market opportunities can lead to some degree of an early exit from a university education (EACEA, Citation2015; Schnepf, Citation2017; Yue & Fu, Citation2017).
Completion rate is recognised as the primary indicator of academic success, which represents the share of students who started and completed their study programme with a degree at some point in the future (Luca et al., Citation2014; Sneyers & De Witte, Citation2017; Vossensteyn et al., Citation2015). However, on-time graduation is one of the most crucial problems in higher education (Yue & Fu, Citation2017). Luca et al. (Citation2014) claim that in many cases, students extend the duration of their studies because they combine education with a professional job. First-year attrition is another significant indicator used to monitor failure in teaching (Chies et al., Citation2019; Sneyers & De Witte, Citation2017). Barra and Zotti (Citation2016) underline that interrupted careers have become a severe problem in higher education in recent decades. These factors contribute significantly to the inefficiency of higher education systems. For example, in Australian universities, student attrition cost more than $1.4 billion a year (Kirk & & others, Citation2018).
There are two methods to calculate completion rates. The true-cohort method requires data about each student from entry to graduation or dropout. The second method based on cross-sectional data uses the ratio of graduates in a given year and the new entrants to these programs accepted several years earlier. Such a period can be equal to the nominal duration of studies (on-time graduation) or prolonged by one additional year (or two years) (EACEA, Citation2015; OECD, Citation2013; Sneyers & De Witte, Citation2017; Vossensteyn et al., Citation2015). The true-cohort survey was performed in some countries, for instance: in Italy (Chies et al., Citation2019), the USA (Chen et al., Citation2017; Yue & Fu, Citation2017) or Australia (DET, Citation2018).
The Australian Department of Education and Training (DET), based on 6-year completion rates, established a ranking of universities for the cohort that started studies in 2010 and completed them in 2015 (University Rankings, Citation2015). Such research is conducted systematically in Australia. However, it concerns only domestic students. The specificity of the Australian higher education system is that it is focused on the broad provision of educational services to international students. In the sample, overseas students accounted for approximately 25% of all students. Thus, the true-cohort studies conducted so far have omitted a quarter of the students. It is the main gap in the previous research which this article tries to fulfil.
This article aims to propose an alternative to the true-cohort method to analyse the study success of bachelor students using a multidimensional model based on historical cross-sectional data. This model considers first-year attrition, on-time graduation rates, and other factors influencing these two phenomena. Both domestic and overseas students are taken into account independently. The following research questions guide the research: Is it appropriate to independently include domestic and overseas students in the model? Is the use of cross-sectional data free of bias compared to a true-cohort method? Is it possible to find factors that would explain the positions of individual universities in the ranking? Are obtained results comparable to the results of other researches?
This paper analyses and assesses the performance of all 37 Australian public universities in the context of academic success. This research contributes to the literature by addressing the measurement possibilities of university efficiency, taking into account the extent of attrition and graduation after the nominal time of the study. The proposed framework helps all stakeholders of higher education institutions better understand the performance of the graduation process. In the ranking of Australian universities, it is essential to include the share of overseas students, which gives a better picture of the efficiency of the graduation process in individual universities. The multidimensional model uses historical cross-sectional data that takes into account both domestic and overseas students. Using the benefit of the doubt approach (BoD), this paper focuses on the impact of undesirable factors (attrition and failure to graduate in nominal duration) on the efficiency of Australian public universities. Calculations were performed using MaxDEA Ultra software (version 6.18).
The remainder of this paper is organised in the following manner. The literature review is outlined in Section 2. Section 3 explains the data and variables. Section 4 presents some information about the methodology. Empirical results and discussion are presented in Section 5. Section 6 concludes.
2. Literature review
Universities are complex, multiproduct organisations. Their three areas of activity are research, teaching and the so-called third mission. According to Murias et al. (Citation2008), the third mission is broadly defined as fulfilling social needs. These social services usually take the form of knowledge transfer, cultural events or consultancy, and the like. However, it is challenging to identify proper measures for the third mission, and therefore few publications take this into account (Agasisti & Johnes, Citation2015). Furthermore, higher education institutions jointly produce research and teaching in different fields and at different levels, making it difficult to assess the performance of these activities. Often, a university that works well in one dimension may worsen in another (Agasisti & Johnes, Citation2015), as universities often have a clear research focus or specialise in teaching (De Witte & Hudrlikova, Citation2013).
The debate on the relationship between teaching and research has been longstanding, and in the opinion of De Witte et al. (Citation2013) is controversial. Leitner et al. (Citation2007) found an ambiguous relationship between teaching and research efficiency in their study of natural and technical faculties at Austrian universities. Johnes (Citation1996) agree that, for simplicity, the performance of universities are analysed separately in the field of teaching and research. Such separate analysis is possible based on the assumption that both types of outputs are independent and have distinct funding mechanisms. This concept is also confirmed by Agasisti (Citation2011), who focused his study of higher education only on the teaching dimension, with the research dimension overlooked to allow a more coherent comparison. De Witte et al. (Citation2013), based on an analysis of thirty studies published by various authors, conclude that the vast majority of them prefer the hypothesis that teaching and research are not intertwined.
Completion rates are influenced by academic selectivity in the teaching process and the selectivity in the admission procedures. Some universities may choose to treat the first year of studies as an additional selection mechanism that ensures selecting only the best students, which, consequently impacting the higher first-year attrition rate (Sneyers & De Witte, Citation2017). Mitić and Mojić (Citation2020) also emphasise the impact of the criteria for choosing a study program by students on the effectiveness of higher education systems. Kenny (Citation2008) points out the difference between effectiveness and efficiency. Efficiency measures how well organisations perform activities and effectiveness how well an organisation is achieving its strategic goals. There is the risk that the drive for efficiency reduces effectiveness and lowers the quality of teaching and learning. According to Cibák et al. (Citation2021) quality education for students is an opportunity to develop the skills and knowledge at the high level that our societies require.
Barra and Zotti (Citation2016) state that high dropout rates may signify that university systems fail to meet the expectations of their students or those young people are using universities as a convenient place to pass a year or two before getting on with their lives. This behaviour is characteristic of a mass access system without selection at the entrance and a high unemployment rate among youth. However, it should be viewed as an inefficient use of public resources, as a significant number of students leave the higher education system without having reached at least the first level of higher education.
According to research based on the true-cohort method conducted in the European Higher Education Area, completion rates range from 48% in Sweden to 88% in Turkey (EACEA, Citation2015). While for Australian public universities analysed in this article, the completion rate, based on the 6-year true-cohort study, for domestic students is 63.6%. The values for individual universities range from 36.4% to 87.7% (University Rankings, Citation2015). However, there is no information about overseas students in this survey.
According to Chen et al. (Citation2017), most existing publications about data envelopment analysis (DEA) applications on university performance evaluation focus on rankings, productivity and efficiency assessment. To the best of their knowledge, few studies consider the problem of graduation rates at universities. Several authors have investigated issues of completion and dropout in various contexts, such as labour market (Schnepf, Citation2017), PhD programmes (Bolli, Agasisti & Johnes, Citation2015), international students (Jung & Kim, Citation2018) or the implementation of the Bologna Process (Agasisti & Haelermans, Citation2016; Chies et al., Citation2019). Vossensteyn et al. (Citation2015) stress that research in this area faces difficulties due to the lack of data and indicators that would make it possible to measure academic success.
3. Australian higher education system and data description
3.1. Description of the Australian higher education system
Before describing the data, a short description of the Australian higher education system is presented (Norton, Citation2019; Williams, Citation2019). This system consists of 37 public universities, three private universities, two foreign universities and 130 other education service providers. Since the mid-1970s, the Federal Government has provided most public funding for universities, despite education being a state matter.
The funding system of Australian public universities has changed over the past 35 years (Norton, Citation2019). Initially, it was a supply-driven system where the Government set the number of undergraduate students in total and at each university and funded it through block grants. However, individual universities decided which courses to offer and which students to choose. Since 2012, when the demand-driven financing system was fully introduced, Universities have had an unlimited number of undergraduate places for which they have received funding. This was the primary reason for the increase in the participation rate in Australia's public higher education system. However, since 2017, a withdrawal from the demand-driven system and a return to the supply-driven system was observed. This was done to lower Government spending, assuming that the subsidy in the following years will be frozen at the level of 2017. Norton (Citation2020) concludes that demand-driven funding was the policy trigger for rapid enrolment increases in Australia between 2009 and 2014. In summary, the increase in enrolment rate is mainly due to political decisions, as in other countries, e.g., Poland.
The globalisation of the world economy causes growth of the rate of internationalisation of studies, practically in all countries. However, Knight (Citation2015) states that internationalisation offers many benefits to higher education and severe risks to the international dimension of higher education. The most important benefits are more internationally oriented staff and students and improved academic quality. At the same time, little evidence exists that internationalisation is seen as a profit-making enterprise for most universities around the world. The most critical risks are the commercialisation and commodification of educational programs and the increase in the number of foreign ‘degree mills’ and low-quality suppliers.
Jiali and Jamieson-Drake (Citation2013) studied the social aspects of internationalisation, indicating that international interactions were consistently and positively correlated with the attainments of domestic students who interacted extensively with overseas students. A higher level of involvement in classes and wider contacts with lecturers was observed. To maximise the benefits, new initiatives are needed to foster more significant interaction between cultures.
Competition between providers is driven by the desire to attract international students and the best domestic students. International students are an essential source of revenue.
International rankings are significant for Australian universities as they influence the choice of universities by international students. As these rankings are based heavily on research performance, most Australian universities highly value research activities. Research activities are subsidized from the income obtained from educating international students, which contributes to improving the position in the international rankings (Williams, Citation2019).
Total education services revenue in 2017 was $32 billion. Between 2017 and 2018, higher education exports totalled $22.2 billion and accounted for 5 per cent of Australia's total exports.
Domestic undergraduate students in public universities are financed by a mix of Federal Government funding (around 60 per cent) and private contributions (around 40 per cent) through an income-contingent loans scheme. At the discipline level, the subsidy to domestic undergraduates varies from 16 per cent for Law and Commerce to around 70 per cent for Agriculture and Health.
Overseas students paying full tuition do not receive funding, just like domestic students who can defer their tuition payments with HELP income-dependent loans (Norton, Citation2019). Instead, overseas students' fees are governed by market forces (only a floor price is defined). The median fees charged to international students who graduated with an undergraduate degree in 2018 ranged from $27,500 to $34,000 per year, depending on the discipline studied. Prices also vary greatly depending on the university's reputation (Norton et al., Citation2018).
In 2015, 1,289,700 students were attending these universities (4.4% doctorate, 22.8% postgraduate, 68.7% undergraduate). Students are diversified according to the type of attendance (71.2% full-time, 28.8% part-time), the citizenship of students (24.6% overseas students), and the mode of attendance (74.3% on-campus, 15.1% off-campus and 10.7% multimodal). The type of attendance, students' citizenship, and the mode of attendance are potential causes of differences in the efficiency of the graduation process.
3.2. Data
Data from all Australian public universities published by the Australian Department of Education and Training (DET, Citation2017), which provides all information on the performance of the Australian higher education system, are used. present the list of universities included in the survey.
Table 1. The list of universities.
Based on the literature review, some basic factors determining academic success are selected. These factors were partly introduced into the model directly, as well they were taken into account in the discussion of the results. The on-time graduation rate and the first-year attrition rate are treated as the primary measures of academic success, taking into account domestic and overseas students separately. This approach is justified because overseas students pay full tuition fees (Abbott & Doucouliagos, Citation2009); thus, their motivation to graduate on time is greater than their domestic counterparts, whom the Government largely subsidises. Abbott and Doucouliagos (Citation2009) confirm that the impact of overseas students on technical efficiency in Australian universities is robust. Factors such as the share of full-time students, the share of internal students and the staff-student ratio were also considered. These factors increase the likelihood of academic success.
The analysis is limited to bachelor's studies, as these students constitute the largest group (67.7% of all students), and it is only for these studies that complete data related to the attrition rate are available (DET, Citation2017). The starting point is the number of students who commenced their studies in 2011 (266,839 candidates) and completed the study in 2015 (170,536 graduates). About 25% of them was overseas students. The average share of part-time bachelor's students in 2015 amounted to 30.6%, ranging from 17% to 60% of all students in the case of individual universities. According to OECD (Citation2013) opinion, full-time students are more likely to complete their education than part-time ones. However, in universities providing distance learning, dropout rates are higher (Zhang & Worthington, Citation2017). Therefore, this issue is a significant problem for the Australian higher education system, as only 74% of students study in the internal mode.
Two groups of important indicators for evaluating the teaching process in the context of the paper's aim are defined. The first one refers only to bachelor's students. The second uses data on all students due to the lack of data on bachelor's students studying full-time or in the internal mode. Information on the number of teachers involved in the bachelor's teaching process is also missing. These indicators are proxies for the characteristics of bachelor's students.
For bachelor's studies, four indicators are defined: the attrition rates for 2011 (defined as the share of students enrolled in a bachelor's course in 2011 who did not complete their first year of studies and did not register for the second year) for domestic (ATTR_D) and overseas (ATTR_O) students and the graduation rates (calculated as the ratio of the number of graduates in 2015 to the number of students commencing in 2011) for domestic (GRAD_D) and overseas (GRAD_O) students. The separate variables for domestic and overseas students are justified by the difference in the values, as shown in .
Table 2. Descriptive statistics of indicators for the 37 universities.
Based on data from 2015, three indicators for all students are formulated: FULL_TIME—the share of full-time students; INT_MODE—the share of students studying in the internal mode; and STUD_STAFF—the student-staff ratio. present the descriptive statistics of all indicators.
The condition ‘the more, the better' must be fulfilled for all the output variables (Cook et al., Citation2014). To meet this condition, the attrition rates for domestic and overseas students (ATTR_D and ATTR_O) are converted to 1-ATTR_D and 1-ATTR_O. Also, STUD_STAFF indicator, the student-staff ratio, is turned into the staff-student ratio. After this transformation, all the variables included in the model meet the postulated condition.
4. Methodology
Composite indicators (CIs) based on DEA are a remarkably useful tool in policy analysis (Guaita Martínez et al., Citation2021). They are also the commonly used method in performance analyses of higher education institutions (De Witte & Hudrlikova, Citation2013). CIs allow the aggregation of multiple sub-indicators into a single measure, enabling comparing many objects according to their multidimensional characteristics. In addition, CIs integrate large amounts of information in a form that is easy to interpret (Shen et al., Citation2011).
Some features of DEA make it especially attractive for the construction of a CI (Murias et al., Citation2008). Benchmarking enables the measurement of performance against real data. The best performance is not a theoretical and abstract concept but is determined by observing the best performer. DEA is the most appropriate method for aggregating sub-indicators because it determines weights endogenously and differentiates their values for all analysed units.
Cherchye et al. (Citation2007) popularised the use of DEA to construct CIs. This approach, known as the BoD–CI construction, is equivalent to the input-oriented DEA model, assuming constant returns to scale, proposed by Charnes et al. (Citation1978). The main difference between the original DEA model and the BoD approach is that the BoD–CI construction examines only achievements without considering the input side (Cherchye et al., Citation2007). As a result, all sub-indicators are the outputs, and the only input is the dummy variable equal to 1 for all objects. In this sense, the dummy input for each object Koopmans (Citation1951) interpreted as the ‘helmsman', which accomplishes specific goals corresponding to different sub-indicators (Murias et al., Citation2008). The super-efficiency procedure, proposed by Andersen and Petersen (Citation1993), is used to obtain a ranking of fully efficient objects. The maximisation problem, which is the input-oriented model with constant returns to scale, can be written for each object k in a linear form as follows:
(1)
(1)
where
is the value of the CI for object k;
is the weight of sub-indicator i for object k;
is the value of sub-indicator i for object k; n is the number of objects incorporated into the analysis, and m is the number of sub-indicators.
Nevertheless, DEA, like other methods, has some disadvantages. One of its main drawbacks is the full flexibility of weighting determination, which can assign zero weights to some of the sub-indicators described. Zero weights may result in basing global performance on a small subset of sub-indicators (Cherchye et al., Citation2007).
Weight restrictions should be added to Equationequation (1)(1)
(1) to avoid this problem (Angulo-Meza & Lins, Citation2002; Cherchye et al., Citation2007). The virtual weight restrictions first proposed by Wong and Beasley (Citation1990) are adopted by entering the following limitations into the model (1) for each output (Angulo-Meza & Lins, Citation2002; Zanella et al., Citation2015):
(2)
(2)
where
and
are the lower and upper bounds, respectively, for output i.
The specification is a value judgement. Such judgements indicate that according to the opinion of the decision-maker, the model better represents the modelled phenomenon because such restrictions are imposed (Wong & Beasley, Citation1990), and the model’s discriminatory power is improved (Angulo-Meza & Lins, Citation2002). The application of weight restrictions requires the classic model to be run without restrictions to determine the initial weight dimension for each output and to apply restrictions accordingly. If the results of a constrained model prove infeasible, then the constraints should be relaxed until the infeasibility disappears (Angulo-Meza & Lins, Citation2002). According to Sarrico and Dyson (Citation2004), imposing restrictions on the virtual weights of outputs requires using an output-oriented model. The output-oriented DEA models with constant returns to scale give the same efficiency scores as input-oriented ones (Van Puyenbroeck, Citation2018; Zanella et al., Citation2015).
5. Results and discussion
The calculations using two models based on the same data set were performed: the basic BoD model described by Equationequation (1)(1)
(1) and the second BoD-R model with additional weights restrictions described by Equationequation (2)
(2)
(2) . The efficiency scores and positions in the ranking and the other indicators used to interpret the results are presented in .
Table 3. Rankings of Australian universities using the proposed approach (in descending order according to the BoD-R model).
The model was validated for domestic students based on completion rates calculated using the true-cohort method. Such validation aims to check the compliance of the results from the proposed model with the true-cohort method. Since the results of the true-cohort studies are published only for domestic students, a new ranking that considers only domestic students was created by modifying the data structure of the BoD-R model. The variables ATTR_O and GRAD_O (attrition rate and on-time graduation rate), which characterise overseas students, were omitted from the calculations (‘BoD-R Domestic' model). In the next step (‘true-cohort Domestic' model), in the modified BoD-R model, the GRAD_D variable was changed to the variable with the values from the cohort study – the completion rate for the cohort that started studies in 2010 and finished them in 2015 (Completion Rates 2018). The results from the two modified models, ‘BoD-R Domestic' and ‘true-cohort Domestic', were compared. The calculated correlation coefficient of the efficiency scores for these two models is 0.988, indicating very high compliance of both models, confirming the usefulness of the proposed concept.
The BoD-R model has higher discriminatory power, as demonstrated by the results. Despite some differentiation, the results from both models are highly positively correlated (0.78), which confirms a high convergence of results.
In the case of the BoD model, there is no university where all variables have non-zero weights. For as many as 17 universities, only one variable had a non-zero weight, and for 11 universities, two variables. Thus, for the vast majority of universities, many variables were omitted from the calculations. This omission is the fundamental premise for supplementing the model with additional weight restrictions.
The positions of universities in the two rankings differ due to the different weighting schemes. After introducing weight restrictions, nineteen universities improved their positions in the ranking, four universities retained their positions, and the positions of fourteen universities dropped. For the five universities that fell the most in the ranking, the BoD model had only one non-zero weight in four cases, and for one university, there were two non-zero weights.
The descriptive statistics () show the differences between domestic and overseas students, confirming the desirability of including these two groups in the model independently. In addition, these two groups also increase the model's flexibility by making it possible to create rankings separately for domestic and overseas students.
Some additional information in the interpretation of the results is included, such as the university's affiliation with a grouping, the year of the university founding, primary data on the subject mix of university courses, and overseas students' participation, which allow explaining the rankings created.
There are four active university groupings in Australia (GROUPS, Citation2018): the Group of Eight (Go8), the Australian Technology Network (ATN), Innovative Research Universities (IRU) and the Regional Universities Network. These groupings act to promote the common objectives of the member universities. Three of them are included in the interpretation of the results. The Go8 includes Australia's leading research universities. The ATN is a coalition of universities focused on the practical dimension of teaching and research. The first two groupings are mostly universities with long traditions dating back to the nineteenth century and the first half of the twentieth century. The IRU includes research universities founded in the 1960s and 1970s. Also, the top five universities from the Online University Rankings list are included (ONLINE, Citation2018). None of these five universities belongs to the above groupings.
The top ten universities in the BoD-R ranking includes all universities from the Go8, one from the ATN and one from the IRU grouping. The second ten includes three universities from the ATN grouping and two from the IRU grouping. Practically all universities provide online education, but the share of external students varies greatly, from 0.3% to 81.9% of all students. Universities that carry out online teaching on a large scale are, in most cases, ranked at the bottom of the ranking (from 25th place). The two exceptions are MONASH (belonging to the Go8), ranked 7th, 8.4% of students studying online, and CURTIN (belonging to the ATN), ranked 19th, with 15.9% of students studying online. UNISA ranked 25th, also belongs to the ATN grouping and has 18.7% of students studying online.
Based on this analysis, it can be concluded that universities with a strong research focus achieve the best results. Also, it can be seen that the universities where students have direct contact with teachers perform better, while the universities dealing with large-scale online learning are at the bottom of the rankings. For example, UNE has 82% of students studying online and is ranked last. However, it is ranked first in the top five online universities (ONLINE, Citation2018) (mainly due to its comprehensive and attractive educational offer).
There is a significant positive correlation (0.51) between the efficiency scores of universities and the share of students studying in science programmes. Thus, the higher the share of science students is, the better the results of the university. A similar analysis was conducted for the efficiency scores and share of students studying in health programmes, with a statistically non-significant negative correlation (-0.23). Thus, universities with a higher share of health students are underperforming. It proves that the best and most motivated candidates choose science studies, so fewer students drop out during the first year, which results in a better on-time graduation rate. The worse performance of universities with a high share of health students may be because these majors are more labour-intensive, resulting in more dropouts and lower on-time graduation rates.
Another analysis concerns the relationship between the efficiency scores and the share of overseas students. The correlation coefficient between these variables is 0.58 and is significant at the 0.001 level. This finding confirms the previously indicated difference in the first-year attrition rates and the share of students graduating on time. Universities with a high percentage of overseas students tend to be better ranked. This result may be because the motivation of such students to complete their studies successfully is higher than that of their domestic peers. In summary, these factors explain, to a large extent, the differences in the position of particular universities in the ranking.
In the top ten universities in the BoD_R ranking, apart from two cases, there are all universities from Go8. It is noteworthy that the universities from Go8 are in the first hundred (except ADELAIDE, which is at the beginning of the second hundred) in the world rankings of Academic Ranking of World Universities (ARWU, Citation2020) and QS World Universities Ranking (QS, 2020). Although ARWU focuses on the evaluation of research activity and QS is mainly directed to candidates choosing universities, the good position of the university in these rankings shows their international reputation. The university's reputation is one of the main factors influencing the choice of a university by candidates (see, for example, Abbott & Doucouliagos, Citation2009; Murias et al., Citation2008). The high international reputation of the universities allows them to attract the best candidates and attain the higher efficiency of the graduation process.
One of the main factors influencing the student decision to choose a university is its prestige (Andersson et al., Citation2017; EACEA, Citation2015). Williams (Citation2019) confirms that ‘international rankings are particularly important for Australian universities as they influence the destination choice of international students, both directly and indirectly by Governments in developing countries specifying the international universities that scholarship winners can attend’. The primary areas of higher education are teaching and research. However, the prestige of a university and its position in the competitive higher education market depends mainly on its research activities. This fact is reflected in the global rankings of universities, such as the most famous and prestigious Shanghai Academic Ranking of World Universities (ARWU). Andersson et al. (Citation2017) stated, ‘that universities with high prestige are more likely to attract ‘good’ students and that they have earned their reputation by producing high-quality output because of this contributory factor'.
6. Conclusion
The policy of open access to higher education, typical for mass education, has its social justification. It gives all potential candidates an equal chance of access to the higher education system but also has negative economic effects in the form of first-year attrition and graduation after a nominal time of the study. The accurate assessment of these effects is not accessible due to their multidimensional nature, so decision-makers need to be provided with the proper measurement tools.
The framework proposed is strictly focused on measuring and assessing efficiency in the context of these adverse effects, based on publicly available statistical data for public universities in Australia. The model allows all stakeholders to understand better the efficiency of the graduation process in universities under study. Indirectly, it allows assessing the open access policy to higher education at different universities by determining the relative efficiency.
The super-efficiency BoD model used, extended by restrictions on virtual weights, allows avoiding the fundamental weakness of nonparametric models, assigning zero weights to variables. Also, it is necessary to include overseas students in the model, which guarantees an entire picture of the efficiency of individual universities.
The proposed model fills the defined gap because it considers overseas students, which gives a complete picture of the efficiency of the diploma process. The introduction of data on domestic and overseas students into the model independently ensures its flexibility. It is possible to analyse both groups separately. Moreover, it allowed for the validation of the model for domestic students. Based on the validation results, it can be concluded that the use of cross-sectional data is bias-free. The proposed model is much simpler than true-cohort research and, therefore, may find broader application in other countries. An analysis was carried out of various factors that help explain the positions obtained by individual universities. Although it is rather a qualitative analysis, its results are confirmed by the results of other research.
The usefulness of the proposed model is confirmed by the achieved findings that are consistent with the results of previous studies of other authors. Full-time students are more likely to complete their studies than part-time students (OECD, Citation2013). The higher attrition rates are in universities providing distance learning (Zhang & Worthington, Citation2017). The impact of overseas students on the efficiency of universities is robust (Abbott & Doucouliagos, Citation2009).
Although the relationship between the efficiency of teaching and research activities of universities is ambiguous (Leitner et al., Citation2007), the results of the presented model indicate that research-oriented universities achieve much better results. However, this is not the result of intensive research activities' direct impact on increasing the quality of teaching, but rather the selection of better-reputable universities, reflected by the good positions of universities in the prestigious ARWU and QS rankings by better candidates.
The analysis of the results considered that the completion rate largely depends on the programme of study. In the interpretation, the share of students in two broad programmes of study: science and health, was used. Further research should examine the impact of the subject mix on academic success in more detail. The proposed framework can be used for any higher education system if the relevant data are available.
Disclosure statement
No potential conflict of interest was reported by the authors.
References
- Abbott, M., & Doucouliagos, C. (2009). Competition and efficiency: Overseas students and technical efficiency in Australian and New Zealand universities. Education Economics, 17(1), 31–57. https://doi.org/10.1080/09645290701773433
- Agasisti, T. (2011). Performances and spending efficiency in higher education: a European comparison through non-parametric approaches. Education Economics, 19(2), 199–224. https://doi.org/10.1080/09645290903094174
- Agasisti, T., & Haelermans, C. (2016). Comparing efficiency of public universities among European countries: Different incentives lead to different performances. Higher Education Quarterly, 70(1), 81–104. https://doi.org/10.1111/hequ.12066
- Agasisti, T., & Johnes, G. (2015). Efficiency, costs, rankings and heterogeneity: The case of US higher education. Studies in Higher Education, 40(1), 60–82. https://doi.org/10.1080/03075079.2013.818644
- Andersen, P., & Petersen, N. C. (1993). A procedure for ranking efficient units in data envelopment analysis. Management Science, 39(10), 1261–1264. https://doi.org/10.1287/mnsc.39.10.1261
- Andersson, C., Antelius, J., Månsson, J., & Sund, K. (2017). Technical efficiency and productivity for higher education institutions in Sweden. Scandinavian Journal of Educational Research, 61(2), 205–223. https://doi.org/10.1080/00313831.2015.1120230
- Angulo-Meza, L., & Lins, M. P. E. (2002). Review of methods for increasing discrimination in data envelopment analysis. Annals of Operations Research, 116(1/4), 225–242. https://doi.org/10.1023/A:1021340616758
- ARWU. (2020). Academic Ranking of World Universities 2019. Retrieved from http://www.shanghairanking.com/ARWU2019.html
- Barra, C., & Zotti, R. (2016). A directional distance approach applied to higher education: An analysis of teaching-related output efficiency. Annals of Public and Cooperative Economics, 87(2), 145–173. https://doi.org/10.1111/apce.12091
- Bolli, T., Agasisti, T., & Johnes, G. (2015). The impact of institutional student support on graduation rates in US Ph.D. programmes. Education Economics, 23(4), 396–418. https://doi.org/10.1080/09645292.2013.842541
- Charnes, A., Cooper, W. W., & Rhodes, E. (1978). Measuring the efficiency of decision making units. European Journal of Operational Research, 2(6), 429–444. https://doi.org/10.1016/0377-2217(78)90138-8
- Chen, Y., Chen, Y., & Oztekin, A. (2017). A hybrid data envelopment analysis approach to analyse college graduation rate at higher education institutions. Infor: Information Systems and Operational Research, 55(3), 188–210. https://doi.org/10.1080/03155986.2016.1262584
- Cherchye, L., Moesen, W., Rogge, N., & Van Puyenbroeck, T. (2007). An introduction to ‘benefit of the doubt’ composite indicators. Social Indicators Research, 82(1), 111–145. https://doi.org/10.1007/s11205-006-9029-7
- Chies, L., Graziosi, G., & Pauli, F. (2019). The impact of the Bologna Process on graduation: New evidence from Italy. Research in Higher Education, 60(2), 203–218. https://doi.org/10.1007/s11162-018-9512-4
- Cibák, L., Kollár, V., & Filip, S. (2021). Measuring and evaluating education quality of future public administration employees at private university in the Slovak Republic. Insights into Regional Development, 3(2), 213–228. https://doi.org/10.9770/IRD.2021.3.3
- Cook, W. D., Tone, K., & Zhu, J. (2014). Data envelopment analysis: Prior to choosing a model. Omega, 44, 1–4. https://doi.org/10.1016/j.omega.2013.09.004
- De Witte, K., & Hudrlikova, L. (2013). What about excellence in teaching? A benevolent ranking of universities. Scientometrics, 96(1), 337–364. https://doi.org/10.1007/s11192-013-0971-2
- De Witte, K., Rogge, N., Cherchye, L., & Van Puyenbroeck, T. (2013). Economies of scope in research and teaching: A non-parametric investigation. Omega, 41(2), 305–314. https://doi.org/10.1016/j.omega.2012.04.002
- DET. (2017). Higher education statistics. https://www.education.gov.au/higher-education-statistics
- DET. (2018). Completion rates of higher education students-cohort analysis, 2005-2014. https://www.education.gov.au/completion-rates-cohort-analyses
- EACEA. (2015). The European Higher Education Area in 2015: Bologna Process implementation report. Publications Office of the European Union.
- EU. (2010). A strategy for smart, sustainable and inclusive growth. https://eur-lex.europa.eu/legal-content/EN/TXT/PDF/?uri=CELEX:52010DC2020&from=EN
- GROUPS. (2018). Groupings of Australian Universities. http://www.australianuniversities.com.au/directory/australian-university-groupings/
- Guaita Martínez, J. M., Martín Martín, J. M., Sol Ostos Rey, M., & de Castro Pardo, M. (2021). Constructing knowledge economy composite indicators using an MCA-DEA approach. Economic Research-Ekonomska Istraživanja, 34(1), 331–351. https://doi.org/10.1080/1331677X.2020.1782765
- Jiali, L., & Jamieson-Drake, D. (2013). Examining the educational benefits of interacting with international students. Journal of International Students, 3(2), 85–101. https://doi.org/10.32674/jis.v3i2
- Johnes, J. (1996). Performance assessment in higher education in Britain. European Journal of Operational Research, 89(1–2), 18–33. https://doi.org/10.1016/S0377-2217(96)90048-X
- Jung, J., & Kim, Y. (2018). Exploring regional and institutional factors of international students' dropout: The South Korea case. Higher Education Quarterly, 72(2), 141–159. https://doi.org/10.1111/hequ.12148
- Kenny, J. (2008). Efficiency and effectiveness in higher education. Australian Universities Review, 50(1), 11–19.
- Kirk, G. (2018). Retention in a bachelor of education (early childhood studies) course: students say why they stay and others leave. Higher Education Research & Development, 37(4), 773–787. https://doi.org/10.1080/07294360.2018.1455645
- Knight, J. (2015). Internationalization brings important benefits as well as risks. International Higher Education, 46, 8–10. https://doi.org/10.6017/ihe.2007.46.7939
- Koopmans, T. C. (1951). Analysis of production as an efficient combination of activities. In T. C. Koopmans (Ed.), Activity analysis of production and allocation (pp. 33–97). Wiley.
- Leitner, K. H., Prikoszovits, J., Schaffhauser-Linzatti, M., Stowasser, R., & Wagner, K. (2007). The impact of size and specialisation on universities’ department performance: A DEA analysis applied to Austrian universities. Higher Education, 53(4), 517–538. https://doi.org/10.1007/s10734-006-0002-9
- Luca, S., Verdyck, M., & Coppens, M. (2014). An approach to estimate degree completion using drop-out rates. Studies in Educational Evaluation, 40, 43–49. https://doi.org/10.1016/j.stueduc.2013.12.001
- Marginson, S. (2016). The worldwide trend to high participation higher education: dynamics of social stratification in inclusive systems. Higher Education, 72(4), 413–434. https://doi.org/10.1007/s10734-016-0016-x
- Mitić, S., & Mojić, D. (2020). Student choice of higher education institutions in a post-transitional country: evidence from Serbia. Economic Research-Ekonomska Istraživanja, 33(1), 3509–3527. https://doi.org/10.1080/1331677X.2020.1774794
- Murias, P., de Miguel, J. C., & Rodriguez, D. (2008). A Composite indicator for university quality assessment: The case of Spanish higher education system. Social Indicators Research, 89(1), 129–146. https://doi.org/10.1007/s11205-007-9226-z
- Norton, A. (2019). Distributing Student Places in Australian Higher Education. Australian Economic Review, 52(2), 217–225. https://doi.org/10.1111/1467-8462.12329
- Norton, A. (2020). After demand driven funding in Australia: Competing models for distributing student places to universities, courses and students. Higher Education Policy Institute.
- Norton, A., Cherastidtham, I., & Mackey, W. (2018). Mapping Australian Higher Education 2018. Grattan Institute.
- OECD. (2013). Education at a Glance 2013: OECD Indicators. OECD Publishing. https://doi.org/10.1787/eag-2013-en
- ONLINE. (2018). University ranking list. https://onlinestudyaustralia.com/university-rankings-list/
- QS. (2020). QS World University Rankings 2020. https://www.topuniversities.com/university-rankings/world-university-rankings/2020
- Sarrico, C. S., & Dyson, R. G. (2004). Restricting virtual weights in data envelopment analysis. European Journal of Operational Research, 159(1), 17–34. https://doi.org/10.1016/S0377-2217(03)00402-8
- Schnepf, S. V. (2017). How do tertiary dropouts fare in the labour market? A comparison between EU countries. Higher Education Quarterly, 71(1), 75–96. https://doi.org/10.1111/hequ.12112
- Shen, Y., Ruan, D., Hermans, E., Brijs, T., Wets, G., & Vanhoof, K. (2011). Modeling qualitative data in data envelopment analysis for composite indicators. International Journal of System Assurance Engineering and Management, 2(1), 21–30. https://doi.org/10.1007/s13198-011-0051-z
- Sneyers, E., & De Witte, K. (2017). The interaction between dropout, graduation rates and quality ratings in universities. Journal of the Operational Research Society, 68(4), 416–430. https://doi.org/10.1057/jors.2016.15
- UNESCO. (1998). World declaration on higher education for the twenty-first century: Vision and action. UNESCO.
- University Rankings. (2015). Bachelor degree completion rates Australian universities. http://www.universityrankings.com.au/degree-completion-rates.html
- Van Puyenbroeck, T. (2018). On the output orientation of the benefit-of-the-doubt-model. Social Indicators Research, 139(2), 415–431. https://doi.org/10.1007/s11205-017-1734-x
- Vossensteyn, H., Stensaker, B., Kottmann, A., Hovdhaugen, E., Jongbloed, B., Wollscheid, S., … Cremonini, L. (2015). Dropout and completion in higher education in Europe. Main report. Publications Office of the European Union. https://doi.org/10.2766/826962
- Williams, R. (2019). Australian Higher Education as an Industry. Australian Economic Review, 52(2), 212–216. https://doi.org/10.1111/1467-8462.12331
- Wong, Y. H. B., & Beasley, J. E. (1990). Restricting weight flexibility in data envelopment analysis. The Journal of the Operational Research Society, 41(9), 829–835. https://doi.org/10.2307/2583498
- World Bank. (2018). School enrollment, tertiary (% gross). http://databank.worldbank.org/data/reports.aspx?source=2&series=SE.TER.ENRR&country#
- Yue, H., & Fu, X. (2017). Rethinking graduation and time to degree: A fresh perspective. Research in Higher Education, 58(2), 184–213. https://doi.org/10.1007/s11162-016-9420-4
- Zanella, A., Camanho, A. S., & Dias, T. G. (2015). Undesirable outputs and weighting schemes in composite indicators based on data envelopment analysis. European Journal of Operational Research, 245(2), 517–530. https://doi.org/10.1016/j.ejor.2015.03.036
- Zhang, L. C., & Worthington, A. C. (2017). Scale and scope economies of distance education in Australian universities. Studies in Higher Education, 42(9), 1785–1799. https://doi.org/10.1080/03075079.2015.112681