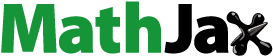
Abstract
This study employs housing data from the nine main regions of England and from overall England to compare the volatility risks of housing prices for the first-time buyer (FTB) and former owner-occupier (FOO) markets. This study collects data from February 2012 to March 2020 to assess the volatility characteristics of housing prices in these two markets in each region of England. Additionally, the effect of interest rate shocks and the wealth effect of the stock market on these two markets are observed. The results reveal that for most regions, the FTB market has higher housing price volatility risks compared with the FOO market. Additionally, the FTB market is more easily affected by interest rate shocks and the wealth effect. In contrast to FOOs, FTBs are generally younger and less wealthy. If FTBs are facing a market with higher housing price risk, especially when the overall economy is affected by a shock (such as the European Debt Crisis or the COVID-19 pandemic), the response of the FTB housing market is greater; then the government must pay special attention when implementing policies that interfere with the market since these policies would particularly affect the welfare of FTBs.
1. Introduction
The goal of this study is to compare housing price risks between the first-time buyer (FTB) market and former owner-occupier (FOO) market in England, which is to observe whether market structures composed of different trader types result in different housing price responses. Because FTBs and FOOs have largely different financial conditions, the volatility of transaction prices in the FTB and FOO housing markets can differ greatly. Numerous studies on the other asset markets (e.g., stock market) have analysed the asset transaction prices associated with various buyers and documented the effect of buyer characteristics on asset prices. However, studies on these two markets (FTB and FOO) are rare in the real-estate market field; therefore, this study analyses the behaviors of real estate traders to provide empirical evidence to the literature.
Although there are a few theoretical studies which have investigated the influence of buyer characteristics on the housing market, empirical studies on this topic remain inadequate. For example, based on the model of Ortalo-Magné and Rady (Citation2006), Benito (Citation2006) proposes that the volatility of the housing price inflation of FOO properties should be greater than that of FTB properties. Benito (Citation2006) also shows that the series for FOOs peaks at a high level in the two housing booms during the sample period. Benito claims that this finding is consistent with Ortalo-Magné and Rady’s (Citation2006) finding of a stronger overshooting tendency of the prices of properties bought by owner-occupiers than that of FTBs.
Studies on this topic are relatively rare in the real estate field; however, many scholars have proposed that the behaviors of real estate traders are worthy of research. For example, Tanaś et al. (Citation2019) find that gender and age would affect the preferences of real estate traders. Ortalo-Magné and Rady (Citation2004) confirm that housing demand fluctuations are key drivers of housing transactions, particularly of changes in housing demand from FTBs. Using models with down-payment constraints, Ortalo-Magné and Rady (Citation2006) propose that the demand, and hence income, of FTBs is especially important to determine the housing price and show that housing prices in the United States follow the incomes of the young population (a proxy for FTBs) more strongly than those of the wider population. In addition, scholars also found that the behaviors of traders can explain the characteristics of house prices and trading volume. Although these studies have discussed the behaviors of traders in the housing markets, few studies have compared the property price risk of FTBs and FOOs. Theoretical models usually study one trader type. For example, the model in Stein (Citation1995) considers only FOOs. Ortalo-Magné and Rady’s (Citation2006) model shows that housing prices overshoot in response to a permanent income shock if only FOOs’ house purchases (i.e., excluding the purchases made by FTBs) are taken into account.
The key difference between FTBs and FOOs is their financial conditions. Because FOOs already own real estate property, they have more sufficient capital. FOOs even need to consider the taxation of real estate transaction income (such as: the land transfer tax) when changing houses (Han et al., Citation2021). By comparison, FTBs generally require loans or are subject to higher loan ratios than FTBs are because they have insufficient self-prepared funds. With fewer financial constraints, FOOs may be offered more favorable loan terms, such as higher loan-to-value ratios or lower interest rates, which differs from FTBs who may be subject to high-ratio loans alongside high interest rates. Therefore, the impact of interest rates on the two types of home buyers may be different.
In addition to the interest rate, increased stock market prices may introduce a wealth effect to housing prices. A wealth effect indicates that increased stock wealth may elevate people’s wealth (e.g., rising housing prices) and in turn increases the amount of consumption (Sousa, Citation2010). Under different financial conditions, the impact of wealth effect on the two types of traders might also be different.Footnote1 Hence, in addition to comparing the volatile risks of housing prices faced by FTBs and FOOs, this study observes the effects of interest rate shocks and the wealth effect on FTB housing markets and FOO housing markets (hereinafter collectively referred as ‘the two markets’).
Bissoondeeal (Citation2021) tests whether there is a statistical relationship between stock prices and house prices in the UK, and claims that it is the first study to analyse this issue at the regional level in the UK. In this current paper, we not only examine the relationship between stock prices and housing prices in different regions of the UK, but also compare data from the two housing markets (FTB housing markets and FOO housing markets). Therefore, this paper can supplement the literature.
Data on housing prices in the nine regions in England and in the overall England housing market are adopted to assess the volatile risks of housing prices faced by FTBs and FOOs. Through this, the risk characteristics of the two markets are compared and the effect of interest rate shocks and the wealth effect on them are inspected. Since the subprime mortgage crisis of 2007, this topic has become particularly critical. This crisis occurred because the public underestimated the risks associated with subprime mortgages and changes in housing prices. Cipollini and Parla (Citation2020) use the data among 93 Italian provincial housing markets. They also find that the exogenous shocks, interpreted as negative housing demand shocks, can affect the house prices and sales in the Italian regional markets. Despite housing markets worldwide having recovered from the subprime mortgage crisis, the price risk faced by different types of traders in the housing market continues to be overlooked, which may result in another mortgage crisis in the future.
The results of this study can provide observation of whether the volatility risk of housing prices changes with the structure of real estate traders. Among recent analyses on financial markets, those on market microstructures have garnered attention from scholars. Multiple scholars in this field have proposed that market systems, trading behavior, and the structure of traders in the market (i.e., the participation proportion of liquidity traders and informed traders) affect the efficiency of trading prices (e.g., Entrop et al., Citation2020; Gupta et al. Citation2019; Schwartz, Citation2021; Wang et al., Citation2021).
The subject of this research is currently more important because the growing wealth gap remains a concern in the global economy; current economic development imposes disadvantageous employment and financial conditions on young adults. Relative to FOOs, FTBs are generally younger and less wealthy. If FTBs are faced with higher housing price risks, particularly when the overall economy is impacted by shocks, the FTB housing market will demonstrate large reactions. Accordingly, the government should directly prevent economic impacts or establish policies to mitigate their effect on young adults or citizens with less wealth. Additionally, the government should be tentative when implementing polices that influence the market because such policies easily influence FTBs.
The paper is organised as follows. Section 2 discusses relevant studies which show that the market for different types of traders will have different price behaviors. The FTB and FOO housing markets may react differently when affected by interest rates and stock prices. Section 3 describes the data and illustrates the empirical evidences, and Section 4 concludes this study.
2. Literature review
2.1. The market for different types of traders will have different price behaviors
The markets for many different types of assets have found that differences in the types of traders affect the behavior of prices in the market. Taking a futures market as an example, Fung and Tsai (Citation2021) find that heterogeneous day traders have different effects on price discovery. Huang et al. (Citation2021), on the other hand, take the trading of Taiwan’s stock and futures markets as an example, and show that there are information asymmetries between different types of institutional traders, affecting expected returns.
This study compared the price volatility risk of the two property types to determine whether differences existed between the FTB and FOO housing markets. Previous studies have also compared different housing markets. Marshall and Marsh (Citation2007) discuss consumer and investment demand for manufactured housing units and find that the elasticities of demand are different for consumers as opposed to investors. Therefore, Marshall and Marsh (Citation2007) suggest that policy makers should delineate the signals sent to consumers and investors when implementing housing programs.
Other studies have examined the behavior of traders in the same type of markets in different countries. For example, Andrew et al. (Citation2006) compare the transition of young adults from renting to first-time homeownership in Britain and the United States. By adopting a common theoretical and methodological framework, Andrew et al. (Citation2006) identify behavioral similarities and differences in transitions in the two countries and find that the high ownership rates among British young adults are caused by quick transitions. In addition, the two populations’ responses to income and wealth are substantially different: young adults’ transitions to homeownership in Britain are more responsive.
At the present time, it is particularly important to discuss the behavior of young people or FTBs and the housing markets they face. Because current economic development imposes disadvantageous employment and financial conditions on young adults (Rainsford et al., Citation2019), Said (Citation2016) also points out that factors such as interest rates, stock markets, and financial turmoil are also more likely to affect young people. The study of Qiu et al. (Citation2020) find that different types of home buyers (such as differences in income, age, education, and occupation) may have different abilities and willingness to find houses and bargain. In contrast to FOOs, FTBs are generally younger and less wealthy. If FTBs are faced with higher housing price risks, particularly when the overall economy is impacted by shocks, the FTB housing market will demonstrate large reactions.
2.2. Affected by interest rates and stock prices, the FTB and FOO housing markets may react differently
Studies have compared various housing markets. For example, Sing et al. (Citation2006) examine the connection between the public resale housing and private housing submarkets in Singapore. Marshall and Marsh (Citation2007) discuss consumer and investment demand for manufactured housing units. Other studies have also compared the behavior of traders in the same market but in different countries. For instance, Andrew et al. (Citation2006) compare the transition of young adults from renting to first-time homeownership in Britain and the United States.
Because most of the previous studies determine why housing markets differ by comparing differences in their housing prices, this study adopts the same concept and compare the prices of the properties owned by various traders to derive the possible causes of price volatility risk. In addition, the wealth effect and interest rates are the fundamental factors that determine whether the housing price is reasonable, drastic changes in these macroeconomic variables also fundamentally transform the housing prices. Hence, the present study measures the factor risk of the housing prices to respond to interest rates and the performance of stock markets in different housing markets.
A large number of studies have demonstrated the considerable effect of the wealth effect and interest rates on housing prices. It is also found that these two factors have different effects on different traders. For example, Bhutta and Ringo (Citation2021) find that the impact of interest rates on home purchases will be affected by the income of traders. This influential difference may be due to the different financing conditions of traders. It is found in Carozzi (Citation2020) that during the financial turmoil (the housing bust of 2008–2009), young households with scarce financial resources were the most affected because they were more susceptible to financing restrictions.
Ortalo-Magné and Rady (Citation2004, Citation2006) propose a property ladder based on the mobility model, which predicts increased housing wealth enhances the housing mobility of households. The evidence shown in Stein (Citation1995) and Ortalo-Magné and Rady (Citation2004, Citation2006) is consistent with the model, which determines increases in the housing utilities of households by trading up starter flats for large and high-quality houses. The above-mentioned documents all illustrate the influence of wealth effect on the decisions of exchanging houses. However, for young people with less wealth, more recent studies have found that because of rising housing prices, it is more and more difficult for young people to buy a house, and the age of the first house purchase is increasing. More recent studies have found that rising housing prices lead the age of the first home purchase to be increasing (Dettling & Hsu, Citation2018; Fry, Citation2013; Thompson, Citation2012). The literature also found that the influence of young people's ability to buy a house for the first time is prone to exogenous factors, such as financial support from parents (Coulter, Citation2017; Druta & Ronald, Citation2017). These differences may cause the correlation between the FOO and FTB property prices and stock prices to be different, that is, the wealth effects of the two markets are different.
According to the above literature, we infer that the financing restrictions caused by scarce financial resources may make FTBs more susceptible to interest rate risks. The property ladder based on the mobility model proposed by Ortalo-Magné and Rady (Citation2004, Citation2006) implies that the wealth effect caused by the rise in the stock market only tend to spill over to the FOO market. When interest rates and stock prices change, FTB and FOO may react differently. Hence, the present study explored the differences and correlations in the housing prices for FTBs and FOOs to determine whether information in the two trader markets differed.
3. Empirical models
By using data on the England housing market to evaluate the house price risks of the FTB and FOO housing markets, this study compares the risk characteristics and the transmission of risk information in the two markets and inspects how two risk factors, namely interest impact and the wealth effect, influence the risks in each market. To compare two markets in the same region, this study first estimates the relationship between the returns and volatilities in the FTB and FOO housing markets. Vector autoregressive (VAR) models are commonly used when considering the relationship between market returns (e.g., Montagnoli & Nagayasu, Citation2015; Tomal, Citation2020), whereas multivariate generalised autoregressive conditional heteroskedasticity (MGARCH) is often used when considering the relationship between market risks (volatility). Karolyi (Citation1995) uses these two models to estimate the return of international stock returns and risk transmission. However, the estimation method used by Karolyi conducts estimations separately using the VAR and MGARCH models, and is thus unable to distinguish whether the correlation between the markets is attributed to returns or risks.
Recent literature has employed the VAR-MGARCH model to estimate the correlation between returns and risks (Andreasson et al., Citation2016; Bekiros, Citation2014; Jiang et al., Citation2015; Sogiakas & Karathanassis, Citation2015). The VAR-MGARCH model is applicable in model frameworks that involve multiple variables and can be used to determine whether the influence on them is attributed to returns or volatility. However, this model does not consider the influence of exogenous factors on volatility. In addition to simultaneously estimating market returns and volatility to compare market risks, this study observes the response of each market when impacted by risk factors; hence this paper uses the Vector autoregressive - Multivariate Generalised Autoregressive Conditional heteroscedasticity -XFootnote2 (VAR-MGARCH-X) model. Adding heteroskedastic volatility into the VAR model increases its rigorousness in impulse response analysis (Hafner & Herwartz, Citation2006; Le Pen & Sévi, Citation2010). To clarify whether the differences existed between the FTB and FOO housing markets and to further discuss which factors affect market risk, the present study extends the model of Bekiros (Citation2014), which is briefly introduced as follows:
Let be the regional house price return of the FTB housing markets,
be the regional house price return of the FOO housing markets, as well as
and
be the residues of the VAR model, which follow a MGARCH process.
(1)
(1)
(2)
(2)
The conditional variance is given as follows.
(3)
(3)
Subsequently, two exogenous factors are considered to discuss whether price volatility risk is affected by economic shocks. The model in EquationEquation (3)(3)
(3) is revised as follows:
(4)
(4)
and
denote long-term interest rate and FTSE 100 stock price index, respectively.
denotes the change rates in the variable
at time
Hence, EquationEquation (4)
(4)
(4) includes exogenous factors to discuss whether they affect the volatility of housing price returns and the transmission between the housing price risk of FTBs and FOOs.
As previously mentioned, this study employs the VAR-MGARCH-X model to estimate housing market risks and compare the effects of interest rate shocks and the wealth effect in the stocks market on housing price risks in different housing markets. Additionally, the volatility impulse response function (VIRF) is adopted to quantify risk contagion in both markets.
4. Data and empirical results
4.1. Preliminary analysis
Monthly data from the regional housing markets in England from 2012 M1 to 2020 M3 are used in this study. These housing price data are obtained from the Nationwide housing price series. The Nationwide housing price methodology has been developed, and the dataset of housing prices is provided by the Nationwide Building Society. The data collected in this study are the housing prices in England and in the nine large regions, namely, North East, North West, Yorkshire and The Humber, East Midlands, West Midlands, East, London, South East, and South West.Footnote3 To observe the degree of sensitivity in FOO and FTB housing prices to changes in macroeconomic variables, a Datastream database, in addition to housing price, is employed to obtain the macroeconomic variable data. In this study, data on UK public debt with a 10-year duration are adopted for measuring the effect of the long term interest rates on housing prices. FTSE 100 stock price index is also adopted for determining whether the wealth effect existed.
Simple statistics for the housing price indices are listed in Appendixes . This study employs the housing price index (HPI) for estimation to prevent heterogenous influence on the data. When observing the housing price trends, this study plots the original housing prices to more clearly describe the preferences of these two types of traders. and reveal that for both the FTB and FOO housing markets, regions with high housing prices are centralised in the Southeastern and Southern regions of England. The trends of the original housing prices and the housing price indices are shown in and . First of all, it can be found from and that, regardless of the FTB housing market or the FOO housing market, the areas with high housing prices in England are concentrated in the southeast and south.
Table 1. Causality tests between the FTB and FOO markets.
Table 2. The VAR-MGARCH-X model.
A comparison of Appendix shows that the cost of properties owned by FOOs is significantly higher than that of properties owned by FTBs. The England's data in and show that the average costs of the properties owned by FOOs and FTBs are £245,883 and £181,995, respectively, demonstrating that the wealth and ability to afford properties differed between the two trader types. Because the FOOs are switching houses—that is, selling an old house to buy a new house—the value of the old house can be used to fund the purchase of the new house. Coupled with the fact that people trade up in property quality and location, the price of the properties FOOs purchase is generally high. However, a high housing price does not necessarily imply high price risk. Because FTBs and FOOs have varying financial constraints, the prices of the properties they purchase and sell differ.
The stationarity of these two kinds of housing prices should be tested before the series is empirically tested. This study applies the Phillips and Perron (Citation1988) test. Appendix summarise the unit root test results. The statistics all suggest that the two kinds of housing prices are non-stationary and are all I(1) series. Appendix lists the two macroeconomic variable data used in this study and illustrates the changing trend of variables over time. Appendix also shows that the two variables are also I(1) series. Hence, in the empirical parts of this paper, the change rates of the variables are used to avoid the problem of nonstationary.
Table 3. Causality tests in mean and in variance.
Appendix reveals a 1.66 mean interest rate during the data collection period. indicates that August 2013 is the month in which the interest rate peaks (2.89%). Subsequently, the interest rates drop. By March 2020, the interest rate is lower than 0.5%. From 2012, the stock market index gradually increases as Europe recovers from the 2010–2011 European Debt Crisis. However, in 2020, the COVID-19 pandemic considerably and severely affects the global economy, resulting in the UK stock market index dropping in February and March. During both months, the correction rates exceed 10%. The economic shocks affect the housing prices; however, different types of trader have different responses when they are faced with the impact. As a result, transaction prices reflect the speed of information spread or the amplitude of the impact, which leads to differences in housing price volatility (risks). Therefore, this study employs the following methods to estimate the volatility risks of housing prices.
4.2. Empirical results
To detail the advantages that the model uses to estimate returns, volatility, and risk factors (namely the VAR-MGARCH-X model), this study first uses the VAR model, which only estimates returns between different markets, to estimate the causal relationship between the two markets. In recent studies, when estimating the VAR model, the spillover index proposed by Diebold and Yilmaz (Citation2009) is also evaluated. As a measure of market relevance, this paper also estimates the spillover indices of the FTB and FOO housing markets in different regions. The results are presented in .
Although and suggest that the HPIs of the FTB and FOO housing markets are relatively similar, the estimates in indicate that the FTB and FOO housing markets are only correlated in four regions, which show that FTB housing market unilaterally affecting the FOO housing market. The West Midlands region of England is the only region in which the FOO housing market affects the FTB housing market. From the national standpoint, the FTB housing market unilaterally affects the FOO housing market. Causality testing of the market results () reveals that even though the FTB housing market has faster responses, the FTB and FOO housing markets are independent from each other in most regions. In addition, in all regions, the spillover indices between the two markets do not exceed 50%. This number is significantly lower than the spillover effects found in other markets in the past empirical literature. For instance, Tsai and Lin (Citation2019) study the US housing market and find that the spillover index between major cities is higher than 80%. A possible reason of that the correlation between the markets is not as high as expected follows. In , the effects of volatility and risk factors are not estimated. Additionally, the model used for does not consider heterogenous volatility, and is thus unable to estimate and compare the time-varying risks.
presents the estimation results computed using the VAR-MGARCH-X model; plots the estimated volatility of the FTB and FOO housing markets listed in , presenting the changes in risks in both markets. indicates that in most regions for the majority of time, the FTB housing market has higher risks of volatility prices, particularly when market volatility suddenly increases. Examples of such instances include the Brexit referendum in 2016 and the COVID-19 outbreak at the start of 2020. These sudden economic impacts immediately affect the interest rates and stock market, which further affect trader income and wealth and impacting the housing transaction price. reveals that for in most regions, the price volatility risks of the FTB housing market are higher, which then reflect greater degrees of information contagion.
The model in comprises three estimation coefficients. One is the coefficient for the VAR model (mean equation), which is used to estimate returns; another is the coefficient for the MGARCH model (variance equation), which is used to evaluate volatility; and the other is the estimation coefficient for the exogenous variable of the variance equation, which is used to evaluate the influence of risk factors. These three estimation coefficients can be used to inspect the correlation between the returns, volatility transmission, and the influence of risk factors between the FTB and FOO housing markets. For clarification, the estimation coefficients are presented in , return-related and volatility transmission results are listed in , and the estimated impacts of the risk factors are displayed in .
Table 4. Factor risk.
The mean equations in and reveal the estimated results for England, indicating that the FTB and FOO housing markets mutually influence each other. In four regions situated in Central and Southern England, the finding is that the housing prices of the FTB and FOO housing markets mutually influence each other. On the other hand, in the South East region, the FTB housing market unilaterally affects the FOO housing market. indicates that aside from the three Southern regions with high housing prices, namely London, the South East, and the South West, it is revealed that volatility in the FTB and FOO housing markets transfers from one to the other. presents the VIRF results, which depict the risk contagion effect between the two markets and indicates that volatility in the FTB housing market is affected by the impact in the FOO housing market, whereas volatility in the FOO housing market is affected by the impact in the FTB housing market. indicates that for most regions which are influenced by the FTB housing market, the FOO housing market has greater volatility response and longer impact periods. Only in Southeastern regions (East and South East) with high housing prices does the FOO housing market exhibit a rapid convergence of impact. However, even in these regions, most of the risks are transferred from the FTB housing market to the FOO housing market.
The results presented in indicate that interest rate risk factors have significant effects on the volatility of the FTB housing market in five regions and that of the FOO housing market in four regions and England. However, the wealth effect only has a significant effect on the FTB housing market of four regions and the FOO housing market of two regions. Aside from two western regions, namely the West Midlands and the South West, in all other regions of England and in England, the two external risk factors significantly affect the volatility of the two markets. This result is consistent with those of , which indicates that the risk impacts of the FTB and FOO housing markets on each other are significant. However, in , the response size and speed indicate that risk impact is transmitted from the FTB housing market to the FOO housing market. The results in this paper all show that the housing market risks faced by the FTBs are relatively high. In addition, past studies have found that the FOO housing market is prone to have larger price increases (Benito, Citation2006). This evidence shows that the FTBs are at a disadvantage when faced with fluctuations in the housing market.
5. Conclusion
This study analyses property transaction data on FTBs and FOOs to determine whether the ability of the housing price to respond to market conditions in the housing market differs according to trader type. Whether house price risk varied with the trader type is explored. The housing prices in nine major England's regions as well as overall England's housing prices are used to estimate.
The results show that the housing prices of FTBs is responsive to market conditions faster than that of FOOs. Because FOOs sell old properties to buy new properties, they are unaffected by the financial burden of a mortgage. Therefore, the decisions of FOOs to purchase houses may not be swayed by interest rates and stock wealth. On the other hand, this study also reveals that in most regions, the FTB housing market consists of higher price volatility risk and that the risk impact is transmitted from the FTB housing market to the FOO housing market. However, in some southeastern regions and southern regions with higher housing prices, the FOO housing market either is independent of the FTB housing market, or rapidly converges after an impact from the FTB housing market. Possible reasons for this include different influential factors in the FOO housing market of regions with higher housing prices. Future studies may investigate the major factors that affect FOOs’ house-purchasing decisions.
This paper not only identifies different risk characteristics exhibited by the price of properties owned by FTBs and FOOs, also explores whether the housing prices of properties owned by the two trader groups were correlated. The prices of properties owned by FOOs are more rigid, and changes in the volatility of properties owned by FOOs are slower than those owned by FTBs.
The empirical results of this study reveal higher housing price risks in the FTB housing market. Additionally, transaction prices in the FTB housing market demonstrate greater responses when they are subjected to the overall economic impact than do the transaction prices in the FOO housing market. Possible reasons include FTBs having a more limited budget and financial restrictions. By contrast, FOOs are subjected to fewer restrictions during decision making, and thus, they enjoy greater autonomy. Compared to FOOs, FTBs are generally younger and less wealthy. The results of this paper imply that FTB faces a higher risk of housing prices, especially when the overall economy volatility increases, the FTB housing market will show a greater response. Therefore, the government should directly prevent economic impact or formulate policies to reduce its impact on young people or citizens with less wealth. In addition, the government should be tentative when implementing policies that affect the market, because such policies can easily affect FTB. In addition to recommending that the government should focus on the risks imposed by macroeconomic impact on FTBs and to implement subsidy measures for them when promulgating policies that influence the market, the results of this study raises the importance of studying the behavior of first-time home buyers.
Acknowledgements
We are immensely grateful to Professor Marinko Škare (Editor) and the two anonymous referees for the constructive comments of this paper.
Disclosure statement
No potential conflict of interest was reported by the authors.
Table
Appendix Table A1. Descriptive statistics and unit root tests (FTB properties).
Appendix Table A2. Descriptive statistics and unit root tests (FOO properties).
Appendix Table A3. Descriptive statistics and unit root tests for the two factors.
Additional information
Funding
Notes
1 Determining the effect of the housing market on consumption by comparing variations in housing, stock wealth, and other variables in every state of the US, Calomiris et al. (Citation2013) report that a wealth effect is influenced by age composition, wealth distribution (with the poverty rate considered the proxy variable), and wealth combinations (estate and stock proportions in total wealth). The results indicate small wealth effects on housing in states with many young people (who were likely to be credit-constrained), whereas large wealth effects were found in states with older homeowners.
2 X denotes a covariate
3 Please see the website (http://www.nationwide.co.uk/) for more information of the housing price series.
References
- Andreasson, P., Bekiros, S., Nguyen, D. K., & Uddin, G. S. (2016). Impact of speculation and economic uncertainty on commodity markets. International Review of Financial Analysis, 43, 115–127. https://doi.org/10.1016/j.irfa.2015.11.005
- Andrew, M., Haurin, D., & Munasib, A. (2006). Explaining the route to owner-occupation: A transatlantic comparison. Journal of Housing Economics, 15(3), 189–216. https://doi.org/10.1016/j.jhe.2006.09.002
- Bekiros, S. D. (2014). Exchange rates and fundamentals: Co-movement, long-run relationships and short-run dynamics. Journal of Banking & Finance, 39, 117–134. https://doi.org/10.1016/j.jbankfin.2013.11.007
- Benito, A. (2006). The down-payment constraint and UK housing market: Does the theory fit the facts? Journal of Housing Economics, 15(1), 1–20. https://doi.org/10.1016/j.jhe.2006.02.001
- Bhutta, N., & Ringo, D. (2021). The effect of interest rates on home buying: Evidence from a shock to mortgage insurance premiums. Journal of Monetary Economics, 118, 195–211. https://doi.org/10.1016/j.jmoneco.2020.10.001
- Bissoondeeal, R. K. (2021). The links between regional house prices and share prices in the UK. Regional Studies, 55(2), 256–268. https://doi.org/10.1080/00343404.2020.1795108
- Calomiris, C. W., Longhofer, S. D., & Miles, W. (2013). The housing wealth effect: The crucial roles of demographics, wealth distribution and wealth shares. Critical Finance Review, 2(1), 49–99. https://doi.org/10.1561/104.00000008
- Carozzi, F. (2020). Credit constraints and the composition of housing sales. Farewell to first-time buyers? Journal of the European Economic Association, 18(3), 1196–1237. https://doi.org/10.1093/jeea/jvz017
- Cipollini, A., & Parla, F. (2020). Housing market shocks in Italy: A GVAR approach. Journal of Housing Economics, 50, 101707. https://doi.org/10.1016/j.jhe.2020.101707
- Coulter, R. (2017). Local house prices, parental background and young adults’ homeownership in England and Wales. Urban Studies, 54(14), 3360–3379. https://doi.org/10.1177/0042098016668121
- Dettling, L. J., & Hsu, J. W. (2018). Returning to the nest: Debt and parental co-residence among young adults. Labour Economics, 54, 225–236. https://doi.org/10.1016/j.labeco.2017.12.006
- Diebold, F. X., & Yilmaz, K. (2009). Measuring financial asset return and volatility spillovers, with application to global equity markets. The Economic Journal, 119(534), 158–171. https://doi.org/10.1111/j.1468-0297.2008.02208.x
- Druta, O., & Ronald, R. (2017). Young adults’ pathways into homeownership and the negotiation of intra-family support: A home, the ideal gift. Sociology, 51(4), 783–799. https://doi.org/10.1177/0038038516629900
- Entrop, O., Frijns, B., & Seruset, M. (2020). The determinants of price discovery on bitcoin markets. Journal of Futures Markets, 40(5), 816–837. https://doi.org/10.1002/fut.22101
- Fry, R. (2013). A rising share of young adults live in their parent’s home: A record 21.6 million in 2012. Pew Research Center report. https://www.pewresearch.org/social-trends/2013/08/01/a-rising-share-of-young-adults-live-in-their-parents-home/
- Fung, S., & Tsai, S.-C. (2021). The price discovery role of day traders in futures market: Evidence from different types of day traders. Journal of Empirical Finance, 64, 53–77. https://doi.org/10.1016/j.jempfin.2021.08.001
- Gupta, R., Lau, C. K. M., Plakandaras, V., & Wong, W.-K. (2019). The role of housing sentiment in forecasting U.S. home sales growth: Evidence from a Bayesian compressed vector autoregressive model. Economic Research-Ekonomska Istraživanja, 32(1), 2554–2567. https://doi.org/10.1080/1331677X.2019.1650657
- Hafner, C. M., & Herwartz, H. (2006). Volatility impulse responses for multivariate GARCH models: An exchange rate illustration. Journal of International Money and Finance, 25(5), 719–740. https://doi.org/10.1016/j.jimonfin.2006.04.006
- Han, L., Ngai, L. R., & Sheedy, K. D. (2021). To own or to rent? The effects of transaction taxes on housing markets. Working paper.
- Huang, H.-G., Tsai, W.-C., Weng, P.-S., & Wu, M.-H. (2021). Volatility of order imbalance of institutional traders and expected asset returns: Evidence from Taiwan. Journal of Financial Markets, 52, 100546. https://doi.org/10.1016/j.finmar.2020.100546
- Jiang, J., Marsh, T. L., & Tozer, P. R. (2015). Policy induced price volatility transmission: Linking the U.S. crude oil, corn and plastics markets. Energy Economics, 52, 217–227. https://doi.org/10.1016/j.eneco.2015.10.008
- Karolyi, G. A. (1995). A multivariate GARCH model of international transmissions of stock returns and volatility: The case of the United States and Canada. Journal of Business & Economic Statistics, 13(1), 11–25.
- Le Pen, Y., & Sévi, B. (2010). Volatility transmission and volatility impulse response functions in European electricity forward markets. Energy Economics, 32(4), 758–770. https://doi.org/10.1016/j.eneco.2009.12.003
- Marshall, M. I., & Marsh, T. L. (2007). Consumer and investment demand for manufactured housing units. Journal of Housing Economics, 16(1), 59–71. https://doi.org/10.1016/j.jhe.2006.09.001
- Montagnoli, A., & Nagayasu, J. (2015). UK house price convergence clubs and spillovers. Journal of Housing Economics, 30, 50–58. https://doi.org/10.1016/j.jhe.2015.10.003
- Ortalo-Magné, F., & Rady, S. (2004). Housing transactions and macroeconomic fluctuations: A case study of England and Wales. Journal of Housing Economics, 13(4), 287–303. https://doi.org/10.1016/j.jhe.2004.09.005
- Ortalo-Magné, F., & Rady, S. (2006). Housing market dynamics: On the contribution of income shocks and credit constraints. Review of Economic Studies, 73(2), 459–485. https://doi.org/10.1111/j.1467-937X.2006.383_1.x
- Phillips, P. C. B., & Perron, P. (1988). Testing for a unit root in time series regression. Biometrika, 75(2), 335–346. https://doi.org/10.1093/biomet/75.2.335
- Qiu, L., Tu, Y., & Zhao, D. (2020). Information asymmetry and anchoring in the housing market: A stochastic frontier approach. Journal of Housing and the Built Environment, 35(2), 573–591. https://doi.org/10.1007/s10901-019-09701-y
- Rainsford, E., Maloney, W. A., & Popa, S. A. (2019). The effect of unemployment and low-quality work conditions on work values: Exploring the experiences of young Europeans. The Annals of the American Academy of Political and Social Science, 682(1), 172–185. https://doi.org/10.1177/0002716219830378
- Said, M. (2016). Arab Youth Employment in the Wake of the global financial crisis. In E. A. Sayre and T. M. Yousef (Eds.), Young generation awakening: Economics, society, and policy on the Eve of the Arab Spring (pp. 50–71). Oxford University Press.
- Schwartz, R. A. (2021). A market microstructure view of the informational efficiency of security prices. The Journal of Portfolio Management, 47(8), 75–84. https://doi.org/10.3905/jpm.2021.1.268
- Sing, T.-F., Tsai, I.-C., & Chen, M.-C. (2006). Price dynamics in public and private housing markets in Singapore. Journal of Housing Economics, 15(4), 305–320. https://doi.org/10.1016/j.jhe.2006.09.006
- Sogiakas, V., & Karathanassis, G. (2015). Informational efficiency and spurious spillover effects between spot and derivatives markets. Global Finance Journal, 27, 46–72. https://doi.org/10.1016/j.gfj.2015.04.004
- Sousa, R. M. (2010). Housing wealth, financial wealth, money demand and policy rule: Evidence from the euro area. The North American Journal of Economics and Finance, 21(1), 88–105. https://doi.org/10.1016/j.najef.2009.11.006
- Stein, J. C. (1995). Prices and trading volume in the housing market: A model with down-payment effects. The Quarterly Journal of Economics, 110(2), 379–406. https://doi.org/10.2307/2118444
- Tanaś, J., Trojanek, M., & Trojanek, R. (2019). Seniors’ revealed preferences in the housing market in Poznań. Economics & Sociology, 12(1), 353–365. https://doi.org/10.14254/2071-789X.2019/12-1/22
- Thompson, D. (2012, February). Adulthood, delayed: What has the recession done to Millennials? The Atlantic. https://www.theatlantic.com/business/archive/2012/02/adulthood-delayed-what-has-the-recession-done-to-millennials/252913/
- Tomal, M. (2020). Spillovers across house price convergence clubs: Evidence from the Polish housing market. Real Estate Management and Valuation, 28(2), 13–20. https://doi.org/10.1515/remav-2020-0012
- Tsai, I-C., & C.-C. Lin, (2019). Variations and influences of connectedness among US housing markets. International Real Estate Review. International Real Estate Review, 22(1), 27–58. https://doi.org/10.53383/100274
- Wang, L., Xu, Y., & Salem, S. (2021). Theoretical and experimental evidence on stock market volatilities: A two-phase flow model. Economic Research-Ekonomska Istraživanja, in Press. https://doi.org/10.1080/1331677X.2021.1874459