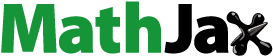
Abstract
As COVID-19 has hit the whole world hard, finding a vaccine that alleviates its effects has been one of the most anticipated events in 2020. This work studies the impact of the two main events in 2020 on companies’ stock exchange activities: announcing COVID-19 as a world pandemic and announcing the first coronavirus vaccine. This study was carried out by an event study methodology using Nasdaq-100 data. Ordinary Least Squares (OLS) and quantile (Q) regression were used to obtain the returns expected from shares and to assess if there were any significant differences in the reactions of the analysed sectors on the market in each event window. The obtained empirical results show different share performances by sectors. Specifically, only those shares from the technology sector positively and significantly reacted to the first announcement. However, the opposite can be stated of the second event insofar as confidence in financial markets recovered to a greater extent in the sectors hardest hit by the first announcement. These conclusions are meaningful for companies, investors and governments to make better decisions or to adopt new policies.
1. Introduction
In December 2019, a new coronavirus (COVID-19) appeared in Wuhan (China) and swiftly spread worldwide. Its spread and seriousness were such that the World Health Organization (WHO) announced the global COVID-19 pandemic on 11 March 2020, which is the first pandemic caused by coronavirus (Shaikh, Citation2021).
This led to a healthcare, economic and financial crisis because this pandemic strongly affects the world’s economic activity. This situation is caused mainly by the measures taken to slow down the pandemic, such as being confined at home or lots of mobility restrictions for companies and citizens alike. All this has reduced production and consumption, many businesses have closed and countries’ poverty has increased (Leduc & Liu, Citation2020). The negative impact of this healthcare crisis on the economy is much stronger than that caused by the global financial crisis (GFC) of 2007–2009 (Georgieva, Citation2020; Shehzad, Xiaoxing, Arif et al., Citation2020), especially on European and United States (US) markets (Espinosa-Méndez & Arias, Citation2021; Shehzad, Xiaoxing, & Kazouz, Citation2020). Thus, as the GFC extended worldwide due to global economic and financial markets, globalisation also enhanced COVID-19, which became a pandemic through international travelling. Indeed the impact of today’s COVID-19 crisis could have been avoided (Woolhouse et al., Citation2016). Many economists forecast that the effects of the economic crisis that has emerged as a result of this pandemic and has been classified as the worst recession since World War II (World Bank, Citation2020), will last for years. Nonetheless, society has been able to cushion the impact of this pandemic by means of digitisation, particularly in sectors like education, retail sales, or, in general terms, teleworking (De Lucas Ancillo et al., Citation2021), which could have a positive effect on the technology industry (TECH).
Ever since the pandemic was announced in March 2020, governments have been mainly concerned about finding a vaccine to enhance the world’s economic recovery. By means of their alliance, on 9 November 2020, the pharmaceutical corporation Pfizer and the German firm BioNTech announced that the efficiency of their vaccine trials against COVID-19 exceeded 90%. This was the announcement of the first effective COVID-19 vaccine, which led to much optimism on financial markets in particular, and in society in general (Ros, Citation2020).
Therefore, we can state that the two events that have marked both society and the economy in 2020 are COVID-19 being announced as a world pandemic and the first COVID-19 vaccine being communicated.
The main objective of this research is to study the effects and duration that these two diverse and opposing events have had on the financial markets and to particularly analyse the impact of one of the main sectors involved in mitigating the negative effects of the pandemic: the TECH sector. This hypothesis is justified by the importance that the TECH sector has acquired (Alcaide et al., Citation2019; Tušek et al., Citation2021), especially in 2020 and owing to the digital transformation of both citizens and companies, which they have had to implement and improve to be able to continue their work as a result of the restrictions brought about by the pandemic. Its secondary objective is to also analyse the effect of the two aforementioned events on other sectors by a cross-sectional study.
This conducted research was about Nasdaq-100 because it is the market index that has most revalued in 2020 by almost 38% (Expansión, Citation2020), which is much higher than other indexes: S&P500 by 13.36%, Nikkei by 13.29%, Shanghai by 13.09%, Dow Jones by 4.50% or DAX by 1.01%. No previous work (Espinosa-Méndez & Arias, Citation2021; Heyden & Heyden, Citation2021; Liu et al., Citation2021; Qureshi, Citation2021) has studied the effects of COVID-19 on the stock returns of Nasdaq-100 firms.
The main contribution of the present study was to measure how the two most relevant events related to the world COVID-19 pandemic impacted Nasdaq-100 stock returns and their sectorial differences. These two events were the pandemic and the first vaccine announcements. We tested the financial market by means of Nasdaq-100, the most important index in the world, along with S&P500, in negotiation volume terms, which is the reference asset to measure how world economies perform. Moreover, the effect of the TECH sector is better covered by this index because it comprises mostly technology-based firms. Hence this work helps to compare the returns performance of the TECH sector to other works, and for company directors and investors to make future investment-financing decisions.
It is worth stressing that no research has yet analysed the effect of COVID-19 vaccines availability on stock markets, particularly the effect of the first COVID-19 vaccine being announced, which is the second main contribution of the present work. Nor has the literature analysed other possible positive events that have appeared with this pandemic, which confers the present work added value because it contributes to improving knowledge on the TECH sector performance, about external shocks, and also on the possible stimuli of a shock such as the first vaccine. A third main contribution is that this measure of the impact on stock returns was taken using many time windows related to the two considered events, which quantified the effects of different economic-financial variables. Other previous studies have only taken into account a single time window or shorter windows. Another fourth contribution is that, with the event methodology followed to analyse this impact, the profitability of the stocks calculated with the market model was estimated by not only OLS regression, as the majority of former studies have done (Broadstock et al., Citation2021; Heyden & Heyden, Citation2021; Liu et al., Citation2021; Schell et al., Citation2020), but also by quantile (Q) regression, which allows the non-normal distributions problem to be solved.
The article is structured as follows: Section 2 presents background evidence. Section 3 explains the data and methodology. Section 4 offers empirical results and discussion. Section 5 ends by concluding.
2. Theoretical background
The effects of pandemics on financial markets are not very well covered by the literature (Goodell, Citation2020). However, given the extremely strong impact that today’s coronavirus pandemic is having on the world’s economies, the literature on this theme is quickly growing (Bouri et al., Citation2021; Espinosa-Méndez & Arias, Citation2021; Liu et al., Citation2021; Qureshi, Citation2021; Ramelli & Wagner, Citation2020; Umar et al., Citation2021), although it is still in its early days.
Some recent works (Broadstock et al., Citation2021; Heyden & Heyden, Citation2021; Liu et al., Citation2021; Schell et al., Citation2020) applied the same methodology as that herein used to analyse the COVID-19 pandemic; that is, the event study methodology. However, as set out in the next section, all the above studies observed some reactions from different perspectives that the pandemic had on stock markets. It is worth stressing that they all focussed on studying a negative event, such as the COVID announcement or the first death caused by this pandemic. However, the literature has not yet analysed the impact that other types of positive events, which are also related to the pandemic, may have on financial markets.
We observed that the literature scarcely contains research works about the impact of this pandemic on the TECH sector, more specifically on its stock returns. Only four works have included this sector. Alam et al. (Citation2021) investigated the indices of eight sectors of the Australian stock market. They analysed their initial volatility and sector performance. The results of their study showed that on the day the pandemic was announced, and generally on the following 10 days, the performance of the indices for food, pharmaceuticals and healthcare was very significant and positive, while that of TECH industries and transportation remained quite stable. Narayan et al. (Citation2022) also analysed the impact of COVID-19 on Australia’s stock return, but their conclusions deferred from a previous work. According to these authors, the sectors that benefitted were health, TECH and consumer staples, while other sectors were either negatively impacted or not affected at all. He et al. (Citation2020) studied how markets reacted to COVID-19 by centring on Chinese industries and the Shangai and Shenzhen A-share markets. Their findings indicated that the hardest-hit industries were transportation, mining, electricity & heating and environment industries. Conversely, the manufacturing, education, TECH, and healthcare industries benefitted the most from the pandemic. Finally, Sherif (Citation2020) performed panel testing to investigate the impact of COVID-19 on Shariah-compliant UK Dow Jones market index performance to observe how stock returns performed in the economy. These authors also carried out a sectorial analysis and concluded that the TECH sector better performed than the market, while consumer discretionary sector performance had a negative impact.
3. Data and methodology
3.1. Data
From the Finance Yahoo database, the daily data of stock prices were collected at the closing price for the 100 firms listed on Nasdaq-100 during the period from 19 May 2019 to 1 December 2020. Our final sample comprised 3950 observations.
We also collected data from each company from 2019 Finance Yahoo about firm size, market-to-book ratio and profitability (Heyden & Heyden, Citation2021; Ramelli & Wagner, Citation2020). These data were processed to include the following variables in our study: total assets (ASSET) indicate firm size; the price-earning ratio (PER) is the quotient between the price and the expected earnings from shares; net results (NR); the total value in books (BV); return on equity (ROE) or the quotient between the net result and BV; return on assets (ROA) or the EBITDA divided by total assets; the leverage (LV) measured as total assets divided by total liabilities because it has been demonstrated to affect firms’ performance during economic downturns (Opler & Titman, Citation1994); a stock´s dividend yield (DY) or the quotient between the shared dividend and the mean share price, which was included to measure how it affected the cross-section of stock returns (Ang et al., Citation2006).
The companies included in our sample were grouped into the following sectors to conduct this research: TECH sector, Healthcare (HC) sector, Consumer cyclical (CC) sector, namely the most numerous ones in Nasdaq-100 after the TECH sector, and Other sectors (OS) (Industrial, Communication, Consumer no cyclical, Financial services and Utilities).
3.2. Methodology
A descriptive data analysis was firstly carried out. Then this study followed the event study methodology (Sorescu et al., Citation2017) to measure how the stock market price reacted when both the events that marked 2020 worldwide were announced, and how time influenced financial market reactions. Recent studies have also followed this methodology to observe how stock markets have reacted to different COVID-19-related events. For instance, Broadstock et al. (Citation2021) examine the role of environmental, social and corporate governance (ESG) performance during the market-wide financial crisis, triggered in response to the COVID-19 global pandemic; Liu et al. (Citation2021) investigate the effect of firm-level operating flexibility on stock market performance during the COVID-19 outbreak in China; Schell et al. (Citation2020) study the differences in stock market reactions during the public health risk emergency of announcements of international concern; Heyden and Heyden (Citation2021) study the short-term market reactions of US and European stocks at the beginning of the COVID-19 pandemic by means of the announcements of the first case, the first death, tax policy and monetary policy measures.
For our study objective, the date of the first event (when the world pandemic was announced) was 11 March 2020 and that of the second event (when the vaccine was announced) was 9 November 2020. Following the approach of MacKinlay (Citation1997), we selected the windows estimation of the last 200 trading days, but excluded the 14 days preceding the events to obtain the expected values of returns in event windows. This means that the estimated windows for the first day event went from 10 May 2019 to 25 February 2020, and our second day event went from 10 January 2020 to 23 October 2020. For our first study, we defined seven different event windows to establish the time period during which the analysed events had a stronger influence on each sector. So if the event day was t = 0, the seven event windows for the study on the pandemic announcement would be t Є {−1,1}, t Є {−1,5}, t Є {−1,10}, t Є {−1,15}, t Є {−1,20}, t Є {−1,25}, t Є {−1,30}. The event windows for the study about announcing the first vaccine would be t Є {−1,1}, t Є {−1,2}, t Є {−1,5}, t Є {−1,10}, t Є {−1,15}. Five event windows and shorter time periods were defined for the second event to collect only the effect of the first vaccine and to reduce the effect of subsequently announced vaccines.
For each firm, we firstly calculated the daily stock returns as:
(1)
(1)
where:
represents the daily profitability of the shares of a firm i on day t belonging to the estimation window;
represents prices of shares of a firm i on day t belonging to the estimation window.
Then we calculated the abnormal returns (ARit) for each firm i on day t as:
(2)
(2)
Basically, three models exist to calculate ARit: the average adjusted return rate model (Klein & Rosenfeld, Citation1987); the market index adjusted return rate model (Huang & Li, Citation2018); the market model (Brenner, Citation1979). We employed this third model because it is the most widely used one. Thus the market model is:
(3)
(3)
where:
was obtained by OLS regression (Heyden & Heyden, Citation2021; Liu et al., Citation2021; Schell et al., Citation2020) following the expected returns model.
is the market return of the Nasdaq-100 index on day t belonging to the same period.
Thirdly, we calculated the cumulative abnormal returns (CARie) for each firm i and for each event window as follows:
(4)
(4)
where t1 and t2 represent the start and end of the event window, respectively.
With these values, we calculated the average CARe for each event window in both the main analysed events (the pandemic and the first vaccine announcements) to perform a preliminary analysis of the short-term effects of each event on Nasdaq-100 sectors.
Finally, to assess our main objective related to the existence of significant differences in the reactions of the TECH sector versus other sectors, we combined two methods: OLS and Q regression (Azimli, Citation2020; Maiti, Citation2021). We firstly used OLS regression (Heyden & Heyden, Citation2021; Liu et al., Citation2021) for each event window with the following expression:
(5)
(5)
Like other studies (Choudhry, Citation2000; Kiymaz & Berument, Citation2003), dummy variables were employed to analyse the impact of daily anomalies on stock returns. We resorted to the TECH dummy variable to learn the impact of both these events on the TECH sector. This dummy variable took the value of 1 if a Nasdaq-100 firm belonged to this sector, and 0 otherwise. The other variables that appear in expression Equation(5)(5)
(5) are explained above and act as control variables.
In order to analyse our secondary objective, the impact of both events on the HC sector, the CC sector and OS sector, three new dummy variables were included. They took a value of 1 if the firm came from the corresponding sector, and 0 otherwise. For instance, dummy variable OS had a value of 0 if the company belonged to the TECH, HC or CC sectors, and 1 otherwise; that is, the effect of the other sectors with very little representation was grouped to reach relevant conclusions. The same expression Equation(5)(5)
(5) was employed by replacing TECH with dummy variables HC, CC and OS related to the other aforementioned sectors.
After applying the OLS regressions of expressions Equation(3)(3)
(3) and Equation(5)
(5)
(5) , we checked to see if the normal distribution hypothesis
and the residuals of each regression, were met to ratify the models’ validity. To do so, we applied the Kolmogorov–Smirnov test (Lilliefors, Citation1967; Pratt & Gibbons, Citation1981), the Normal Probability Plot and the Detrended Normal Plot.
If normality was not met, Q regression (Koenker & Bassett, Citation1978; Maiti, Citation2021) was used, which does not assume any previous distribution for and the residuals. This was done specifically for the 25%, 50% and 75% quantiles to improve and complete the OLS regression results.
For the mathematical expression of the quantile
th of CARe in relation to the explanatory variables was:
(6)
(6)
In the quantile regression, the coefficients of the explanatory variables were estimated using linear programming by minimising the sum of the absolute errors instead of summing quadric errors, which is done with OLS regression. Q regression allows the degree of each quantile’s dependence to be estimated. If the coefficients for each quantile are equal, the dependence structure is constant (Azimli, Citation2020). However, coefficients might be high in tails and low in intermediate quantiles (U-shaped symmetric dependence), or vice versa (inverted U-symmetric dependence). Another possibility is that the dependence structure is asymmetric: left-tailed when coefficients increase as quantiles increase; right-tailed when coefficients lower.
4. Results and discussion
4.1. Descriptive analysis
A descriptive analysis of each sector was performed (see ). There were 46 analysed companies from the TECH sector, plus 17 from HC, 19 from CC and 18 from other sectors. This evidenced that the companies from OS were poorly represented if not grouped as one single sector.
Table 1. Descriptive statistics of the control variables per sector.
Regarding the PER, we found that TECH, HC and OS obtained similar values, which came close to 30, while the PER of the CC sector was higher and came close to 50. The dispersion of the PER in the TECH and CC sectors stood out. The sectorial values for DY ranged between 0% for the HC sector and 1.39% for the OS sector. Of sectors’ returns, the CC sector’s high financial profitability stood out, and dispersion was also high. Once again the CC sector obtained the highest values for employing debt to finance assets.
4.2. Preliminary analysis
offers the descriptive statistics of the CARe values for each sector for every event window in relation to the Covid-19 pandemic (Panel A) versus the first vaccine (Panel B), specifically the average, median, standard deviation, the 25% and 75% quartiles, and the Kolmogorov–Smirnov test and its p-value.
Table 2. Descriptive statistics of sectorial cumulative abnormal returns (CARe) (%) for the event windows to the COVID-19 pandemic announcement (Panel A) versus the first vaccine (Panel B).
Our results revealed that the only sector with positive average short-term CARe values after the pandemic was announced was the TECH, with a maximum of 3.52% after 10 trading days, after which time its CARe started to lower and became moderately negative after 25 trading days. Conversely, these values for the HC and OS sectors started to obtain positive average CARe values after 15 and 20 trading days, respectively, which became especially higher for the HC sector with 9.29% at 30 trading days. Conversely, CC was the hardest hit sector throughout the study period because its worse average CARe value was obtained at 5 trading days with −17.40%. From this time, its performance slightly improved, but values were still negative. Practically the opposite occurred after the first vaccine was announced. CC was the sector with the best average CARe values in all the event windows, especially at 10 trading days when it obtained its maximum (10.08%). The same can be stated of OS, which also obtained its maximum at 10 trading days with 4.66%. However, this announcement had no strong impact for the TECH and HC sectors whose average short-term CARe values went up and down, and were practically irrelevant.
According to the Kolmogorov–Smirnov test, CARe followed normal distribution in all the event windows, except in event windows {−1,20}, {−1,25} and {−1,30} for panel A (the Covid-19 pandemic announcement) and in event window {−1,1} for panel B (the first vaccine announcement). Consequently, Q regression was carried out in these event windows.
4.3. Empirical evidence
In order to extend and empirically compare the results of our preliminary analysis, shows our main objective results and includes the reactions of TECH firms’ CAR as opposed to the other sectors in relation to the WHO’s official announcement of the world COVID-19 virus pandemic. Only the significant variables appeared in the model in each window, except for the variable TECH, which was included in all the models because this was our objective.
Table 3. OLS and quantile (Q) regression analysis of the cumulative abnormal returns (CARe) to the COVID-19 pandemic announcement: TECH sector versus other sectors.
reveals that TECH firms’ CARe were positively and significantly impacted in the event windows from t Є {−1,1} to t Є {−1,20} as opposed to the other sectors represented by the constant, which was negative in the first three windows and was only significant in the first window. The strongest impact by the highest coefficient (7.490) of TECH was t Є {−1,10}; that is, on the 10 trading days of the official pandemic announcement. In t Є {−1,10}, the highest R-squared value was 0.368, which ratified the explanation level of the model obtained in this window. In t Є {−1,20}, Q regressions 25% and 50% still had a positive effect on the TECH sector, but the effect in the 75% quantile was negative. This demonstrates a right-tailed dependence structure. At 25 trading days, the effect that this event had was no longer significant on TECH companies, and Nasdaq-100 firms considerably recovered as the increased constant in the last two windows indicated. Moreover, the higher expected profits made by Nasdq-100 firms, which were measured by the PER, helped higher CARe to be reached in t Є {−1,10}, but not in t Є {−1,20}. The LN ASSET variable appeared in almost all the windows with a negative sign, which indicates that the negative impact of this announcement was not as marked on small-sized Nasdaq-100 firms as on large-sized ones. The Q regression for windows {−1,25} and {−1,30} showed a dependence structure for the right-tailed LN ASSET. Likewise, the positive coefficient of the LN BV variable in several windows explained how the companies with a higher BV value obtained higher CARe. In windows {−1,25} and{−1,30}, Q regression presented a left-tailed dependence structure for ROA in the former window, and a right-tailed one in the latter window for both ROA and ROE.
includes the reactions of TECH firms’ CARe to the official first COVID-19 vaccine announcement made by companies Pfizer and BioNTech. In all the analysed windows, this event had a positive and significant impact on Nasdaq-100 firms in general, as deduced from the constant, but a negative and significant impact on the TECH sector, conversely to what happened when the pandemic was announced (). In t Є {−1, 5}, the strongest impact took place (−4.529); that i, on 5 trading days from the time the first vaccine was announced, as opposed to the 10 days (−3.788) after announcing the pandemic. However, differences were very small. The negative effect of TECH at 15 days was compensated in relation to the constant. The only significant control variable to appear in the first three models was DY, which took a positive sign. This means that the Nasdaq-100 firms with more DYs in 2019 obtained higher CARe on the first 5 days after the event. This is a logical result because this event raised hopes for more profits being made for both capital gains and dividends. In t Є {−1,1}, Q regressions ratified the results obtained in the OLS regression. The announcement of the vaccine negatively impacted TECH firms compared to others and had an asymmetric right-tailed structure. The DY for window {−1,1} followed an inverted-U symmetric distribution.
Table 4. OLS and quantile (Q) regression analysis of the cumulative abnormal returns (CARe) to the first vaccine announcement: TECH sector versus other sectors.
4.4. Cross-sectional analysis
The sectorial analysis results appear in and . includes the reactions of non-TECH firms’ CARe, HC, CC or OS to the WHO’s official publication of the world COVID-19 pandemic. provides the reactions to the first COVID-19 vaccine announcement.
Table 5. OLS and quantile (Q) regression analysis of the cumulative abnormal returns (CARe) to the COVID-19 pandemic announcement of the main non-TECH sectors versus the TECH sector.
Table 6. OLS and quantile (Q) regression analysis of the cumulative abnormal returns (CARe) to the first vaccine announcement of the main non-TECH sectors versus the TECH sector.
shows that the sector with the strongest significant negative impact on its CARe when the pandemic was announced, and in all the event windows, was the CC sector, especially on the 5 and 15 trading days after being announced. Then 20, 25 and 30 days after the event, Q regressions ratified the negative effect of OLS regressions, but the dependence structure in each window differed. This is a logical result because the CC sector includes tourist companies, retail sales, etc., which were the hardest hit by limited mobility. The HC sector was also affected by the pandemic being announced in t Є {−1,1} and t Є {−1,10}, as was the OS sector in t Є {−1,1}, t Є {−1,10} and t Є {−1,15}. In both these sectors, this significant impact was negative, and the highest R-squared values were obtained in these event windows with 0.295, 0.235 and 0.377, respectively. In this analysis, the control variables had a more diluted effect than in the study performed with the TECH companies. Nasdaq-100 firms considerably recovered for the 30 trading days series as the model’s constant indicates, although this effect was not equal for the HC and CC sectors. Besides, LN PER only influenced windows {−1,1}, {−1,10} and {−1,20}, while LN NR and ROA impacted window {−1,15} and {−1,30}, respectively, and showed a right-tailed dependence structure.
indicates how the only sector whose CARe were very significantly and positively impacted when the first vaccine was announced, and in all the event windows, was the CC sector. This was, in fact, the hardest hit sector when the pandemic was announced. Neither the HC and nor the OS sector was affected. This situation with the former is perhaps because it comprises different industries, which are not all pharmaceutical, and the pharmaceutical industries in this sector have different drugs to treat other diseases. Once again, as shows, the only control variable that was significant was DY, which took a positive sign. This implies that the main effect of announcing the first vaccine affected those companies whose shareholders had the most expectations. The results per quantile in window {−1,1} corroborated that the firms from sector CC positively impacted CARe and had an inverted-U symmetric structure, and the same could be stated of DY.
5. Conclusion
Despite the major economic recession that most countries and sectors are undergoing as a result of today’s healthcare crisis and restrictions, this work empirically analysed the short-term effects of stock prices with the announcements of COVID-19 as a world pandemic and the first efficient vaccine for this virus. To do so, we followed an event study methodology centred on Nasdaq-100 companies, and used the market model to estimate the profitabilities made with stocks by means of OLS and Q regressions. This paper evidences that not all the economic sectors comprising Nasdaq-100 have reacted in the same way. Our preliminary results informed us that in the short term, especially up to 20 trading days after the pandemic was announced, the TECH sector was the only sector to benefit as regards its average CARe. However, the most benefitted sector was HC when we considered a slightly longer study period (as of 25 trading days). No relevant impact on the average values of both these sectors when the first vaccine was announced was found. The weak impact on the average CARs for the HC sector could be because the companies in the Nasdaq-100 sector perform vastly varied activities and not only vaccination research, and the company Pfizer is not featured among them. This preliminary study indicated that the hardest sector hit by the first announcement was CC, which was also the sector to get the most benefit from the announcement of the first vaccine. The regression analysis allowed us to provide more results in detail, and specifically revealed that the TECH sector was positively impacted on the first few days after announcing the pandemic compared to other sectors. This effect was particularly strong on the 10 trading days after the WHO made its official announcement. The remaining sectors were negatively impacted, mainly the CC sector for 5 and 15 trading days. Yet the impact on the HC and OS sectors was not so strong. Our results also revealed that this announcement influenced larger companies more than smaller ones, while those companies with higher BV had higher CARe.
Our results about the performance of stock returns in the TECH sector coincide with the literature (He et al., Citation2020; Narayan et al., Citation2022; Sherif, Citation2020), except for the work of Alam et al. (Citation2021), for whom this sector remained relatively stable. Conversely, while the short-term performance of the HC sector was better than the market for all the other authors (Alam et al., Citation2021; He et al., Citation2020; Narayan et al., Citation2022), our results revealed slightly worse performance. We ought to remember that the HC sector is made up of a wide range of firms as far as their economic activity and size are concerned. Our results coincide with those reported by Sherif (Citation2020) insofar as the CC sector was the hardest hit one. This seems logical because it includes industries like tourism or retail, which are among the hardest-hit by the restrictions imposed by each country. Our work grouped the other sectors as a single variable given their insufficient representation to provide significant evidence. However, the reviewed literature reveals more divergences.
The first vaccine announcement had the opposite effect because it raised Nasdaq-100’s expectations, especially those companies with higher DYs. The hardest-hit sector by the pandemic (CC) obtained higher CARs in all the event windows, whereas the TECH sector was negatively impacted.
Quantiles regression helped to better analyse the abnormal returns in event windows whenever normality requirements were not met.
The present research work suggests that the diversification portfolio should be considered for external shocks because each sector is differently impacted and, likewise, each has distinct adaptation capacities. Today’s pandemic has driven the TECH sector towards digitisation, whereas others like CC have been seriously threatened. The present work also contributes to the scarce literature about external stocks stimuli because the analysis done of the first announced vaccine evidenced that opportunities or new threats were also created during the crisis that would differently affect each sector. Therefore, studying them would help us to anticipate them and benefit from these stimuli.
These conclusions are meaningful for companies, investors and governments to make better decisions or to adopt new policies because we would be more able to know which sectors would be better or worse able to adapt to similar shocks.
Disclosure statement
No potential conflict of interest was reported by the authors.
References
- Alam, M. M., Wei, H., & Wahid, A. N. M. (2021). COVID-19 outbreak and sectoral performance of the Australian stock market: An event study analysis. Australian Economic Papers, 60(3), 482–495. https://doi.org/10.1111/1467-8454.12215
- Alcaide, M. Á., De la Poza, E., & Guadalajara, N. (2019). Assessing the sustainability of high-value brands in the IT sector. Sustainability, 11(6), 1598. https://doi.org/10.3390/su11061598
- Ang, A., Hodrick, R. J., Xing, Y., & Zhang, X. (2006). The cross-section of volatility and expected returns. The Journal of Finance, 61(1), 259–299. https://doi.org/10.1111/j.1540-6261.2006.00836.x
- Azimli, A. (2020). The impact of COVID-19 on the degree of dependence and structure of risk-return relationship: A quantile regression approach. Finance Research Letters, 36, 101648. https://doi.org/10.1016/j.frl.2020.101648
- Bouri, E., Naeem, M. A., Nor, S. M., Mbarki, I., & Saeed, T. (2021). Government responses to COVID-19 and industry stock returns. Economic Research-Ekonomska Istraživanja, 1–24. https://doi.org/10.1080/1331677X.2021.1929374
- Brenner, M. (1979). The sensitivity of the efficient market hypothesis to alternative specifications of the market model. The Journal of Finance, 34(4), 915–929. https://doi.org/10.1111/j.1540-6261.1979.tb03444.x
- Broadstock, D. C., Chan, K., Cheng, L. T. W., & Wang, X. (2021). The role of ESG performance during times of financial crisis: Evidence from COVID-19 in China. Finance Research Letters, 38, 101716. https://doi.org/10.1016/j.frl.2020.101716
- Choudhry, T. (2000). Day of the week effect in emerging Asian stock markets: Evidence from the GARCH model. Applied Financial Economics, 10(3), 235–242. https://doi.org/10.1080/096031000331653
- De Lucas Ancillo, A., Del Val Núñez, M. T., & Gavrila, S. G. (2021). Workplace change within the COVID-19 context: A grounded theory approach. Economic Research-Ekonomska Istraživanja, 34(1), 2297–2316. https://doi.org/10.1080/1331677X.2020.1862689
- Espinosa-Méndez, C., & Arias, J. (2021). COVID-19 effect on herding behaviour in European capital markets. Finance Research Letters, 38, 101787. https://doi.org/10.1016/j.frl.2020.101787
- Expansión. (2020). Cotizaciones índices bolsas de los mercados de valores. Retrieved December 1, 2020, from https://datosmacro.expansion.com/bolsa/
- Georgieva, K. (2020). IMF managing director Kristalina Georgieva's statement following an G20 ministerial call on the coronavirus emergency. Press Release 20/98. International Monetary Fund. Retrieved March 2, 2021, from https://www.imf.org/en/News/Articles/2020/03/23/pr2098-imf-managing-director-statement-following-a-g20-ministerial-call-on-the-coronavirus-emergency
- Goodell, J. W. (2020). COVID-19 and finance: Agendas for future research. Finance Research Letters, 35, 101512. https://doi.org/10.1016/j.frl.2020.101512
- He, P., Sun, Y., Zhang, Y., & Li, T. (2020). COVID–19’s impact on stock prices across different sectors – An event study based on the Chinese stock market. Emerging Markets Finance and Trade, 56(10), 2198–2212. https://doi.org/10.1080/1540496X.2020.1785865
- Heyden, K. J., & Heyden, T. (2021). Market reactions to the arrival and containment of COVID-19: An event study. Finance Research Letters, 38, 101745. https://doi.org/10.1016/j.frl.2020.101745
- Huang, H., & Li, M. (2018). An overview of event study methodology. Statistics & Decision, 34(13), 66–71.
- Kiymaz, H., & Berument, H. (2003). The day of the week effect on stock market volatility and volume: International evidence. Review of Financial Economics, 12(4), 363–380. https://doi.org/10.1016/S1058-3300(03)00038-7
- Klein, A., & Rosenfeld, J. (1987). The influence of market conditions on event-study residuals. The Journal of Financial and Quantitative Analysis, 22(3), 345–351. https://doi.org/10.2307/2330968
- Koenker, R., & Bassett, G. (1978). Regression quantiles. Econometrica, 46(1), 33–50. https://doi.org/10.2307/1913643
- Leduc, S., & Liu, Z. (2020). The uncertainty channel of the coronavirus. FRBSF Economic Letters, 2020(07), 1–05. Research from Federal Reserve Bank of San Francisco.
- Lilliefors, H. W. (1967). On the Kolmogorov-Smirnov test for normality with mean and variance unknown. Journal of the American Statistical Association, 62(318), 399–402. https://doi.org/10.2307/2283970
- Liu, H., Yi, X., & Yin, L. (2021). The impact of operating flexibility on firms' performance during the COVID-19 outbreak: Evidence from China. Finance Research Letters, 38, 101808. https://doi.org/10.1016/j.frl.2020.101808
- MacKinlay, A. C. (1997). Event studies in economics and finance. Journal of Economic Literature, 35(1), 13–39. https://www.jstor.org/stable/2729691
- Maiti, M. (2021). Quantile regression, asset pricing and investment decision. IIMB Management Review, 33(1), 28–37. https://doi.org/10.1016/j.iimb.2021.03.005
- Narayan, P. K., Gong, Q., & Ali Ahmed, H. J. (2022). Is there a pattern in how COVID-19 has affected Australia's stock returns? Applied Economics Letters, 29(3), 179–182. https://doi.org/10.1080/13504851.2020.1861190
- Opler, T. C., & Titman, S. (1994). Financial distress and corporate performance. The Journal of Finance, 49(3), 1015–1040. https://doi.org/10.1111/j.1540-6261.1994.tb00086.x
- Pratt, J. W., & Gibbons, J. D. (1981). Kolmogorov–Smirnov two-sample tests. In Concepts of nonparametric theory. Springer series in statistics (pp. 318–344). New York, NY: Springer. https://doi.org/10.1007/978-1-4612-5931-2_7
- Qureshi, F. (2021). COVID-19 pandemic, economic indicators and sectoral returns: Evidence from US and China. Economic Research-Ekonomska Istraživanja Journal, 1–31. https://doi.org/10.1080/1331677X.2021.1934508
- Ramelli, S., & Wagner, A. F. (2020). Feverish stock price reactions to COVID-19 [Swiss Finance Institute Research Paper, No. 20-12]. https://doi.org/10.2139/ssrn.3550274
- Ros, L. (2020). ¿Por qué han subido las bolsas tras el anuncio de vacunas efectivas contra la COVID-19? La Vanguardia. Retrieved November 23, 2020, fromhttps://www.lavanguardia.com/vida/junior-report/20201118/49525954637/por-que-han-subido-las-bolsas-tras-el-anuncio-de-vacunas-efectivas-contra-la-covid-19.html
- Schell, D., Wang, M., & Huynh, T. L. D. (2020). This time is indeed different: A study on global market reactions to public health crisis. Journal of Behavioral and Experimental Finance, 27, 100349. https://doi.org/10.1016/j.jbef.2020.100349
- Shaikh, I. (2021). Impact of COVID-19 pandemic disease outbreak on the global equity markets. Economic Research-Ekonomska Istraživanja, 34(1), 2317–2336. https://doi.org/10.1080/1331677X.2020.1863245
- Shehzad, K., Xiaoxing, L., Arif, M., Rehman, K. U., & Ilyas, M. (2020). Investigating the psychology of financial markets during COVID-19 era: A case study of the US and European markets. Frontiers in Psychology, 11, 1924. https://doi.org/10.3389/fpsyg.2020.01924
- Shehzad, K., Xiaoxing, L., & Kazouz, H. (2020). COVID-19’s disasters are perilous than global financial crisis: A rumor or fact? Finance Research Letters, 36, 101669. https://doi.org/10.1016/j.frl.2020.101669
- Sherif, M. (2020). The impact of Coronavirus (COVID-19) outbreak on faith-based investments: An original analysis. Journal of Behavioral and Experimental Finance, 28, 100403. https://doi.org/10.1016/j.jbef.2020.100403
- Sorescu, A., Warren, N. L., & Ertekin, L. (2017). Event study methodology in the marketing literature: An overview. Journal of the Academy of Marketing Science, 45(2), 186–207. https://doi.org/10.1007/s11747-017-0516-y
- Tušek, B., Ježovita, A., & Halar, P. (2021). The importance and differences of analytical procedures' application for auditing blockchain technology between external and internal auditors in Croatia. Economic Research-Ekonomska Istraživanja, 34(1), 1385–1408. https://doi.org/10.1080/1331677X.2020.1828129
- Umar, M., Xu, Y., & Mirza, S. S. (2021). The impact of Covid-19 on Gig economy. Economic Research-Ekonomska Istraživanja, 34(1), 2284–2296. https://doi.org/10.1080/1331677X.2020.1862688
- Woolhouse, M. E. J., Brierley, L., McCaffery, C., & Lycett, S. (2016). Assessing the epidemic potential of RNA and DNA viruses. Emerging Infectious Diseases, 22(12), 2037–2044. https://doi.org/10.3201/eid2212.160123
- World Bank. (2020). Global economic prospects, June 2020. World Bank. https://doi.org/10.1596/978-1-4648-1553-9