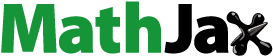
Abstract
Using efficient and cleaner energy is environmentally friendly and is vital for combating the negative effect of emissions on the environment. Focusing on both developed and developing nations, it is important to report their environmental conditions while targeting economic and energy-related factors. In this regard, the current study is an attempt to investigate the influence of energy efficiency (ENEF), financial inclusion (FIN), economic growth (GDP), environmental-related technological innovation (ERTI), and human capital index (HCI) on the carbon dioxide (CO2) emissions for the five selected nations of RCEP. The variables are found associated in terms of long-run cointegration relationships. The panel quantile regression estimator is utilized for empirical estimations, which provide highly significant estimates across the three selected quantiles (25th, 50th and 75th). The results report that FIN and GDP significantly aggravate environmental degradation by enhancing the CO2 emission level, among which the strongest CO2 emission growth is found in the second quantile. Besides, the ENEF, ERTI and HCI significantly reduce CO2 emission. Based on the empirical findings, this study provides practical implication focusing on the improvement of energy efficiency policies and revising financial inclusion policies in promotion of green investments.
1. Introduction
Among the most important factors, energy efficiency is considered an effective strategy to reduce energy consumption which is associated with emission and environmental degradation, as suggested by the United Nations Intergovernmental Panel on Climate Change (IPCC), the International Energy Agency (IEA) (Grant et al., Citation2016). Carbon dioxide (CO2) is believed to be the most dominant greenhouse gas emission delivered by human activities and is considered the main factor for climate change and environmental degradation. Industrialization is among those primary factors which increase the production of CO2 via electricity consumption and fossil fuel burning (Fernando & Hor, Citation2017). Every country across the globe targets higher economic growth, due to they are extensively focusing on industrialization. However, the remarkable economic development has rapidly increased demand for fossil fuel energy over the last four decades (Al-Mulali et al., Citation2015).
At the same time, the World Energy Outlook (Citation2017) special report reveals that it is expected that the energy demand will grow by 48% from 2017 to 2040. Both the developing and developed nations are held accountable for this tremendous increase in energy to attain and sustain economic growth. However, this rapid increase in energy demand raises global concerns about the adverse effects of fossil fuel energy consumption on environmental sustainability (Danish et al., Citation2019). Consequently, maintaining balanced economic growth while reducing climate change remains the primary challenge for every country in recent times. Therefore, countries across the globe focus on achieving environmental sustainability while maintaining economic growth. Thus, energy efficiency could be a vital policy used to minimize global warming and reduce CO2 emission.
Concerning economic growth, every country targets different factors to achieve and maintain higher economic development. Among others, financial inclusion is considered an essential indicator of economic growth and environmental performance. Financial inclusion is the access of individuals and businesses to various financial services and products fulfilling their financial requirements in a convenient, responsible, affordable, and sustainable way (World Bank, Citation2018). Financial services and products consist of transactions, savings, payments, credits, remittances flow, insurance, etc. Further, greater access to such financial products and services minimizes income inequality in the country and prominently leads to economic stability (Sahay et al., Citation2015). Financial inclusion is expected to encourage economic growth; thus, it could also be expected that a higher level of financial inclusivity influences the quality of the environment. The primary reason behind the influence of financial inclusion on the environment is that the higher level of financial inclusion supports the formation of higher fixed Capital, which increases energy demand and adversely affects the environment. Studies have empirically investigated the influence of financial inclusion for different regions and groups across the globe (Le et al., Citation2020; Renzhi & Baek, Citation2020). However, these studies provide contradictory findings in terms of environment-financial inclusion nexus.
Besides these environmental, economic, and financial indicators, environmental-related technological innovation and human Capital also play crucial roles in determining the environmental quality of the country or region. As per the World Economic Forum (Citation2017), many authors argued that CO2 emission is linked with each country's human capital. In contrast, the inconsistent outcomes concerning climate change could be recognized to the omission of human capital. Regardless of the role of human Capital in determining energy consumption and CO2 emissions, many studies empirically investigated the crucial role of human Capital and CO2 emissions (Huang et al., Citation2022a; Citation2022b; Sheraz et al., Citation2021). These studies argued that the high level of human development in a country directly impacts the quality of the environment. The high level of human development promotes environmental quality, technical research, and education, consequently increasing pro-environmental measures. Similarly, environmental-related technological innovation help advance technical research and promote the consumption of technologically advanced products which use less energy or environmentally friendly energy sources. Therefore, it will substantially enhance environmental quality by reducing the CO2 emission level in the atmosphere. Concerning environmental-related technologies, many studies empirically provide evidence that environmental-related innovations reduce CO2 emissions (Bai et al., Citation2020; Huang & Khan, Citation2022; Huang et al., Citation2021a, Citation2021b; Razzaq et al., Citation2021; Töbelmann & Wendler, Citation2020; Zhang et al., Citation2017). Hence, the role of environmental-related innovations cannot be ignored while investigating environmental quality in terms of CO2 emissions.
The current study focuses on the Regional Comprehensive Economic Partnership (RCEP) economies that jointly approved the region's free trade agreement. RCEP region, which accounts for 30% of the total GDP of the world (approximately 26 trillion US$) and 28% of the global trade, will reduce the tariffs gradually across the 15 economies in 20 years.Footnote1 However, Patricia RanaldFootnote2 claimed that RCEP focused only on trade gains while ignoring labor, human rights, and environmental sustainability. The current study analyzes five RCEP economies, including Australia, China, Indonesia, Japan, and South Korea. The selected five countries are playing the primary role in the energy trade of RCEP economies as they are amongst the leading energy importer and leading carbon-emitting countries in the world. Since these economies consist of developed and emerging countries, they could be more biased towards their environmental concerns.
Based on the above discussion, it is noticed that countries across the world face the challenge of rapid CO2 emission, which is a primary cause of environmental degradation and global warming. However, various international organizations claimed that energy efficiency could be used as a remedial measure to achieve a sustainable environment. Therefore, this study firstly investigates the influence of energy efficiency on the CO2 emission level of the five selected RCEP economies, which remained ignored in the empirical investigation. Besides, financial inclusion is assumed to be a factor of economic growth that facilitates the households and the industrial sectors via financial products, credits, and services, which influence the energy demand and contribute to economic growth. Thus, the second objective of this study is to investigate the impact of financial inclusion on the CO2 emission of the said countries. Economic growth is also one of the leading factors determining the income level and energy demand at both household and aggregate levels in developed and developing economies. Hence, the third objective of the paper is to examine the influence of economic growth on the CO2 emission level. Moreover, the developed economies are advanced in technologies, innovations, and human Capital and are prominent in reducing CO2 emissions. Therefore, the final objective of the current study is to analyze the impact of environmental-related technological innovation and human capital index on CO2 emission reduction. This study attempts to achieve the aforementioned objectives via advanced and reliable econometric techniques.
The rest of the study is organized as follows. Section 2 presents relevant Literature Review; Section 3 provides the Methodology and the specified Model(s) used in the study; Section 4 reveals the Results, their interpretation and discussion of the results obtained; lastly, Section 5 provides Conclusion and the practical policy implication based on the empirical results obtained.
2. Literature review
Energy efficiency is considered as one of the main remedies to environmental degradation abatement. In recent times, the use of traditional fossil fuel energy has caused damage to the environment in the form of CO2 and other greenhouse gas emissions. However, the main contributor to environmental degradation, climate change, and global warming is the emission of CO2. An extensive literature investigated the influence of energy efficiency on environmental degradation. Such studies include Akram et al. (Citation2020), Grant et al. (Citation2016), Fernando and Hor (Citation2017), Wang and Wei (Citation2014), Mahapatra and Irfan (Citation2021), etc., which all argued that energy efficiency promotes environmental sustainability by reducing CO2 emission level in the atmosphere.
Specifically, Akram et al. (Citation2020) studied the influence of energy efficiency and renewable energy on the CO2 emission for 66 developing countries over the 1990–2014 period. The study used panel ordinary least square and the fixed effect panel quantile regression. It concluded that the EKC hypothesis is valid for the panel, while energy efficiency significantly degrades carbon emission across all the panels but is strongest in the 90th quantile. Also, renewable energy is reported to have a significant negative association with CO2 emissions. Besides, GDP is positive related with environmental degradation by increasing the CO2 emission level in the atmosphere. Concerning the global and organizational factors on the effect of energy efficiency on carbon emissions, Grant et al. (Citation2016) investigated the age, size and location of the fossil fuel power plants and concluded that each of these factors significantly interacts with the energy efficiency and forms the environmentally harmful rebound effects. For the case of the Malaysian manufacturing industry, Fernando and Hor (Citation2017) argued that the Malaysian energy management practices are still at the beginning level. However, the empirical estimation on the survey data collected from ISO 14,000 certified firms reveals that energy efficiency and the energy audit are the critical factors for carbon emission abatement. Concerning the Chinese industrial energy efficiency and CO2 emission reduction, Wang and Wei (Citation2014) examined 30 major Chinese cities from 2006 to 2010. The findings reveal that economically developed cities have relatively higher energy efficiency than the less developed cities in China. The study argued that energy efficiency could be a powerful tool for hazardous CO2 emissions reduction. Besides, the authors witnessed the N-shaped EKC hypothesis in the Chinese cities, where the threshold level of per capita income is reported as 12,052 and 12,341 US$, which drastically reduces CO2 emissions.
Energy efficiency remains the primary focus in energy saving and CO2 emission reduction. Mahapatra and Irfan (Citation2021) examined the effects of energy efficiency on CO2 emission while comparing 28 developed and 34 developing economies covering the 1990–2017 period. The study used the autoregressive distributed lag (ARDL) approach and concluded that a positive shock in the energy efficiency significantly decreases CO2 emissions while negative shock increases CO2 emissions in both developed and developing countries. Huang et al. (Citation2021b) examined the impact of green investment, renewable energy consumption and technological innovation on CO2 emissions of 30 sample provinces of China from 1995–2019. The results of CS-ARDL approach shows that renewable energy, technological innovation and green investment is important in abating CO2 emissions in China. It is also found that any policy change in green investment, financial development, renewable energy, technological innovation, and natural resource rent has strong implications for environmental quality of China. Therefore, shifting the economic structure to renewable energy is an important strategy to reduce carbon emissions. One of the earlier studies of Worrell et al. (Citation2001) investigated the US iron and steel industry for examining the energy efficiency and CO2 emissions reduction. Examining 47 technologies concerning energy efficiency, the results concluded that energy efficiency efficiently reduces CO2 emissions with a three-year payback period. Besides, Wang et al. (Citation2020) and Huang et al. (Citation2021a) revealed that the prime reason for the CO2 emission level surge is the deterioration in the energy efficiency level. However, with the increase of the coal, diesel, and metal processing efficiencies, the carbon intensity is decreased.
Regarding the influence of financial inclusion on CO2 emissions, scholars and researchers provide extensive literature with contradictory findings. Some studies provided results that describe the financial inclusion impact as positive on the environmental quality, while others showed the negative influence of financial inclusion on environmental quality. Such studies include Qin et al. (Citation2021), Renzhi and Baek (Citation2020), Le et al. (Citation2020) while providing contradictory results for different countries and regions. Specifically, the recent study of Qin et al. (Citation2021) studied the financial inclusion in promoting a sustainable environment for emerging seven economies throughout 2004–2016. Using the quantile regression estimator, the study found that financial inclusion positively affects CO2 emissions and aggravates environmental degradation. Besides, the authors also confirmed the validity of the EKC hypothesis in the region. On the contrary, Renzhi and Baek (Citation2020) investigated 103 economies in the period of 2004–2014 and claimed the validity of inverted U-shaped financial inclusion based EKC hypothesis in the selected panel. The study further argued that financial inclusion could be used as a CO2 emissions mitigation measure in the selected group of 103 economies. On the contrary, Le et al. (Citation2020) examined the influence of financial inclusion on the Asian economies' CO2 emissions covering the period of 2004–2014. The study's findings reveal that financial inclusion, economic growth or income, and the other macroeconomic variables positively influence CO2 emissions. However, trade openness is the only variable found in the study that negatively affects CO2 emissions. Based on panel data of 30 provinces in China from 2009 to 2017, Huang and Chen (Citation2021) utilized the spatial Durbin model (SDM) and the threshold model to examine the effect of green finance on environmental quality. They found green finance has a significant positive effect on local environmental quality but has negative effects on that of its neighbors during the research period; however, the spatial spillover effect of green finance is heterogeneous in the three subsamples.
Besides energy efficiency and financial inclusion, other variables, including economic growth, environmental-related technological innovation, and human Capital, also determine the environmental quality of a country or region. In this regard, the scholars provided extensive literature examining the symmetric or asymmetric impact of economic growth on the level of CO2 emissions. Such studies include Wang and Zhang (Citation2020), Dong et al. (Citation2020), Banday and Aneja (Citation2020), and Ahmad et al. (Citation2018). Notably, the studies of Ahmad et al. (Citation2018) revealed that economic growth and CO2 emissions are cointegrated. Besides, economic growth significantly increases CO2 emissions. Banday and Aneja (Citation2020) investigated BRICS economies over the period from 1990 to 2017 via the Bootstrap panel causality approach. They found a unidirectional relationship between GDP and CO2 emissions for these countries except for Russia. Similarly, Wang and Zhang (Citation2020) also worked on the decoupling state between economic growth and CO2 emissions in BRICS economies and provided mixed results for countries. In contrast, Dong et al. (Citation2020) investigated economic growth and the CO2 emission nexus in China. They revealed that declination in carbon emission enhances the up-gradation of the industrial structure, which further promotes economic growth. However, both the industrial structure up-gradation and economic growth negatively affect CO2 emission in the region.
In addition to the prior, many existing studies empirically examined the association between economic growth and CO2 emissions and other economic and non-economic indicators for various countries and regions. Specifically, Huang et al. (Citation2021c) demonstrate that the targeted economic growth leads developing economies towards environmental degradation, whereas human Capital and green energy are yet to develop. Additionally, Mushta et al. (Citation2020) demonstrate that income inequality and economic growth strongly influence CO2 emissions in China, while technological innovation moderates this nexus or level of influence. On the other hand, Ozturk and Salah Uddin (Citation2012) empirically demonstrate that economic growth and energy consumption strongly cause each other, contributing to CO2 emissions surge and causing environmental degradation. On the contrary, Ozturk et al. (Citation2021) revealed that economic growth negatively and significantly affects CO2 emissions in Saudi Arabia, whereas oil prices and energy consumption positively affects CO2 emissions in the country. Concerning the causal nexus of economic growth and CO2 emissions, Obradović and Lojanica (Citation2017) asserted that energy consumption and economic growth cause CO2 emissions in the long run, whereas no causal association exists in the short-run. The study of Khoshnevis Yazdi and Golestani Dariani (Citation2019) revealed that urbanization increases energy consumption and leads to higher CO2 emissions in Asian economies. Moreover, the study of Wahab et al. (2021) validated the earlier positive association between economic growth and CO2 emissions. However, the study claimed that energy productivity, technological innovation, and exports are negatively associated with CO2 emissions and promote environmental sustainability.
While discussing CO2 emission reduction, the role of environmental-related technological innovation and human Capital cannot be ignored. Several studies identified the influence of innovation on CO2 emissions for different regions and countries. Such as, Töbelmann and Wendler (Citation2020) investigated 27 European Union economies over the period 1992–2014 by using the generalized method of moment (GMM) approach. The estimated results asserted that environmental-related innovation contributes to CO2 emission reduction, while general innovation does not contribute to CO2 emission reduction. Zhang et al. (Citation2017) used the system-GMM method for the case of China throughout 2000–2013 and revealed that environmental innovation contributes to CO2 emission reduction. However, energy efficiency played a major role in environmental innovation measures, followed by resource innovation and knowledge innovation. Similarly, Huang et al. (Citation2022b) scrutinized the nexus between information and communication technologies (ICT), renewable energy, economic complexity, human capital, financial development, and ecological footprint for E-7 (developing) and G-7 (developed) countries over the period from 1995 to 2018. Moreover, other studies, including Razzaq et al. (Citation2021) and Bai et al. (Citation2020), found a significant negative association of innovation and technological innovation on CO2 emissions in China. In contrast, Cheng et al. (Citation2019) investigated OECD economies using panel quantile regression techniques and found that economic growth significantly enhances CO2 emission level; however, the development of the patent showed positive but insignificant influence concerning CO2 emission reduction.
Human Capital plays a crucial role in every economy's economic and environmental conditions. Many studies extensively investigated the influence of human Capital on both economic growth and the environment. Concerning the environment, Bano et al. (Citation2018) investigated the influence of human Capital on the CO2 emission level in Pakistan covering the 1971–2014 period. The study used ARDL and causality tests and revealed that human capital improvement significantly improves environmental quality by reducing CO2 emissions. Besides, a bidirectional causal association is found between human Capital and CO2 emissions. In continuation, the studies of Sheraz et al. (Citation2021), Huang et al. (Citation2022a), Sarkodie et al. (Citation2020), and Mahmood et al. (Citation2019) investigated different countries and regions across the world. They found that human capital's positive influence on emissions mitigation and environmental sustainability due to education and skill factors without affecting economic growth. In contrast, Huang et al. (Citation2022b) found that there is a wide discrepancy in E-7 (developing) and G-7 (developed) countries. On the one hand, ICT, economic complexity, and human capital increase the pollution level while renewable energy significantly reduces it in E-7 countries. On the other hand, in G-7 countries, all potential factors significantly improve the environmental quality except financial development.
3. Methodology and specification of the model
3.1. Empirical modeling and data
Dietz and Rosa (Citation1997) established the stochastic impact by regression on population, affluence, and technology (STIRPAT) model as a substitute to IPAT identification, which was widely utilized in prior research evaluating the causes and components for the destruction of the environment. The initial focus of this Model was on measuring the impact of the rising global population on the environment; but, as time passed by, it has expanded to include technology and affluence. The IPAT model monitors the consequences of human activities on the environment; however, it is controversial since several of its deficiencies contradict the environmental Kuznets curve (EKC) theory. It offers a unit (one) elasticities and evaluates technological progress or technological innovations. It gives no clear guidance about how to integrate or address different cultures. Furthermore, it is limited to measuring the assessment of a particular variable to track environmental impacts such as air or water pollution. Besides, there may be the interrelationship between variables like technology, affluence, and population, resulting in an econometric problem in the Model such as multicollinearity (Chertow, Citation2000). Generally, advanced analytical techniques for identifying the impact of technology, affluence, and population are lacking in IPAT identification. The following is the IPAT identity equation:
(1)
(1)
From the above equation, I depicts pollution, P demonstrates urban population, which could also be replicated with the human capital index since urbanization is a factor of improved human capital. Thus, a demographic factor is covered. Additionally, A indicates affluence such as GDP and/or financial inclusion, whereas T could be asserted as environmental related technologies and energy efficiency in this context. In contrast, the STIRPAT model may be utilized here, which is relatively superior to the IPAT model. It is more efficient to employ almost all types of data, such as panel data, cross-section data, and time-series data, to create a far more versatile measurement framework via effective delivery of the elasticity of impact for each variable. Furthermore, the residuals derived or antilog is interpreted as a pollution intensity technology multiplier. It offers a robust quantitative framework for investigating the influence of various variables on the environment, which could be expressed as follows:
(2)
(2)
In the above EquationEq. (2)(2)
(2) , some of the terms of STIRPAT model including I, P, A, and T are similar to that of IPAT model indicated as EquationEq. (1)
(1)
(1) . In comparison, t could be differentiated from the above Model, which demonstrates time period. In the STIRPAT model,
represents the term for intercept, whereas
and
reveal the coefficients drawing the influence of P, A, and T on environment, respectively. Besides,
indicates the term used for random error, which consists of other factors the affect the dependent variable (I) implicitly. Current study uses carbon dioxide (CO2) emissions as a proxy for pollution (I). At the same time, two variables that may represent affluence in this context is financial inclusion (FIN) and gross domestic product (GDP), which are used in the two separate models since both covers the economic factors for the Model. Additionally, the urban population indicates (P) is proxied by the human capital index (HCI), which the earlier studies have depicted as a strong influencer of environmental pollution (Bano et al., Citation2018; Huang et al., Citation2022a; Sarkodie et al., Citation2020). Lastly, the proxy for technology, in this case, is energy efficiency and energy-related technological innovation, which could affect environmental pollution (Mahapatra & Irfan, Citation2021; Huang et al., Citation2021b). Following the above-mentioned models, this study emphasizes on the STIRPAT model.
Based on the prior literature as provided in Sec. 2 and the theoretical notion above, it is observed that the recent trend of reducing the CO2 emission in controlling environmental degradation via energy efficiency attracts the scholars' and researchers' attention. However, many environmental and economic indicators have been used to identify the impact of these indicators on environmental sustainability or environmental degradation. Still, the number of indicators remained unexplored or provided mixed or diverse results for different groups, regions, and countries. In this regard, the current study used five exogenous variables that are assumed to influence environmental performance by affecting the carbon emission level in the selected panel. The considered variables include financial inclusion, gross domestic product (a proxy for economic growth), energy efficiency, environmental-related technological innovation, and human capital index. The first exogenous variable is the index calculated by utilizing variables including Institutions of commercial banks, Branches of commercial banks, Outstanding deposits with commercial banks (% of GDP), Numbers of ATMs per 100,000 adults and outstanding loans from commercial banks (% GDP). The second exogenous variable is GDP and measured at constant US$2010 prices. The third exogenous variable, energy efficiency, takes energy consumption per unit of GDP as proxy and is measured as constant 2017 PPP $per kg of oil equivalent. These discussed variables are considered for the selected five Regional Comprehensive Economic Partnership (RCEP) countries, including Australia, China, Indonesia, Japan, and South Korea, covering the latest dataset from 2004 to 2019. Variables under consideration, their specifications and data sources are provided in .
Table 1. Variables' specifications and data sources.
The current study constructs two models from the variables under consideration to demonstrate the influence of two exogenous variables, i.e., financial inclusion and GDP, in distinction. Two models are efficient in ‘what if’ or robust analysis. The constructed models are presented in general form as Model 1 and Model 2 below.
Model 1
Model 1 reveals that carbon emission is the function of financial inclusion, energy efficiency, environmental-related technological innovation, and human capital index across the selected panel throughout the selected period.
Model 2
where Model 2 indicates that carbon emission is the function of GDP, energy efficiency, environmental-related technological innovation and human capital index across the selected panel throughout the selected period, the modified form of these two models into regression equation are EquationEqs. (3)
and Equation(4)
given below, respectively.
(3)
(3)
(4)
(4)
where
represents the intercept, and
and
represent the slope coefficient for each exogenous variable taken under consideration in both Model 1 and Model 2, respectively. Furthermore, the ‘i’ and ‘t’ in the subscript signify the cross-section and time-series of the selected countries in the panel, respectively.
3.2. Estimation strategy
After the theoretical background and specification of the regression model, the current study uses various econometric techniques to estimate these models empirically. In this regard, we use different econometric approaches, including normality test, panel cross-section and slope heterogeneity tests, unit root test, cointegration test, long run estimates of the Model, and the causality test for the selected group of countries.
3.2.1. Normality test
In an empirical investigation, it is important to analyze the normality of the data as it leads to adopting a valid and efficient estimator. In this regard, the current study uses the Jarque and Bera (Citation1987) normality test. The said test assumed the skewness and excess kurtosis to be zero, which specifies the data's normality. The Jarque–Bera test usually considers both the skewness and kurtosis to indicate the normality of the data for each variable.
3.2.2. Slope heterogeneity and panel cross-section dependence/independence
After the normality of the data, this study moves toward empirical investigation and begins with assessing slope heterogeneity and cross-section dependency. Since the industrial revolution, countries worldwide wholly or partially have been dependent on other countries for various economic, environmental, political, and financial reasons. The interdependence among economies instigates these countries similar in some respects while different in others. However, the homogeneous characteristics of countries in an econometric investigation could provide biased estimates. Therefore, this study examines the slope heterogeneity of the selected RCEP economies to avoid biased outcomes using the Pesaran and Yamagata (Citation2008) slope coefficient homogeneity (SCH) test across the selected 5 RCEP countries. The said test holds the null hypothesis as homogeneous slopes across the panel, which could be rejected after significant results. Generally, the Pesaran and Yamagata (Citation2008) SCH test provides both the SCH and the adjusted SCH (ASCH) results. The final form of the SCH and ASCH test is given below as EquationEqs. (5)(5)
(5) and Equation(6)
(6)
(6) :
(5)
(5)
(6)
(6)
where the
and
Designate adjusted slope coefficient heterogeneity and slope coefficient heterogeneity, respectively.
As priorly mentioned, some factors increase the dependence of one country on other countries. However, the cross-section dependence issue is more common in panel data. Besides, ignoring such issue would also lead to inconsistent and biased estimates (Campello et al., Citation2019). The current study employs the Pesaran (Citation2004) cross-section dependence (CD) test to investigate the cross-section dependence of the selected 5 countries and avoid biased estimates. The Pesaran (Citation2004) CD test assumed that the cross-sections across the panel are independent. Specifically, the said test reveals no cross-section dependence between the selected countries, assuming the null hypothesis. The Pesaran (Citation2004) CD test is presented in the final form as EquationEq. (7)(7)
(7) below:
(7)
(7)
3.2.3. Unit root testing
After examining the basic required tools such as variables' normality, cross-section dependence and slope heterogeneity of the cross-sections, this study further analyzes the stationarity of the data across time. One of the main reasons for the biased or inconsistent outcomes is that the data is non-stationary throughout the selected time span. Therefore, we utilize the cross-sectionally augmented IPS (CIPS) unit root test which Pesaran (Citation2007) provided. This unit root test is efficient in tackling the slopes heterogeneity and the cross-section dependency issues. We use the Pesaran (Citation2007) CIPS unit root test on the data under consideration for both the level [I(0)] and first differenced [IEquation(1)(1)
(1) ] data. The said test assumed the null hypothesis as the non-stationarity or the presence of the unit root in data.
3.2.4. Cointegration testing
After testing for the stationarity of the data, we further investigate the long-run relationship between the variables under consideration for the selected RCEP economies. Thus, we use the Westerlund (Citation2007) error correction model (ECM) to test the cointegration association among the variables. The said test is an efficient cointegration test because of the high power of tackling the cross-section dependency and slope coefficient heterogeneity issues by considering both the group mean statistics and the panel statistics. Generally, the group mean statistics and the panel statistics are presented in the final form as and
for group mean statistics, and
and
for panel statistics.
3.2.5. Quantile regression and panel causality test
After the preliminary obligations of the empirical investigation, including the normality test, slope heterogeneity and cross-section dependence tests, and the cointegration test, we empirically analyze the long-run influence of the variables under consideration on the CO2 emission using the quantile regression approach provided by Koenker and Bassett (Citation1978). The reason for using the quantile regression approach is that the Jarque and Bera (Citation1987) test estimates reveal that the data for considered variables are non-normal, whereas the conventional techniques could not provide reliable estimates. Additionally, to avoid the over and under-estimate biase of the coefficient estimates in these conventional approaches, this study employs the quantile regression approach, which provides the estimated coefficient at each selected quantile. The panel quantile regression method is more efficient than the ordinary least square approach due to handling both individual and distributional heterogeneity, which provides thorough information of the relationship between the variables under consideration (Cheng et al., Citation2019). Besides, the quantile regression has more power than the conventional regression, providing only the exogenous variables' average effect. Moreover, the said estimator efficiently handles the slopes heterogeneity and the cross-sectional dependency issues (Huang et al. Citation2022a). The aforementioned regression equations, i.e., EquationEqs. (3)(3)
(3) and Equation(4)
(4)
(4) , could be modified into the panel quantile regression form as provided in EquationEqs. (8)
(8)
(8) and Equation(9)
(9)
(9) , respectively.
(8)
(8)
(9)
(9)
where
signifies the quantile for each variable under consideration, however, this study adopts three quantiles, i.e., Q25, Q50, and Q75, to empirically investigate the influence of financial inclusion, GDP, energy efficiency, environmental-related technological innovation, and the human capital index on the CO2 emission of the five RCEP economies.
After estimating the long-run coefficients of the exogenous variables, we further analyze the causal relationship between the under discussion variables employing the Dumitrescu and Hurlin (Citation2012) Granger panel causality heterogeneous test. It is assumed that when the time-series is not equal to the cross-section, the Dumitrescu and Hurlin (Citation2012) Granger panel causality heterogeneous test provides efficient estimates. Besides, the said test also tackles the panel data issues such as slope coefficient heterogeneity and the cross-section dependency.
4. Results and discussion
This analysis section begins with the descriptive statistics and the normality test estimates as provided in . The mean and median values of the endogenous variable CO2 emissions are approximately the same and accounted for 5.992177 and 5.767571 kt emissions in the five RCEP economies. The standard deviation is reported as 0.505765 deviations from the mean value. However, the Jarque and Bera (Citation1987) normality test notify the probability value as 0.000235, which is less than the 1% significance level. Thus, the under-discussion variable rejected the null hypothesis and concluded the non-normal distribution of the variable's data. The mean values for the exogenous variables, i.e., financial inclusion and GDP, are reported as 0.399730 and 12.32890, respectively. While the standard deviation for both variables is less than the endogenous variable's standard deviation, it accounted for 0.270255 and 0.398053 values, respectively. The Jarque–Bera probability values for both the financial inclusion and the GDP are found significant at 10% and 5% levels, leading to the rejection of the null hypothesis and revealing that the data for both variables are non-normal. Besides, the environmental-related technological innovation is found insignificant regarding the Jarque–Bera normality test at 1%, 5% and 10%. This leads to accepting the null hypothesis of the normal distribution of the data. Additionally, the mean and the median values are 0.952141 and 0.972431, respectively. At the same time, the standard deviation is found 0.106007, which is the lowest from the priorly mentioned variables. The data carries the mean and median values of the human capital index as 0.476544 and 0.535608, respectively. However, the standard deviation is reported as 0.083599, the lowest among all the variables under consideration. Still, the Jarque–Bera (1987) probability values are found significant at 1% level, rejecting the null hypothesis that the data is normal and concluding that the human capital index data is not normally distributed. Lastly, the energy efficiency variables have the mean and median values of 0.887862 and 0.904632, respectively. The said variable holds a lower standard deviation than the CO2 emissions, financial inclusion, and the GDP, accounting for 0.133457 deviations from the mean value. Moreover, the Jarque–Bera normality test holds the probability value of 0.038105, significant at the 5% level. Thus, it rejected the null hypothesis of variable data's normality and assumed that the data for the energy efficiency is not normally distributed.
Table 2. Data normality tests.
The estimated results for the slope coefficient heterogeneity and the cross-section dependence are provided in . Regarding slope heterogeneity via the Pesaran and Yamagata (Citation2008) test, both the SCH and the adjusted SCH values are significant at 1% in Model 1 and Model 2. This leads to the rejection of the null hypothesis of homogeneous slopes across the panel and concludes that the slopes coefficients are heterogeneous. Since the industrial revolution, globalization and trade openness have been playing a crucial role in eliminating barriers across the territories. This further increases one country's dependency on other economies for the demand and supply of various goods and services to achieve different economic, financial, and political targets. In this concern, the Pesaran (Citation2004) CD test provides significant estimates for CO2 emission, environmental related innovations, GDP, human Capital, and energy efficiency, rejecting the null hypothesis of no cross-section dependence across the panel. Instead, the panel assumes that the five RCEP economies are cross-sectionally dependent on CO2 emissions, environmental-related technological innovation, GDP, human capital index, and energy efficiency. In contrast, financial inclusion showed insignificant results that hold the null hypothesis of no cross-section dependency across the panel regarding financial inclusion.
Table 3. Slope heterogeneity and cross-section dependence.
The estimated results for the Pesaran (Citation2007) unit root testing are provided in . Firstly, the unit root test is estimated on the data at level [I(0)], where the outcomes for all the variables are found insignificant and the unit root's presence in the data. However, after analyzing the unit root test on the data at first difference [IEquation(1)(1)
(1) ], the values for each variable are reported significant, which validates the rejection of the null hypothesis and concludes that the data is stationary and has no unit root. The stationary data further leads us to examine the long-run cointegration relationship between the variables under discussion.
Table 4. Unit root testing (Pesaran, Citation2007).
The estimated cointegration results obtained by employing the Westerlund (Citation2007) error correction model are presented in . The said test assumes that the error correction term is zero in a conditional panel error correction model, i.e., ECT = 0. However, the values of both the group mean and panel statistics are found significant in the two selected models at the 1% level. The values of both the group mean statistics and the panel statistics are found negative significant, revealing the convergence of the variables to the equilibrium state instead of being at the equilibrium state. Hence, it is determined that carbon emission, financial inclusion, GDP, environmental-related innovation, human capital index and energy efficiency encompass the long-run cointegration relationship.
Table 5. Cointegration results (Westerlund Citation2007).
presents the estimated results for the panel quantile regression identifying the influence of financial inclusion, environmental-related technological innovation, human capital index, and energy efficiency on the CO2 emission in model 1, and the impact of GDP, environmental-related technological innovation, human capital index, and energy efficiency on CO2 emission in Model 2 at 25th, 50th and 75th quantile. Specifically, the results of the Model 1 reveal that a one per cent increase in the financial inclusion increases the CO2 emission level by 1.753, 1.765 and 1.470% in the 25th, 50th and 75th quantile, respectively. The results are found statistically significant at a 1% level in all three quantiles. It is noted that the influence of financial inclusion is the strongest in the 50th quantile. The current study's findings are consistent with the earlier findings of Qin et al. (Citation2021) and Le et al. (Citation2020), which argued that financial inclusion supports the industrial and manufacturing sectors in the form of loans and other financial support to expand and produce more goods. This increased demand for fossil fuel energy consumption and consequently enhanced CO2 emission level, adversely affecting environmental conditions. In contrast, the environmental-related technological innovation (ERTI), human capital index (HCI), and energy efficiency (ENEF) significantly help in reducing CO2 emissions across the three quantiles. Specifically, a one per cent increase in the ERTI significantly reduces CO2 emissions by 0.0265, 0.286 and 0.180% in the 25th, 50th and 75th quantile in model 1 at 1% level, respectively. The influence of ERTI is found strongest in the 50th quantile. These findings showed consistency to the earlier findings of Töbelmann and Wendler (Citation2020), Zhang et al. (Citation2017), Huang et al. (Citation2022a), Razzaq et al. (Citation2021) and Bai et al. (Citation2020) that claims the positive influence of environmental-related and technological related innovations on the environment due to consumption of environmentally friendly resources.
Table 6. Panel quantile regression results.
A one per cent increase in the human capital index significantly decreases CO2 emissions by 0.646, 0.624 and 0.432% across the three selected quantiles in model 1, respectively. The results are significant at a 1% level in all three quantiles. However, the HCI strongly affects the CO2 emission reduction in the first 25th quantile. The estimated results concerning HCI is consistent with the findings of earlier studies, including Bano et al. (Citation2018), Sheraz et al. (Citation2021), Sarkodie et al. (Citation2020), Huang et al. (Citation2022a), and Mahmood et al. (Citation2019), which argued that the higher education in specific, could lead the manufacturing, as well as the domestic sectors, consume environmentally friendly energy sources and efficient utilization of the energy while targeting the energy-saving approach, which consequently reduces the CO2 emission level in the atmosphere and promotes environmental sustainability. Similarly, the ENEF negatively affects the CO2 emission of the five RCEP economies by 1.498, 1.647 and 1.302% in the three quantiles in model 1, respectively, if increases by one per cent. The results of the ENEF are found significant at a 1% level and consistent with the findings of Akram et al. (Citation2020), Fernando and Hor (Citation2017), Mahapatra and Irfan (Citation2021). The primary reason behind the negative effect of the ENEF is that the advancement in energy-efficient products enhances the energy saving of those resources that contribute to environmental degradation. At the same time, fossil fuel energy saving significantly reduces the CO2 emission level in the atmosphere and promote environmental quality.
Concerning Model 2, the GDP is reported to have a significant positive influence on the CO2 emission level. Specifically, a one per cent increase in the GDP significantly increases the CO2 emission level by 0.942, 0.947 and 0.914% in the 25th, 50th and 75th quantile, respectively. However, the results reveal that the GDP strongly affects the CO2 emission in the 50thquantile. The estimated results are found statistically significant at 1% level and support the earlier findings of Wang and Zhang (Citation2020), Dong et al. (Citation2020), Banday and Aneja (Citation2020), Huang et al. (Citation2021a), and Ahmad et al. (Citation2018). The significant positive impact of GDP on CO2 emissions is that the demand for fossil fuel consumption increases due to increased income. Also, the revenue and profit to the manufacturing industries increase, ultimately increasing the demand for fossil fuel consumption. This is well known that fossil fuel consumption releases more hazardous gases to the environment, including CO2; thus, higher income leads to environmental degradation. Besides, other variables, including ERTI, HCI, and ENEF, exert the similar effect as in model 1 on the CO2 emission level. However, the magnitude of these variables is reported slightly transformed, still highly statistically significant at 1% level.
This study further investigates the panel causal association among the variables. The long-run estimates of the panel quantile regression do not provide any causal relationship between the study variable. Therefore, the study employs the Dumitrescu and Hurlin (Citation2012) Granger panel causality heterogeneous test, and the estimated results are presented in . Though, it is mentioned in the earlier discussion that FIN enhances environmental degradation in the five RCEP economies. However, the policies targeting FIN are essential from the environmental perspective due to the unidirectional causal relationship running from FIN to CO2 emissions. This supports the priorly discussed findings that financial inclusion adversely affects the environment. The findings are consistent with the study of Qin et al. (Citation2021), which found the unidirectional causal relationship running from financial inclusion to CO2 emissions in emerging seven economies. Similarly, the environmental-related technologies unidirectionally and significantly cause CO2 emissions in the five RCEP economies.
Table 7. Causality check.
In contrast, this study finds a bidirectional causal association between GDP and CO2 emissions. Unlike the study of Banday and Aneja (Citation2020), who found a unidirectional causal association between these two for Brazil, Russia, India and China, this study provides significant results at a 1% level. The main reason for the bidirectional causal association is that the higher economic growth further enhances industrial production and consumers' demand, which increases the use of fossil fuel energy and consequently enhances CO2 emissions. On the other hand, CO2 emission occurs due to fossil fuel consumption. However, higher fossil energy consumption instantly fuels economic activities and promotes economic growth. Similarly, this study finds a bidirectional causal association between the HCI and CO2 emission, which is consistent with the findings of Huang et al. (Citation2022b). This reveals that higher education and skills levels promote economic activities in the economy, which extensively use environmentally hazardous energy and cause environmental degradation. Lastly, the energy efficiency and CO2 emission are reported to be bi-directionally and significantly associated. The CO2 emissions, when reached to a high level, the economy focus on protecting the environment and promoting the energy-saving and energy efficiency projects, which in turn reduce the atmospheric CO2 emissions level and lead to environmental sustainability.
5. Conclusion and policy implications
5.1. Conclusion
The Regional Comprehensive Economic Partnership (RCEP) economies includes developed and developing economies grouped for the free trade agreement, which also affects the demand and supply structure of these economies for both environmentally friendly and hazardous goods and services. It is well studied that increasing economic activities lead to economic growth, financial inclusivity, and environmental degradation by enhancing CO2 emissions. However, countries are concerned about increasing energy efficiency to reduce environmental impacts. Therefore, it is necessary to investigate the influence of energy efficiency, financial inclusion, and economic growth along with human capital and environmental-related technological innovation. This study attempts to investigate the impact of these variables on the carbon emission level of the five selected RCEP economies covering the period from 2004 to 2019. As both the time series and the cross-sections are in focus, this study utilizes panel data techniques such as the Pesaran and Yamagata (Citation2008) slope coefficient heterogeneity, Pesaran (Citation2004) cross-section dependence, Pesaran (Citation2007) cross-sectionally augmented IPS, and the Westerlund (Citation2007) cointegration test. The estimated results reveal that slope coefficients are found heterogenous in the panel. In addition, it is also reported in the empirical estimations that these variables are cross-sectionally dependent. Besides, the data is stationary at IEquation(1)(1)
(1) rather than the I(0). Also, the variables under consideration are found having cointegration relationship in the long-run, which further leads the study to investigate the influence of each concerning variable on the CO2 emission in the five RCEP economies. Thus, we utilizes a quantile regression estimator, which provides an efficient estimate at each quantile rather than the conventional OLS approach. The results reveal that financial inclusion and economic growth significantly promote the CO2 emission level in the five RCEP economies at all three quantiles, i.e., 25th, 50th and 75th quantile. However, financial inclusion and economic growth are stronger in the second quantile than in the 25th and 75th quantiles.
In contrast, energy efficiency, human capital, and environmental-related technological innovation negatively and significantly affect CO2 emissions and promote environmental sustainability. Moreover, the panel causality test of Dumitrescu and Hurlin (Citation2012) reports the unidirectional causal association running from financial inclusion and environmental-related technological innovation to CO2 emissions. However, bidirectional causality is found between GDP, energy efficiency and human capital index with CO2 emission in the five RCEP economies.
5.2. Policy implications
This study provides some practical policy implications based on the empirical findings of the five RCEP economies as the concerned group of countries includes both developed and developing economies. Therefore, the probability of higher environmental degradation could be assumed due to the industrialization process of developed economies and the shifting of pollution-intensive industries to the developing nations, termed as the pollution Haven hypothesis. Thus, it is strongly recommended for these regions to adopt energy-efficient resources that could promote the culture of energy savings in both developed and developing economies. Enhancement in energy saving and energy efficiency throughout these economies will reduce demand for conventional fossil fuel energy, simultaneously reducing CO2 emissions in the region and promoting environmental sustainability. In addition, financial inclusion is reported to have an aggravation effect on the CO2 emissions of these regions, yet these economies are struggling for improvement in financial inclusion. In this regard, concerning policies must be revised that promote financial inclusion and encourage green investments, green bonds, and investment in environmental related technologies to combat CO2 emission in the region. Besides, human Capital could also be used as a remedial measure for environmental degradation, as depicted in the empirical results. Therefore, it is suggested that these economies promote higher education and skills improvement, which helps to efficiently use of energy, boost energy-saving attitude, reduce energy intensive products and services, and contribute to environmental sustainability or environmental recovery. Lastly, policies concerning economic growth should also be revised since the empirics reveals its adverse impact on environmental quality. Therefore, the higher economic growth could be targeted for environmental recovery, which includes various policy measures such as adopting renewables or environmentally friendly energy sources, energy-saving measures, structural transformation of the industrial sector, and investing in human capital development to mitigate the CO2 emissions in RCEP regions.
Correction Statement
This article has been republished with minor changes. These changes do not impact the academic content of the article.
Additional information
Funding
Notes
References
- Ahmad, M., Khan, Z., Ur Rahman, Z., & Khan, S. (2018). Does financial development asymmetrically affect CO2 emissions in China? An application of the nonlinear autoregressive distributed lag (NARDL) model. Carbon Management, 9(), 631–644. https://doi.org/10.1080/17583004.2018.1529998
- Akram, R., Chen, F., Khalid, F., Ye, Z., & Majeed, M. T. (2020). Heterogeneous effects of energy efficiency and renewable energy on carbon emissions: Evidence from developing countries. Journal of Cleaner Production, 247, 119122. https://doi.org/10.1016/j.jclepro.2019.119122
- Al-Mulali, U., Saboori, B., & Ozturk, I. (2015). Investigating the environmental Kuznets curve hypothesis in Vietnam. Energy Policy, 76, 123–131. https://doi.org/10.1016/j.enpol.2014.11.019
- Bai, C., Feng, C., Yan, H., Yi, X., Chen, Z., & Wei, W. (2020). Will income inequality influence the abatement effect of renewable energy technological innovation on carbon dioxide emissions? Journal of Environmental Management, 264, 110482. https://doi.org/10.1016/j.jenvman.2020.110482
- Banday, U. J., & Aneja, R. (2020). Renewable and non-renewable energy consumption, economic growth and carbon emission in BRICS. International Journal of Energy Sector Management, 14(1), 248–260. https://doi.org/10.1108/IJESM-02-2019-0007
- Bano, S., Zhao, Y., Ahmad, A., Wang, S., & Liu, Y. (2018). Identifying the impacts of human Capital on carbon emissions in Pakistan. Journal of Cleaner Production, 183, 1082–1092. https://doi.org/10.1016/j.jclepro.2018.02.008
- Campello, M., Galvao, A. F., & Juhl, T. (2019). Testing for slope heterogeneity bias in panel data models. Journal of Business & Economic Statistics, 37(4), 749–760. https://doi.org/10.1080/07350015.2017.1421545
- Cheng, C., Ren, X., & Wang, Z. (2019). The impact of renewable energy and innovation on carbon emission: An empirical analysis for OECD countries. Energy Procedia, 158, 3506–3512. https://doi.org/10.1016/j.egypro.2019.01.919
- Chertow, M. R. (2000). The IPAT equation and its variants. Journal of Industrial Ecology, 4(4), 13–29. https://doi.org/10.1162/10881980052541927
- Danish, B., M. A., Mahmood, N., & Zhang, J. W. (2019). Effect of natural resources, renewable energy and economic development on CO2 emissions in BRICS countries. Science of the Total Environment, 678, 632–638. https://doi.org/10.1016/j.scitotenv.2019.05.028
- Dietz, T., & Rosa, E. A. (1997). Effects of population and affluence on CO2 emissions. Proceedings of the National Academy of Sciences of the United States of America, 94(1), 175–179. https://doi.org/10.1073/pnas.94.1.175
- Dong, B., Xu, Y., & Fan, X. (2020). How to achieve a win-win situation between economic growth and carbon emission reduction: Empirical evidence from the perspective of industrial structure upgrading. Environmental Science and Pollution Research, 27(35), 43829–43844. https://doi.org/10.1007/s11356-020-09883-x
- Dumitrescu, E. I., & Hurlin, C. (2012). Testing for Granger non-causality in heterogeneous panels. Economic Modelling, 29(4), 1450–1460. https://doi.org/10.1016/j.econmod.2012.02.014
- Fernando, Y., & Hor, W. L. (2017). Impacts of energy management practices on energy efficiency and carbon emissions reduction: A survey of Malaysian manufacturing firms. Resources, Conservation and Recycling, 126, 62–73. https://doi.org/10.1016/j.resconrec.2017.07.023
- Grant, D., Jorgenson, A. K., & Longhofer, W. (2016). How organizational and global factors condition the effects of energy efficiency on CO2 emission rebounds among the world's power plants. Energy Policy, 94, 89–93. https://doi.org/10.1016/j.enpol.2016.03.053
- Huang, Y., Ahmad, M., Ali, S., & Kirikkaleli, D. (2022a). Does eco-innovation promote cleaner energy? Analyzing the Role of Energy Price and Human Capital, Energy, 239(Part D), 122268. https://doi.org/10.1016/j.energy.2021.122268.
- Huang, Y., & Chen, C. (2021). The spatial spillover and threshold effect of green finance on environmental quality: evidence from China. Environmental Science and Pollution Research. https://doi.org/10.1007/s11356-021-16892-x
- Huang, Y., Haseeb, M., Muhammad Usman, M., & Ozturk I. (2022b). Dynamic association between ICT, renewable energy, economic complexity and ecological footprint: Is there any difference between E-7 (developing) and G-7 (developed) countries?. Technology in Society, 68, 101853. https://doi.org/10.1016/j.techsoc.2021.101853
- Huang, Y., & Khan, J. (2022). Has the information and communication technology sector become the engine of China’s economic growth? Review of Development Economics, 26, 510–533. https://doi.org/10.1111/rode.12821
- Huang, Y., Kuldasheva, Z., & Salahodjaev, R. (2021a).Renewable Energy and CO2 Emissions: Empirical Evidence from Major Energy- Consuming Countries. Energies, 14, 7504. https://doi.org/10.3390/en14227504
- Huang,Y., Xue, L., & Khan Z. (2021b) What abates carbon emissions in China: Examining the impact of renewable energy and green investment. Sustainable Development. 29, 823–834. https://doi.org/10.1002/sd.2177
- Huang, S. Z., Chien, F., & Sadiq, M. (2021c). A gateway towards a sustainable environment in emerging countries: The nexus between green energy and human Capital. Economic Research-Ekonomska Istraživanja, 1–18. https://doi.org/10.1080/1331677X.2021.2012218
- Jarque, C. M., & Bera, A. K. (1987). A test for normality of observations and regression residuals. International Statistical Review / Revue Internationale de Statistique, 55(2), 163–172. https://doi.org/10.2307/1403192
- Khoshnevis Yazdi, S., & Golestani Dariani, A. (2019). CO2 emissions, urbanisation and economic growth: Evidence from Asian countries. Economic research-Ekonomska Istraživanja, 32(1), 510–530. https://doi.org/10.1080/1331677X.2018.1556107
- Koenker, R., & Bassett, G. (1978). Regression quantiles. Econometrica, 46(1), 33–50. https://doi.org/10.2307/1913643
- Le, T. H., Le, H. C., & Taghizadeh-Hesary, F. (2020). Does financial inclusion impact CO2 emissions? Evidence from Asia. Finance Research Letters, 34, 101451. https://doi.org/10.1016/j.frl.2020.101451
- Mahapatra, B., & Irfan, M. (2021). Asymmetric impacts of energy efficiency on carbon emissions: A comparative analysis between developed and developing economies. Energy, 227, 120485. https://doi.org/10.1016/j.energy.2021.120485
- Mahmood, N., Wang, Z., & Hassan, S. T. (2019). Renewable energy, economic growth, human Capital, and CO2 emission: An empirical analysis. Environmental Science and Pollution Research, 26(20), 20619–20630. https://doi.org/10.1007/s11356-019-05387-5
- Mushta, A., Chen, Z., Ud Din, N., Ahmad, B., & Zhang, X. (2020). Income inequality, innovation and carbon emission: Perspectives on sustainable growth. Economic research-Ekonomska Istraživanja, 33(1), 769–787. https://doi.org/10.1080/1331677X.2020.1734855
- Obradović, S., & Lojanica, N. (2017). Energy use, CO2 emissions and economic growth–causality on a sample of SEE countries. Economic Research - Ekonomska Istraživanja, 30(1), 511–526. https://doi.org/10.1080/1331677X.2017.1305785
- Ozturk, I., & Salah Uddin, G. (2012). Causality among carbon emissions, energy consumption and growth in India. Economic research-Ekonomska Istraživanja, 25(3), 752–775. https://doi.org/10.1080/1331677X.2012.11517532
- Ozturk, I., Aslan, A., & Altinoz, B. (2021). Investigating the nexus between CO2 emissions, economic growth, energy consumption and pilgrimage tourism in Saudi Arabia. Economic Research - Ekonomska Istraživanja, 1–16. https://doi.org/10.1080/1331677X.2021.1985577
- Pesaran, M. H. (2004). General diagnostic tests for cross-sectional dependence in panels. Empirical Economics, 60, 13–50. https://doi.org/10.1007/s00181-020-01875-7
- Pesaran, M. H. (2007). A simple panel unit root test in the presence of cross-section dependence. Journal of applied econometrics, 22(2), 265–312. https://doi.org/10.1002/jae.951
- Pesaran, M. H., & Yamagata, T. (2008). Testing slope homogeneity in large panels. Journal of Econometrics, 142(1), 50–93. https://doi.org/10.1016/j.jeconom.2007.05.010
- Qin, L., Raheem, S., Murshed, M., Miao, X., Khan, Z., & Kirikkaleli, D. (2021). Does financial inclusion limit carbon dioxide emissions? Analyzing the role of globalization and renewable electricity output. Sustainable Development, 29(6), 1138–1154. https://doi.org/10.1002/sd.2208
- Razzaq, A., Sharif, A., Ahmad, P., & Jermsittiparsert, K. (2021). Asymmetric role of tourism development and technology innovation on carbon dioxide emission reduction in the Chinese economy: Fresh insights from QARDL approach. Sustainable Development, 29(1), 176–193. https://doi.org/10.1002/sd.2139
- Renzhi, N., & Baek, Y. J. (2020). Can financial inclusion be an effective mitigation measure? Evidence from panel data analysis of the environmental Kuznets curve. Finance Research Letters, 37, 101725. https://doi.org/10.1016/j.frl.2020.101725
- Sahay, R., Cihak, M., N’Diaye, P. M. B. P., Barajas, A., Mitra, S., Kyobe, A., Mooi, Y. N., & Yousefi, S. R. (2015). Financial inclusion: Can it meet multiple macroeconomic goals? International Monetary Fund. https://doi.org/10.5089/9781513585154.006
- Sarkodie, S. A., Adams, S., Owusu, P. A., Leirvik, T., & Ozturk, I. (2020). Mitigating degradation and emissions in China: The role of environmental sustainability, human Capital and renewable energy. The Science of the Total Environment, 719, 137530. https://doi.org/10.1016/j.scitotenv.2020.137530
- Sheraz, M., Deyi, X., Ahmed, J., Ullah, S., & Ullah, A. (2021). Moderating the effect of globalization on financial development, energy consumption, human Capital, and carbon emissions: Evidence from G20 countries. Environmental Science and Pollution Research, 28(26), 35126. https://doi.org/10.1007/s11356-021-13116-0
- Töbelmann, D., & Wendler, T. (2020). The impact of environmental innovation on carbon dioxide emissions. Journal of Cleaner Production, 244, 118787. https://doi.org/10.1016/j.jclepro.2019.118787
- Wahab, S., Zhang, X., Safi, A., Wahab, Z., & Amin, M. (2021). Does energy productivity and technological innovation limit trade-adjusted carbon emissions? Economic Research - Ekonomska Istraživanja, 34(1), 1896–1912. https://doi.org/10.1080/1331677X.2020.1860111
- Wang, F., Sun, X., Reiner, D. M., & Wu, M. (2020). Changing trends of the elasticity of China's carbon emission intensity to industry structure and energy efficiency. Energy Economics, 86, 104679. https://doi.org/10.1016/j.eneco.2020.104679
- Wang, K., & Wei, Y. M. (2014). China's regional industrial energy efficiency and carbon emissions abatement costs. Applied Energy, 130, 617–631. https://doi.org/10.1016/j.apenergy.2014.03.010
- Wang, Q., & Zhang, F. (2020). Does increasing investment in research and development promote economic growth decoupling from carbon emission growth? An empirical analysis of BRICS countries. Journal of Cleaner Production, 252, 119853. https://doi.org/10.1016/j.jclepro.2019.119853
- Westerlund, J. (2007). Testing for error correction in panel data. Oxford Bulletin of Economics and Statistics, 69(6), 709–748. https://doi.org/10.1111/j.1468-0084.2007.00477.x
- World Bank. (2018). Financial inclusion. https://www.worldbank.org/en/topic/financialinclusion/overview#1
- World Economic Forum. (2017). The global human capital report 2017: Preparing people for the future of work. In World economic forum. Switzerland. http://hdl.voced.edu.au/10707/444259.
- World Energy Outlook. (2017). Energy access outlook 2017: From poverty to prosperity. Special report. International Energy Agency.
- Worrell, E., Price, L., & Martin, N. (2001). Energy efficiency and carbon dioxide emissions reduction opportunities in the US iron and steel sector. Energy, 26(5), 513–536. https://doi.org/10.1016/S0360-5442(01)00017-2
- Zhang, Y. J., Peng, Y. L., Ma, C. Q., & Shen, B. (2017). Can environmental innovation facilitate carbon emissions reduction? Evidence from China. Energy Policy, 100, 18–28. https://doi.org/10.1016/j.enpol.2016.10.005