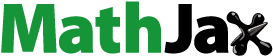
Abstract
The global temperature has been on the rise in recent times. Due to the worsening environmental quality and greenhouse gas emissions, countries around the globe are looking for energy productivity. Various countries have successfully promoted cleaner technologies. This study empirically examines the determinants of energy productivity for the panel of seven emerging economies during 2004–2019 to understand why some countries are more energy productive compared to others. This study contributes to the previous literature by identifying the new influencing factors for a selected set of emerging countries that help import universal suggestions for improving energy productivity, green growth, and sustainable development. Using an Augmented Mean Group (A.M.G.) approach, the results suggest that financial inclusion (F.I.N.I.N.C.), globalisation (G.L.B.), human capital index (H.C.I.), composite risk index (C.R.I.) and income are important factors contributing to energy productivity in sample countries. Specifically, an increase in one unit's F.I.N.I.N.C. brings about a 3% increase in the value of energy productivity. Hence, we conclude that a well-functioning financial system is important in achieving sustainable development goals (S.D.G.s).
1. Introduction
Continuous industrialisation and rapid output growth have harmed the world's environmental sustainability. The Earth's heat balance has become unbalanced as a result of the emission of high-temperature gases (Su et al., Citation2020). Various countries have successfully promoted energy saving technologies to combat environmental challenges. To increase the output with sustainable environment, countries use the concept of energy productivity, which means using less energy to perform the same task (Li et al., Citation2020). Recently, attaining energy productivity has been given global importance due to its significant connotation for green growth and sustainable development. However, measuring energy productivity is a debatable issue. The issue of gauging energy productivity has received much attention from environmentalists and policymakers over the years. The issue has received even more attention in recent years due to its widespread implications for energy, economic, and environmental security.
Energy productivity plays a vital role in improving environmental performance (Huang et al., Citation2017; Ji et al., Citation2021). Nevertheless, several authors argue that focusing on energy productivity may not inevitably lead to abating carbon emissions due to the fact that improvement in energy productivity may decrease the energy prices, which in turn increase energy consumption (Hanley et al., Citation2009). To gauge energy productivity, researchers used energy intensity for many years, which is the reciprocal of energy productivity. Nevertheless, one of the limitations of using energy intensity is that it does not capture the real change in the sectoral energy productivity. The debate regarding the concerning factors of energy productivity is still going on. A bulk of research is carried out to identify the potential determinants of energy productivity (Bloch et al., Citation2015; Carvalho, Citation2016; Lee & Chien, Citation2010; Shahbaz & Feridun, Citation2012; Sineviciene et al., Citation2016; Zhang, Citation2013). Fisher-Vanden et al. (Citation2004) and Shahbaz et al. (Citation2013) identified two main channels through which energy productivity changes. The first is a sectoral effect, which is the changes in the sectoral energy productivity due to changes in technological methods, behavioural changes, and changes in product mix. The second is the structural effect, which is the aggregate energy productivity changes due to the structural shift of the economy from less productive sectors to more productive sectors such as financial services.
There is a dire need to address the problem of climate change and provide access to affordable, clean energy services. However, a low carbon energy system transition requires enough financial resource. A well-functioning financial system is important in achieving sustainable development goals (S.D.G.s). Countries with a better financial system can get funds and offer innovative financing. Financial inclusion (F.I.N.I.N.C.) represents the accessibility of households to a range of financial services (Kim & Park, Citation2016). F.I.N.I.N.C. aims to increase the accessibility of businesses and individuals to financial products and services at an affordable and sustainable cost. An increase in F.I.N.I.N.C. leads to increased saving and investment, promoting economic growth. Economic growth is generally linked with high energy demand. Hence, there is a positive linkage between F.I.N.I.N.C. and energy consumption (Zhang, Citation2013). However, Ziolo et al. (Citation2019) argue that financial development can achieve the social, economic and environmental aspects of sustainable development. Shahbaz et al. (Citation2015) argue that financial development can reduce energy demand through energy efficiency if funds received from financial institutions are invested in low energy intensive technologies. On the same analogy, it can be argued that F.I.N.I.N.C., an integral dimension of financial development, is linked with improved energy efficiency, less energy intensity and high energy productivity. Moreover, financial deepness supports businesses in increasing their R&D expenditures and producing less energy saving commodities, which reduce energy demand (Kim & Park, Citation2016). Due to different opinions regarding the nexus between F.I.N.I.N.C. and energy productivity, it is imperative to analyze the impact of F.I.N.I.N.C. on energy productivity, which is the main concern of this study.
Therefore, this endeavour empirically examines the deep determinants of energy productivity for the panel of seven emerging economies during 2004–2019 to understand why some countries are more energy productive compared to others. Identifying the determinants of energy productivity is important in two meaningful ways; first, countries worldwide are setting the targets of doubling energy productivity in the upcoming years. Hence, to achieve the target of doubling energy productivity, it is imperative to identify the influential factors of energy productivity. Second, it is widely accepted that energy productivity has implications for global environmental sustainability and hence, identifying the factors is of significant importance for policymakers. We include F.I.N.I.N.C. (F.I.N.I.N.C.), globalisation (G.L.B.), composite risk index (C.R.I.), human capital and G.D.P. as important explanatory variables in the model due to their strong linkage with aggregate energy productivity.
The emerging seven (E7) countries are in the process of transitioning their industrial structures to more sustainable energies. These seven countries are investing in cost-saving technologies to achieve this goal. China, Russia, and Turkey outperform the other E7 countries in terms of energy productivity because they have more resources to upgrade their technologies. Other E7 countries, such as India, Brazil, Mexico, and Indonesia, have not achieved impressive levels of energy productivity. The financial system of the E7 countries could be one of the reasons for their disparity in achieving energy productivity. A well-functioning financial system is critical to accomplishing long-term development goals (S.D.G.s). If monies obtained from financial institutions are invested in low energy intensive technology, a robust financial system can reduce energy demand through energy productivity (Shahbaz et al., Citation2015). The socioeconomic situations of the E7 countries varied greatly as well. In terms of growth, China and India account for the majority of the E7's growth. Hence, different factors might be responsible for energy productivity in these countries. The E7 countries clearly exceed the developing countries in terms of productivity growth. However, it is debatable whether the higher productivity and income development in the E7 countries can be linked to greater F.I.N.I.N.C.. Furthermore, the E7 countries invest billions of dollars each year in cost-cutting technologies. As a result, it is critical to investigate the relationship between F.I.N.I.N.C. and energy productivity. This study empirically investigates the impact of F.I.N.I.N.C. on energy productivity across E7 countries (such as China, India, Brazil, Mexico, Russia, Indonesia and Turkey) from 1990 to 2017. This study adds to the previous literature by investigating a new set of potential energy productivity variables such as F.I.N.I.N.C., G.L.B., risk, and human capital. Income, R&D investment, energy prices, and growth are all seen as key factors of technological innovation in the extant research. However, F.I.N.I.N.C. has never been studied as a driver of energy productivity. Furthermore, this study uses G.L.B., human capital, and risk as novel determinants of energy production. Furthermore, this initiative employs advanced econometric techniques that outperform traditional econometric methods.
The next section presents the review of previous studies on the responsible factors of energy productivity. Section three presents the theoretical background, data, models and analytical strategy to estimate the models. Section four presents the results and corresponding discussions. The last section concludes the findings and import policies.
2. Literature review
The issue of energy productivity is well explained in the literature. The literature well documented that energy productivity is linked with green growth and sustainable development (Hao et al., Citation2021; Rodríguez et al., Citation2018). Moreover, the literature on the possible influencing factors of energy productivity is also rich (Bloch et al., Citation2015; Haggar, Citation2012; Hwang & Yoo, Citation2014; Lee, Citation2006; Lee & Chien, Citation2010; Metcalf, Citation2008; Shahbaz et al., Citation2013; Shahbaz & Feridun, Citation2012; Sineviciene et al., Citation2016; Soytas & Sari, Citation2003; Zhang, Citation2013). The literature examining the determinants of energy productivity can be categorised into three groups. The first group studies the effect of economic growth in the form of per capita income and energy prices on energy productivity (Bloch et al., Citation2015; Haggar, Citation2012; Hang & Tu, Citation2007; Hwang & Yoo, Citation2014; Metcalf, Citation2008). The second group considers the impact of multiple arrays of control variables on energy productivity (Carvalho, Citation2016; Cornillie & Fankhauser, Citation2004; Fisher-Vanden et al., Citation2004; Lee & Chien, Citation2010; Nepal et al., Citation2014; Shahbaz & Feridun, Citation2012; Shahbaz et al., Citation2013; Sineviciene et al., Citation2016; Soytas & Sari, Citation2003; Zhang, Citation2013). Among others, energy prices, structural and technological changes, institutional reforms are identified as the most important determinants of energy productivity. In the literature, two main proxies are used to capture energy efficiency; energy intensity and energy productivity. Energy productivity has received much importance in recent years as it is directly linked with sustainable development.
The research is scant on the role of F.I.N.I.N.C. in affecting energy productivity. Studies so far, have investigated the impact of financial development on energy consumption (Baloch et al., Citation2019; Danish et al., Citation2018), on energy intensity (Canh et al., Citation2020), on economic development (Ohene-Asare et al., Citation2020). Moreover, studies are carried out to investigate several variables' impact on F.I.N.I.N.C.. In the literature, F.I.N.I.N.C. is linked with financial efficiency (Charfeddine & Kahia, Citation2019; Demirgüç-Kunt et al., Citation2017), economic growth (Kim et al., Citation2018; Sharma, Citation2016) and environmental degradation (Tamazian et al., Citation2009). Further, studies have also been carried out to examine the impact of F.I.N.I.N.C. on energy poverty (Bruhn & Love, Citation2014; Koomson & Ibrahim, Citation2018; Koomson et al., Citation2020). The main argument behind the nexus between F.I.N.I.N.C. and energy poverty is that an increase in F.I.N.I.N.C. leads to accessibility of households to a wide range of financial services such as credit and insurance, which leads to an increase in income the households. An increase in income ultimately leads to a decrease in energy poverty. Hence, larger F.I.N.I.N.C. has the potential of achieving more inclusive growth (larger F.I.N.I.N.C. has the potential of achieving more inclusive growth (UNDP, 2016) and can lower energy poverty (Koomson et al., Citation2020). In the literature, financial development is widely recognised as the influencing factor of energy consumption (Sadorsky, Citation2010; Zhang, Citation2011). A well-functioning financial system enables households to acquire funds for businesses at a low cost, which helps them increase the production scale and, hence, increase energy consumption. Moreover, Kim and Park (Citation2016) argue that the introduction of renewable energies diversifies the energy mix, leading to an increase in energy security. However, a deep financial system is a pre-requisite to deploying renewable energies. Providing easier bank loans and equity financing is an effective way to promote renewable energies. Moreover, F.I.N.I.N.C. increases households' access to credit, enabling them to increase energy demand by purchasing more energy commodities. Contrary to the above argument, Tamazian et al. (Citation2009), Kim and Park (Citation2016) and Hermes and Lensink (Citation2003) argue that a deep financial system reduces energy demand as it lessens the financial constraints on businesses which enable them to deploy advanced technologies, which in turn improve energy efficiency. The deployment of low-carbon advanced energy technologies is generally linked with low energy intensity and high energy productivity (International Renewable Energy Agency [IRENA] and Copenhagen Centre of Energy Efficiency [C2E2], Citation2015).
In the existing literature, globalisation (G.L.B.) is generally linked with economic growth (Ali & Malik, Citation2021), environmental quality (Shahbaz et al., Citation2015), technological changes (Chang & Lu, Citation2012; Filippaios & Kottaridi, Citation2013; Keller, Citation2010; Miremadi et al., Citation2019) and sustainable development (Tang et al., Citation2020). However, its link with energy productivity is missing. Few studies are conducted to establish the link between G.L.B. and energy consumption and sustainable development. Rees (Citation2002) investigated the relationship between G.L.B.and sustainable development and found that G.L.B. has increased the exploitation of natural resources on a large scale for production of industrial goods and, hence, leads to unsustainable development. Soytas et al. (Citation2007) investigated the relationship between G.L.B. and energy consumption and found that G.L.B. boosts F.D.I. and leads to an increase in output as well as a spillover of technologies across the borders, which causes higher energy consumption. However, Shahbaz et al. (Citation2016) argue that G.L.B. causes a decline in energy demand because G.L.B. improves investment in energy saving technologies with strict regulations, which lead to an increase in energy efficiency. This study hypothesises that ‘globalization improves energy productivity. On the role of globalization in affecting energy efficiency, the research is rich’ (Boqiang & Hongxun, Citation2015; Farrow et al., Citation2018; Meng et al., Citation2015; Moreau & Vuille, Citation2018; Pan et al., Citation2019; Shah et al., Citation2019; Xing et al., Citation2021; Yao et al., Citation2020; Zhang et al., Citation2017; Zhao & Lin, Citation2019). It is widely accepted that advanced energy-saving technologies can be transformed across borders through international trade and F.D.I. Hence, the process of G.L.B., on the one hand, causes fewer carbon emissions by exposure to eco-innovative technologies and, on the other hand, leads to improvement in energy efficiency due to the adaptation of advanced energy saving technologies. Xing et al. (Citation2021) argue that trade along with technological progress is an effective way to improve energy efficiency. Hence, the existing literature is agreed on the positive impact of G.L.B. on energy efficiency. However, the major limitation of the previous studies is that these studies used energy intensity and total energy efficiency factors (Dan, Citation2002; Wu & Shao, Citation2016). Moreover, most of these studies used the relationship between G.L.B. and energy efficiency for the industrial sectors of different countries. However, G.L.B. contributes to improved energy productivity is a topic of great concern, which is missing in the previous literature.
To sum up, there is a gap in the literature on the role of F.I.N.I.N.C., G.L.B., C.R.I., and human capital in affecting energy productivity. The main contribution of this study is the identification of new influencing factors for a selected set of emerging countries that helps to import universal suggestions for the improvement of energy efficiency, green growth and sustainable development. This is the first attempt to investigate the potential relationship between energy productivity and F.I.N.I.N.C., G.L.B., the C.R.I., and human capital to the best of our knowledge. Until recently, no study has examined the influence of the C.R.I. and human capital on energy production. Even though energy productivity and energy intensity are equal metrics from a different perspective, earlier research focused more on the influence of energy efficiency on environmental sustainability. Furthermore, previous research has used energy intensity as an indicator of energy efficiency. However, because energy productivity offers a better route forward, this study uses energy productivity to capture energy efficiency.
3. Methodology
3.1. Theoretical background
The theoretical foundation for the relationship between energy productivity and its determinants such as F.I.N.I.N.C., G.L.B., risk, G.D.P. and H.C.I. is depicted in . Energy productivity, which is G.D.P. per unit of total primary energy supply. Hence, it is an energy-adjusted estimate that considers output and energy supply simultaneously. Energy productivity is a more accurate indicator to represent energy efficiency and is considered a more reliable metric to represent a country's energy efficiency. It is argued that energy productivity is more aligned with the generally understood idea of energy efficiency, as both calculate output divided by input. Energy productivity, which is the ratio of output per unit of energy use, is a measure that depicts the country's relative performance in terms of economic and environmental issues. Hence, energy productivity measures the amount of energy consumed for producing one unit of output (Atalla & Bean, Citation2017; Wurlod & Noailly, Citation2018).
F.I.N.I.N.C. suggests more financial products and services are available to a broad range of people. F.I.N.I.N.C., which is an integral dimension of financial development, is generally linked with improved energy efficiency. The theoretical underpinning behind the positive impact of F.I.N.I.N.C. on energy productivity is that with the increase in the availability of funds, the probability of investment of these funds in low energy intensive technologies increase. The increased investment in low energy intensive technologies further improved energy productivity. Moreover, it is also argued that increased F.I.N.I.N.C. also increases the R&D investment, which has implications for energy efficiency and energy productivity. Hence, improved F.I.N.I.N.C. means deepening the financial system and improved energy productivity.
It is expected that G.L.B. is positively related to energy productivity. The process of G.L.B. enables countries to transfer modern energy saving technologies through imports and F.D.I. More output can be produced by adopting these technologies from consuming less energy (improvement in energy productivity). Hence, following Xing et al. (Citation2021), Yao et al. (Citation2020), Pan et al. (Citation2019) and Zhao and Lin (Citation2019), we expect a positive impact of G.L.B. on energy productivity. Due to the high political, economic and financial risks, firms barely meet their requirement of paying back the loans. Hence, financial institutions are reluctant to finance costly energy-saving, technologically innovative projects. Improving the composite risk may bring a positive spillover effect and hence, helps improve energy productivity. Therefore, improved political, economic and financial risks (improved C.R.I.) are positively related to energy productivity.
Based on the theoretical framework, the model specification is given as:
(1)
(1)
The set of panel data comprising of seven cross-sections ‘’, i.e., seven emerging economies including Russia, India, Indonesia, China, Mexico, Turkey and Brazil, from 2004 to 2019. We investigate the impact of F.I.N.I.N.C., G.L.B., and C.R.I. on energy productivity in the initial stage. Here,
is for energy productivity, which is G.D.P. per unit of Total primary energy supply. The data is acquired from OECD (Citation2020). F.I.N.I.N.C. is an index calculated by using variables such as institutions of commercial banks, branches, bank deposits and loans, and numbers of A.T.M.S. C.R.I. covers all three political, economic and financial risk indices. The data on F.I.N.I.N.C. and C.R.I. are from IMF (2020). G.L.B., which is a composite index of three sub-indices.
The data is obtained from Gygli et al. (Citation2019). In the next stage, we extend the model by including:
(2)
(2)
where, H.C.I. represents Human Capital Index and is obtained from Penn World Table 10.0.
Finally, we extend the model by including the variable G.D.P. Improved human capital implies more awareness of the general masses regarding efficient energy use. Hence, improved human capital is positively related with increased energy productivity
(3)
(3)
Next, we include G.D.P. as a new explanatory variable in the model. The increase in output (increased G.D.P.) may enable countries to invest in cost saving technologies ad shift their industrial structure to more sustainable energies. It is widely accepted that rich countries are more able to improve their energy efficiency because they have more resources to improve their technologies. Hence, high income level is positively related to increased energy productivity.
3.2. Analytical techniques
3.2.1. Slope heterogeneity and cross-section dependence
The conventional panel data methods assume the panel members to be cross-Sectionally independent, which raises several econometric problems (Ali & Malik, Citation2021). The introduction of second-generation methods explicitly considers the correlation across members of the panel (Pesaran, Citation2007). Hence, testing C.S.D. is an important step to further employing unit root and cointegration methods. The increasing interaction of countries and a policy shock in one series in one country may have severe consequences for other countries (Khan et al., Citation2020). Ignoring C.S.D. and slope heterogeneity lead to inconsistent estimates; hence, it is crucial to check the C.S.D. and slope heterogeneity (Sarafidis & Wansbeek, Citation2012). For that reason, to obtain some consistent estimates, this study employs C.D. test developed by Pesaran (Citation2021). Moreover, the study uses slope heterogeneity introduced by Pesaran and Yamagata (Citation2008).
3.2.2. Unit root tests
In the literature, several panel unit root tests have been used, taking into account the assumption of C.S.D. This study uses the Cross Sectionally Augmented IPS (C.I.P.S.) test, popularised by Pesaran (Citation2007). The test allows C.S.D. and also deals with S.H. The general form for the regression is given below as:
(4)
(4)
where, V represents the cross-section averages. The t-ratio of the coefficient of Xi, t-1 in EquationEquation (7)
(7)
(7) . is the ADF for the i-th cross sectional unit and that is supposed to be CDFi. Using CDF values, we get the CIPS statistic:
(5)
(5)
3.2.3. Westerlund (2007) cointegration
To examine the relationship between energy productivity (E.P.) and its determinants such as F.I.N.I.N.C., G.L.B., C.R.I., H.C.I. and income (proxied by G.D.P.), for the E7 countries, this endeavour employs Westerlund (Citation2007) test. The test came as a response to identifying cointegration by taking into account structural dynamics instead of residual dynamics, thus, eliminating any common factor restriction. Westerlund (Citation2007) method is vital as it provides consistent results in the wake of SH and CSD (Kapetanios et al., Citation2011). The test uses the following group-mean and panel statistics:
(6)
(6)
(7)
(7)
(8)
(8)
(9)
(9)
Where represents the standard error of
. Westerlund (Citation2007) test can calculate the speed at which long run equilibrium is restored.
3.2.4. Augmented mean group (A.M.G.)
The next step is to find out the cointegration vector in order to examine the role of F.I.N.I.C., G.L.B., C.R.I., H.C.I. and G.D.P. in determining energy productivity in E7 countries. To do so, this study uses the augmented mean group (A.M.G.) by Eberhardt (Citation2012). The test offers consistent results in the presence of endogeneity and C.S.D. The first generation cointegration techniques consider the cross sections independent of each other (Khan et al., Citation2021). But, factors such as G.L.B., F.I.N.I.N.C., F.D.I., etc. may cause a strong correlation between cross sections error terms, which may let the assumption of cross-sectional independency by conventional cointegration techniques face solid criticism. Since this study explores the relationship between energy productivity, F.I.N.I.N.C., and G.L.B. in the E7 countries, any global shock will affect these factors interdependently. Such unseen common factors can affect energy productivity. The A.M.G. equations for all three models are given as:
(10)
(10)
(11)
(11)
(12)
(12)
The cross-sectional averages are denoted by s.
3.2.5. Panel causality test
The causal relationship among energy productivity with F.I.N.I.N.C, G.L.B., C.R.I., H.C.I. and G.D.P. has been found by employing the panel granger causality test of Dumitrescu and Hurlin (Citation2012). These techniques have received much consideration for variables having both cross sectional (N) and time series dimensions (T). The test provides consistent results in the presence of C.S.D. and SH. The test's equation is given as:
(13)
(13)
The autoregressive parameters are shown by
4. Results and discussions
presents the results of C.S.D. and S.H. tests. It is evident from the upper part of the table that all three models suffer from S.H. problem. The significant delta and adjusted delta statistics confirm our claim. Moreover, the significant C.S.D. statistics confirm the dependency of variables across countries, i.e., any shock observed in any of the E7 economies will be propagating to the other countries. Hence, we find that all E7 countries are interconnected and depend on each other.
Table 1. Slope heterogeneity and cross-section dependence.
presents the C.I.P.S. results. It is evident that null hypothesis of non-stationarity is rejected for all variable E.P., F.I.N.I.N.C., G.L.B., H.C.I., and G.D.P. at a 5% level of significance. Hence, the results of same order of integration of variables lead us to employ Westerlund (Citation2007) and A.M.G. methods ().
Table 2. Pesaran (Citation2007) unit root test.
Table 3. Westerlund (Citation2007) cointegration.
presents the results of Westerlund (Citation2007) test. It is confirmed that the variable energy productivity (E.P.) is cointegrated with its determinants such as F.I.N.I.C., G.L.B., C.R.I., H.C.I. and G.D.P. for the E7 countries. The long run relationship is evident in all three models. Hence, adding an additional variable of HCI in model 2 and further by G.D.P. in model 3 does not accept the null hypothesis of no cointegration. The group mean statistics Gτ and Gα and presented in the first two columns of , whereas the next two columns represent the panel statistics Pτ and Pα. It is, therefore, summarised that any temporary disequilibrium in the short run in all three models of energy productivity is adjusted back in the long run.
Table 4. AMG results.
Table 4. Dumitrescu Hurlin panel causality.
After confirmation of the long run equilibrium, the next step is to find out the long run estimates. The A.M.G. method is employed to serve this purpose. presents the results of the A.M.G. for the F.I.N.I.N.C.-energy productivity nexus. The A.M.G. estimates of model 1 are given in the second column of . Column two (Model 1) presents the impact of F.I.N.I.N.C., G.L.B. and C.R.I. on energy productivity, whereas column 3 (model 2) represents the impact of H.C.I. (along with the explanatory variables of model 1) on energy productivity. Column 4 (model 3) represents the impact of G.D.P. (along with the explanatory variables of model 2) on energy productivity. The results confirm that F.I.N.I.N.C. positively and significantly impacts energy productivity in all three models. According to model 1, a one-unit increase in F.I.N.I.N.C. brings about a 0.03 unit increase in the value of energy productivity. The magnitude of the coefficient of F.I.N.I.N.C. is greater in model 1 as compared to other 2 models. According to current estimates, F.I.N.I.N.C. is positively related to energy productivity in the E7 countries, supporting the argument that F.I.N.I.N.C. can be used as an important tool to successfully improve energy productivity by reducing energy demand and investing in energy-saving efficient technologies. As an inherent component of financial development, F.I.N.I.N.C. enables firms and people to invest in green technologies and purchase energy-efficient products, resulting in increased energy productivity and sustainability. As a result of increased F.I.N.I.N.C., businesses and households behave more sustainably. According to the World Bank, F.I.N.I.N.C. is a critical facilitator of the United Nations S.D.G.s’ ‘Affordable and Clean Energy’ aim. The positive impact of financial deepening on energy productivity can also be seen in the studies of Kim and Park (Citation2016), Danish et al. (Citation2018) and Demirgüç-Kunt et al. (Citation2017).
The results further show that G.L.B. is positively related to energy productivity in all three models. Similar to the coefficient of F.I.N.I.N.C., the size of the parameter of G.L.B. is also greater in model 1 as compared to other 2 models (0.499***> 0.430**> 0.371***). It implies that adding additional variables in the model decrease the impact of G.L.B. on energy productivity. A one-unit increase in G.L.B. brings about a 0.49, 0.43 and 0.37 unit increase in the value of energy productivity in model 1, 2 and 3, respectively. The positive impact of G.L.B. on energy productivity in E7 countries needs an explanation. Since, E7 countries are actively involved in trade with other countries; they may import energy-saving efficient technologies from other countries, which contribute to energy productivity. These findings agree with those of Xing et al. (Citation2021), Pan et al. (Citation2019) and Zhao and Lin (Citation2019). Since imports and FDI are two fundamental parts of G.L.B., governments can obtain energy-saving technology from other countries and substitute them for inefficient domestic energy technologies. More output can be produced using less energy by implementing these energy-saving technologies.
The results further show that energy productivity is stimulated by improving C.R.I. in all three models. Similar to the coefficient of C.R.I., the magnitude of the coefficient of C.R.I. is also greater in model 1 than the other two models. It implies that adding additional variables in the model decrease the impact of C.R.I. on energy productivity. A one-unit increase in F.I.N.I.N.C. brings about a 0.36 unit increase in the value of energy productivity in model 1. The positive impact of the C.R.I. on energy productivity in E7 countries needs an explanation. The C.R.I. comprises indicators for political, economic, and financial concerns. High composite risk makes it difficult for enterprises to repay their debts, and as a result, banks are hesitant to finance high-cost projects. A reduction in these hazards has a positive spillover effect, and so energy productivity can be increased.
The results further show that the H.C.I. is positively related to energy productivity in models 2 and 3. A one unit increase in H.C.I. causes 0.10 unit and 0.15 unit increase in energy productivity in models 2 and 3, respectively. The H.C.I. enhances energy productivity by raising knowledge among residents and entrepreneurs about the efficient use of energy. As a result, productivity can be boosted by swapping energy-saving technology for inefficient energy technologies. According to current estimations, human capital development may be used as an effective technique to successfully improve energy productivity by reducing energy consumption and investing in energy-efficient equipment.
The results further show that G.D.P. is positively related to energy productivity (model 3). A one unit increase in G.D.P. leads to 1.15 unit increase in energy productivity. The positive impact of G.D.P. on energy productivity suggests that rich countries are better equipped to invest in energy-saving technologies and greater energy efficiency. Overall, the study concludes that E7 countries may enhance their energy productivity by improving F.I.N.I.N.C., G.L.B., the C.R.I., the H.C.I., and G.D.P.
To check the causal relationship between E.P. and its determinants such as F.I.N.I.C., G.L.B., C.R.I., H.C.I. and G.D.P., we employ the Dumitrescu Hurlin panel causality test. The results show that energy productivity is bi-directionally related to F.I.N.I.C., G.L.B., C.R.I., H.C.I. and G.D.P. Any change in energy productivity in E7 countries has policy implications for F.I.N.I.C., G.L.B., C.R.I., H.C.I. and G.D.P. Moreover, any policy change in the variables F.I.N.I.C., G.L.B., C.R.I., H.C.I. and G.D.P. has will have consequences for energy productivity in E7 countries. These findings suggest that F.I.N.I.N.C., G.L.B., C.R.I., H.C.I. and G.D.P. are important factors causing changes in energy productivity in E7 countries. Nonetheless, any improvement in energy productivity has important implications for F.I.N.I.N.C., G.L.B., C.R.I., H.C.I. and G.D.P.
5. Conclusions and policy implications
Due to the worsening environmental quality and greenhouse gas emissions, countries around the globe are looking for energy efficiency. Energy productivity is a more accurate indicator to represent energy efficiency. The debate regarding the concerning factors of energy productivity is still going on. This study investigates the determinants of energy productivity for the panel of seven emerging economies during 2004–2019 to understand why some countries are more energy productive compared to others. The study uses advanced panel econometric methods, which deal with cross sectional dependency and heterogeneity. The econometric results offer robust results; Equation(1)(1)
(1) all variables are dependent across countries, i.e.. C.S.D.; Equation(2)
(2)
(2) all three models suffer from slope heterogeneity; Equation(3)
(3)
(3) all variables are integrated of same order one; Equation(4)
(4)
(4) in the long run, energy productivity is cointegrated with F.I.N.I.N.C., G.L.B., C.R.I., H.C.I. and G.D.P.; Equation(5)
(5)
(5) the variables F.I.N.I.N.C., G.L.B., C.R.I., H.C.I. and G.D.P. positively affect energy productivity in the long run; (6) any change in variables F.I.N.I.N.C., G.L.B., C.R.I., H.C.I. and G.D.P. has consequences for energy productivity; and (7) any policy change in variable energy productivity has consequences for F.I.N.I.N.C., G.L.B., C.R.I., H.C.I. and G.D.P. This finding suggested that E7 countries have benefited from F.I.N.I.N.C., G.L.B., improvement in C.R.I., human capital development and income by either increasing productivity or reducing energy consumption.
This study suggests that E7 countries should design policies that aim to combat climate change and provide cheap, sustainable energy services in terms of policy implications. However, transitioning to a low-carbon energy system necessitates sufficient financial resources. These seven emerging countries need to transfer funds from projects that emphasise on inefficient energy technologies to projects that use energy saving technologies, i.e., renewable. Hence, through F.I.N.I.N.C., countries can shift the structure of their economies towards more energy productive sectors. Moreover, these seven countries need to minimise the risk. Because of the risks, enterprises rarely achieve their loan repayment requirements, making financial institutions hesitant to finance costly energy-saving technologically advanced initiatives. Improving the composite risk may have a positive spillover effect and so aids in increasing energy productivity. Moreover, our findings imply that E7 countries should use G.L.B. as an effective tool to transfer energy saving technologies from other parts of the world. Further, through creating awareness, E7 countries can opt for energy saving new environmental protection technologies to transform their economies to sustainable energy sources smoothly. Our findings imply that E7 countries should improve their C.R.I. by focusing on economic, financial and political dimensions. An improvement in C.R.I. will enable E7 countries to improve energy productivity.
Disclosure statement
No potential conflict of interest was reported by the authors.
References
- Ali, S., & Malik, Z. K. (2021). Revisiting economic globalization led growth: The role of economic opportunities. Journal of Public Affairs, 21(2), e2193. https://doi.org/10.1002/pa.2193
- Atalla, T., & Bean, P. (2017). Determinants of energy productivity in 39 countries: An empirical investigation. Energy Economics, 62, 217–229. https://doi.org/10.1016/j.eneco.2016.12.003
- Baloch, M. A., Danish, & Fanchen, M. (2019). Modeling the non-linear relationship between financial development and energy consumption: Statistical experience from OECD countries. Environmental Science and Pollution Research, 26, 8838–8846.
- Bloch, H., Rafiq, S., & Salim, R. (2015). Economic growth with coal, oil and renewable energy consumption in China: Prospects for fuel substitution. Economic Modelling, 44, 104–115. https://doi.org/10.1016/j.econmod.2014.09.017
- Boqiang, L., & Hongxun, L. (2015). Do energy and environment efficiency benefit from foreign trade? – The case of China's industrial sectors. Economic Research Journal, 9.
- Bruhn, M., & Love, I. (2014). The real impact of improved access to finance: Evidence from Mexico. The Journal of Finance, 69(3), 1347–1376.
- Canh, N. P., Thanh, S. D., & Nasir, M. A. (2020). Nexus between financial development & energy intensity: Two sides of a coin? Journal of Environmental Management, 270, 1–14. https://doi.org/10.1016/j.jenvman.2020.110902
- Carvalho, A. (2016). Energy efficiency in transition economies: A stochastic frontier approach [CEERP Working Paper no. 4].
- Chang, P.-L., & Lu, C.-H. (2012). Risk and the technology content of FDI: A dynamic model. Journal of International Economics, 86(2), 306–317. https://doi.org/10.1016/j.jinteco.2011.09.001
- Charfeddine, L., & Kahia, M. (2019). Impact of renewable energy consumption and financial development on CO2 emissions and economic growth in the MENA region: A panel vector autoregressive (PVAR) analysis. Renewable Energy, 139, 198–213. https://doi.org/10.1016/j.renene.2019.01.010
- Cornillie, J., & Fankhauser, S. (2004). The energy intensity of transition countries. Energy Economics, 26(3), 283–295. https://doi.org/10.1016/j.eneco.2004.04.015
- Dan, S. (2002). The improvement of energy consumption efficiency in China's economic growth. Economic Research Journal, 49–56.
- Danish, Saud, S., Baloch, M. A., & Lodhi, R. N. (2018). The nexus between energy consumption and financial development: Estimating the role of globalization in Next-11 countries. Environmental Science and Pollution Research, 25(19), 18651–18661.
- Demirgüç-Kunt, A., Klapper, L., & Singer, D. (2017). Financial inclusion and inclusive growth: A review of recent empirical evidence. Policy research working paper series 8040, the World Bank. http://documents.worldbank.org/curated/en/403611493134249446/pdf/WPS8040.pdf
- Dumitrescu, E. I., & C. Hurlin. (2012). Testing for Granger non-causality in heterogeneous 484 panels. Economic Modelling, 29(4), 1450–1460.
- Eberhardt, M. (2012). Estimating panel time-series models with heterogeneous slopes. The Stata Journal: Promoting Communications on Statistics and Stata, 12(1), 61–71. https://doi.org/10.1177/1536867X1201200105
- Farrow, K., Grolleau, G., & Mzoughi, N. (2018). Less is more in energy conservation and efficiency messaging. Energy Policy, 122, 1–6. https://doi.org/10.1016/j.enpol.2018.07.007
- Filippaios, F., & Kottaridi, C. (2013). Complements or substitutes? New theoretical considerations and empirical evidence on the imports and FDI relationship in Central and Eastern European Countries. International Review of Applied Economics, 27(6), 766–797. https://doi.org/10.1080/02692171.2013.826633
- Fisher-Vanden, K., Jefferson, G. H., Liu, H., & Tao, Q. (2004). What is driving China's decline in energy intensity. Resource and Energy Economics, 26(1), 77–97. https://doi.org/10.1016/j.reseneeco.2003.07.002
- Gygli, S., Haelg, F., Potrafke, N., & Sturm, J.-E. (2019). The KOF globalization index-revisited. The Review of International Organizations, 14(3), 543–574. https://doi.org/10.1007/s11558-019-09344-2
- Haggar, M. H. (2012). Greenhouse gas emissions, energy consumption and economic growth: A panel cointegration analysis from Canadian industrial sector perspective. Energy Economics, 34, 358–364.
- Hang, L., & Tu, M. (2007). The impacts of energy prices on energy intensity: Evidence from China. Energy Policy, 35(5), 2978–2988. https://doi.org/10.1016/j.enpol.2006.10.022
- Hanley, N. D., McGregor, P. G., Swales, J. K. & Turner, K. (2009). Do increases in energy efficiency improve environmental quality and sustainability?. Ecological Economics, 68, 692–709.
- Hao, L.-N., Umar, M., Khan, Z., & Ali, W. (2021). Green growth and low carbon emission in G7 countries: How critical the network of environmental taxes, renewable energy and human capital is? Science of the Total Environment, 752, 141853. https://doi.org/10.1016/j.scitotenv.2020.141853
- Hermes, N., & Lensink, R. (2003). Foreign direct investment, financial development and economic growth. Journal of Development Studies, 40(1), 142–163. https://doi.org/10.1080/00220380412331293707
- Huang, J., Du, D., & Tao, Q. (2017). An analysis of technological factors and energy intensity in China. Energy Policy, 109, 1–9. https://doi.org/10.1016/j.enpol.2017.06.048
- Hwang, Y. H., & Yoo, S. H. (2014). Energy consumption, CO2 emissions, and economic growth: Evidence from Indonesia. Quality & Quantity, 48(1), 63–73. https://doi.org/10.1007/s11135-012-9749-5
- International Renewable Energy Agency (IRENA) and Copenhagen Centre of Energy Efficiency (C2E2). (2015). Synergies between Renewable Energy and Energy Efficiency. A Working Paper (Abu Dhabi, IRENA, and Copenhagen, C2E2).
- Ji, X., Umar, M., Ali, S., Ali, W., Tang, K., & Khan, Z. (2021). Does fiscal decentralization and eco-innovation promote sustainable environment? A case study of selected fiscally decentralized countries. Sustainable Development, 29(1), 79–88. https://doi.org/10.1002/sd.2132
- Kapetanios, G., Pesaran, M. H., & Yamagata, T. (2011). Panels with non-stationary multifactor error structures. Journal of Econometrics, 160(2), 326–348. https://doi.org/10.1016/j.jeconom.2010.10.001
- Keller, W. (2010). International trade, foreign direct investment, and technology spillovers. In Handbook of the economics of innovation (pp. 793–829). Elsevier.
- Khan, Z., Ali, S., Dong, K., & Li, R. Y. M. (2021). How does fiscal decentralization affect CO2 emissions? The roles of institutions and human capital. Energy Economics, 94, 105060. https://doi.org/10.1016/j.eneco.2020.105060
- Khan, Z., Ali, S., Umar, M., Kirikkaleli, D., & Jiao, Z. (2020). Consumption-based carbon emissions and international trade in G7 countries: The role of environmental innovation and renewable energy. The Science of the Total Environment, 730, 138945. https://doi.org/10.1016/j.scitotenv.2020.138945
- Kim, D. W., Yu, J. S., & Hassan, M. K. (2018). Financial inclusion and economic growth in OIC countries. Research in International Business and Finance, 43, 1e14–1e14. https://doi.org/10.1016/j.ribaf.2017.07.178
- Kim, J., & Park, K. (2016). Financial development and deployment of renewable energy technologies. Energy Economics, 59, 238–250. https://doi.org/10.1016/j.eneco.2016.08.012
- Koomson, I., & Ibrahim, M. (2018). Financial Inclusion and Growth of Non-farm Enterprises in Ghana. In Financing Sustainable Development in Africa (pp. 369–396). Springer. https://doi.org/10.1007/978-3-319-78843-2_14
- Koomson, I., Villano, R. A., & Hadley, D. (2020). Effect of financial inclusion on poverty and vulnerability to poverty: Evidence using a multidimensional measure of financial inclusion. Social Indicators Research, 25(4), 375–387. https://doi.org/10.1007/s11205-019-02263-0
- Lee, C. (2006). The causality relationship between energy consumption and GDP in G-11 countries revisited. Energy Policy, 34(9), 1086–1093. https://doi.org/10.1016/j.enpol.2005.04.023
- Lee, C., & Chien, M. (2010). Dynamic modelling of energy consumption, capital stock, and real income in G-7 countries. Energy Economics, 32(3), 564–581. https://doi.org/10.1016/j.eneco.2009.08.022
- Li, J., Zhang, X., Ali, S., & Khan, Z. (2020). Eco-innovation and energy productivity: New determinants of renewable energy consumption. Journal of Environmental Management, 271(1), 111028. https://doi.org/10.1016/j.jenvman.2020.111028
- Meng, J., Liu, J., Guo, S., Huang, Y., & Tao, S. (2015). The impact of domestic and foreign trade on energy-related PM emissions in Beijing. Applied Energy, 184, 853–862. https://doi.org/10.1016/j.apenergy.2015.09.082
- Metcalf, G. E. (2008). An empirical analysis of energy intensity and its determinants at the state level. The Energy Journal, 29(3), 1–26.
- Miremadi, I., Saboohi, Y., & Arasti, M. (2019). The influence of public R&D and knowledge spillovers on the development of renewable energy sources: The case of the Nordic countries. Technological Forecasting and Social Change, 146, 450–463. https://doi.org/10.1016/j.techfore.2019.04.020
- Moreau, V., & Vuille, F. (2018). Decoupling energy use and economic growth: Counter evidence from structural effects and embodied energy in trade. Applied Energy, 215, 54–62. https://doi.org/10.1016/j.apenergy.2018.01.044
- Nepal, R., Jamasb, T., & Tisdell, C. A. (2014). Market-related reforms and increased energy efficiency in transition countries: Empirical evidence. Applied Economics, 46(33), 4125–4136. https://doi.org/10.1080/00036846.2014.952894
- OECD. (2020). The world economy on tightrope. OECD Economic Outlook: Statistics and Projection (database).
- Ohene-Asare, K., Tetteh, E. N., & Asuah, E. L. (2020). Total factor energy efficiency and economic development in Africa. Energy Efficiency, 13(6), 1177–1194. https://doi.org/10.1007/s12053-020-09877-1
- Pan, X., Uddin, K., Han, C., & Pan, X. (2019). Dynamics of fi nancial development, trade openness, technological innovation and energy intensity: Evidence from Bangladesh. Energy, 171, 456–464. https://doi.org/10.1016/j.energy.2018.12.200
- Pesaran, M. H. (2007). A simple panel unit root test in the presence of cross‐section dependence. Journal of Applied Econometrics, 22(2), 265–312. https://doi.org/10.1002/jae.951
- Pesaran, M. H. (2021). General diagnostic test for cross-sectional dependence in panel. Empirical Economics, 60(1), 13–38. https://doi.org/10.1007/s00181-020-01875-7
- Pesaran, M. H., & Yamagata, T. (2008). Testing slope homogeneity in large panels. Journal of Econometrics, 142(1), 50–93. https://doi.org/10.1016/j.jeconom.2007.05.010
- Rees, William E. (2002). Socially Just Eco-Integrity: Getting Clear on the Concept. In Peter Miller and Laura Westra (eds.), Just Integrity. Lanham, MD: Rowman and Littlefield.
- Rodríguez, C. M., Ivan, H., & Martin, S. (2018). Environmentally adjusted multifactor productivity: Methodology and empirical results for OECD and G20 countries. Ecological Economics, 153, 147–160. https://doi.org/10.1016/j.ecolecon.2018.06.015
- Sadorsky, P. (2010). The impact of financial development on energy consumption in emerging economies. Energy Policy, 38(5), 2528–2535. https://doi.org/10.1016/j.enpol.2009.12.048
- Sarafidis, V., & Wansbeek, T. (2012). Cross-sectional dependence in panel data analysis. Econometric Reviews, 31(5), 483–531. https://doi.org/10.1080/07474938.2011.611458
- Shah, W. U. H., Yasmeen, R., & Padda, I. U. H. (2019). An analysis between financial development, institutions, and the environment: A global view. Environmental Science and Pollution Research International, 26(21), 21437–21449. https://doi.org/10.1007/s11356-019-05450-1
- Shahbaz, M., & Feridun, M. (2012). Electricity consumption and economic growth empirical evidence from Pakistan. Quality & Quantity, 46(5), 1583–1599. https://doi.org/10.1007/s11135-011-9468-3
- Shahbaz, M., Jam, F. A., Bibi, S., & Loganathan, N. (2015). Multivariate granger causality between CO2 emissions, energy intensity and economic growth in Portugal: Evidence from cointegration and causality analysis. Technological and Economic Development of Economy, 22(1), 47–74. https://doi.org/10.3846/20294913.2014.989932
- Shahbaz, M., Khan, S., & Tahir, M. I. (2013). The dynamic links between energy consumption, economic growth, financial development and trade in China: Fresh evidence from multivariate framework analysis. Energy Economics, 40, 8–21. https://doi.org/10.1016/j.eneco.2013.06.006
- Shahbaz, M., Mallick, H., Mahalik, M. K., & Sadorsky, P. (2016). The role of globalization on the recent evolution of energy demand in India: Implications for sustainable development. Energy Economics, 55, 52–68. https://doi.org/10.1016/j.eneco.2016.01.013
- Sharma, D. (2016). Nexus between financial inclusion and economic growth: Evidence from the emerging Indian economy. Journal of Financial Economic Policy, 8(1), 13e36. https://doi.org/10.1108/JFEP-01-2015-0004
- Sineviciene, L., Draksaite, A., & Melnyk, L. (2016). Research on the relationship between economic growth and energy productivity in Lithuania and Ukraine. In H. Zhang (Ed.), ISSGBM International Conference on Information and Business Management (ISSGBM-IB 2016), 03-04 SEP 2016, Book Series: Lecture Notes in Management Science, vol. 61, pp. 3–8.
- Soytas, U., & Sari, R. (2003). Energy consumption and GDP: Causality relationship in G-7 countries and emerging markets. Energy Economics, 25(1), 33–37. https://doi.org/10.1016/S0140-9883(02)00009-9
- Soytas, U., Sari, R., & Ewing, T. (2007). Energy consumption, income, and carbon emissions in the United States. Ecological Economics, 62, 482–489.
- Su, C.-W., Naqvi, B., Shao, X.-F., Li, J.-P., & Jiao, Z. (2020). Trade and technological innovation: The catalysts for climate change and way forward for COP21. Journal of Environmental Management, 269, 110774. https://doi.org/10.1016/j.jenvman.2020.110774
- Tamazian, A., Chousa, J. P., & Vadlamannati, K. C. (2009). Does higher economic and financial development lead to environmental degradation: Evidence from BRIC countries. Energy Policy, 37(1), 246–253. https://doi.org/10.1016/j.enpol.2008.08.025
- Tang, S., Wang, Z., Yang, G., & Tang, W. (2020). What are the implications of globalization on sustainability? – A comprehensive study. Sustainability, 12(8), 3411. https://doi.org/10.3390/su12083411
- Westerlund, J. (2007). Testing for error correction in panel data. Oxford Bulletin of Economics and Statistics, 69(6), 709–748. https://doi.org/10.1111/j.1468-0084.2007.00477.x
- Wu, X., & Shao, J. (2016). The influence of import openness on manufacturing energy efficiency in China – An empirical study based on tariff reduction. Finance, Trade and Economics, 6.
- Wurlod, J. D., & Noailly, J. (2018). The impact of green innovation on energy intensity: An empirical analysis for 14 industrial sectors in OECD countries. Energy Economics, 71, 47–61. https://doi.org/10.1016/j.eneco.2017.12.012
- Xing, Y., Shah, W. U. H., Yasmeen, R., Zhang, Y., Kamal, M. A., & Khan, A. (2021). The impact of trade on energy efficiency in the global value chain: A simultaneous equation approach. Science of the Total Environment, 765, 142759. https://doi.org/10.1016/j.scitotenv.2020.142759
- Yao, X., Yasmeen, R., Haq Padda, I. U., Hassan Shah, W. U., & Kamal, M. A. (2020). Inequalities by energy sources: An assessment of environmental quality. PLoS One, 15(3), e0230503. https://doi.org/10.1371/journal.pone.0230503
- Zhang, F. (2013). The energy transition of the transition economies: An empirical analysis. Energy Economics, 40, 679–686. https://doi.org/10.1016/j.eneco.2013.09.001
- Zhang, Y. J. (2011). The impact of financial development on carbon emissions: An empirical analysis in China. Energy Policy, 39(4), 2197–2203. https://doi.org/10.1016/j.enpol.2011.02.026
- Zhang, Z., Zhao, Y., Su, B., Zhang, Y., Wang, S., Liu, Y., & Li, H. (2017). Embodied carbon in China’s foreign trade: An online SCI-E and SSCI based literature review. Renewable and Sustainable Energy Reviews, 68, 492–510. https://doi.org/10.1016/j.rser.2016.10.009
- Zhao, H., & Lin, B. (2019). Will agglomeration improve the energy efficiency in China's textile industry: Evidence and policy implications. Applied Energy, 237, 326–337. https://doi.org/10.1016/j.apenergy.2018.12.068
- Ziolo, M., Filipiak, B. Z., Bąk, I., Cheba, K., Tîrca, D. M., & Novo-Corti, I. (2019). Finance, sustainability and negative externalities. An overview of the European context. Sustainability, 11(15), 4249. https://doi.org/10.3390/su11154249