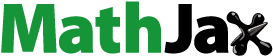
Abstract
This paper aims to investigate the impact of liquidity on the return dynamics between the carbon emission trading market and the stock market in China from 2013 to 2021. In the carbon emission trading market, we find that liquidity on any given day can significantly predict the cross-section returns the next day. Furthermore, we examine the spillover effect between the two markets and find the carbon market has a greater impact on the stock market. We also find evidence that stock market liquidity can significantly improve the liquidity of the carbon market. Finally, we observe that the volatility in the stock market not only deteriorates the liquidity of the stock market but also the carbon market, where the impact for the latter is from decreasing trading volume and increasing prices.
1. Introduction
Currently, climate change is one of the biggest global issues for the entire world. From the emission of carbon dioxide in various countries, greenhouse gases have increased, which poses a large threat to global ecosystems (Jiao et al., Citation2021; Umar et al., Citation2020). This is exactly why the 21st United Nations Climate Change Conference adopted and signed the Paris Agreement. As part of this agreement, countries around the world will reduce greenhouse gas emissions (Umar et al., Citation2020; Yu et al., Citation2022). Since China is the largest developing country and the largest carbon emitter in the world (J. Zheng et al., Citation2019), it has assumed important responsibilities. At the United Nations General Assembly in September 2020, China announced its goal of achieving a carbon peak by 2030 and carbon neutrality by 2060 (Ji et al., Citation2021; Tao et al., Citation2022). For this to occur, the establishment of a national carbon emission trading market is required as a major step in controlling and reducing greenhouse gas emissions in China (Wen et al., Citation2020; Yan et al., Citation2022).
The carbon emissions trading market is a measurement mechanism for carbon emissions to be capped, traded and priced (Oestreich & Tsiakas, Citation2015). It is also a powerful tool to promote economic development, achieve green and low-carbon transformation, strengthen ecological progress, and fulfil international commitments on emission reduction (Bibi et al., Citation2021). Since 2011, China has piloted carbon emission trading markets in seven places, such as Beijing, Tianjin and Shanghai, and an increasing number of companies are participating in the carbon market, which has also attracted a large amount of capital injection (R. Wang et al., Citation2020). Therefore, investors’ have become attracted to the carbon emission as an asset class. Meanwhile, as one of the most fundamental factors in markets, liquidity influences asset prices and other asset characteristics (Ielasi et al., Citation2018; Mirza et al., Citation2020; Umar et al., Citation2021). The quality of liquidity is important for investors in their investment planning.
With the improvement of the financial system, the relationships of all markets have become stronger (Kaiser & Welters, Citation2019; Naqvi et al., Citation2021). The price or return of a specific market is influenced not only by itself but also by other markets (Li et al., Citation2020; Liang et al., Citation2020; Lobato et al., Citation2021). To the best of our knowledge, the stock market plays a role in the economy as a barometer, which reflects the situation of the whole economy therefore the relationship between the stock market and the carbon emission trading market has become an issue of focus (Itani et al., Citation2020).
Recently, there has been some research on the correlation between the carbon emission trading market and the stock market. (Jiménez-Rodríguez, Citation2019) noted that the stock market and the carbon emission trading market are related from a theoretical view. There is an asymmetric relationship between these markets in China (Y. Zheng et al., Citation2021). (Wen et al., Citation2020) stated that there is a positive relation between the carbon emission trading market and stock returns. In addition, some research investigated the risk between the carbon market and stock market (Cong & Lo, Citation2017; Krueger et al., Citation2020; Lin & Wu, Citation2022; Zhu et al., Citation2020). Obviously, the conclusions of these studies are not consistent. We further explore how these two markets affect each other.
In this paper, we investigate the relationship between the carbon emission trading market and the stock market from the perspective of liquidity. We contribute to the literature as follow. First, we enrich the literature on the relationship between the carbon and stock markets. Many studies focus on one market, either the carbon market or the stock market (Bahmani-Oskooee et al., Citation2020; Behrendt & Schmidt, Citation2021; Cong & Lo, Citation2017; Kanamura, Citation2016; Liang et al., Citation2020; Liang et al., Citation2020). Furthermore, this paper sheds light on a new relationship between the carbon and stock markets from the liquidity perspective. We show the spillover effect from the stock market to the carbon market is far greater than anticipated, especially the liquidity spillover effect. Our empirical findings are helpful for investors and companies to better understand and predict the carbon emission trading market and stock market, to enhance market participation, to realize the policy of strengthening the construction of the carbon emission trading market and to help to achieve the goals of ‘peak carbon dioxide emissions’ and ‘carbon neutrality’ in the 14th five-year plan.
The remainder of the paper is organized as follows. Section 2 describes the methodology. Section 3 shows the data of this paper. In addition, Section 4 presents the empirical results. Section 5 presents the extensions. Section 6 ends this paper.
2. Methodology
2.1. Fama-MacBeth regression
Liquidity is a fundamental financial concept that is abstract and difficult to measure (Amihud & Mendelson, Citation1986). Some studies employed alternative indicators of liquidity to conduct research on the stock market from three dimensions, including width, depth and price impact (Amihud, Citation2002; Chordia et al., Citation2005; Fong et al., Citation2017; Goldstein & Kavajecz, Citation2000; Goyenko et al., Citation2009; Rhee & Wang, Citation2009). Based on data available in the carbon market, we mainly adopt the trading volume and illiquidity measurements, where the former captures the depth of liquidity and the latter captures the price impact information.
Following the methodology suggested by (Fama & MacBeth, Citation1973), we test the effect of liquidity on individual carbon market returns using daily frequency data. The cross-sectional model is:
(1)
(1)
where
indicates the return of individual carbon market
on day
is one measure of trading volume (
) and illiquidity of (Amihud, Citation2002) (
).
and
denote the trading volume and the illiquidity for individual carbon market
on day
and both of them take the natural logarithm. In addition, there are total 8 individual carbon emission markets, including Beijing, Shanghai, Guangdong, Shenzhen, Tianjin, Chongqing, Hubei, and Fujian. The regression covers the period of 2013-2021. For each month, we fit the cross-sectional model using the daily data of individual carbon markets before the time-series average for these estimations.
2.2. Vector autoregressive model
We employ the vector autoregressive model designed by (Sims, Citation1980) to estimate the dynamic relationship between the carbon emission trading market and the stock market. This model is an extension of the AR model, which regresses several lagging variables for all variables with all current variables in the model. The vector autoregressive model is thus:
(2)
(2)
where Yt denotes a vector composed of 7 variables: AGGCRet, AGGCVol, AGGCIlliq, MktRV, MKtRet, MktVol and MktIlliq, c is the constant vector, Al expresses the coefficient matrix, and εt represents the residual vector.We then refer to the Bayesian information criterion to determine the lag order, and the lag order is 2.
Based on the above vector autoregressive model, we also conduct the Granger causality analysis to show the spillover effect between the carbon market and the stock market in China. We may infer that there is Granger causality from a given variable (causing variable) to another variable (caused variable) if the null is rejected, where the test is whether the coefficients associated with the 2 lags of the causing variable are jointly 0 in a particular equation with the caused variable as the dependent variable.
3. Data
We use daily data on the carbon market and the stock market, and the sample period is from 18 June 2013 to 12 January 2021, which is obtained from the Oxford Man Institute of Quantitative Finance and the Wind Economic database. Specifically, the extension experiments use weekly data, and the data indicators are uniformly calculated according to the average of daily data within a week.
In addition, the liquidity indicators are presented below. The first indicator is volume (Chordia et al., Citation2001), which is defined as the total volume of the underlying asset during the day. The second indicator is illiquidity of (Amihud, Citation2002), specifically:
(3)
(3)
where
is the simple return of underlying asset i on day
reveals the descriptive statistics of the market variables in this paper. In particular, there are two kinds of market variables, one for the aggregated carbon emission trading market and another for the stock market. The variable AGGCRet represents the carbon market return, AGGCVol indicates the carbon market volume, and AGGCIlliq is the illiquidity of the carbon market. AGGCRet, AGGCVol, and AGGCIlliq are aggregated based on trading-volume weights of 8 individual carbon markets, respectively. In the stock market, MktRV is the realized volatility of the Shanghai Stock Exchange Index, MKtRet, MktVol, and MktIlliq are the return, trading volume, and illiquidity of the Shanghai Stock Exchange Index, respectively.
Table 1. Descriptive statistics for market variables.
Additionally, under the augmented Dickey-Fuller (ADF) statistics (Dickey & Fuller, Citation1981), since the unit root null hypothesis is rejected at the 1% significance level, all variables are stationary.
4. Empirical results
4.1. Liquidity impacts on returns for individual carbon emission trading markets
presents the regression results based on the cross-sectional data of all individual carbon markets from the perspective that carbon market liquidity could influence its return. We mainly explain liquidity through two aspects: trading volume (volume) and price impact (illiquidity).
Table 2. Estimation results of Fama-MacBeth regressions.
The results in show that carbon market volume and illiquidity are both significant when lagging for two periods. The relationship between trading volume and return of the carbon market is consistent with the relationship between volume and price mentioned in (H. Wang & Boatwright, Citation2019). In addition, we test the relationship between carbon market liquidity and returns from the perspective of illiquidity. shows that the coefficient of is significantly positive (0.029) at the 5% level. In contrast, an increase in trading volume
will lead to a decrease in return, which is significantly negative (-0.026) at the 10% level. These results show that individual carbon market’s illiquidity has a stronger impact on its future return than trading volume. In the next section, we continue to explore the properties of the carbon market from the market-level perspective. Moreover, spillover effects between the aggregate carbon market and the stock market in China are investigated by considering several important asset characteristics, including the return, trading volume, illiquidity, and volatility.
4.2. Spillover effects between the carbon market and the stock market
4.3.1. Equation results of the vector autoregressive model
We investigate the specific relationship between the two markets by introducing the 7 market variables into a VAR model with the lag order of 2. shows the VAR’s equation results. We find that the three characteristics of the carbon emission trading market have no significant impact on the realized volatility of the stock market (MktRV). The volume of the carbon emission trading market (AGGCVol) with a one-period lag is significantly and negatively related to the return of the stock market (MktRet) at the 10% level. This demonstrates that the increasing trading volumes in the carbon emission trading market could weakly decrease future returns in the stock market. On the future trading volume of the stock market (MktVol), the one-period lagged trading volume of the carbon emission trading market (AGGCVol) has a negative impact, and the two-period lagged one has a positive impact. Three carbon market variables do not have a significant impact on the illiquidity of the stock market (MktIlliq). This is intuitive because the stock market is more developed and more liquid than the newly established carbon market.
Table 3. Equation results of VAR(2).
For the spillover effect from the stock market to the carbon emission trading market, we find the stock market variables have no significant impact on the future return of the carbon market (AGGCRet). However, the realized volatility, trading volume, and illiquidity of the stock market significantly impact the trading volume of the carbon market, and the realized volatility and trading volume of the stock market also show significant coefficients on the carbon market illiquidity. These results indicate that: first, the stock market liquidity can significantly improve the liquidity of the carbon emission trading market; second, higher stock market volatility deteriorates not only the liquidity within the stock market but also the carbon market liquidity, where the impact for the latter is from two aspects of decreasing trading volume and increasing the price impact.
Within the system of the carbon market, both the trading volume and illiquidity do not predict future aggregate carbon market returns. The relation between liquidity and return at the carbon market level is inconsistent with the previous evidence from individual carbon markets. This suggests that due to the presence of liquidity differences across individual carbon markets, investors will demand additional liquidity compensation in some individual carbon markets that lack liquidity.
Overall, the transmission between the two markets is non-symmetrical from the above evidence, and the spillover effect from the stock market to the carbon market is greater than the one from the carbon market to the stock market. The positive liquidity spillover effect from the stock market to the carbon market could be due to high investor sentiment. Intuitively, when investor sentiment is higher in the stock market, these investors are easier to participate in other asset markets due to their high tolerance, especially when they have profited from the stock market. From the risk perspective, within an interconnected economic system, risks from large asset markets are more easily transmitted to small asset markets. Therefore, the increasing price uncertainty in the stock market also deteriorates the quality of liquidity in the carbon market.
4.3.2. Granger causality based on the vector autoregressive model
The above equation results of VAR show specific impacts of the lagged individual variable on an underlying variable. further presents the Granger causality results between the carbon market and the stock market to directly demonstrate the significance of the overall impact of a market characteristic from the stock market to the carbon market, or from the carbon market to the stock market. This table shows the F-statistics and p-values from the causal correlation for these carbon and stock market variables. In Panel A, we report the results from the stock market to the carbon market and show that the trading volume of the stock market (MktVol) significantly causes the carbon market liquidity, both for illiquidity (AGGCIlliq) and trading volume (AGGCVol). This is consistent with the above findings in specific equation results. The stock market realized volatility (MktRV) also causes the trading volume of the carbon market (AGGCVol) with an F-statistic of 3.217. Panel B reports the results from the carbon market to the stock market. However, F-statistics are not significant only excluding the impact of the carbon market trading volume on the stock market trading volume, where AGGCVol weakly causes MktVol with a p-value of 0.086. These results indicate that the impacts from the carbon market to the stock market are weak.
Table 4. Ganger causality tests from VAR model.
Overall, the spillover effect from the stock market to the carbon market is stronger than the opposite situation, especially for the role of trading activities and price uncertainty of the stock market.
5. Extensions
Our main empirical analysis using daily data expresses the short-term connection between two markets. We further conduct the same analysis with using weekly data to investigate the long-term connection. reports the descriptive statistics of the weekly market variables, and they are all stationary. We fit a VAR(1) model with the 7 market variables by using weekly data. The specific equation results are shown in , and we find there is no significant impact from the return and liquidity of the carbon market to the stock market. The stock market presents a persistently significant impact on the carbon market at a weekly frequency. Specifically, the trading volume of the stock market negatively and significantly predicts the weekly return of the carbon market, where the t-statistic is −2.355. The weekly return of the stock market also has a significant impact on the illiquidity of the carbon market. In particular, in the carbon market, the weekly trading volume and illiquidity positively predict future market returns. Their predictive impact could be driven by different mechanism, specifically, the positive volume-return and illiquidity-return relations could be based on the role of investor sentiment and liquidity compensation, respectively. The Granger causality results in further reveal the impacts from the stock market to the carbon market.
Table 5. Descriptive statistics for market variables: Weekly results.
Table 6. Equation results of VAR(1): Weekly results.
Table 7. Ganger causality tests from VAR model: Weekly results.
In conclusion, the relations between the stock market and the carbon market distinct from the daily and weekly frequencies. The stock market still shows a stronger role in the carbon market from the perspectives of its trading volume and returns.
6. Conclusion
In this paper, we investigate the relationship between the carbon emission trading market and the stock market from the perspective of liquidity. The following are some noteworthy findings. First, within the carbon emission trading market, the illiquidity of the individual carbon markets positively predicts the cross-sectional carbon returns in the next day, and in terms of liquidity depth, the trading volume has a negatively predictive role. Second, considering the relationship between the carbon market and the stock market, we find that the transmission between the two markets is non-symmetrical, and the spillover effect from the stock market to the carbon market is greater than the one from the carbon market to the stock market. Specifically, the trading volume of the stock market can significantly improve the liquidity of the carbon market. In particular, the increasing price uncertainty in the stock market also deteriorates the quality of liquidity in the carbon market. Risks from large asset markets are more easily transmitted to small asset markets.
China is the biggest carbon emitter in the world. Therefore, China has a strong responsibility to manage the carbon emission trading market. This paper investigates the relationship between carbon market liquidity and returns on the basis of price-volume relationship theory (Behrendt & Schmidt, Citation2021). Meanwhile, we further understand the relationship and impact of the carbon emission trading market and the stock market. The flow of capital between the two markets is asymmetric, which is also a concern for investors as market entities. Therefore, it is necessary to scientifically explore carbon prices for policy-makers when adjusting the industrial structure, developing emission reduction technologies, and strengthening environmental governance. At the same time, regulators should pay attention to observations of market liquidity and improve the carbon emission trading system to ensure the stable operation of each market.
At present, the research is mainly about the relationship between the carbon emission trading market and the energy market. Although the markets involved are different, most of the methods are similar (Aatola et al., Citation2013; Gong et al., Citation2021; Hintermann et al., Citation2020; Kanamura, Citation2016; Liang et al., Citation2020; Xu, Citation2021). For future research, the use of intraday high-frequency data and complex nonlinear forecasting models (such as neural network models) to predict prices, returns and volatility between different markets, as well as research on new energy pricing and hedging, may become a possible future research direction.
Disclosure statement
No potential conflict of interest was reported by the authors
References
- Aatola, P., Ollikainen, M., & Toppinen, A. (2013). Impact of the carbon price on the integrating European electricity market. Energy Policy, 61, 1236–1251. https://doi.org/10.1016/j.enpol.2013.06.036
- Amihud, Y. (2002). Illiquidity and stock returns: Cross-section and time-series effects. Journal of Financial Markets, 5(1), 31–56. https://doi.org/10.1016/S1386-4181(01)00024-6
- Amihud, Y., & Mendelson, H. (1986). Asset pricing and the bid-ask spread. Journal of Financial Economics, 17(2), 223–249. https://doi.org/10.1016/0304-405X(86)90065-6
- Bahmani-Oskooee, M., Ghodsi, S. H., & Hadzic, M. (2020). Asymmetric causality between stock returns and usual hedges: An industry-level analysis. The Journal of Economic Asymmetries, 21, e00160. https://doi.org/10.1016/j.jeca.2020.e00160
- Behrendt, S., & Schmidt, A. (2021). Nonlinearity matters: The stock price–trading volume relation revisited. Economic Modelling, 98, 371–385. https://doi.org/10.1016/j.econmod.2020.11.004
- Bibi, A., Zhang, X., & Umar, M. (2021). The imperativeness of biomass energy consumption to the environmental sustainability of the United States revisited. Environmental and Ecological Statistics, 28(4), 821–841. https://doi.org/10.1007/s10651-021-00500-9
- Chordia, T., Roll, R., & Subrahmanyam, A. (2001). Market liquidity and trading activity. The Journal of Finance, 56(2), 501–530. https://doi.org/10.1111/0022-1082.00335
- Chordia, T., Sarkar, A., & Subrahmanyam, A. (2005). An empirical analysis of stock and bond market liquidity. Review of Financial Studies, 18(1), 85–129. https://doi.org/10.1093/rfs/hhi010
- Cong, R., & Lo, A. Y. (2017). Emission trading and carbon market performance in Shenzhen. Applied Energy, 193, 414–425. https://doi.org/10.1016/j.apenergy.2017.02.037
- Dickey, D. A., & Fuller, W. A. (1981). Likelihood ratio statistics for autoregressive time series with a unit root. Econometrica, 49(4), 1057–1072. https://doi.org/10.2307/1912517
- Fama, E. F., & MacBeth, J. D. (1973). Risk, return, and equilibrium: Empirical tests. Journal of Political Economy, 81(3), 607–636. https://doi.org/10.1086/260061
- Fong, K. Y., Holden, C. W., & Trzcinka, C. A. (2017). What are the best liquidity proxies for global research? Review of Finance, 21(4), 1355–1401. https://doi.org/10.1093/rof/rfx003
- Goldstein, M. A., & Kavajecz, K. A. (2000). Eighths, sixteenths, and market depth: Changes in tick size and liquidity provision on the NYSE. Journal of Financial Economics, 56(1), 125–149. https://doi.org/10.1016/S0304-405X(99)00061-6
- Gong, X., Shi, R., Xu, J., & Lin, B. (2021). Analyzing spillover effects between carbon and fossil energy markets from a time-varying perspective. Applied Energy, 285, 116384. https://doi.org/10.1016/j.apenergy.2020.116384
- Goyenko, R. Y., Holden, C. W., & Trzcinka, C. A. (2009). Do liquidity measures measure liquidity? Journal of Financial Economics, 92(2), 153–181. https://doi.org/10.1016/j.jfineco.2008.06.002
- Hintermann, B., Peterson, S., & Rickels, W. (2020). Price and market behavior in phase ii of the EU ETS: A review of the literature. Review of Environmental Economics and Policy, 10(1), 108–128.
- Ielasi, F., Rossolini, M., & Limberti, S. (2018). Sustainability-themed mutual funds: An empirical examination of risk and performance. The Journal of Risk Finance, 19(3), 247–261. https://doi.org/10.1108/JRF-12-2016-0159
- Itani, R., Azeem, M., & Mirza, N. (2020). Arab Spring and COVID-19: Ex post facto examination of the Lebanese banking sector (the contemporary stakeholder analysis). Banks and Bank Systems, 15(4), 121–136. https://doi.org/10.21511/bbs.15(4).2020.11
- Ji, X., Zhang, Y., Mirza, N., Umar, M., & Rizvi, S. K. A. (2021). The impact of carbon neutrality on the investment performance: Evidence from the equity mutual funds in BRICS. Journal of Environmental Management, 297, 113228.
- Jiao, Z., Shahid, M. S., Mirza, N., & Tan, Z. (2021). Should the fourth industrial revolution be widespread or confined geographically? A country-level analysis of fintech economies. Technological Forecasting and Social Change, 163, 120442. https://doi.org/10.1016/j.techfore.2020.120442
- Jiménez-Rodríguez, R. (2019). What happens to the relationship between EU allowances prices and stock market indices in Europe? Energy Economics, 81, 13–24. https://doi.org/10.1016/j.eneco.2019.03.002
- Kaiser, L., & Welters, J. (2019). Risk-mitigating effect of ESG on momentum portfolios. The Journal of Risk Finance, 20(5), 542–555. https://doi.org/10.1108/JRF-05-2019-0075
- Kanamura, T. (2016). Role of carbon swap trading and energy prices in price correlations and volatilities between carbon markets. Energy Economics, 54, 204–212. https://doi.org/10.1016/j.eneco.2015.10.016
- Krueger, P., Sautner, Z., & Starks, L. T. (2020). The importance of climate risks for institutional investors. The Review of Financial Studies, 33(3), 1067–1111. https://doi.org/10.1093/rfs/hhz137
- Li, Y., Liang, C., Ma, F., & Wang, J. (2020). The role of the IDEMV in predicting European stock market volatility during the COVID-19 pandemic. Finance Research Letters, 36, 101749.
- Liang, C., Ma, F., Li, Z., & Li, Y. (2020). Which types of commodity price information are more useful for predicting US stock market volatility? Economic Modelling, 93, 642–650. https://doi.org/10.1016/j.econmod.2020.03.022
- Liang, C., Tang, L., Li, Y., & Wei, Y. (2020). Which sentiment index is more informative to forecast stock market volatility? Evidence from China. International Review of Financial Analysis, 71, 101552. https://doi.org/10.1016/j.irfa.2020.101552
- Liang, C., Wei, Y., Li, X., Zhang, X., & Zhang, Y. (2020). Uncertainty and crude oil market volatility: New evidence. Applied Economics, 52(27), 2945–2959. https://doi.org/10.1080/00036846.2019.1696943
- Lin, B., & Wu, N. (2022). Will the China’s carbon emissions market increase the risk-taking of its enterprises? International Review of Economics & Finance, 77, 413–434. https://doi.org/10.1016/j.iref.2021.10.005
- Lobato, M., Rodríguez, J., & Romero, H. (2021). A volatility-match approach to measure performance: The case of socially responsible exchange traded funds (ETFs). The. The Journal of Risk Finance, 22(1), 34–43. https://doi.org/10.1108/JRF-04-2020-0066
- Mirza, N., Reddy, K., Hasnaoui, A., & Yates, P. (2020). A Comparative Analysis of the Hedging Effectiveness of Farmgate Milk Prices for New Zealand and United States Dairy Farmers. Journal of Quantitative Economics, 18(1), 129–142. https://doi.org/10.1007/s40953-019-00172-0
- Naqvi, B., Mirza, N., Rizvi, S. K. A., Porada-Rochoń, M., & Itani, R. (2021). Is there a green fund premium? Evidence from twenty seven emerging markets. Global Finance Journal, 50, 100656. https://doi.org/10.1016/j.gfj.2021.100656
- Oestreich, A. M., & Tsiakas, I. (2015). Carbon emissions and stock returns: Evidence from the EU Emissions Trading Scheme. Journal of Banking & Finance, 58, 294–308. https://doi.org/10.1016/j.jbankfin.2015.05.005
- Rhee, S. G., & Wang, J. (2009). Foreign institutional ownership and stock market liquidity: Evidence from Indonesia. Journal of Banking & Finance, 33(7), 1312–1324. https://doi.org/10.1016/j.jbankfin.2009.01.008
- Sims, C. A. (1980). Macroeconomics and reality. Econometrica, 48(1), 1–48. https://doi.org/10.2307/1912017
- Tao, R., Su, C.-W., Naqvi, B., & Rizvi, S. K. A. (2022). Can Fintech development pave the way for a transition towards low-carbon economy: A global perspective. Technological Forecasting and Social Change, 174, 121278. https://doi.org/10.1016/j.techfore.2021.121278
- Umar, M., Ji, X., Kirikkaleli, D., Shahbaz, M., & Zhou, X. (2020). Environmental cost of natural resources utilization and economic growth: Can China shift some burden through globalization for sustainable development? Sustainable Development, 28(6), 1678–1688. https://doi.org/10.1002/sd.2116
- Umar, M., Ji, X., Kirikkaleli, D., & Xu, Q. (2020). COP21 Roadmap: Do innovation, financial development, and transportation infrastructure matter for environmental sustainability in China? Journal of Environmental Management, 271, 111026.
- Umar, M., Ji, X., Mirza, N., & Naqvi, B. (2021). Carbon neutrality, bank lending, and credit risk: Evidence from the Eurozone. Journal of Environmental Management, 296, 113156 https://doi.org/10.1016/j.jenvman.2021.113156
- Wang, H., & Boatwright, A. L. (2019). Political uncertainty and financial market reactions: A new test. International Economics, 160, 14–30. https://doi.org/10.1016/j.inteco.2019.07.004
- Wang, R., Mirza, N., Vasbieva, D. G., Abbas, Q., & Xiong, D. (2020). The nexus of carbon emissions, financial development, renewable energy consumption, and technological innovation: What should be the priorities in light of COP 21 Agreements? Journal of Environmental Management, 271, 111027 https://doi.org/10.1016/j.jenvman.2020.111027
- Wen, F., Wu, N., & Gong, X. (2020). China’s carbon emissions trading and stock returns. Energy Economics, 86, 104627. https://doi.org/10.1016/j.eneco.2019.104627
- Wen, F., Zhao, L., He, S., & Yang, G. (2020). Asymmetric relationship between carbon emission trading market and stock market: Evidences from China. Energy Economics, 91, 104850. https://doi.org/10.1016/j.eneco.2020.104850
- Xu, Y. (2021). Risk spillover from energy market uncertainties to the Chinese carbon market. Pacific-Basin Finance Journal, 67, 101561. https://doi.org/10.1016/j.pacfin.2021.101561
- Yan, L., Mirza, N., & Umar, M. (2022). The cryptocurrency uncertainties and investment transitions: Evidence from high and low carbon energy funds in China. Technological Forecasting and Social Change, 175, 121326. https://doi.org/10.1016/j.techfore.2021.121326
- Yu, B., Li, C., Mirza, N., & Umar, M. (2022). Forecasting credit ratings of decarbonized firms: Comparative assessment of machine learning models. Technological Forecasting and Social Change, 174, 121255. https://doi.org/10.1016/j.techfore.2021.121255
- Zheng, J., Mi, Z., Coffman, D., Milcheva, S., Shan, Y., Guan, D., & Wang, S. (2019). Regional development and carbon emissions in China. Energy Economics, 81, 25–36. https://doi.org/10.1016/j.eneco.2019.03.003
- Zheng, Y., Zhou, M., & Wen, F. (2021). Asymmetric effects of oil shocks on carbon allowance price: Evidence from China. Energy Economics, 97, 105183. https://doi.org/10.1016/j.eneco.2021.105183
- Zhu, B., Zhou, X., Liu, X., Wang, H., He, K., & Wang, P. (2020). Exploring the risk spillover effects among China’s pilot carbon markets: A regular vine copula-CoES approach. Journal of Cleaner Production, 242, 118455. https://doi.org/10.1016/j.jclepro.2019.118455