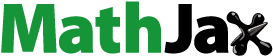
Abstract
The COVID-19 pandemic has reached more than 215 countries across the globe, and it has also led to severe damage to the worldwide financial markets. Therefore, the current study explores the role of the novel COVID-19 pandemic, the energy prices, and the subsequent financial uncertainty on the US market's environment and social responsibility index (ESRI), which is considered a good representation of the global market. The study has applied Quantile Autoregressive Lagged Approach (QARDL) for estimation using data from January 2010 to July 2021. The findings of the aforementioned relationships have been reported to be similar to the outcomes that have been observed for the short run, as reported in the longer period of time. Precisely, for energy prices and ESRI, the nature of the relationship remains negative. Moreover, in terms of the relationship between the financial uncertainty and ESRI, the nature of the relationship remains negative, while for the relationship observed between COVID and ESRI, the nature of the relationship remains positive. Based on these findings, maintaining the level of compliance towards ESRI, following the relevant SOPs, and improving the productivity of energy resources are viable measures that can be recommended.
1. Introduction
Increasing concerns aimed towards environmental deterioration have pressurised the policy-making institutions to embed the essence of sustainability, without tempering the agenda for economic development and growth (Guo et al., Citation2022; Umar, Ji, Mirza, & Naqvi, Citation2021; Yu et al., Citation2022). According to the United Nations (Citation2019), the regular increase in economic growth across the globe is one of the prime factors causing abnormal climatic conditions. It should also be noted that the industries are contributing a major amount of chunk to the economic growth; therefore, industrialization itself should be considered as a considerably responsible party for the change that is occurring in the climatic conditions (Su, Umar, et al., Citation2021; Su, Yuan, et al., Citation2021; Sun et al., Citation2021). following this realisation, manufacturers must assume their due responsibility, and incorporate philosophies of sustainability within their operations (X. Ji, Chen, et al., Citation2021; Umar, Ji, Kirikkaleli, Shahbaz, et al., Citation2020). In addition to this, the manufacturer should also aim towards expanding their scope of responsibility, which can thus be regarded as the ‘Extended Producer’s Responsibility’ (Najmi et al., Citation2021).
Although numerous researchers have urged manufacturers to play their respective role for the environment's well-being (Rizvi et al., Citation2021; Su et al., Citation2020), it must be noted that it is the industries that are the primary profit-driven institutions. In this regard, there are certain initiatives that can be taken on the industry level, by which these institutions can reduce the levels of operational costs. These initiative include activities such as collaboration with stakeholders at the development of the product stage (Najmi & Khan, Citation2017), improving their capabilities and competitiveness (Ahmed et al., Citation2019), improving the management of their available resources (Ahmed et al., Citation2019), incorporating green innovation in their operations (B. Naqvi et al., Citation2021; Umar, Ji, Kirikkaleli, & Xu, Citation2020), moving towards green supply chain operations (Ahmed et al., Citation2019), remaining responsible for efficient disposition of waste (Najmi et al., Citation2021), and moving swiftly towards renewable energy solutions (Akram et al., Citation2022; Su, Khan, et al., Citation2021). However, the most notable hurdle that hinders their readiness towards environmentally and socially compliant operations include the potential investments and the costs that would be incurred to implement the aforementioned improvements, which make all these solutions dearth and lead organizations to compromise on these responsibilities for profits.
The eruption of the novel coronavirus, which is termed as COVID 19, has been considered as the most lethal and devastating pandemic in human history. In contrast, the interest of the researchers, and the respective publications for evaluating its consequences are at a parallel pace with the eruption of the COVID −19 virus (Harjoto et al., Citation2021). However, evidence shows that there were other events in history that have been declared as pandemics that severely challenged the overall objectives of the affected economies in general, and their respective healthcare expenditure. These include the 1918s Spanish Flue, 2003s SARS, 2012s MERS, and the spread of 2014s Ebola virus (World Health Organization, Citation2020). However, in terms of the losses incurred, including those that are financial and human in nature, no pandemic has been as disastrous as the COVID 19 (Wang et al., Citation2021). In contrast, the failure to handle such an outbreak of disease by the global government institutions and international bodies has also raised the level of uncertainties, chaos, and anarchy (Szczygielski et al., Citation2021). As this pandemic has now reached more than 215 countries across the world, it has severely damaged the financial markets in its stride, as also done by the earlier pandemics seen by the world (Ali et al., Citation2020; Bekaert et al., Citation2014; McTier et al., Citation2013).
Despite the failure in handling the COVID 19 virus, certain initiatives have been taken by the respective governments and international bodies for countering its spread and flattening the curve of the outbreak. The most common one of these include the implementation of nationwide lockdowns (De Vito and Gomez, Citation2020), in which all the activities have been completely put to a halt, in order to curb any further infection and subsequently eruption of the virus (Hu et al., Citation2021; Jiang et al., Citation2021). However, these very lockdowns have destroyed the overall financial circulation and penetration of finances into the economies (Nguyen et al., Citation2021). For instance, increased bankruptcies (Akhtaruzzaman et al., Citation2019); lower stock returns (Al-Awadhi et al., Citation2020); uncertainties leading to volatilities in the stock markets (Zaremba et al., Citation2020); an increase in the level of liabilities (D. Zhang et al., Citation2020); deficiencies observed in the supply and demand of cash leading to financial crunch (De Vito & Gomez, Citation2020); and an adverse effect on the value and supply chain were some of the effects that have been reported thus far as a result of the lockdowns following the spread of Covid-19 (Nguyen et al., Citation2021).
Researchers across the globe have categorized the financial uncertainties and the contrary effects of the policy measures that have been taken by the law enforcement institutions into two categories (Umar, Ji, Mirza, & Rahat, Citation2021). The first category reflects the imbalance that arises in the portfolios of the stock market, where the potential investors withdraw their investments from the industry, particularly those who are reported to be at higher risk, and invest in the companies that are performing better and remain stable (Ielasi et al., Citation2018; Kaiser & Welters, Citation2019; Karim et al., Citation2022). For example, in the scenario of COVID −19, the most volatile and affected industry has been the tourism and airlines, whereas the healthcare industry has reportedly been a less affected and stable industry (Umar, Rizvi, & Naqvi, Citation2021). The second category reflects the clear wipe and withdrawal of the investments from the stock markets, which has eventually decreased the stock market's overall levels and, accordingly, disturbed the overall stock market health and behaviour (Baele et al., Citation2020; Q. Ji et al., Citation2020; Zaremba et al., Citation2020). Nevertheless, financial uncertainty has further affected economic productivity and growth, whereas, it has also hampered the compliance that is typically expected towards social and environmental responsibility (Sinha et al., Citation2021).
In the current pandemic situation, the literature is also revolving, whereas researchers have explored the diversified aspects of the relationships between different economic indicators in the context of COVID (Su, Huang, et al., Citation2021). For instance, the possible consequences faced by the financial markets in the situation of the COVID pandemic (Ali et al., Citation2020); disturbance in the drama and film industry (Hu et al., Citation2021); media penetration and coverage (Haroon & Rizvi, Citation2020) connection with the oil and energy prices (Apergis & Apergis, Citation2020; Gil-Alana & Monge, Citation2020), however, none of the study have explored the level of noncompliance and deterioration caused to the global environment and social responsibility index (ESRI) which is supposed to be the first relinquish that management used to make to keep them stable in such pandemic situation.
There are several potential contributions that the current study is expected to make. Firstly, the current study extracts the S&P 500 global data for ESRI, energy prices, and financial uncertainty of the US market, which are considered a good representation of the global market, whereas studying the nexus of the aforementioned phenomena in the current pandemic is highly significant. Secondly, the current study explores the scope of ESRI by using energy prices, COVID 19, and financial uncertainty as to their determinants which itself is a great contribution as no study is reported to explore similar types of relationships among the studied variables. Thirdly, the application of a recent quantile based technique is also a potential contribution, namely ‘Quantile Autoregressive Distributed Lag’ (QARDL) which is extremely helpful in exploring the in-depth meanings and insights of the data, their relationships, and behaviour as it links the predictor effects to the multiple quantiles of the criterion variable, thus lead to more meaningful results (Cho et al., Citation2015). In addition, the issues at hand are significantly important for the policymakers to formulate suitable policies based upon the current scenario.
The rest of the current research is ordered as the next section will summarize the most related literature findings, followed by the section in which the methodology of the statistics employed is discussed. The outcome generated from those techniques is reported, and lastly, the research is concluded, and relevant implications are recommended.
2. Literature review
COVID-19 and its related literature have explored the diversified aspects in terms of its economic effects. The different aspects of financial markets have been explored in such literature, including investment companies, insurance companies, financial institutions, and stock markets. In contrast, in terms of commodities, gold prices, oil prices energy prices have been explored by multiple researchers (Jiang et al., Citation2021). The majority of the literature has reported a negative relationship between COVID 19 with different attributes of financial markets across the globe (Dzigbede & Pathak, Citation2020). On the other hand, while compared with the health sector, the financial sector is reported to be least performing due to disruption in the performance of economic activities, which destructs the confidence of the investors for further future investments in the financial markets (Ibn-Mohammed et al., Citation2020; Nguyen et al., Citation2021; Topcu & Gulal, Citation2020). Moreover, the study by Pak et al. (Citation2020) have an in-depth exploration of the determinants of the reduction in the income levels and the volatilities faced by the financial sector because of the destructed economic indicators due to COVID and concluded that the COVID has distressed and troubled the whole value and supply chain. Furthermore, (Caggiano et al., Citation2020) also reported the adverse effects of COVID19 and reported the higher uncertainties in the global market due to COVID.
Rizvi et al. (Citation2020) revealed the COVID-19 pandemic and asset management nexus in EU economies. According to the empirical findings, managers prefer to shift their findings to low-risk options from the high-risk options and sector due to the pandemic. Similarly, in another interesting study (Mirza et al., Citation2020) also highlighted the interconnection between price reaction, volatility, and performance of European investment funds and the COVID-19 pandemic (Gao et al., Citation2021). Social entrepreneurship funds performed better and showed resilience during the pandemic as opposed to the investment funds. Yarovaya et al. (Citation2020) described the influence of human capital efficiency on equity funds in selected EU economies (X. Ji, Zhang, et al., Citation2021). The study's empirical results elaborated that equity funds perform better compared to other available investment options in the market. In another interesting study, Mirza et al. (Citation2020) also highlighted that the recent pandemic impacted the solvency profile of the selected firms badly. In addition, there are three most affected sectors, retail, mining, and manufacturing. Similarly, Mirza et al. (Citation2020) took the case study of Latin American economies and confabulated that funding options with higher human capital efficiency outperform other investment options. Yarovaya et al. (Citation2021) also supported the previously mentioned notion that investments funds with higher human capital efficiency can perform better than their counterparts with lower human capital efficiency.
In recent years we have observed a surge in research about the significance of green finance for different economies. For instance, S. A. A. Naqvi et al. (Citation2021) compared the performance of green and conventional funds; however, based on the selected sample of 27 emerging markets, the empirical results supported the notion that traditional funda performs better compared to renewable counterparts. Lobato et al. (Citation2021) compared the performance of two kinds of funds, conventional and responsible exchange-traded funds. The empirical analysis could not find a significant difference between the performance of these two alternative funding options. Further, in the same context, X. Ji, Zhang, et al. (Citation2021) also elucidated that green financing funds have an edge on other comparable funding options, i.e., brown and black funds. Umar, Ji, Mirza, and Naqvi (Citation2021) also confabulated that green funds proved to be one of the essential sources to improve the quality of the banking sector. Umar, Ji, Mirza, and Naqvi (Citation2021) explored the commodity prices and banking performance nexus for 12 developing economies. The study confabulated that the resource curse is one of the possible drivers of the default of the banking. In addition to this, X. Ji, Zhang, et al. (Citation2021) also elucidated that firms and investors have to pay the premium for investing in green funds, therefore these funds do not seem a profitable opportunity for the firms and investers. Similarly, Taghizadeh-Hesary and Yoshino (Citation2020) also illustrated that economic growth is one of the most prominent factors to enhance the energy exports in case of the Russian Federation and its export partners. Umar, Su, Rizvi, and Lobonţ (Citation2021) confabulated that it is essential for the policy makers to detect the crude oil price bubbles and formulate the suitable policies.
The present empirical analysis contributes to the mainstream literature in many ways. For instance, while connecting the ESRI with the financial investments, Sinha et al. (Citation2021) have explored the potential of green investments and bond financing on the S&P 500 discussed ESRI. The study has employed Quantile and Quantile regression, linking the predictors' quantiles with the criterion variable's quantiles and the Wavelet decomposition approach. The data from 1st Oct 2020 to 31st July 2020 reported a negative relationship among the aforementioned relationships. On the other hand, the study by Sharif et al. (Citation2020), with the help of wavelet-based methodology, reported that energy prices were highly affected in the US due to COVID 19, leading to the creation of a higher level of uncertainties. Similar findings were reported by Peng et al. (Citation2020), who explored the relationship between oil prices and the stock market in the context of China, followed by the study of W. Zhang and Hamori (Citation2021), who also reported similar relationships in the stock markets of Germany, Japan and US during the erupted period of COVID. Nevertheless, the study where financial uncertainty, COVID, and energy prices were collectively explored on the ESRI found a gap and is intended to be filled by the current study. The empirical findings of the study provide a deeper understanding to policymakers for effective policy delivery.
3. Methodology
3.1. Data
The current study is an attempt to understand the in-depth relationships of Energy prices (OIL), COVID-19 (COVID), and financial uncertainty (VIX) with the environment and social responsibility (ESRI) by the application of a recent and advanced ‘Quantile Autoregressive Distributed Lag’ (QARDL). In order to measure the OIL, the proxy of oil prices is being used, whereas COVID is the reflection of a number of individuals who reported to have been diagnosed with COVID. VIX reflects volatility that occurs in the financial market and causes uncertainty, whereas ESRI is a global index that reflects the environment and social concerns perceived by the firms globally. Furthermore, the data frequency of the aforementioned phenomena is the daily data taken from January 1st, 2020, to July 31st, 2021. In addition to this, all of the studied phenomena are global and are extracted from the database of Bloomberg except for COVID, which was extracted from the database of Worldometer.
3.2. Quantile autoregressive distributed lag (QARDL)
As mentioned earlier, QARDL is a recent and advanced technique used in the current study to understand the relationships among the studied variables. This technique was developed by Cho et al. (Citation2015), which can enlighten the effects of predictors in the current study, OIL, COVID, and VIX on the quantiles of criterion variables, ESRI. In addition to this, the examination of the integration among the studied variables based on the time-varying principles was done through the Wald test, which is capable of evaluating the level of steadiness of the coefficients integrated throughout the quantiles Umar, Su, Rizvi, and Shao (Citation2021). The mathematical representation of traditional ARDL, which explores the associations among the studied variables, are mentioned below:
(1)
(1)
As mentioned in EquationEq. (1)(1)
(1) ,
is the reflection of the error term, which is further explained as
The term
represents the minimum
-field which is yielded through
The selection of equation’s optimized orders of lag is made through ‘Schwarz Information Criterion’, which are
Moreover, the logarithm form of the predictor variables are represented by
whereas the logarithm form of the criterion variable
In addition to this, the traditional ARDL mentioned in EquationEq. (1)
(1)
(1) is further expanded and restructured by Cho et al. (Citation2015) with an objective to accommodate the initial type of QARDL (p,q) model as mentioned below:
(2)
(2)
Referring to EquationEq. (2)(2)
(2) , the error term is expanded as
whereas
is the description of
th quantile of
subjected to the set of information
as mentioned earlier Kim and White (Citation2003). The reformulation of EquationEq. (2)
(2)
(2) for analysing the QARDL is mentioned below:
It should be noted that the parameters shown in Eq. (3) are used to evaluate the dynamics in a shorter period of time. The relationship in the longer period of time is examined by re-structuring Eq. (3), which is shown below as EquationEq. (4)(4)
(4) .
(4)
(4)
Referring EquationEq. (4)(4)
(4) ,
and
and
with
and
The computation of
and
is done similarly.
and
have been explained as
and
In addition to this, the possibility of serial correlation of was avoided through the generalization of QARDL as mentioned below:
(5)
(5)
Despite of the aforementioned restructuring, there is still the possibility of having dubious outcome due to the simultaneous nature of correlations among the Such kind of correlations can be avoided through the utilization of projected estimations of
over
through the formation of
This led to the variance of
unrelated with the estimates of
The earlier projected estimations are incorporated in EquationEq. (5)
(5)
(5) , which generalizes the quantile regression framework. Therefore, the projected QARDL-ECM is formulated as mentioned below:
(6)
(6)
The accumulated effects for the shorter period of time is measured of the previous observation of ESRI on the latest observation of ESRI through The computation of accumulated effects in the shorter period of time of existing and earlier observations for OIL, COVID, and VIX on ESRI is accordingly made through
and
Furthermore, the cointegration in the longer period of time for OIL, COVID, and VIX is calculated through
and
Separately. In addition to this, the accumulated estimating and cointegration parameters for shorter and longer periods of time, respectively, are calculated with the help of the Delta Method. Moreover,
which is the reflection of the parameter of ECM should necessarily be negative and statistically significant.
On the other hand, the employment of Wald Test is made, which originally is made on the principles of distribution of Chi-Square for the purpose of investigating the asymmetric and non-linear effects of the predictor on criterion and their respective statistical significance. By the help of this test, the examination of the null and alternate hypothesis for the parameters of both longer and shorter period of time :
Against
Against
Against
Against
F and f are the pre-determined matrices in the aforementioned hypotheses: h*ps and h*l. Moreover, S and s are also similar and are h*s and h*l, whereas the h is actually the reflection of restrictions imposed (Cho et al., Citation2015), whereby i is the reflection of predictor variables, OIL COVID and VXI. Precisely, the Wald test was applied to examine the non-linear associations according to the speed of adjustment made by the parameter and the integration made in a longer period of time. On the contrary, four different tests were applied for every single parameter and respective groups.
4. Estimations and results
Prior to applying the QARDL (as discussed in the earlier section), some other tests were accordingly employed to assess the level of coherence, robustness, and internal consistency. Therefore, at the initial stage, descriptive statistics of the studied variables were assessed in . Among the predictor variables, OIL, COVID, and VIX, the mean value of OIL was found to be 0.3061, and its standard deviation was reported to be 1.0413, whereas the maximum and minimum values of these variables are observed to be 0.401 and 0.1602, respectively. In the case of COVID, the mean value was found to be 0.0612, and its standard deviation was reported to be 0.4042, whereas the maximum and minimum values of this variable are 0.1215 and 0.0340, respectively. Moreover, in the case of VIX, the mean value was found to be 0.5010, and its standard deviation was reported to be 1.0403, whereas the maximum and minimum values of this variable are observed to be 0.7020 and 0.1103, respectively. On the other hand, considering the criterion variable in the current study is ESRI, the mean value was found to be 0.0100, and its standard deviation was reported to be 0.0132, whereas the maximum and minimum values of these variables are observed to be 0.0710 and 0.0085, respectively.
Table 1. Results of descriptive statistics.
On the contrary, the application of the Test of Jarque-Bera was made to assess the existence of normality among the dataset. The presence of normality is extremely crucial as its absence ignite the possibilities of the contagious outcome. The hypothesis statements of this test entail the absence of normality if there is a refusal of the null hypothesis, whereas the data is said to be normal if there is the approval of the alternate hypothesis. The results depicted in acknowledges that all of the studied variables including predictors and criterion are having a normal distribution which is also found to be significant at 1% level of significance (Razzaq, Sharif, Ahmad, et al., Citation2021; Shahbaz et al., Citation2018; Sharif et al., Citation2019).
Once the exploration of the descriptive statistics was done, the existence of integration in the dataset along with their order respectively was made in the later stage. Such assessment is also considered as essential and vital before the application of QARDL is made. Hence for the said purpose, the unit root test of ‘Augmented Dickey-Fuller (ADF)’ along with the test of Zivot and Andrews (Citation2002) (ZA) was employed, which is in accordance with the existing similar studies (Godil et al., Citation2020; Razzaq, Sharif, Najmi, et al., Citation2021). It should be noted that the test of ZA is superior to any other similar kind of tests assessing unit roots with an additional competency of ascertaining the structural breaks. The summarized outcome mentioned in confirms that all of the studied variables are free to form the unit root problem as they are statistically significant at a 1% significance level. Moreover, the outcome of the ZA test also ascertains the structural tests present in the variables, as mentioned in . The findings of these tests are actually the assurance of the quality attributes which eventually justify that QARDL can be applied in this kind of data set. The application of QARDL is legitimate even when the data possess the attributes of non-linearity, having multiple and dynamic trends and structural breaks (Aziz et al., Citation2020, Citation2021).
Table 2. Results of Unit root test.
After confirming stationarity in the dataset, the employment of QARDL is finally made as per the objectives of the current study in which there are three predictor variables, namely OIL, COVID, and VIX, and once criterion variable, which is ESRI. The outcome generated through the application of QARDL is summarized in . In the first step, the values of ECM were evaluated, which, as discussed earlier, need to be statistically significant, whereas its nature should also be negative. The outcome confirms that all of the ECM values are negative for all of the quantiles and statistically significant at a 1% level of significance for all levels of the quantiles of ESRI.
Table 3. Results of quantile autoregressive distributed lag (QARDL).
Precisely for exploring the effects of energy prices on ESRI quantiles, the nature of the relationship remains negative for all quantiles, whereas the nature of significance evolves from low quantiles to high quantiles. As reported in , the OIL is significant at a 10% level of significance on lower quantiles, whereas it is significant at a 1% level of significance for higher quantiles. The negative relationship is logical and justifiable, as when there are low prices of energy, there will be additional rational or irresponsible consumption which eventually deteriorates the ESRI. On the other hand, when the prices will be high, there will be less consumption, and it eventually improves the ESRI. Similarly, in the context of the relationship between COVID and ESRI, the nature of the relationship remains positive for all quantiles, whereas there is a difference in the nature of significance among low quantiles to high quantiles. As reported in , the COVID is significant at a 1% level of significance on lower quantiles, whereas for higher quantiles, it is also significant at a 1% level of significance; however, it remains for mid quantiles insignificant. This kind of relationship and significance is because whenever there is a certain level of COVID, there will be a response by the economy and institutions to counter the level of COVID for further improving the environmental and social responsibility. For the mid-levels, the relationship becomes insignificant because there will be enough response at the institutional level for addressing the COVID, which eventually makes the existing strategies remain legitimate to be pursued further. On the other hand, as evident in the higher quantiles, the higher level of COVID requires higher support at the institutional level. Hence the relationship is significant because at this stage, there will be extra dedication and concerns from the institutions for countering the consequences of COVID.
On the contrary, in terms of the relationship between financial uncertainty and ESRI, the nature of the relationship remains negative for all of the quantiles, whereas the nature of significance evolves from low quantiles to high quantiles. As reported in , the relationship was statistically insignificant on lower quantiles in terms of significance. However, as it moves towards the higher quantiles, it becomes significant. Precisely, the relationship was significant at a 10% significance level for mid-level quantiles, and for higher quantiles, the relationship was significant at a 1% level of significance. This means that for higher uncertainty, there will be destruction in the performance of the ESRI, whereas low uncertainty is always good for the economy to fulfil its environment and social responsibility.
In the shorter period of time, the aforementioned relationships were reported to be similar outcomes as reported in the longer period. Precisely, for energy prices and ESRI, the nature of the relationship remains negative for all of the quantiles, whereas the nature of significance evolves from low quantiles to high quantiles. At lower quantiles, it was significant, whereas, for higher quantiles, it becomes insignificant. This difference is because of the shared areas of the end of a shorter period of time and the start of a longer period of time. Similarly, for the relationship between COVID and ESRI, the nature of the relationship remains positive for all of the quantiles, whereas there is a difference in the nature of significance among low quantiles to high quantiles. At lower quantiles, it was significant, whereas, for higher quantiles, it becomes insignificant. This difference is because of the shared areas of the end of a shorter period of time and the start of a longer period of time. On the other hand, for the relationship between financial uncertainty and ESRI, the nature of the relationship remains positive for all of the quantiles, whereas the nature of significance evolves from low quantiles to high quantiles. It was insignificant for initial low quantiles; however, the relationships also evolve in terms of significance as the quantiles evolve. The estimated results are depicted in .
Once the QARDL is successfully employed and the outcome is generated, the Wald test is applied in the subsequent step with an objective to ascertain whether asymmetries among the studied variables. Though the results have already established the absence of any specific asymptotic distribution, the further capability of this test is to explore the level of uncertainties at the level of coefficients and intercepts. Furthermore, tests' capabilities also include identifying any possible subsequent change in the nature of structural breaks, even if they are sufficient to be identified or not (Godil et al., Citation2020). The outcome generated provides sufficient evidence to assume the existence of symmetries in both the time period, which are long run and short run, which was assessed among all of the studied variables. The summarized outcome of Wald tests is mentioned in .
Table 4. Results of the Wald test for the constancy of parameters.
Lastly, the causality and its respective direction were assessed with the help of the Test of Granger Causality, in which the nature of causality was assessed for all of the predictors along the quantiles of the criterion variable. Though the sort of causality and its nature is already explained through the help of QARDL, this test enables one to evaluate the causality the other way around, which means not just from predictor(s) to criterion but also from criterion to predictor(s). The outcome generated through the application of the test of Granger Causality reports the bi-directional nature of causality for all of the studied relationships. Precisely, for the relationship between OIL and ESRI, the OIL is reported to cause ESRI, which is already discussed. However, ESRI will also have the capability to cause OIL as for pursuing the responsibility towards the environment and social aspects, and there will be lessor expenditure on OIL which will eventually affect the demand and supply of the OIL and hence the prices respectively. On the other hand, considering the relationship between COVID and ESRI, the COVID is reported to cause ESRI, which is already discussed. However, ESRI will also have the capability to cause COVID as for pursuing the responsibility towards the environment and social aspects, and there will be an additional concern of the institutions to counter the level of COVID. Lastly, considering the relationship between VIX and ESRI, the VIX is reported to cause ESRI, which is already discussed. However, ESRI will also have the capability to cause VIX to pursue the responsibility towards the environment and social aspects. There will be additional attention of the governmental institutions to counter the level of uncertainties and improve the level of confidence. The summarized outcome of the Test of Granger Causality are mentioned in .
Table 5. ‘Granger causality in quantile test results’.
5. Conclusion and recommendations
The regular increase in economic growth across the globe is considered the prime factor causing abnormal climatic conditions. Moreover, at the same time, increased concerns towards environmental deterioration have pressured the policy-making institutions to embed the essence of sustainability without tempering the agenda for economic development and growth. Despite the fact that Industries are primarily profit-driven institutions, numerous researchers have urged manufacturers to play their role for the environment's well-being.
The pandemic has reached to more than 215 countries, it also has severely damaged the financial markets, and in terms of losses, including both financial and human casualties, no pandemic is found to be as disastrous as COVID 19. In contrast, the failure to handle it by government institutions and international bodies has also raised the level of uncertainties, chaos, and anarchy. Therefore, the current study extracts the S&P 500 global data for ESRI, energy prices, and financial uncertainty of the US market, which are considered the good representation of the global market, and explored the respective relationships as studying the nexus of the aforementioned phenomena in the current pandemic is highly significant.
The application of a recent quantile based technique is made, namely ‘Quantile Autoregressive Distributed Lag’ (QARDL) which is extremely helpful in exploring the in-depth meanings and insights of the data, their relationships, and behaviour as it links the predictor effects to the multiple quantiles of the criterion variable, thus lead to more meaningful results. Furthermore, the data frequency of the aforementioned phenomena is the daily data taken from January 1st, 2020, to July 31st, 2021.
The findings of the aforementioned relationships were reported to be the similar outcome for the short-run as reported in the longer period of time. Precisely, for energy prices and ESRI, the nature of the relationship remains negative for all of the quantiles, whereas the nature of significance evolves from low quantiles to high quantiles. On the contrary, in terms of the relationship between financial uncertainty and ESRI, the nature of the relationship remains negative for all of the quantiles, whereas the nature of significance evolves from low quantiles to high quantiles. As reported in , the relationship was statistically insignificant on lower quantiles in terms of significance. However, as it moves towards the higher quantiles, it becomes significant. Similarly, for the relationship between COVID and ESRI, the nature of the relationship remains positive for all of the quantiles, whereas there is a difference in the nature of significance among low quantiles to high quantiles. At lower quantiles, it was significant, whereas, for higher quantiles, it becomes insignificant.
Lastly, the causality and its respective direction were assessed with the help of the Test of Granger Causality, in which the nature of causality was assessed for all of the predictors along the quantiles of the criterion variable. The outcome generated through the application of the test of Granger Causality reports the bi-directional nature of causality for all of the studied relationships.
Based on the findings, there are numerous policy implications that the current study intends to make. First and foremost, the government and law enforcement institutions need to maintain the level of compliance by the industries in the pursuit of environmental and social sustainability. It is obvious that since these activities have adverse effects on the companies' profits, the companies can relinquish them. Therefore, the role of institutions in maintaining compliance is pivotal for sustainability. Secondly, despite the hardships humanity faces in these times due to pandemics, the profit-making companies have to come forward and accordingly need to assume their responsibilities for the environment and social health, welfare and well-being. Thirdly, individuals need to follow and maintain the propositions of SOPs as communicated by the institutions because the world is currently now at the recovery stage, and the lockdown is lifted from almost every country. So if the individuals regularly follow SOPs, then the situation will be in control, and there will be no such decision of imposing lockdown again. Lastly, the organizations need to improve their resource management through optimization to consume energy efficiently, which makes the organization fulfil their environment and social responsibility.
6. Limitations and way forward
We have used the one proxy of ESRI. However, based on the limitations, future researchers' directions include exploring further determinants of ESRI, which is a good proxy for measuring sustainability. Moreover, the concept of sustainability comprises three streams: environmental, social, and financial aspects. In ESRI, the financial aspect is missing, so a more advanced index is recommended, which incorporates the financial aspect. In addition to this, similar relationships should be explained to draw the comparison and similarity in terms of developing and developed countries. Most importantly, the countries regarded as the epicentre in different points of time for COVID 19, like China, England, India, etc., should be explored because these countries were reported to be at the highest risk. Lastly, in terms of methodology, a similar relationship should be explored by linking the quantiles of the predictors with the quantile of the criterion so that more data insights can be extracted. Such exploration is possible by applying Quantile on Quantile Regression which will serve the purpose mentioned above.
Disclosure statement
No potential conflict of interest was reported by the authors.
Additional information
Funding
References
- Ahmed, W., Najmi, A., Khan, F., & Aziz, H. (2019). Developing and analyzing framework to manage resources in humanitarian logistics. Journal of Humanitarian Logistics and Supply Chain Management, 9(2), 270–291. https://doi.org/10.1108/JHLSCM-01-2019-0012
- Akhtaruzzaman, M., Abdel-Qader, W., Hammami, H., & Shams, S. (2019). Is China a source of financial contagion? Finance Research Letters, 101393, 1–13.
- Akram, R., Umar, M., Xiaoli, G., & Chen, F. (2022). Dynamic linkages between energy efficiency, renewable energy along with economic growth and carbon emission. A case of MINT countries an asymmetric analysis. Energy Reports, 8, 2119–2130. https://doi.org/10.1016/j.egyr.2022.01.153
- Al-Awadhi, A. M., Alsaifi, K., Al-Awadhi, A., & Alhammadi, S. (2020). Death and contagious infectious diseases: Impact of the COVID-19 virus on stock market returns. Journal of Behavioral and Experimental Finance, 27, 100326.
- Ali, M., Alam, N., & Rizvi, S. A. R. (2020). Coronavirus (COVID-19) – An epidemic or pandemic for financial markets . Journal of Behavioral and Experimental Finance, 27, 100341. https://doi.org/10.1016/j.jbef.2020.100341
- Apergis, E., & Apergis, N. (2020). Can the COVID-19 pandemic and oil prices drive the US Partisan Conflict Index. Energy Research Letters, 1(1), 13144.
- Aziz, N., Mihardjo, L. W., Sharif, A., & Jermsittiparsert, K. (2020). The role of tourism and renewable energy in testing the environmental Kuznets curve in the BRICS countries: Fresh evidence from methods of moments quantile regression. Environmental Science and Pollution Research International, 27(31), 39427–39441.
- Aziz, N., Sharif, A., Raza, A., & Jermsittiparsert, K. (2021). The role of natural resources, globalization, and renewable energy in testing the EKC hypothesis in MINT countries: New evidence from Method of Moments Quantile Regression approach. Environmental Science and Pollution Research International, 28(11), 13454–13468.
- Baele, L., Bekaert, G., Inghelbrecht, K., & Wei, M. (2020). Flights to safety. The Review of Financial Studies, 33(2), 689–746. https://doi.org/10.1093/rfs/hhz055
- Bekaert, G., Ehrmann, M., Fratzscher, M., & Mehl, A. (2014). The global crisis and equity market contagion. The Journal of Finance, 69(6), 2597–2649. https://doi.org/10.1111/jofi.12203
- Caggiano, G., Castelnuovo, E., & Kima, R. (2020). The global effects of Covid-19-induced uncertainty. Economics Letters, 194, 109392.
- Cho, J. S., Kim, T., & Shin, Y. (2015). Quantile cointegration in the autoregressive distributed-lag modeling framework. Journal of Econometrics, 188(1), 281–300. https://doi.org/10.1016/j.jeconom.2015.05.003
- De Vito, A., & Gomez, J.-P. (2020). Estimating the COVID-19 cash crunch: Global evidence and policy. Journal of Accounting and Public Policy, 39(2), 106741. https://doi.org/10.1016/j.jaccpubpol.2020.106741
- Dzigbede, K. D., & Pathak, R. (2020). COVID-19 economic shocks and fiscal policy options for Ghana. Journal of Public Budgeting, Accounting & Financial Management, 32(5), 903–917. https://doi.org/10.1108/JPBAFM-07-2020-0127
- Gao, X., Ren, Y., & Umar, M. (2021). To what extent does COVID-19 drive stock market volatility? A comparison between the US and China. Economic Research-Ekonomska Istraživanja, 1–21. https://doi.org/10.1080/1331677X.2021.1906730
- Gil-Alana, L. A., & Monge, M. (2020). Crude oil prices and COVID-19: Persistence of the shock. Energy Research Letters, 1(1), 13200.
- Godil, D. I., Sarwat, S., Sharif, A., & Jermsittiparsert, K. (2020). How oil prices, gold prices, uncertainty and risk impact Islamic and conventional stocks? Empirical evidence from QARDL technique. Resources Policy, 66, 101638. https://doi.org/10.1016/j.resourpol.2020.101638
- Guo, X., Liang, C., Umar, M., & Mirza, N. (2022). The impact of fossil fuel divestments and energy transitions on mutual funds performance. Technological Forecasting and Social Change, 176, 121429. https://doi.org/10.1016/j.techfore.2021.121429
- Harjoto, M. A., Rossi, F., & Paglia, J. K. (2021). COVID-19: Stock market reactions to the shock and the stimulus. Applied Economics Letters, 28(10), 795–801. https://doi.org/10.1080/13504851.2020.1781767
- Haroon, O., & Rizvi, S. A. R. (2020). COVID-19: Media coverage and financial markets behaviour—A sectoral inquiry. Journal of Behavioral and Experimental Finance, 27, 100343.
- Hu, J., Yue, X.-G., Teresiene, D., & Ullah, I. (2021). How COVID19 pandemic affect film and drama industry in China: An evidence of nonlinear empirical analysis. Economic Research-Ekonomska Istraživanja, 1–19. https://doi.org/10.1080/1331677X.2021.1937262
- Ibn-Mohammed, T., Mustapha, K. B., Godsell, J. M., Adamu, Z., Babatunde, K. A., Akintade, D. D., Acquaye, A., Fujii, H., Ndiaye, M. M., & Yamoah, F. A. (2020). A critical review of the impacts of COVID-19 on the global economy and ecosystems and opportunities for circular economy strategies. Resources, Conservation and Recycling, 164, 105169. https://doi.org/10.1016/j.resconrec.2020.105169
- Ielasi, F., Rossolini, M., & Limberti, S. (2018). Sustainability-themed mutual funds: An empirical examination of risk and performance. The Journal of Risk Finance, 19(3), 247–261. https://doi.org/10.1108/JRF-12-2016-0159
- Jiang, C., Zhang, Y., Razi, U., & Kamran, H. W. (2021). The asymmetric effect of COVID-19 outbreak, commodities prices and policy uncertainty on financial development in China: Evidence from QARDL approach. Economic Research-Ekonomska Istraživanja, 1–20.
- Ji, X., Chen, X., Mirza, N., & Umar, M. (2021). Sustainable energy goals and investment premium: Evidence from renewable and conventional equity mutual funds in the Euro zone. Resources Policy, 74, 102387. https://doi.org/10.1016/j.resourpol.2021.102387
- Ji, X., Zhang, Y., Mirza, N., Umar, M., & Rizvi, S. K. A. (2021). The impact of carbon neutrality on the investment performance: Evidence from the equity mutual funds in BRICS. Journal of Environmental Management, 297, 113228 https://doi.org/10.1016/j.jenvman.2021.113228
- Ji, Q., Zhang, D., & Zhao, Y. (2020). Searching for safe-haven assets during the COVID-19 pandemic. International Review of Financial Analysis, 71, 101526. https://doi.org/10.1016/j.irfa.2020.101526
- Kaiser, L., & Welters, J. (2019). Risk-mitigating effect of ESG on momentum portfolios. The Journal of Risk Finance, 20(5), 542–555. https://doi.org/10.1108/JRF-05-2019-0075
- Karim, S., Naeem, M. A., Mirza, N., & Paule-Vianez, J. (2022). Quantifying the hedge and safe-haven properties of bond markets for cryptocurrency indices. The Journal of Risk Finance, 23(2), 191–205. https://doi.org/10.1108/JRF-09-2021-0158
- Kim, T.-H., & White, H. (2003). Estimation, inference, and specification testing for possibly misspecified quantile regression. Emerald Group Publishing Limited.
- Lobato, M., Rodríguez, J., & Romero, H. (2021). A volatility-match approach to measure performance: The case of socially responsible exchange traded funds (ETFs). The Journal of Risk Finance, 22(1), 34–43. https://doi.org/10.1108/JRF-04-2020-0066
- McTier, B. C., Tse, Y., & Wald, J. K. (2013). Do stock markets catch the flu? Journal of Financial and Quantitative Analysis, 48(3), 979–1000. https://doi.org/10.1017/S0022109013000239
- Mirza, N., Hasnaoui, J. A., Naqvi, B., & Rizvi, S. K. A. (2020). The impact of human capital efficiency on Latin American mutual funds during Covid-19 outbreak. Swiss Journal of Economics and Statistics, 156(1), 1–7. https://doi.org/10.1186/s41937-020-00066-6
- Najmi, A., Kanapathy, K., & Aziz, A. A. (2021). Understanding consumer participation in managing ICT waste: Findings from two-staged structural equation modeling–artificial neural network approach. Environmental Science and Pollution Research International, 28(12), 14782–14796.
- Najmi, A., & Khan, A. A. (2017). Does supply chain involvement improve the new product development performance? A partial least square-structural equation modelling approach. International Journal of Advanced Operations Management, 9(2), 122–141. https://doi.org/10.1504/IJAOM.2017.086680
- Naqvi, B., Mirza, N., Rizvi, S. K. A., Porada-Rochoń, M., & Itani, R. (2021). Is there a green fund premium? Evidence from twenty seven emerging markets. Global Finance Journal, 50, 100656. https://doi.org/10.1016/j.gfj.2021.100656
- Naqvi, S. A. A., Shah, S. A. R., Anwar, S., & Raza, H. (2021). Renewable energy, economic development, and ecological footprint nexus: Fresh evidence of renewable energy environment Kuznets curve (RKC) from income groups. Environmental Science and Pollution Research International, 28(2), 2031–2051.
- Nguyen, D. T., Phan, D. H. B., Ming, T. C., & Nguyen, V. K. L. (2021). An assessment of how COVID-19 changed the global equity market. Economic Analysis and Policy, 69, 480–491. https://doi.org/10.1016/j.eap.2021.01.003
- Pak, A., Adegboye, O. A., Adekunle, A. I., Rahman, K. M., McBryde, E. S., & Eisen, D. P. (2020). Economic consequences of the COVID-19 outbreak: The need for epidemic preparedness. Frontiers in Public Health, 8, 241.
- Peng, Y., Chen, W., Wei, P., & Yu, G. (2020). Spillover effect and Granger causality investigation between China’s stock market and international oil market: A dynamic multiscale approach. Journal of Computational and Applied Mathematics, 367, 112460. https://doi.org/10.1016/j.cam.2019.112460
- Razzaq, A., Sharif, A., Ahmad, P., & Jermsittiparsert, K. (2021). Asymmetric role of tourism development and technology innovation on carbon dioxide emission reduction in the Chinese economy: Fresh insights from QARDL approach. Sustainable Development, 29(1), 176–193. https://doi.org/10.1002/sd.2139
- Razzaq, A., Sharif, A., Najmi, A., Tseng, M.-L., & Lim, M. K. (2021). Dynamic and causality interrelationships from municipal solid waste recycling to economic growth, carbon emissions and energy efficiency using a novel bootstrapping autoregressive distributed lag. Resources, Conservation and Recycling, 166, 105372. https://doi.org/10.1016/j.resconrec.2020.105372
- Rizvi, S. K. A., Naqvi, B., & Mirza, N. (2021). Is green investment different from grey? Return and volatility spillovers between green and grey energy ETFs. Annals of Operations Research, https://doi.org/10.1007/s10479-021-04367-8
- Rizvi, S. K. A., Yarovaya, L., Mirza, N., & Naqvi, B. (2020). The impact of COVID-19 on valuations of non-financial European firms. Available at SSRN 3705462.
- Shahbaz, M., Lahiani, A., Abosedra, S., & Hammoudeh, S. (2018). The role of globalization in energy consumption: A quantile cointegrating regression approach. Energy Economics, 71, 161–170. https://doi.org/10.1016/j.eneco.2018.02.009
- Sharif, A., Aloui, C., & Yarovaya, L. (2020). COVID-19 pandemic, oil prices, stock market, geopolitical risk and policy uncertainty nexus in the US economy: Fresh evidence from the wavelet-based approach. International Review of Financial Analysis, 70, 101496. https://doi.org/10.1016/j.irfa.2020.101496
- Sharif, A., Shahbaz, M., & Hille, E. (2019). The transportation-growth nexus in USA: fresh insights from pre-post global crisis period. Transportation Research Part A: Policy and Practice, 121, 108–121.
- Sinha, A., Mishra, S., Sharif, A., & Yarovaya, L. (2021). Does green financing help to improve environmental & social responsibility? Designing SDG framework through advanced quantile modelling. Journal of Environmental Management, 292, 112751. https://doi.org/10.1016/j.jenvman.2021.112751
- Su, C.-W., Huang, S.-W., Qin, M., & Umar, M. (2021). Does crude oil price stimulate economic policy uncertainty in BRICS? Pacific-Basin Finance Journal, 66, 101519. https://doi.org/10.1016/j.pacfin.2021.101519
- Su, C.-W., Khan, K., Umar, M., & Zhang, W. (2021). Does renewable energy redefine geopolitical risks? Energy Policy, 158, 112566. https://doi.org/10.1016/j.enpol.2021.112566
- Su, C.-W., Naqvi, B., Shao, X.-F., Li, J.-P., & Jiao, Z. (2020). Trade and technological innovation: The catalysts for climate change and way forward for COP21. Journal of Environmental Management, 269, 110774 https://doi.org/10.1016/j.jenvman.2020.110774
- Sun, T.-T., Su, C.-W., Mirza, N., & Umar, M. (2021). How does trade policy uncertainty affect agriculture commodity prices? Pacific-Basin Finance Journal, 66, 101514. https://doi.org/10.1016/j.pacfin.2021.101514
- Su, C.-W., Umar, M., & Khan, Z. (2021). Does fiscal decentralization and eco-innovation promote renewable energy consumption? Analyzing the role of political risk. Science of the Total Environment, 751, 142220. https://doi.org/10.1016/j.scitotenv.2020.142220
- Su, C.-W., Yuan, X., Tao, R., & Umar, M. (2021). Can new energy vehicles help to achieve carbon neutrality targets? Journal of Environmental Management, 297, 113348.
- Szczygielski, J. J., Bwanya, P. R., Charteris, A., & Brzeszczyński, J. (2021). The only certainty is uncertainty: An analysis of the impact of COVID-19 uncertainty on regional stock markets. Finance Research Letters, 43, 101945. https://doi.org/10.1016/j.frl.2021.101945
- Taghizadeh-Hesary, F., & Yoshino, N. (2020). Sustainable solutions for green financing and investment in renewable energy projects. Energies, 13(4), 788. https://doi.org/10.3390/en13040788
- Topcu, M., & Gulal, O. S. (2020). The impact of COVID-19 on emerging stock markets. Finance Research Letters, 36, 101691.
- Umar, M., Ji, X., Kirikkaleli, D., Shahbaz, M., & Zhou, X. (2020). Environmental cost of natural resources utilization and economic growth: Can China shift some burden through globalization for sustainable development? Sustainable Development, 28(6), 1678–1688. https://doi.org/10.1002/sd.2116
- Umar, M., Ji, X., Kirikkaleli, D., & Xu, Q. (2020). COP21 Roadmap: Do innovation, financial development, and transportation infrastructure matter for environmental sustainability in China? Journal of Environmental Management, 271, 111026.
- Umar, M., Ji, X., Mirza, N., & Naqvi, B. (2021). Carbon neutrality, bank lending, and credit risk: Evidence from the Eurozone. Journal of Environmental Management, 296, 113156.
- Umar, M., Ji, X., Mirza, N., & Rahat, B. (2021). The impact of resource curse on banking efficiency: Evidence from twelve oil producing countries. Resources Policy, 72, 102080. https://doi.org/10.1016/j.resourpol.2021.102080
- Umar, M., Rizvi, S. K. A., & Naqvi, B. (2021). Dance with the devil? The nexus of fourth industrial revolution, technological financial products and volatility spillovers in global financial system. Technological Forecasting and Social Change, 163, 120450. https://doi.org/10.1016/j.techfore.2020.120450
- Umar, M., Su, C.-W., Rizvi, S. K. A., & Lobonţ, O.-R. (2021). Driven by fundamentals or exploded by emotions: Detecting bubbles in oil prices. Energy, 231, 120873. https://doi.org/10.1016/j.energy.2021.120873
- Umar, M., Su, C.-W., Rizvi, S. K. A., & Shao, X.-F. (2021). Bitcoin: A safe haven asset and a winner amid political and economic uncertainties in the US? Technological Forecasting and Social Change, 167, 120680. https://doi.org/10.1016/j.techfore.2021.120680
- United Nations. (2019). World Population Prospects 2019: Highlights. https://population.un.org/wpp/Publications/Files/WPP2019_10KeyFindings.pdf
- Wang, J., Umar, M., Afshan, S., & Haouas, I. (2021). Examining the nexus between oil price, COVID-19, uncertainty index, and stock price of electronic sports: Fresh insights from the nonlinear approach. Economic Research-Ekonomska Istraživanja, 1–17. https://doi.org/10.1080/1331677X.2021.1937260
- World Health Organization. (2020). Coronavirus Disease 2019 (Covid-19): Situation Report, 73. https://apps.who.int/iris/handle/10665/331686
- Yarovaya, L., Mirza, N., Abaidi, J., & Hasnaoui, A. (2021). Human capital efficiency and equity funds’ performance during the COVID-19 pandemic. International Review of Economics & Finance, 71, 584–591. https://doi.org/10.1016/j.iref.2020.09.017
- Yarovaya, L., Mirza, N., Rizvi, S. K. A., Saba, I., & Naqvi, B. (2020). The resilience of Islamic equity funds during COVID-19: Evidence from risk adjusted performance, investment styles and volatility timing. Investment Styles and Volatility Timing, November 25, 2020.
- Yu, B., Li, C., Mirza, N., & Umar, M. (2022). Forecasting credit ratings of decarbonized firms: Comparative assessment of machine learning models. Technological Forecasting and Social Change, 174, 121255. https://doi.org/10.1016/j.techfore.2021.121255
- Zaremba, A., Kizys, R., Aharon, D. Y., & Demir, E. (2020). Infected markets: Novel coronavirus, government interventions, and stock return volatility around the globe. Finance Research Letters, 35, 101597.
- Zhang, W., & Hamori, S. (2021). Crude oil market and stock markets during the COVID-19 pandemic: Evidence from the US, Japan, and Germany. International Review of Financial Analysis, 74, 101702. https://doi.org/10.1016/j.irfa.2021.101702
- Zhang, D., Hu, M., & Ji, Q. (2020). Financial markets under the global pandemic of COVID-19. Finance Research Letters, 36, 101528.
- Zivot, E., & Andrews, D. W. K. (2002). Further evidence on the great crash, the oil-price shock, and the unit-root hypothesis. Journal of Business & Economic Statistics, 20(1), 25–44. https://doi.org/10.1198/073500102753410372