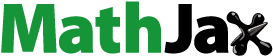
Abstract
There often exist different types of information due to the subjective and objective criteria in practical decision-making problems, thus it is necessary to develop some efficient frameworks to deal with the decision-making problems with heterogeneous information. The paper proposes a framework for group decision-making problems with heterogeneous information with thermodynamical parameters consisting of three parts to achieving this goal. The first part builds the rectifications of criteria weights according to decision makers’ confidence in evaluations. The second part adopts thermodynamical parameters to measure the numerical values and the data distribution of heterogeneous information to characterize the heterogeneous information fully. The last part applies the TODIM (an acronym in Portuguese for Interactive and Multicriteria Decision Making) to aggregate the decision-making results based on the characterized heterogeneous information without transforming it into a unified form. By depicting decision makers’ different sensitive attitudes towards uncertainty by several mathematical expressions, experiments are performed to assess the sensitive attitudes’ impacts on decision-making results with the proposed framework. Finally, a case study on the selection of a green supplier under the low-carbon economy is provided to illustrate the flexibility and feasibility of the proposed framework.
1. Introduction
Human management practices emerged almost simultaneously with the dawn of human civilization. Going with the development of human being’s exercising activities, decision making as a branch of management has made its way into the fields of economics (Bateman et al., Citation2013; Buyukozkan & Guleryuz, Citation2016; Farahani & Asgari, Citation2007; Johansson-Stenman, Citation2018; Kaya et al., Citation2019; Leiser & Azar, Citation2008; Mendoza, Citation2018; Rani et al., Citation2020; Santoyo-Castelazo & Azapagic, Citation2014; Strantzali & Aravossis, Citation2016; Zhang et al., Citation2019), engineering (Liu et al., Citation2018; Mori et al., Citation2018; Wessel et al., Citation1984), military (Salinas, Citation2017; Sánchez-Lozano et al., Citation2015), etc. Initially, the classical decision theory was established based on the expected utility theory (Von et al., Citation1944), which shows the idea that in the decision-making process, decision makers usually look forward to the current environment and the future development, and respond to the possible gains and losses, finally choose the most desirable way to achieve the maximum satisfaction. The utility theory defines individual behavior in decision making and supports decision theory development until now.
With modern decision-making evolution, the environment’s complexity and uncertainty determine that the decision makers are limited to providing accurate evaluations of objects. Vague expressions, such as fuzzy set (Zadeh, Citation1965), intuitionistic fuzzy set (IFS) (Atanassov, Citation1983, Citation1986), hesitant fuzzy set (HFS) (Torra, Citation2010), linguistic term set (LTS) (Zadeh, Citation1975), hesitant fuzzy linguistic term set (HFLTS) (Rodríguez et al., Citation2012), etc., were proposed, where an IFS describes an object by a membership and a non-membership, a HFS describes an object by several possible memberships, a LTS describes an object by a linguistic term, and a HFLTS describes an object by several continuous linguistic terms. The past years have seen increasingly rapid advances in the field of fuzzy decision-making methods with different fuzzy sets (e.g., Kahneman et al., Citation2015; Liao & Xu, Citation2015; Ren et al., Citation2021).
In practice, decision makers meet the decision-making problems with both subjective and objective criteria frequently. For example, suppose a capitalist considers investing in a corporation among several possible corporations. In that case, he may measure them by the following criteria: asset-liability ratio, annual profit, corporate image, corporate credit, and corporate development program. The data types of evaluations concerning criteria are diverse in such cases. Under this background, researchers have shown an increased interest in addressing the decision-making problems with heterogeneous information. The techniques can be briefly divided into two categories: (1) transforming all heterogeneous information into common types of data and making a decision (Herrera & Martinez, Citation2000; Li et al., Citation2010; Tan et al., Citation2019; Xu et al., Citation2016); (2) introducing an appropriate method to directly use heterogeneous information to make a decision (Lourenzutti et al., Citation2017; Peng et al., Citation2011; Verma et al., Citation2015; Xu, Citation2007; Zhang et al., Citation2019, Yue, Citation2019, Liang et al., Citation2020; Zheng et al., Citation2020).
The above research represents the evaluations on objects towards each criterion by different forms, highlighting that decision makers may have different levels of understanding about an object’s performance under each criterion. These different forms of evaluations reflect the different uncertain degrees of decision makers on objects to some extent. However, the above research solves the decision-making problems by using numerical characters of the heterogeneous information, which ignores its distributed characters. In some cases, taking the distributed characters of information is necessary and meaningful. For example, suppose that, in a group decision-making problem, four decision makers assign “0.3, 0.6, 0.5, 0.4” to an object, and assign “0.5, 0.5, 0.4, 0.4” to another object, the average evaluations show that the performances of the two objects are identical, then should we accept the results? The answer is no because the initial evaluations of them are disparate. Obviously, in decision-making problems, choosing the object with “0.5, 0.5, 0.4, 0.4” as the optimal one is more dependable for its centralized evaluation of four decision makers.
Considering this weakness, Verma and Rajasankar (Citation2017) introduced the thermodynamical knowledge in physics to construct the decision-making methods with real numbers (i.e., numerical number, the abbreviation is RNs) and triangular fuzzy numbers (i.e., a simple form of fuzzy numbers, the abbreviation is TFNs). The method uses energy, exergy, and entropy indicators to depict the judgments with group concentration (valid judgments) and group dispersion (invalid judgments). Motivated by this idea, Ren et al. (Citation2017a) extended the method to depict the thermodynamical characteristic of decision information in an intuitionistic fuzzy environment. Due to the bounded rationality of human beings, the thermodynamical method was further applied to handle the hesitant fuzzy information (Ren et al., Citation2017b). Furthermore, by combining the thermodynamical parameters and the prospect theory, the thermodynamical decision-making methods were developed in a hesitant fuzzy linguistic environment, a probabilistic linguistic environment, and a Pythagorean fuzzy environment (Coban & Onar, Citation2021; Liao et al., Citation2018; Wang et al., Citation2020).
So far, the above thermodynamical decision-making approaches are confined to solving decision-making problems in specified circumstances, limiting the universality of handling decision-making problems with different types of information. To improve the thermodynamical decision-making methods’ applicability, we develop a thermodynamical framework for heterogeneous information involving RNs, utility values (i.e., a measure of people's satisfaction, the abbreviation is UVs), LTs, and HFLTSs, in which RNs are used to depict the objective values on criteria, UVs, LTs, and HFLTSs are the commonly used descriptions in economic and management activities. For example, suppose that we let a decision maker evaluate an alternative with respect to a subjective criterion. In that case, he may assign his utility on the alternatives as “8” (from 1 to 10), or may express his feeling on alternatives by the terms of “good” or even “more than slightly good”. It is easy to catch that such descriptions of “8”, “good” and “more than slightly good” are people’s common expressions, which are called UVs, LTs, and HFLTSs.
The main innovations of the paper can be summarized as follows:
Based on that UVs, LTs, and HFLTSs are the expression forms people commonly use in economic and management activities, the paper builds a decision-making process by considering these subjective evaluations, which would fruit the framework of decision making in economics and management.
Considering different types of subjective evaluations indicate different levels of fuzzy mind, the paper rectifies the original weight of the criterion that people are hesitant to give accurate evaluations accordingly, which would decrease the impacts brought by uncertain factors and limitations of decision makers’ thoughts.
The paper applies thermodynamical parameters to describe the centralized degree of decision-making information, which would improve the reliability and precision of the results.
The paper introduces the TODIM (an acronym in Portuguese for Interactive and Multicriteria Decision Making) to collect each alternative’s overall score without the form of transformation and measure information with prospect theory, which would avoid information loss, as well as increase the significance of results in economics and management with uncertainty and risk.
The remainder of the paper is organized as: Section 2 reviews basic knowledge about related fuzzy sets and the thermodynamical decision-making method, then shows the implications of the proposed framework in the economic and management field. Section 3 defines heterogeneous thermodynamical decision-making parameters and provides the rectifying weights according to decision makers’ confidence levels. Section 4 presents a heterogeneous group decision-making framework, including correcting the criteria weights according to the decision maker’s confidence level, characterizing the numerical values and distribution of decision-making information simultaneously, and introducing the TODIM to derive the final decision-making results. Experiments are performed in Section 5 to discuss how the decision makers’ sensitive attitudes towards uncertainty impact decision-making results. The proposed framework is applied to address a green supplier selection problem under the low-carbon economy in Section 6 to demonstrate its flexibility and feasibility. The paper ends up with conclusions in Section 7.
2. Preliminaries and implications
2.1. Linguistic variables
Based on a finite and ordered LTS (
is a positive number), the LT (Zadeh, Citation1975), expressed as
is utilized to describe the individual fuzzy mind towards objectives qualitatively. Some operations and measurements for any two LTs
and
were proposed as (Xu, Citation2005): (1) sα ⊕ sβ = sα+β
(2) λsα = sλα
(
) and (3)
Based on a finite and ordered LTS (
is a positive number), a HFLTS (Rodríguez et al., Citation2012), defined as
(Liao & Xu, Citation2015), is used to capture individual fuzzy mind towards objectives with several consecutive LTs in
where the hesitant fuzzy linguistic element (HFLE)
(Liao & Xu, Citation2015) is expressed by at least one LT in
To keep the operational characters of HFLTSs, Zhu and Xu (Citation2014) proposed adding some elements in the shorter one(s). If the shorter HFLTS is expressed as then the added elements
can be determined by
(
). Furthermore, Zhu and Xu (Citation2014) defined
as the ordered lower index set of
and Zhang and Wu (Citation2014) introduced the following comparative technique for two HFLTSs
and
If
then
If
then
Suppose that and
are two HFLTSs with the same length, their operations (Zhu & Xu, Citation2014) and measurements (Liao et al., Citation2014) are: (1)
(2)
(
); (3)
where
and
are the
th largest element in
and
respectively.
The current researchers fail to give a general conclusion for the subtraction between any two linguistic variables since the results may be out of the initial LTSs. To ensure the scope of operation, referring to the inverse of the additive operation, we define the following subtractive operations for LTs and HFLTSs with the restricted condition:
For any two LTs
and
if
then
For any two HFLTSs
and
with the same length, if
for all
then
2.2. Thermodynamical decision-making approach
For a multi-criteria decision-making (MCDM) problem with alternatives
and
criteria
decision makers assign the weights of the criteria as
and utilize the matrices
where
(
) are RNs, to evaluate the alternatives with respect to criteria. The thermodynamical decision-making process to handle it can be briefly introduced (Verma & Rajasankar, Citation2017) as:
For an alternative
(
), define the decision values
as its potential with respect to the criterion
and the weight
as its force, for
and
Calculate the quality
of the potential
by
for
and
Construct the energy matrix
and the exergy matrix
respectively, where
and
for
Obtain the energy indicator and the exergy indicator of the alternative
for each decision maker by
and
respectively;
Get the overall energy indicator and the overall exergy indicator of the alternative
by
and
respectively;
Calculate the overall entropy indicator of the alternative
by
and rank alternatives. The optimal alternative corresponds to the one with minimum entropy.
2.3. Implications in economic and management field
The construction of the proposed framework yields implications in the economic and management field, which can be summarized as follows:
In practical economic and management activities, people likely use different types of expressions to evaluate objects. By addressing the information of RNs, UVs, LTs and HFLTSs, the proposed framework provides an efficient tool for some common economic and management decision-making problems.
Considering the uncertainty and complexity exist in economic and management activities, the proposed framework introduces thermodynamical parameters to take full use of available information, which would improve the reasonability of the economic and management results.
As economic and management activities usually face risks, the proposed framework considers decision maker’s sensitive attitude to the fuzzy mind (manifested by vague expressions) during weight reification. It develops people’s characters of risk-aversion, risk-neutral, and risk-preference in economics into the actual decision-making process.
In general, the proposed framework is built based on taking the economic and management situations, i.e., uncertainty, complexity and risk into account. It would provide a way to improve the reasonability of the economic and management decision-making results.
3. Heterogeneous thermodynamical parameters and weight rectification
In this section, we present thermodynamical parameters to manifest the data features from the aspects of numerical values and distributions. Furthermore, considering that evaluations on alternatives regarding subjective criteria are inevitably ambiguous, we introduce a weight rectification way to decrease the relative weight of the criterion that corresponds to the evaluations with more uncertainty.
3.1. Heterogeneous thermodynamical decision-making parameters
Suppose that an MCDM problem contains decision makers
alternatives
subjective criteria
and
objective criteria
Decision makers assign the vector of criteria weights as
in which
and
for all
Decision makers use UVs, LTs, or HFLTSs to evaluate the alternatives towards the subjective criteria, and RNs manifest the objective performances of the alternatives
The heterogeneous decision matrix
which consists of all objective performances and individual evaluations, can be presented as:
(1)
(1)
where
is a UV expressed by a crisp value,
and
are respectively an LT and an HFLTS that are both provided based on LTS
for
and
Note that decision makers are not required to assign their judgments to the alternatives with respect to the objective criteria.
To establish the thermodynamical decision-making method in a heterogeneous environment, we firstly present some heterogeneous thermodynamical definitions:
Definition 3.1.
In a decision-making problem, the evaluation of an alternative with respect to a subjective or objective criterion is defined as the heterogeneous potential. The corresponding criterion weight is defined as the heterogeneous force. The heterogeneous potential can be presented by each form such as UV, LT, HFLTS, RN, etc., and the heterogeneous force is located in
In physics, the energy of an object is the sum of its kinetic energy and potential energy (Charles & Herbert, Citation1980; Perrot, Citation1998). Applying this relation to the decision-making circumstance, the energy of an alternative is equal to the potential energy for its static state.
Definition 3.2.
In each state, an alternative's heterogeneous energy is its efficacy in the heterogeneous decision-making system at that state, calculated by the product of its heterogeneous potential and the corresponding heterogeneous force.
By Definition 3.2, different types of potentials correspond to different types of energies, and a proposition to obtain the potentials is presented as:
Proposition 3.1.
For a heterogeneous decision matrix (
and
), where
may be expressed as a UV
an LT
an HFLTS
or an RN
the heterogeneous energy of the alternative
under the criterion
can be obtained by one of the following situations:
If the heterogeneous potential
is a UV, then we call the heterogeneous energy as utility energy, which can be calculated by
If the heterogeneous potential
is an LT, then we call the heterogeneous as energy linguistic energy, which can be calculated by
If the heterogeneous potential
is an HFLTS, then we call the heterogeneous energy as hesitant fuzzy linguistic energy, which can be calculated by
(Liao et al., Citation2018);
If the heterogeneous potential
is an RN, then we call the heterogeneous energy as real energy, which can be calculated by
(Verma & Rajasankar, Citation2017),
For general decision-making problems, if the evaluations on alternatives are described in the same form, then we can get the overall values of the alternatives by using the weighted aggregated operations; or if the evaluations on alternatives are described in different forms, then we can (1) normalize them into the same scale and calculate the weighted average of alternatives, or (2) make relative comparisons among all alternatives with respect to each criterion and collect the comparative results of alternatives. These methods can compare alternatives and rank them numerically. Let us further consider a situation as described before: for two alternatives, if two sets of data have the same aggregated results, but the distribution of one set is more centralized than the other, which should be judged as the better one? In most cases, we likely choose the one with the more centralized distribution for its stable performance, similar to the case that an individual or corporation is willing to invest a stable fund rather than a steep one to guarantee profit and avoid risk. Then, in the following, we introduce the parameters to capture the centralized degree of the heterogeneous information.
Definition 3.3.
The heterogeneous static quality of a heterogeneous potential on an alternative is manifested by the concentration degree of the potential assigned by a decision maker to the mean of heterogeneous potentials assigned by all decision makers.
Proposition 3.2.
For a heterogeneous decision matrix (
and
), where
may be expressed as a UV
an LT
an HFLTS
or an RN
the heterogeneous static quality of any heterogeneous potential
(
) over all other heterogeneous potentials
(
) is
If the heterogeneous potential
is a UV, then we call the heterogeneous static quality as utility static quality, calculated by
If the heterogeneous potential
is an LT, then we call the heterogeneous static quality as linguistic static quality, calculated by
If the heterogeneous potential
is an HFLTS, then we call heterogeneous static quality as hesitant fuzzy linguistic static quality, calculated by
If the heterogeneous potential
is an RN, then
for all
and real static quality is
Furthermore, we put forward the concept of heterogeneous exergy as follows:
Definition 3.4.
Heterogeneous exergy is defined as the maximum centralized effect that a heterogeneous potential possesses, which can be calculated by the product of heterogeneous energy and heterogeneous static quality of each alternative.
Proposition 3.3.
For a heterogeneous decision matrix (
and
), where
is a UV
an LT
an HFLTS
or an RN
the heterogeneous exergy of a heterogeneous potential
(
) is
For a UV
its utility exergy
For an LT
its linguistic exergy
For an HFLTS
its hesitant fuzzy linguistic exergy
(Liao et al., Citation2018);
For an RN
its real exergy
(Verma & Rajasankar, Citation2017).
3.2. Weight rectification with the confidence level
With the idea that people always give accurate descriptions and evaluations to an alternative which they know very well and give ambiguous descriptions and evaluations to another which they are unfamiliar with, we propose to rectify the criteria weights to decrease the relative importance of the criteria correspond to indeterminate evaluations and increase relative weights of the criteria correspond to accurate evaluations.
Firstly, a concept to describe the confidence degree of a decision maker is introduced as:
Definition 3.6.
The confidence level of a decision maker with respect to his/her given HFLTS is a parameter to measure its practical dependability in the decision-making process, which can be calculated by
(2)
(2)
Remark 1.
The confidence level of hesitant fuzzy linguistic information focuses on recording the expression accuracy of its linguistic length. The accuracy is proposed based on the motivation of relative standard deviation in mathematics (statistics).
Definition 3.7.
For an uncertain decision value, its confidence level is calculated as suppose that a decision maker initially assigns its weight as
then its rectified weight can be obtained by
(3)
(3)
where
is a function of the confidence level
Remark 2.
The technique to rectify the weights for the evaluations with more fuzziness helps reduce the impact on the decision-making results caused by the unknown factors or individual thought limitations.
Proposition 3.5.
The form of can be determined by a decision maker’s sensitive attitude to the accuracy of decision value, specifically
(1) If decision makers are neutrally sensitive to the accuracy, then is a linear function,
(2) If decision makers are sensitive to the accuracy, then is a locally convex function,
(3) If decision makers are insensitive to the accuracy, then is a locally concave function,
Intuitively, the situations in Proposition 3.5. can be presented by .
4. A framework of heterogeneous thermodynamical decision making
Based on Section 3, the section aims to establish a decision-making framework for the MCDM problem with subjective and objective criteria, which involves the following three algorithms:
Algorithm 1.
Weights rectifying
Input: (1) The alternatives (2) the criteria
(3) the heterogeneous decision matrices
(
) that contain the heterogeneous potentials of alternatives towards subjective criteria (expressed by the UVs
the LTs
and the HFLTSs
) for
and the objective criteria (presented by the RNs
) for
and
(4) the weights of criteria
assigned by each decision maker; (5) the decision makers’ functions of the confidence levels according to their sensitive preferences;
Output: The rectified weights of criteria for
Step 1. For all heterogeneous decision matrices
(
and
), select the criteria
that correspond to the evaluations expressed by HFLTSs for
and
go the next step; If no criteria as such exist, end this algorithm;
Step 2. Denoted the selected criteria and their original weights as
(
and
) and
(
and
) respectively, go the next step;
Step 3. Obtain the confidence levels of the decision makers towards the selected criteria
(
and
) by
where is the length of the normalized HFLTSs for
th criteria given by
th decision maker. Go to the next step;
Step 4. According to the function of the confidence levels provided by the decision makers, calculate the rectified weights of the criteria
(
and
) by
and go to Step 5;
Step 5. For the criteria expressed by UVs, LTs, and RNs, their weights can be adjusted:
(6)
(6)
End.
For convenience, we note the rectified weights of criteria as for
which are composed of all above-rectified weights.
It is worth noting that considering the known information is shared among all decision makers in a heterogeneous group decision-making problem, we assume that decision makers reach a consensus to assign the evaluations to which criteria by more nebulous information because they contain more unknown factors. It means that the columns in these decision matrices, which correspond to the evaluations expressed by HFLTSs, are the same.
Based on Algorithm 1, a process can be furtherly proposed to obtain the thermodynamical features of alternatives:
Algorithm 2.
Thermodynamical features processing
Input: (1) The alternatives (2) the criteria
(3) the heterogeneous decision matrices
(
) that contain the heterogeneous potentials of alternatives towards subjective criteria (expressed by the UVs
the LTs
and the HFLTSs
) for
and the objective criteria (presented by the RNs
) for
and
(4) the rectified weights of criteria
Output: The heterogeneous exergy matrix;
Step 1. Obtain the heterogeneous energy of each heterogeneous potential by Proposition 3.1, and then construct the corresponding heterogeneous energy matrix
of each heterogeneous decision matrix
for
and
Go to the next step;
Step 2. Calculate the individual heterogeneous static quality matrix
for each individual heterogeneous decision matrix (
and
), where the elements
(
and
) can be obtained by Proposition 3.2. Turn to Step 3;
Step 3. Acquire the individual heterogeneous exergy matrix
of each individually heterogeneous decision matrix (
and
), whose elements can be obtained by Proposition 3.3. Go to Step 4;
Step 4. Synthesize all individual heterogeneous exergy matrices into an overall heterogeneous exergy matrix
(
and
), where
for
(7)
End.
Through Algorithm 1 and Algorithm 2, we can improve the rationality of the decision-making procedure from weight setting and available decision-making information characterizing. Next, a question arises – how to utilize the charactered information and make a decision?
Currently, there exist two paths to handle heterogeneous decision-making information. One is to transform information into the same type, then aggregate the homogeneous data; another is to introduce a method that makes a decision by relatively comparing alternatives with respect to each criterion. Since the latter can avoid distorting or losing information in the transformation process, we apply such thinking to our following decision analysis.
Prospect theory proposed by Kahneman and Tversky (Citation1979) indicates that people hold different attitudes towards gains and losses. Specifically, they are more sensitive to losses than gains. TODIM (Gomes & Lima, Citation1992), a popular decision-making method developed based on prospect theory, can relatively compare alternatives through their superiorities and inferiorities. With this property, the TODIM is suitable to deal with different types of information independently. Therefore, we extend the traditional TODIM method to make decisions with heterogeneous data. Two interpretations ought to be presented respectively, (1) there exists no research work that uses the TODIM to handle UVs, LTs, HFLTSs, and RNs simultaneously; (2) we address the TODIM procedure based on the thermodynamical feature decision matrix rather than a collection of original decision matrices, which contains more practical and refined group decision- making information and is better applied in actual problems.
Algorithm 3.
TODIM procedure for heterogeneous information with thermodynamical features
Input: The heterogeneous exergy matrix for
and
the rectified weights of criteria
for
Output: The global values and ranking of alternatives;
Step 1. Normalize the elements in the heterogeneous exergy matrix
that are expressed by UVs or RNs into 0-1.
If
is a UV, denoted as
then
(8)
(8)
If
is an RN, denoted as
then
(9)
(9)
Subsequently, we get a normalized thermodynamical feature decision matrix
Go to Step 2;
Step 2. Obtained the overall rectified weights
based on the individual rectified weights
for
by
Turn to Step 3;
Step 3. Calculate the relative weight of the criterion
to the criterion
for
(11)
Go to Step 4;
Step 4. Towards each criterion, we obtain the dominance degree of the alternative
over the alternative
with respect to the criteria
for
Specifically,
If
is a UV or an RN, then
(12)
(12)
If
is an LT or an HFLTS, then
(13)
(13)
where is the distance between
or
Go to the next step;
Step 5. Calculate the dominance degree of the alternative
over the alternative
by
Step 6. Obtain the global value of each alternative by Eq. (4.12), and then sort all alternatives according to the global values.
End.
5. Impact of sensitive attitudes on decision results
Since people could be divided into risk-aversion, risk-neutral, and risk-preference categories when they engage in economics and management activities, in this chapter, we consider the impact of their different risk attitudes towards fuzzy minds on the results obtained by the proposed framework. Specifically, the fuzzy minds are manifested by the different types of fuzzy expressions, and our proposed framework addresses the weight rectification on the criteria with more obscure expressions. As a result, we design experiments to analyze the effects of weight rectification on decision results caused by the decision makers’ different sensitive attitudes.
Suppose a group MCDM problem with four alternatives and four criteria (including an objective criterion and three subjective criteria). Three decision makers are invited to judge alternatives with respect to each subjective criterion by UVs, LTs, and HFLTSs, respectively, where LTs and HFLTSs are based on (
is a positive number). According to these settings, we randomly generate three decision matrices representing three decision makers’ judgments, and randomly generate three vectors of criteria weights. Then, we address the weight vectors by the following situations:
Situation 1. For the column that is evaluated by HFLTSs, suppose that the decision makers are neutrally sensitive to the accuracy of decision information, then we set the function of the confidence level as
to rectify its weight;
Situation 2. For the column that is evaluated by HFLTSs, suppose that the decision makers are sensitive to the accuracy of decision information, then we set the function of the confidence level as
to rectify its weight;
Situation 3. For the column that is evaluated by HFLTSs, suppose that the decision makers are insensitive to the accuracy of decision information, then we set the function of the confidence level as
to rectify its weight;
Situation 4. For the column that is evaluated by HFLTSs, we reserve its original weight.
We substitute these four weight vectors into Algorithm 2 and Algorithm 3, obtain the corresponding four alternative rankings, and record the optimal selection of each ranking. Repeating the above procedure 1000 times, we list four optimal selection rankings towards the four situations.
We further let the decision matrices be with five alternatives and five criteria, six alternatives and six criteria, seven alternatives and seven criteria, eight alternatives and eight criteria, nine alternatives and nine criteria, ten alternatives and ten criteria, then record the rankings with different situations under each order of decision matrices.
Through every 1000 simulations with different orders of matrices, we can get four rankings of the optimal selections for heterogeneous thermodynamical group decision making with original weights, the rectified weights under neutrally sensitive attitude, the rectified weights under sensitive attitude, and the rectified weights under insensitive attitudes, respectively.
One way to assess the impacts of sensitive attitudes on decision results is by making comparisons between the rankings with the original weights and the rectified weights towards different sensitive attitudes. Pearson product-moment correlation coefficient (PPMCC) is an easy and common statistical analysis technique to determine the relationship between two sequences of data from the perspective of linear correlation. The data without centralization can be utilized to analyze the observations of two sequences in pairs. Each pair of observations is independent, which is appropriate for the sequences obtained with different types of weight processing. Therefore, we use the PPMCC to test the correlations between two ranking sequences derived from simulations, and show the results in .
Table 1. The correlations between the rankings with the original weights and the rectified weights with different sensitive attitudes.
The correlation coefficients in are all significant since they all pass the significance test. The test shows that the decision results obtained through the original weights and the rectified weights with sensitive attitude/neutrally sensitive attitude are correlative. It is worth noting that the decision results obtained through the original weights and the rectified weights with insensitive attitudes can completely fit with each other because the correlation coefficients are all 1, which verifies that the decision makers’ insensitive attitude towards the accuracy of decision information does not influence the final optimal selections whatever the orders of the decision matrices are. The results accord with decision makers’ behaviors, i.e., with the decreasing sensitivity of decision makers, the influence of the rectified weights decreases, verifying the reasonability of the proposed framework.
It is apparent from that decision results obtained by the rectified weights with neutrally sensitive/sensitive attitudes can change the optimal selections. To further understand the effects of different sensitive attitudes towards uncertain decision informtion on optimal selections and find whether there exists any factor to impact these effects, we compare the optimal selections with the rectified weights and the optimal selections with the original weights, then record the different selections. The results are highlighted in .
Results in suggest that: (1) with the order of decision matrices increases, the numbers of different optimal selections decrease whatever decision makers are neutrally sensitive or sensitive towards the uncertain decision information; (2) when the orders of decision matrices are equal to or greater than seven and decision makers’ attitudes towards uncertain information are neutrally sensitive, the optimal selection is hardly to be impacted by the rectified weights.
Conclusions obtained by these experiments have some scientific contributions and significances, which can be summarized as follows:
(1) Decision makers who are insensitive to the fuzzy mind do not require weight rectification with our proposed framework, whereas decision makers who are sensitive or neutrally sensitive to the fuzzy mind need the weight rectification. The conclusion would improve the completeness and feasibility of the proposed framework in practical application with different kinds of decision makers.
(2) Under our proposed framework, the experiment conclusions strongly suggest that risk-preference people are hardly to be impacted by the fuzziness. However, the fuzzy impact on risk-neutral and risk-aversion people gradually increases. In other words, people with risk aversion mostly consider the uncertain or risk factor, which accords with human psychology and behavior in the real world.
6. Application to the selection of a green supplier under Low-Carbon economy
With climate change and global warming in recent years, human beings have suffered from various disasters, such as glacier melting, sea-level rise, frequent floods, etc. These situations destruct the biodiversity, reduce the crops, and increase diseases, threatening humanity’s survival and sustainable development (Cheng, Citation2010). Since the primary cause of global warming is the massive greenhouse gas emissions, and carbon dioxide is the primary kind of greenhouse gas, controlling and reducing carbon dioxide emissions is an absolute and direct path to ease global warming. Since the Copenhagen summit of 2009, numerous countries have focused on carbon emissions, and the concept of a low-carbon economy has been introduced.
Various countries towards carbon emission reduction have promulgated some laws. The government policy is becoming more stringent. For example, some developed countries have proposed to impose a carbon tariff on imported goods, and Singapore planned to levy a carbon tax in 2019. Similarly, the National Development and Reform Commission in China works with the Ministry of Finance and the Legislative Affairs Office to introduce China's carbon tax policy. The government faces the impact of the low-carbon wave, and consumers prefer low-carbon products. They no longer only pay attention to traditional economic factors like quality and price but also consider the corporations’ environmental performance essential when purchasing products. What is more, some corporations have considered low-carbon in their industrial chains. For example, Dell has required the major suppliers to report their carbon emissions and propose its requirements on carbon emissions since 2007; simultaneously, Wal-Mart has required all product suppliers to establish their low-carbon certification and reporting system.
Nowadays, low-carbon has become the inevitable trend of the development of contemporary corporations, and it has become a crucial factor for corporations to survive and enhance their competitiveness. Therefore, selecting an appropriate green supplier is worth being discussed in the corporation’s supply chain management.
The establishment of indicators has been deeply investigated. Based on introducing the environmental performance, Noci (Citation1997) divided the ecological management strategies into negative and positive strategies and then constructed the indicators for evaluating green suppliers, including green competitiveness, environmental management efficiency, green image, and life cycle from the perspective of positive management strategy. Later on, Humphreys et al. (Citation2003) provided a set of indicators for evaluating the suppliers’ environmental performance. He further proposed that it is reasonable to combine the qualitative and quantitative indicators to judge suppliers. After reviewing some references related to green suppliers’ selection problem, Wang (Citation2017) conducted that it is valid to measure the environmental performance by green image, contamination control, environmental management system, green design, green capability, product recycling, and pollution treatment cost. Moreover, different indicator systems were built to evaluate suppliers in different industries, such as the electronics industry (Lee et al., Citation2009; Lu et al., Citation2007) and the manufacturing industry (Buyukozkan & CifcI, Citation2011), etc.
Distinctly, it is needed for a corporation to comprehensively judge a green supplier from various aspects to promote long-term cooperation, such as its quality, reliability, and green ability, etc. Therefore, the following indicators are used in this paper: (1) Quality. It mainly indicates the quality guarantee of the products or techniques provided by a supplier, which includes: a) the certificates of quality such as ISO9000, ISO9001; b) the efficient ways to control non-conforming products; c) the measures to rectify or recall non-conforming products; (2) Delivery reliability. It is an indicator to judge if a green supplier can deliver the ordered products on time, which influences the companies’ business proceedings in the supply chain. It is by the ratio of the orders delivered and total orders. (3) Flexibility. It is an indicator to reflect the capacity of a green supplier, including a) the response capacity to react to the particular requirements on products; b) the diversity of products it provides; c) the capacity to deal with information sharing. (4) Green image. To some extent, it presents the responsibility of a supplier on social benefits, enterprise and society’s long-term development, and other aspects, which accord with the fundamental interests of modern society. (5) Technical and green research and development ability (R&D ability). It invisibly measures a supplier’s green innovation capacity from the perspectives of its technological development level, cleantech, green R&D ability for products, etc.
Suppose that there are four upstream suppliers for a manufacturing corporation to be chosen in a green supply chain, and a leader of the manufacturing corporation, including three division heads from the purchasing, operational, and planning departments, is needed to determine a supplier to cooperate. We denote the above five criteria as
and
the three heads respectively assign the weights of criteria as
and
Furthermore, the division heads give their opinions according to the known information as (): where the judgments with respect to
are expressed by RNs, the judgments with respect to
are expressed by UVs, the judgments with respect to
and
are expressed by LTs, the judgments with respect to
are expressed by HFLTSs, and LTs and HFLTSs are assigned based on the LTS:
Table 2. Decision matrices of the green supplier selection problem.
Before we process this decision-making problem, we list the reasons why we apply the proposed framework to solve it:
The criteria considered in the problem contain subjective and objective properties, which causes the judgments to be expressed in different forms. Thus, a decision-making method for heterogeneous information, including LTs, RNs, HFLTSs, and UVs, is needed to be utilized here.
Due to informational barriers existing in commercial activities, the three heads must provide their opinions with limited information. Inevitably, decision makers may have different attitudes to the known and unknown things. Therefore, the attitudes of the decision makers towards uncertainty should be considered in practice.
Suppose that the three heads are risk aversion and are sensitive to uncertain information, they prefer to use available information to make decisions and suppose that the function can represent their sensitive preferences. According to Algorithm 1, the weights of criteria assigned by each decision maker can be rectified as:
Based on the rectified weights, we apply Algorithm 2 to obtain a heterogeneous exergy matrix that synthesizes three heads’ judgments and includes numerical values and data distributions (as shown in ).
Table 3. Thermodynamical feature decision matrix of the green supplier selection problem.
With the thermodynamical feature decision matrix that includes heterogeneous decision information, we can apply Algorithm 3 to obtain the suppliers’ final ranking, and the results are presented in .
Table 4. Decision results of the green supplier selection problem.
From , we can know that, by the proposed model, the best cooperative partner is Supplier 2. The global values distinctly express differences among all suppliers, which the decision makers can visually use to make the final decisions. However, a drawback of the proposed model should be noted, i.e., the global score cannot react to each supplier’s superior aspect(s) in detail.
In this chapter, we perform the proposed framework to solve the green supplier selection problem under a low-carbon economy. It shows that with the evaluations given by decision makers, the proposed framework is available to derive a ranking for decision makers, where the ranking is meaningful for economic and management activities by considering peoples’ different types of evaluations, their fuzzy mind under uncertainty and their different risk attitudes.
7. Conclusions
This paper develops a framework for group decision-making problems with heterogeneous information with thermodynamical parameters. It includes rectifying criteria weights according to decision makers’ confidences on evaluations, adopting thermodynamical parameters to fully characterize the heterogeneous information from numerical values and the data distribution, and applying the TODIM to aggregate the decision-making results without the transformation of the characterized heterogeneous information. We further studied different decision makers’ sensitive attitudes’ impacts on decision results with the proposed framework, which provide economic and management implications for decision making with uncertainty and risk. Finally, we perform a case study on the selection of a green supplier under the low-carbon economy to illustrate the feasibility of the proposed framework.
Note that the proposed framework is full of economic and management significance since it addresses the features in economic and management activities manifesting as peoples’ different expression ways, the fuzzy mind under uncertainty, and different risk attitudes.
In addition, the proposed framework has limitations in handling the decision-making problems with other natural expressions used by people. In addition, the proposed framework considers the static decision-making problem, which limits solving dynamic decision-making situations. It would be better to overcome the above limitations in future work, specifically, discussing the proposed framework in different types of heterogeneous environments and developing the proposed framework to address a multi-stage decision-making problem, or even the problem with a stream of heterogeneous information.
Disclosure statement
No potential conflict of interest was reported by the authors.
References
- Atanassov, K. (1983). Intuitionistic fuzzy sets. Sofia. In V. Sgurev (Ed.), VII ITKR's Session, Sofia.
- Atanassov, K. (1986). Intuitionistic fuzzy set. Fuzzy Sets and Systems, 20(1), 87–96. https://doi.org/10.1016/S0165-0114(86)80034-3
- Bateman, I. J., Harwood, A. R., Mace, G. M., Watson, R. T., Abson, D. J., Andrews, B., Binner, A., Crowe, A., Day, B. H., Dugdale, S., Fezzi, C., Foden, J., Hadley, D., Haines-Young, R., Hulme, M., Kontoleon, A., Lovett, A. A., Munday, P., Pascual, U., … Termansen, M. (2013). Bringing ecosystem services into economic decision-making: Land use in the United Kingdom. Science (New York, N.Y.), 341(6141), 45–50. https://doi.org/10.1126/science.1234379
- Buyukozkan, G., & CifcI, G. (2011). A novel fuzzy multi-criteria decision framework for sustainable supplier selection with incomplete information. Computers in Industry, 62, 164–174.
- Buyukozkan, G., & Guleryuz, S. (2016). An integrated DEMATEL-ANP approach for renewable energy resources selection in Turkey. International Journal of Production Economics, 182, 435–448.
- Charles, L., & Herbert, K. (1980). Thermal physics. Macmillan.
- Cheng, S. W. (2010). New energy and low carbon economy. Business Review, 22, 4–8.
- Coban, V., & Onar, S. C. (2021). Emergency decision making problem of power cut in Turkey using Pythagorean fuzzy thermodynamic approach with prospect theory [Paper presentation].Intelligent and Fuzzy Techniques: Smart and Innovative Solutions. INFUS 2020 Conference (Vol. 1197, pp. 415–422).
- Farahani, R. Z., & Asgari, N. (2007). Combination of MCDM and covering techniques in a hierarchical model for facility location: A case study. European Journal of Operational Research, 176(3), 1839–1858. https://doi.org/10.1016/j.ejor.2005.10.039
- Gomes, L., & Lima, M. (1992). TODIM: Basics and application to multicriteria ranking of projects with environmental impacts. Foundations of Computing and Decision Science, 16, 113–127.
- Herrera, F., & Martinez, L. (2000). An approach for combining linguistic and numerical information based on the 2-tuple fuzzy linguistic representation model in decision-making. International Journal of Uncertainty, Fuzziness and Knowledge-Based Systems, 08(05), 539–562. https://doi.org/10.1142/S0218488500000381
- Humphreys, P., McIvor, R., & Chan, F. (2003). Using case-based reasoning to evaluate supplier environmental management performance. Expert Systems with Applications, 25(2), 141–153. https://doi.org/10.1016/S0957-4174(03)00042-3
- Johansson-Stenman, O. (2018). Animal welfare and social decisions: Is it time to take Bentham seriously. Ecological Economics, 145, 90–103. https://doi.org/10.1016/j.ecolecon.2017.08.019
- Kahneman, C., Onar, S. C., & Oztaysi, B. (2015). Fuzzy multicriteria decision-making: A literature review. International Journal of Computational Intelligence Systems, 8, 637–666.
- Kahneman, D., & Tversky, A. (1979). Prospect theory: An analysis of decision under risk. Econometrica, 47(2), 263–292. [Database] https://doi.org/10.2307/1914185
- Kaya, I., Colak, M., & Terzi, F. (2019). A comprehensive review of fuzzy multi criteria decision making methodologies for energy policy making. Energy Strategy Reviews, 24, 207–228. https://doi.org/10.1016/j.esr.2019.03.003
- Lee, A. H. I., Kang, H. Y., Hsu, C. F., & Hung, H. C. (2009). A green supplier selection model for high-tech industry. Expert Systems with Applications, 36(4), 7917–7927. https://doi.org/10.1016/j.eswa.2008.11.052
- Leiser, D., & Azar, O. H. (2008). Behavioral economics and decision making: Applying insights from psychology to understand how people make economic decisions. Journal of Economic Psychology, 29(5), 613–618. https://doi.org/10.1016/j.joep.2008.08.001
- Li, D. F., Huang, Z. G., & Chen, G. H. (2010). A systematic approach to heterogeneous multiattribute group decision making. Computers & Industrial Engineering, 59(4), 561–572. https://doi.org/10.1016/j.cie.2010.06.015
- Liang, Y. Y., Qin, J. D., Martínez, L., & Liu, J. (2020). A heterogeneous QUALIFLEX method with criteria interaction for multi-criteria group decision making. Information Sciences, 512, 1481–1502. https://doi.org/10.1016/j.ins.2019.10.044
- Liao, H. C., & Xu, Z. S. (2015). Approaches to manage hesitant fuzzy linguistic information based on the cosine distance and similarity measures for HFLTSs and their application in qualitative decision making. Expert Systems with Applications, 42(12), 5328–5336. https://doi.org/10.1016/j.eswa.2015.02.017
- Liao, H. C., Wu, D., Huang, Y. L., Ren, P. J., Xu, Z. S., & Verma, M. (2018). Green logistic provider selection with a hesitant Fuzzy linguistic thermodynamic method integrating cumulative prospect theory and PROMETHEE. Sustainability, 10(4), 1291. https://doi.org/10.3390/su10041291
- Liao, H. C., Xu, Z. S., & Zeng, X.-J. (2014). Distance and similarity measures for hesitant fuzzy linguistic term sets and their application in multi-criteria decision making. Information Sciences, 271, 125–142. https://doi.org/10.1016/j.ins.2014.02.125
- Liu, M., Milke, M. W., Heiler, D., & Giovinazzi, S. (2018). Postearthquake decision making on sewer recovery and the roles of damage and repair data: Case study of Christchurch, New Zealand. Journal of Infrastructure Systems, 24(1), 05017007.1–05017007.11. https://doi.org/10.1061/(ASCE)IS.1943-555X.0000406
- Lourenzutti, R., Krohling, R. A., & Reformat, M. Z. (2017). Choquet based TOPSIS and TODIM for dynamic and heterogeneous decision making with criteria interaction. Information Sciences, 408, 41–69. https://doi.org/10.1016/j.ins.2017.04.037
- Lu, L. Y. Y., Wu, C. H., & Kuo, T. C. (2007). Environmental principles applicable to green supplier evaluation by using multi-objective decision analysis. International Journal of Production Research, 45(18–19), 4317–4331. https://doi.org/10.1080/00207540701472694
- Mendoza, R. L. (2018). Bringing the patient back in: Behavioral decision-making and choice in medical economics. Journal of Medical Economics, 21(4), 313–317. https://doi.org/10.1080/13696998.2018.1434532
- Mori, Y., Mizutani, Y., Kang, J. D., & Idota, H. (2018). Upgrade decision-making for earthquake-vulnerable wooden houses using probabilistic damage index functions. ASCE-ASME Journal of Risk and Uncertainty in Engineering Systems, Part A: Civil Engineering, 4(1), 04017037. https://doi.org/10.1061/AJRUA6.0000945
- Noci, G. (1997). Designing “green” vendor rating systems for the assessment of a suppliers environmental performance. European Journal of Purchasing & Supply Management, 3(2), 103–114. https://doi.org/10.1016/S0969-7012(96)00021-4
- Peng, D. H., Gao, C. Y., & Wu, L. X. (2011). TOPSIS-based multi-criteria group decision making under heterogeneous information setting. International Journal of Computational Intelligence Systems, 378–379, 525–530.
- Perrot, P. (1998). A to Z of thermodynamics. Oxford University Press.
- Rani, P., Mishra, A. R., Mardani, A., Cavallaro, F., Alrasheedi, M., & Alrashidi, A. (2020). A novel approach to extended fuzzy TOPSIS based on new divergence measures for renewable energy sources selection. Journal of Cleaner Production, 257, 120352. https://doi.org/10.1016/j.jclepro.2020.120352
- Ren, P. J., Xu, Z. S., & Hao, Z. N. (2017a). Hesitant fuzzy thermodynamic method for emergency decision making based on prospect theory. IEEE Transactions on Cybernetics, 47(9), 2531–2543. https://doi.org/10.1109/TCYB.2016.2638498
- Ren, P. J., Xu, Z. S., Liao, H. C., & Zeng, X.-J. (2017b). A thermodynamic method of intuitionistic fuzzy MCDM to assist the hierarchical medical system in China. Information Sciences, 420, 490–504. https://doi.org/10.1016/j.ins.2017.08.070
- Ren, P. J., Xu, Z. S., Wang, X. X., & Zeng, X. J. (2021). Group decision making with hesitant fuzzy linguistic preference relations based on modified extent measurement. Expert Systems with Applications, 171, 114235. https://doi.org/10.1016/j.eswa.2020.114235
- Rodríguez, R. M., Martínez, L., & Herrera, F. (2012). Hesitant fuzzy linguistic terms sets for decision making. IEEE Transactions on Fuzzy Systems, 20(1), 109–119. https://doi.org/10.1109/TFUZZ.2011.2170076
- Salinas, G. (2017). The decision to attack: Military and intelligence cyber decision-making. Perspectives on Politics, 15(1), 283–284. https://doi.org/10.1017/S1537592716005119
- Sánchez-Lozano, J. M., Serna, J., & Dolón-Payán, A. (2015). Evaluating military training aircrafts through the combination of multi-criteria decision making processes with fuzzy logic. A case study in the Spanish Air Force Academy. Aerospace Science and Technology, 42, 58–65. https://doi.org/10.1016/j.ast.2014.12.028
- Santoyo-Castelazo, E., & Azapagic, A. (2014). Sustainability assessment of energy systems: Integrating environmental, economic and social aspects. Journal of Cleaner Production, 80, 119–138. https://doi.org/10.1016/j.jclepro.2014.05.061
- Strantzali, E., & Aravossis, K. (2016). Decision making in renewable energy investments: A review. Renewable and Sustainable Energy Reviews, 55, 885–898. https://doi.org/10.1016/j.rser.2015.11.021
- Tan, R. P., Zhang, W. D., Yang, L. H., & Chen, S. Q. (2019). Multi-attribute decision-making method based on prospect theory in heterogeneous information environment and its application in typhoon disaster assessment. International Journal of Computational Intelligence Systems, 12, 881–896.
- Torra, V. (2010). Hesitant fuzzy sets. International Journal of Intelligent Systems, 25, 529–539. https://doi.org/10.1002/int.20418
- Verma, M., & Rajasankar, J. (2017). A thermodynamical approach towards group multi-criteria decision making (GMCDM) and its application to human resource selection. Applied Soft Computing, 52, 323–332. https://doi.org/10.1016/j.asoc.2016.10.033
- Verma, M., Rajasankar, J., Anandavalli, N., Prakash, A., & Iyer, N. R. (2015). Fuzzy similarity approach for ranking and health assessment of towers based on visual inspection. Advances in Structural Engineering, 18(9), 1399–1414. https://doi.org/10.1260/1369-4332.18.9.1399
- Von Neumann, J., & Morgenstern, O. (1944). Theory of games and economic behavior. Princeton University Press.
- Wang, M. W., Liang, D. C., Xu, Z. S., & Ye, D. J. (2020). The evaluation of mobile health apps: A psychological perception-based probabilistic linguistic belief thermodynamic multiple attribute decision making method. Journal of the Operational Research Society, 8, 1–15.
- Wang, X. X. (2017). Review of researches on green supplier selection. Economic Research Guide, 4, 194–196.
- Wessel, J., Bidwell, R., & Pullen, E. J. (1984). Decision making in coastal engineering. Water Science and Technology, 16(3–4), 759–765. https://doi.org/10.2166/wst.1984.0109
- Xu, J., Wan, S. P., & Dong, J. Y. (2016). Aggregating decision information into Atanassov’s intuitionistic fuzzynumbers for heterogeneous multi-attribute group decision making. Applied Soft Computing, 41, 331–351. https://doi.org/10.1016/j.asoc.2015.12.045
- Xu, Z. S. (2005). Deviation measures of linguistic preference relations in group decision making. Omega, 33(3), 249–254. https://doi.org/10.1016/j.omega.2004.04.008
- Xu, Z. S. (2007). Multiple-attribute group decision making with different formats of preference information on attributes. IEEE Transactions on Systems, Man, and Cybernetics, Part B (Cybernetics), 37, 1500–1511.
- Yue, C. (2019). A normalized projection-based group decision-making method with heterogeneous decision information and application to software development effort assessment. Applied Intelligence, 49(10), 3587–3605. https://doi.org/10.1007/s10489-019-01473-w
- Zadeh, L. A. (1965). Fuzzy sets. Information Control, 8(3), 338–353. https://doi.org/10.1016/S0019-9958(65)90241-X
- Zadeh, L. A. (1975). The concept of a linguistic variable and its application to approximate reasoning-Part I. Information Sciences, 8(3), 199–249. https://doi.org/10.1016/0020-0255(75)90036-5
- Zhang, B. W., Dong, Y. C., & Herrera-Viedma, E. (2019). Group decision making with heterogeneous preference structures: An automatic mechanism to support consensus reaching. Group Decision and Negotiation, 28(3), 585–617. https://doi.org/10.1007/s10726-018-09609-y
- Zhang, H. H., Kou, G., & Peng, Y. (2019). Soft consensus cost models for group decision making and economic interpretations. European Journal of Operational Research, 277(3), 964–980. https://doi.org/10.1016/j.ejor.2019.03.009
- Zhang, Z. M., & Wu, C. (2014). On the use of multiplicative consistency in hesitant fuzzy linguistic preference relations. Knowledge-Based Systems, 72, 13–27. https://doi.org/10.1016/j.knosys.2014.08.026
- Zheng, J., Wang, Y. M., & Zhang, K. (2020). Solution of heterogeneous multi-attribute case-based decision making problems by using method based on TODIM. Soft Computing, 24(10), 7081–7091. https://doi.org/10.1007/s00500-020-04844-5
- Zhu, B., & Xu, Z. S. (2014). Consistency measures for hesitant fuzzy linguistic preference relations. IEEE Transactions on Fuzzy Systems, 22(1), 35–45. https://doi.org/10.1109/TFUZZ.2013.2245136