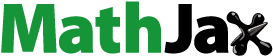
Abstract
This paper assesses the sustainability of pension systems of New Member States of the European Union that have undergone the transition to a market economy and the establishment of a multi-pillar pension model. Investigating pension sustainability from a slightly different perspective, we apply the Mercer CFA Institute Global Pension Index methodology, whose sustainability sub-index measures indicators that have a significant influence on the likelihood that the current pension system will be able to provide benefits into the future. However, this methodology predefines the weights for each indicator, which can be limiting for countries that have large oscillations between indicators. To obtain a sustainability analysis without predefined weights, we apply data envelopment analysis (DEA) to calculate efficiency scores using sustainability indicators as inputs. Furthermore, since DEA generally allows each country to load on their strong indicators as much as possible, allowing thus for self-appraisal, we perform the analysis of the perturbations in the data and examine how these changes affect the overall relative positions of the countries in the set. Also, we explore the cross-efficiency approach to define peer-appraisal as a more objective efficiency score. As a final step, a comparison of all EU Member States provides an overall perspective.
1. Introduction
Population aging poses a major challenge for pension systems sustainability in European countries. Reduced fertility rates and an increase in life expectancy lead to a deterioration in the ratio of the elderly and the working population, which burdens pension systems. Aside from unfavorable demographic trends, pension systems of European countries, particularly former socialist economies, are characterized by poor design features including high shares of early retirement, high system dependency ratios, and fiscal imbalances (Krpan et al., Citation2020). A common strategy used to study European pension systems’ sustainability is by analyzing the indicators offered by the Pensions strand of the Open Method of Coordination (OMC) (Eurostat, Citation2020a). However, Monash Centre for Financial Studies developed a pension index called the Mercer CFA Institute Global Pension Index, which is based on three sub-indices: adequacy, sustainability, and integrity. The overall index value for each system represents the weighted average of the sub-indices. The weightings used are 40 percent for the adequacy sub-index, 35 percent for the sustainability sub-index, and 25 percent for the integrity sub-index which have remained unchanged since the first Index in 2009 (Mercer, Citation2019).
By focusing solely on the sustainability sub-index of the Mercer CFA Institute Global Pension Index our paper uses indicators representing the long-term sustainability of current pension systems on a set of New Member States of the European Union to measure sustainability by employing the new approach in this area, Data Envelopment Analysis (DEA). Measurement and the analysis of the sustainability of the pension systems of New Member States of the European Union (NMS), which is the focus of this paper, is lacking in the Mercer Global Pension Index assessmentFootnote1. The main reason for omitting NMS by the Monash Centre for Financial Studies is the unavailability of data, especially for non-OECD countries, which is why most research on pension systems includes solely the most developed economies. We, therefore, analyzed these countries and investigated their level of sustainability.
The sustainability sub-index, being a part of the Mercer CFA Institute Global Pension Index, includes several indicators that are believed to influence the likelihood that the current system will be able to provide benefits into the future. It encompasses factors concerning the economic importance of the private pension system, its funding level, the length of expected retirement both now and in the future, the labor force participation rate of the older population, the current level of government debt, and the level of real economic growth. The first part of the indicators measures the financing of private pension systems and their economic importance. These include mandatory contributions towards funded public benefits, expressed as a percentage of wages, the proportion of the working-age population that are members of private pension plans, and total assets in funded and private pension arrangements as a percentage of GDP. It is here assumed that a higher share of funding implies a greater level of sustainability since it reduces future dependence on the public pension while also adjusting the expectations of many workers. This is in line with World Bank’s (Citation1994) initial objectives for the introduction of mandatory private pension schemes, which is supported by many international institutions (e.g. OECD).
The next two indicators are general government consolidated gross debt as a share of GDP and real economic growth rate averaged over seven years. They assess the financial capacity of the future government concerning coverage and levels of future pension benefits, among others. The pressure of aging on the long-term sustainability of pension systems and the government’s labor market and pension system’s policies focused on encouraging people to work longer present the next perspective contained in the sustainability sub-index. It is represented by the following indicators: the gap between life expectancy at birth and the state pension age, both now and projected for 2040, then projected old-age dependency ratio in 2040, estimated total fertility rate for the 2015–2020 period, and labor force participation rates for the 55-64 age group, as well as for the population over 65. Lastly, the sustainability sub-index evaluates the possibility of older workers to access part of their retirement savings or pension and continue working, which would enable these individuals to continue contributing to the pension system.
The use of the DEA method on a set of indicators, that were chosen by the Monash Center to be the representatives of the long-term sustainability of current pension systems, for relatively homogeneous countries allows for a comparative comparison of several perspectives of the pension systems of the chosen economies. Additionally, it gives insight into the comparative strengths and weaknesses of an individual country concerning these indicators. Furthermore, the efficiency scores from DEA analysis allow for the evaluation of the performances of the pension systems of the selected economies to their future long-term sustainability. This is especially important in conditions where countries are undergoing reforms to the pension system in an aging environment and financial imbalances. As a next step, sensitivity and cross-efficiency analyses allow us to compare the resilience of the DEA efficiency results with the results that are obtained when an individual indicator is omitted from the analysis or when weights from other economies from DEA are used. Finally, a comparison of all EU Member States is conducted to provide an overall perspective.
The paper is structured as follows: the first section discusses the sustainability sub-index and previous empirical research. The second section is concerned with the Data Envelopment Analysis methodology. The third section presents the data and delivers our results. The fourth section is devoted to sensitivity and cross-efficiency analysis. The fifth section brings the research conclusions.
2. Literature review
A large number of existing studies have examined the sustainability of pension systems. In a broader sense, these can be divided into sustainability analyses within the Overlapping Generations (OLG) framework or other complex forecasting models, which are often single-country studies; and comparative analyses that aim to classify or rank countries using a set of sustainability indicators or a synthetic indicator. Several OLG studies of pension systems and pension reforms are important to mention. Seminal contributions have been made by İmrohoroglu et al. (Citation1995), Razin and Sadka (Citation1999), Fougere and Merette (Citation1999), Börsch-Supan et al. (Citation2006), Verbič et al. (Citation2006), Boldrin and Montes (Citation2009), Fanti and Gori (Citation2010), Georges et al. (Citation2016), Pavković (Citation2021) and many others. A different method employed by De La Fuente and Doménech (Citation2013) is a preliminary estimate of the impact of the reform of the Spanish public pension system constructed using a decomposition of public pension expenses as a percentage of GDP, whereas the other half is related to the evolution of the ‘generosity’ ratio.
In the same manner, European Commission (Citation2009), Moscarola (Citation2009), Pallares-Miralles et al. (Citation2012), Marcinkiewicz and Chybalski (Citation2014), Chybalski (Citation2014), Krpan et al. (Citation2019), and Škuflić et al. (Citation2020) approach the analysis of sustainability by studying public pension expenditure as a percentage of GDP that Eurostat (2020a) considers as the main indicator of sustainability. In 2018, with 6.48 percent of GDP, Estonia recorded the lowest share of pension expenses among NMS, while Croatia and Poland had the largest pension expenditure, amounting to more than 10 percent (Eurostat, Citation2020b). Eurostat (2020a) lists the employment rate of older workers and the duration of working life, as two other primary indicators of sustainability, but these will be included in this study. World Bank’s experts such as Kasek et al. (Citation2008), Pallares-Miralles et al. (Citation2012) present more than twenty pension sustainability indicators, including demographic factors, labor market indicators, replacement rates, the structure of pension expenditure, and many more. Holzmann et al. (Citation2004) argue that it is important to consider unfunded public pension liabilities as part of an assessment of the overall fiscal situation of pension systems and show that almost all of the NMS rank high in levels of implicit pension debt (Slovenia, Romania, and Poland are in the first five among low and middle-income countries).
However, most authors recognize the need for developing different techniques and indicators to approach the pension sustainability issue. In recent years, synthetic indicators have become one of the most commonly used tools of analytical measurement in practice in many fields of social reality (Alonso-Fernandez et al., Citation2018), and also in pension economics. The two most important synthetic indicators of pension systems include The Allianz Pension Sustainability Index (PSI) and Mercer CFA Institute Global Pension Index (Mercer, 2019, Citation2021), which is used in this research. The latest version of the PS index available is from 2016 and analyzes 54 countries according to the following indicators: old-age dependency ratio, level of pension benefit from the first pillar and coverage of workforce, legal/effective retirement age, the strength of the funded pillar and reserve fund (as a percent of GDP), pension payments over GDP, public indebtedness over GDP, and need for welfare support. Hence, the PS index encompasses private pension schemes as a determinant of pension sustainability, as does the Mercer CFA index, but unlike the Mercer CFA index includes Central and Eastern European (CEE) economies. Their estimates show that Latvia, Estonia, and Lithuania top the list for CEE countries, while Estonia managed to even enter into the top ten in the overall ranking. Conversely, Croatian and Slovenian pension systems remain at the bottom of the CEE list.
Several authors have developed their pension indicators, such as Chybalski (Citation2016) and Alonso-Fernandez et al. (Citation2018). Chybalski (Citation2016) points out that the multidimensional nature of his indicators enables comparisons of many different empirical pension systems. Since the calculation of the indicators does not require prior data standardization, this method is more resistant to the relativeness of the measurement and comparisons in cross-section studies. However, the relativeness enables the analysis since the efficiency-inefficiency border is not determined. Therefore, the proposed approach is efficient only when a few pension systems are compared, and then it is possible to rank pension systems. Chybalski (Citation2016) shows that the fastest increase in efficiency is reported by Baltic states: Lithuania, Latvia, and Estonia, once again confirming a relatively higher level of pension sustainability in these economies. Different from Chybalski (Citation2016) that uses the Open Method of Coordination (OMC) definitions and methodology, this study offers a test of the Mercer CFA Institute Global Pension Index methodology for the pension sustainability issue. Additionally, in assessing the sustainability of New Member States of the European Union, using indicators proposed by the Monash Centre for Financial Studies, this paper uses the new DEA methodology in this area, while also emphasizing private pension schemes, a path rarely taken in the pension sustainability analyses. A closer look at the DEA methodology and relevant literature is subject of the next section.
3. Data envelopment analysis
In economics, efficiency is a concept defined through the ratio of outputs and inputs. In this paper, we use the DEA methodology and the slack based measure (SBM) of efficiency to evaluate the sustainability of pension systems of eleven New Member States of the European Union. DEA models assume that the decision-making units (DMUs) use a certain number of inputs to produce a certain number of outputs. Following the logic of a production process, a DMU aims to produce more output with the given level of inputs or to use as small inputs as possible to achieve a certain level of output. The basic idea is to detect a DMU which, relative to other DMUs, uses its inputs in a more efficient way to produce its outputs, or achieves higher outputs with its inputs. In this sense, DEA can be used to estimate relative efficiency not only of production units but also of other homogenous and comparable units that can be described in terms of their inputs and outputs. However, in the efficiency analysis concerned with the actual production process, the inputs and the outputs are distinctively defined. In other cases, efficiency is a relative feature that is defined by a set of criteria stemming from the nature of the study. Following the defined set of criteria, variables in DEA are divided into the outputs, whose values we prefer to be as great as possible, and inputs, whose values we prefer to be as small as possible.
This study employs variables that we consider as potential determinants of the sustainability of a pension system, treating some of them as inputs and the other ones as outputs. This allows us to define the relative efficiency of pension systems in EU countries in the area of sustainability and to observe their positions relative to others. The relative efficiency of sustainability in a particular pension system defines its sustainability prospects relative to others, which is why we treat this analysis as the sustainability analysis. The greatest advantage of DEA methodology is that it enables the efficiency analysis based on multiple criteria. Furthermore, pre-defined weights for particular variables are not required. The method generally finds weights for each DMU that puts this DMU in the best possible position regarding others. This allows excluding any subjectivity from the study and allows detecting the comparative strengths or weaknesses of a particular DMU. Also, the comparative advantages of DMU can be used to mitigate deficiencies in a certain amount. This approach enables a new perspective on the sustainability analysis compared to the existing ones, especially in the pension systems' sustainability assessment literature.
Although similar to the traditional DEA models, such as CCR (Rhodes et al., Citation1978) or BCC (Banker et al., Citation1984), SBM represents the extension of traditional models and allows dealing with problems where assumptions of traditional models are too limited. It was proposed by Tone (Citation2001). The first step is to introduce the assumptions of the model. Let there be N DMUs that are all characterized by the same set of inputs that ‘produce’ the same set of outputs
Then
and
Y are input and output matrices, respectively, for the observed set of DMUs. The production possibilities set is defined as:
(1.1)
(1.1)
The SBM model is non-radial, meaning that it takes into account the case in which the observed changes of inputs cause disproportionate changes of outputs. The original SMB measure is unit invariant concerning units of data and is monotone decreasing in each slack in input and output. The SBM of efficiency can take values between zero and one, but in contrast to traditional DEA models, the unity (indicating efficiency) is obtained if and only if DMU is on the efficiency frontier of the production possibilities set with no input or output slacks (strongly efficient).
While traditional models ignore possible slacks, SMB deals with slacks directly by punishing undesirable excesses or shortfalls. However, when the number of DMUs is too small compared to the number of employed criteria, the measure suffers from insufficient discriminatory power, as do all DEA models. This problem can be solved by the use of the super-efficiency models. The super-efficiency model for SBM (super-SBM) is proposed by Tone (Citation2002). The production possibilities set now excludes the evaluated DMU0 and in this case turns to:
(1.2)
(1.2)
The formulation of the super-SBM model is as follows. In the input-oriented model, the measure of SBM efficiency with variable returns to scale is obtained by solving the following program for each DMU0=DMUj (Tone, Citation2002):
(1.3)
(1.3)
In (1.3) and
are defined as:
(1.4)
(1.4)
(1.5)
(1.5)
and derived from the projection of DMU0 on the production possibility frontier where
is a vector of input excess and
a vector of output shortfall. The rationality for this measure is to minimize a sort of weighted l1 distance from an observed DMU0 to the production possibility set excluding the DMU0. The procedure for solving this program can be found originally in Tone (Citation2002).
In case of the whole EU reference set, some data was missing, so it was replaced with a significantly large value (for inputs) or a small value (close to zero) (for output), as proposed by Kuosmanen (Citation2009). In this way, we were able to keep a country in the sample, but these 'penalty' values are not recognized as strengths that contribute to the efficiency of a considered DMU and therefore are not included in the objective function (zero weight is assigned). Furthermore, since the model does not work with negative data, and Greece had a negative value for variable S8 (real economic growth rate averaged over seven years), Greece was excluded from the analysis.
Software DEA Solver Pro v.12 was used for the analysis. The obtained efficiency scores indicate how efficient are the observed DMUs. The higher the efficiency score, the more efficient is DMU compared to others. Thus, the model allows the ranking of DMUs by their efficiency score. Slacks can indicate which variable needs the most attention, in terms of possible increases or decreases to reach the production possibilities frontier.
In classical DEA models, as well as in the model (1.3), it is assumed that the observed set of DMUs has at least one input and at least one output. However, in many cases, especially in those where DMUs are not actual production units, DMUs can be described either only with inputs or only with outputs. In those situations, Kostrzewa et al. (Citation2011) propose a modified approach in DEA, in which it is enough to assume that all DMUs have only one input (or output) which has the same value for all DMUs (for example, that all DMUs have one input equal to one). In such models, the obtained result is a modified synthetic indicator of efficiency given only values of input or outputs.
The last part of our study includes cross-efficiency and sensitivity analysis. As previously mentioned, DEA models allow each DMU to put itself in the best possible position regarding others by loading on its best qualities. The cross-efficiency model is based on the idea of how well a DMU would position if assigned weights of other DMUs. This approach was developed by Sexton et al. (Citation1986) and further developed by Doyle and Green (Citation1994). The cross-efficiency method allows peer evaluation rather than self-evaluation (Cook & Zhu, Citation2015). The output of the cross-efficiency analysis is a cross-efficiency matrix, presented in . Each Eij in the cross-efficiency matrix is an efficiency score of a DMUi using the optimal weights of a DMUj. The row and column arithmetic averages have their special interpretations. The column averages are interpreted as peer appraisals, where row averages are the average appraisal of peers.
Table 1. Cross-efficiency matrix.
Finally, we perform a sensitivity analysis of the efficiency scores by excluding one by one variable. By doing this, we aim to find out how heavy each DMU is loading on a specific variable and conclude whether its position is resilient enough to changes in the variable set.
4. Data and findings
Our sample contains data describing the pension systems of 11 New Member States (NMS) of the European Union (Bulgaria, Croatia, Czech Republic, Estonia, Hungary, Latvia, Lithuania, Poland, Romania, Slovakia, and Slovenia). The data includes the values of 12 indicators that are chosen to recreate the structure of the Mercer CFA Institute Global Pension Index sustainability sub-index, and a thorough explanation of the methodology can be found in Mercer (Citation2021). All values are taken for the year 2018 and represent common indicators available in statistical databases:
Proportion of the working-age population that are members of private pension plans (S1),
Total assets in funded and private pension arrangements as a percentage of GDP (S2),
Gap between life expectancy at birth and the state pension age (2020) (S3a),
Projected gap between life expectancy at birth and the state pension age in 2040 (S3b),
Projected old-age dependency ratio in 2040 (S3c),
Estimated Total Fertility Rate (TFR) for 2015-2020 (S3d),
Mandatory contributions set aside for retirement benefits as a percentage of wages (S4),
Labor force participation rate (55-64) (S5a),
Labor force participation rate (65+) (S5b),
General government consolidated gross debt as a percentage of GDP (S6),
Older workers (65+) yes/no (S7),
and Real economic growth rate averaged over seven years (four previous years, three estimated) (S8).
4.1. Descriptive statistics and main results
Data on private pension schemes (indicators S1 and S2) were taken from the OECD database - Pension Markets in Focus (2019) that provide information on funded and private pension systems across the world. Furthermore, European Commission (Citation2018) published the so-called Country fiches on pension projections that were prepared together with national pension ministries or offices that give information on projected life expectancy and state retirement age (indicators S3a, S3b), as well as on share of mandatory contributions for private schemes (indicator S4). Most of the data were taken from the Eurostat database (2020 b) (indicators S3c, S5a, S5b, S6, and S7). Finally, estimates of total fertility rates (indicator S3d) were taken from the United Nations database (Citation2020), whereas rates of real economic growth (indicator S8) were calculated using the International Monetary Fund’ (Citation2020) data and projections.
In our analysis, all variables are indicators that are believed to affect the long-term sustainability of the pension system. Therefore, we treat all of them as inputs. Since we only have inputs in our analysis, we assume that all DMUs have a unit output. The indicators whose higher values are believed to be positively linked with the concept of the long-term sustainability of the pension system are used in the analysis in their inverse form. Therefore, variables S1, S2, S3d, S4, S5a, S5b, S7, and S8 are used in the analysis as the following inputs: 1/S1, 1/S2, 1/S3d, 1/S4, 1/S5a, 1/S5b, 1/S7, and 1/S8. The descriptive statistics of the data are presented in , to give an aggregate overview of the analyzed indicators and get an initial insight into the situation in the economies in the year 2018. A more thorough analysis of the time dimension of the data is available in Škuflić et al. (Citation2020), where cross-sectional dimension is also studied within a panel setting.
Table 2. Descriptive statistics.
Although pension reforms are aimed at increasing the state pension age and consequently reducing time spent in retirement and expenditures on retirement benefits, the average gap between life expectancy at birth and the state pension age is expected to increase until 2040 from 14 to 16.31 years (according to the results from for indicators S3a and S3b). The highest increase is expected for Bulgaria, Hungary, and Latvia, while Croatia is the only analyzed economy where the reduction in the time spent in retirement is expected. The projected share of the population over 65 in the working-age population for 2040 (an indicator S3c) ranges from 39,4 percent in Slovenia to 52 percent in Lithuania. Data on the indicators of the labor force participation of older workers (S5a and S5b), especially those over 65 (S5b), show relatively high variability, ranging from only 2.95 percent in Croatia to 13.98 percent in Estonia. Below average are also Hungary, Slovakia, Slovenia, Poland, and Bulgaria. The same indicator for the population 55-64 ranges from 44.75 percent in Croatia to 73.85 percent in Lithuania. The other two Baltic economies join Lithuania in recording the highest labor force participation rate of the elderly. The average proportion of the working-age population that are members of private pension arrangements is 63.8 percent with the relatively highest variability among the NMS. It ranges from 18.7 percent in Hungary to 100 percent in Latvia.
The ability of future governments to pay pension benefits, represented by the indicator S6, is reduced for Croatia, Slovenia, and Hungary, recording general government consolidated gross debt of above 70 percent of GDP. The above-average is also Slovakia and Poland. Estonia, on the other hand, recorded 8.4 percent. Another indicator that is expected to affect the government's financial position is the indicator S8. The real economic growth rate, averaged over seven years, ranges from 2.8 percent in Croatia to 4.33 percent in Romania. Finally, the average share of total assets in funded and private pension arrangements was 11.3 percent, ranging from 5.23 percent in Romania to 27.35 percent in Croatia. Since there are 12 variables/indices in our model, and we also have 11 DMUs, standard SBM is not discriminatory enough. Therefore, we use the super-SBM model. Super efficiency models also allow efficiency ranking or DMUs (Chen & Du, Citation2015). The results obtained by solving the model (1.3) are presented in . Scores greater or equal to 1 indicate pension systems which are relatively more successful than the others according to the given criteria, indicating that they have greater sustainability prospects. The efficiency scores exist on an ordinal scale which is used for ranking. For example, score 1.3 is better than 1, but the distances between them are unknown, meaning that 1.3 is not necessarily 30% better than 1.
Table 3. Super-SMB scores and ranks.
Having the greatest l1 distance from the production possibilities set (1.2), Estonia is recognized as the economy with the highest efficiency score or overall best long-term sustainability perspective of the pension system in comparison to the other countries in the set. Estonia is followed by Croatia and Latvia. Slovenia and Slovakia are at the bottom of the list. Other countries are in between. The reason for such scores and rankings could be found by analyzing Equationequations (1.4)(1.4)
(1.4) and Equation(1.5)
(1.5)
(1.5) which represent each DMU in terms of its projection on the nearest point on the production possibilities frontier (whereas that particular DMU is excluded from the set), corrected for slacks. In this way, slacks in the value of a certain indicator, expressed in percentages and displayed in , indicate deviations from the projection of a particular DMU on the production possibilities frontier for the analyzed indicator. Therefore, slacks allow us to detect the weaknesses and strengths of the individual DMU in the particular aspect of the long-term sustainability of the pension system. This alternative rank is based on the sum of the negative percentage deviations.
Table 4. Deviations (in percent) from projections on the production possibilities frontier.
To understand this alternative ranking, presents positive and negative values of slacks. Positive values of slacks in indicate that the analyzed economy has positive percentage deviations in the value of the observed indicator from projections on the frontier of the production possibilities set. They, therefore, imply the analyzed economy’s strengths in this particular aspect of the long-term sustainability of the pension system. On the other hand, negative values of slacks indicate that the analyzed country should strive to reduce the value of that indicator since it negatively deviates from its projection to the frontier of the production possibilities set for this particular indicator. In this way, negative deviations indicate possible improvements in certain areas that affect the long-term sustainability of the pension system of the analyzed economy.
The results presented in can be interpreted for the individual country, but also each indicator. They show that Estonia has comparative strengths in almost all indicators, except in the S3a (Gap between life expectancy at birth and the state pension age (2020)) and S8 (Real economic growth rate averaged over seven years (four previous years, three next years estimated). Its comparative strength is the highest in the value of the indicator S6 (variable General government consolidated gross debt as a percentage of GDP). Specifically, its projection on the production possibilities frontier has the value of this indicator 165 percent higher than the actual registered value for Estonia, implying that the general gross debt of Estonia is significantly lower than in the rest of NMS. Similarly, Estonia’s projection on the production possibilities frontier has the value of the input 1/S5b (S5b - Labor force participation rate (65+)) 139 percent higher than the actual registered value for Estonia. Note that the first eight inputs in are inverse values of the used variables. Therefore, the interpretation should be adjusted accordingly.
The same principle is applied in the interpretation of other indicators. reveals why Croatia is ranked as the second-best concerning the efficiency score presented in and Latvia as the third best. Precisely, Croatia has a relatively high positive slack in input 1/S2 indicating a relatively high value of variable S2 (Total assets in funded and private pension arrangements as a percentage of GDP), which is thought to be positively related to the sustainability of the pension system. Here it is important to note that, among the observed countries, Croatia is the only one that is not drifting away from the funded pensions, by reducing its relative importance. Latvia’s comparative strengths are variables S3a (Gap between life expectancy at birth and the state pension age in 2020), and variable 1/S1 (S1 - Proportion of the working-age population that are members of private pension plans). Note that Romania has a positive slack in variable 1/S8 (Real economic growth rate averaged over seven years (4 years, 3 estimated), but the model recognized that Romania had no positive deviations in other inputs. Other countries also did not have such distinctive preferred differences relative to others.
The vast majority of the analyzed economies (except for Estonia) show relatively poorer results concerning the two indicators: S6 (General government consolidated gross debt as a percentage of GDP) and S5b (Labor force participation rate (65+)). This conclusion is carried out based on negative deviations from their projections to the production possibilities frontier in the values of these two indicators. Estonia set a high standard for other economies, registering the lowest general government consolidated gross debt as a percentage of GDP of 8.4 percent, with the second in line being Bulgaria with 22.3 percent.
Furthermore, Estonia also registered the highest labor force participation rate of the population over 65, with 13.98 percent. The other two Baltic economies, Latvia and Lithuania followed with 9,78 percent and 9,35 percent, respectively. Moreover, we observe that Croatia and Hungary have very large negative slacks in many variables. Croatia shows the highest negative deviations concerning the general government consolidated gross debt as a share of GDP. Also, the Croatian Government should encourage higher labor force participation of the elderly, especially those over 65. Slovakia, Hungary, Poland, and Bulgaria should follow the same strategy. When it comes to the economic growth rate, Romania shows its comparative strength. But, its weakness lies in the lowest share of total assets in funded and private pension arrangements in GDP among NMS.
Since Croatia is ranked very high, we conclude that efficiency scores of the model (1.3) are obtained by putting a lot of weight on the comparative strengths of DMUs. If we sum all positive percentage deviations for each DMU and rank countries accordingly, from highest to lowest values, we would obtain the ranking presented in the third column of . However, if we were to rank DMUs based on the sum of the negative percentage deviations, we would get a different ranking. This alternative ranking is presented in the last column of , called Alternative rank (Alt. rank). Estonia is still at the top of the list, but the position of Croatia and Hungary has changed significantly. On the other hand, the position of Bulgaria and Lithuania is significantly improved. However, when the best and the worst country were excluded from the sample, Latvia’s pension system is proven as the most efficient and Croatia’s as the least efficient.
The model assumes that each projection on the production possibility frontier is possible and can be expressed as a combination of other DMUs, called peers. The peers form a reference set of a DMU. The relative strength of a DMU within the observed set can also be evaluated in terms of frequency of appearance in the reference set of other DMUs (Friedman et al., Citation2002). shows reference sets for all DMUs.
Table 5. Reference sets and peers.
A DMU in the first column is projected as a combination of other using the weights shown in the table (empty cells indicate that a specific DMU is not in the reference set of an observed DMU) (Gardijan et al., Citation2021). The last row of shows the number of occurrences of a specific DMU in the reference sets of other DMUs. It is obvious that the most efficient DMU, which is Estonia, can be ranked first in the light of this criterion as well. It is followed by Lithuania. Although Croatia is ranked as a second given its efficiency score, it now shows some difficulties and the lack of relative strength in terms of the long-term sustainability perspectives of the pension system.
Before running sensitivity and cross-efficiency analyses, the efficiency of pension systems of eleven New EU Member States was compared to the rest of the EU member states. presents the newest rankings.
Table 6. Comparison of the Efficiency of Pension Systems of EU Member States.
The leading countries among the New EU Member States, Latvia and Estonia, are now placed high in the rankings of all EU member states (excluding Greece), while others are spread across the board. Comparing the average rankings older Member States do have a better average ranking (13.29) than the new members (15). However, the date of the accession is not a relevant determinant of the efficiency of the pension systems (given the used criteria).
4.2. Sensitivity and Cross-Efficiency analysis
The results of the efficiency of the pension systems of selected countries presented so far in respect of the indicators that the Monash Centre considers as indicators of the long-term sustainability of current pension systems are based on the use of 12 indicators. Below we conduct the sensitivity analysis of the results obtained and present the results of 12 analyses that have been obtained so that in each next step one indicator is excluded from the analysis. In this way, the existing efficiency assessment relies on the use of 11 indicators. This approach allows us to detect how the order of economies is changing and how important a particular indicator is for the final rating of the country.
shows that regardless of which indicator is excluded from the analysis, Estonia remains first. Croatia, which was ranked second shows great dependence on the second indicator because when it is taken from the analysis, Croatia takes ninth place. In this context, Latvia takes second place. In all other cases, except where the analysis omits the ninth indicator, Latvia takes third place. In addition to Estonia and Latvia, high resilience also shows Poland. On the other hand, Slovakia takes the last place, regardless of which indicator is omitted from the analysis. Slovenia also shows weaker sustainability results, which do not depend on the chosen indicator. On the other hand, Romania and Hungary, like Croatia, show dependence on the indicators in which they have a good relative position.
Table 7. Rankings when one indicator is dropped from the analysis (12 columns for each new ranking).
The final step is to present the results of the cross-efficiency analysis that evaluates the efficiency of a country in such a way that it uses the optimal weights of other countries in the efficiency assessment.
presents the efficiency scores obtained by a cross efficiency model, from which similar conclusions are drawn as from the previous part of the analysis. The most resilient from the top are Estonia, Latvia, and Poland, while the bottom is still held by Lithuania, Slovakia, and Slovenia. The biggest change in ranking concerning our results shows Croatia.
Table 8. The results of the cross-efficiency analysis.
5. Conclusion
This paper employs Data Envelopment Analysis (DEA) to obtain the sustainability assessment of pension systems of eleven New Member States of the European Union: Bulgaria, Croatia, the Czech Republic, Estonia, Hungary, Latvia, Lithuania, Poland, Romania, Slovakia, and Slovenia. The conducted analysis is based on several indicators that compose the sustainability sub-index of the Mercer CFA Institute Global Pension Index, developed by the Australian Monash Center for Financial Studies. The chosen indicators are believed to influence the long-term sustainability of current pension systems of NMS, whereby several aspects are emphasized: the economic importance of the private pension system, its funding level, the length of expected retirement both now and in the future, the labor force participation rate of the older population, the current level of government debt and the level of economic growth. In particular, it is believed that the chosen indicators affect the likelihood that the current pension system will be able to provide benefits into the future. Accordingly, the DEA methodology and the super slack based measure (SBM) of efficiency is used in obtaining efficiency scores for pension systems of analyzed economies. All indices are treated as inputs, in their original or inverse form, whereby it is assumed that all DMUs (analyzed economies) have a unit output.
Estonia is recognized as the economy with the highest efficiency score or overall best long-term sustainability perspective of the pension system in comparison to the other countries in the set. Results show that Estonia has comparative strengths in almost all indicators, except in the Gap between life expectancy at birth and the state pension age for 2020, and the real economic growth rate averaged over seven years. Estonia also stands out in other empirical studies. Price and Rudolph (Citation2013) and Altiparmakov and Nedeljković (Citation2018) acknowledge Estonia as one of the rare countries that successfully implemented the austerity measures that are mandatory to finance pension privatization. Krpan et al. (Citation2019) show that Estonia ranks first among NMS in the labor force participation rate of the elderly and very high in the fiscal balance of the pension system. When comparative strengths are given the most weight, Estonia is followed by Croatia and Latvia. Slovenia and Slovakia are at the bottom of the list. However, if we were to rank DMUs based on their weaknesses, the position of Croatia and Hungary would deteriorate significantly, and those of Bulgaria and Lithuania would improve. The vast majority of the analyzed economies (except for Estonia) show relatively poorer results concerning the two indicators: General government consolidated gross debt as a percentage of GDP and Labor force participation rate of the population over 65. Therefore, governments of New Member States should generally encourage higher labor force participation of the elderly, especially those over 65 to improve the sustainability of their pension systems. Finally, the sensitivity and cross-efficiency analyses are conducted to detect how the order of economies is changing when a particular indicator is dropped from the analysis and how important a particular indicator is for the final rating of the country. Additionally, cross-efficiency analysis uses the weights of the other countries in obtaining the efficiency results. Both results show that the most resilient economies from the top are Estonia, Latvia, and Poland, while the bottom is still held by Lithuania, Slovakia, and Slovenia. The biggest change in ranking concerning our results shows Croatia.
There are several limitations to this approach to measuring pension sustainability. A limitation already presented is the notion of the role of private pension schemes in the sustainability of pension systems. They are included in this study under the assumption that they reduce the burden of aging in the first pension pillar, although their importance also depends on the way the transition is carried out. Indicator of part-time employment and the possibility of phased retirement is used according to the methodology of Mercer (2019), but the index gives a score between 0 and 2 depending on the circumstances and practice, meaning it is not an objectively measured factor, so this could be enhanced in future studies. Also, given the extremely large number of sustainability indicators and related factors, it is possible to expand the set of indicators in future research. Future research could focus on combining a greater number of indicators of both public and private pension schemes, with different sets of economies.
In summary, this paper shows how different techniques and indicators should be used to approach the concept of pension sustainability. The present findings confirm the rankings of New Member States from different empirical studies, emphasizing the favorable situation in Baltic countries. Aside from the common classification and clustering of economies, the results provide evidence to comparative strengths and weaknesses of analyzed pension systems, forming a basis for suggestions to improve each retirement income system.
Disclosure statement
There are no relevant financial or non-financial competing interests to report.
Notes
1 Poland is the only New Member State currently included in the Mercer CFA Institute Global Pension Index.
References
- Alonso-Fernandez, J., Meneu-Gaya, R., Devesa-Carpio, E., Devesa-Carpio, M., Dominguez-Fabian, I., & Encinas-Goenechea, B. (2018). From the replacement rate to the synthetic indicator: A global and gender measure of pension adequacy in the European Union. Social Indicators Research, 138(1), 165–186. https://doi.org/10.1007/s11205-017-1653-x
- Altiparmakov, N., & Nedeljković, M. (2018). Does pension privatization increase economic growth? Evidence from Latin America and Eastern Europe. Journal of Pension Economics and Finance, 17(1), 46–84. https://doi.org/10.1017/S1474747216000160
- Banker, R. D., Charnes, A., & Cooper, W. W. (1984). Some models for estimating technical and scale inefficiencies in data envelopment analysis. Management Science, 30(9), 1078–1092. https://doi.org/10.1287/mnsc.30.9.1078
- Barr, N. (2000). Reforming pensions: truths, myths, and policy choices. IMF Working Paper WP/00/139. IMF. https://doi.org/10.5089/9781451856033.001
- Boldrin, M., & Montes, A. (2009). Assessing the efficiency of public education and pensions. Journal of Population Economics, 22(2), 285–309. https://doi.org/10.1007/s00148-007-0178-z
- Börsch-Supan, A., Ludwig, A., & Winter, J. (2006). Ageing, pension reform and capital flows: A multi-country simulation model. Economica, 73(292), 625–658. https://doi.org/10.1111/j.1468-0335.2006.00526.x
- Chen, Y., & Du, J. (2015). Super-efficiency in data envelopment analysis. In Z. Joe (Ed.), Data envelopment analysis: A handbook of models and methods. Springer. 381–414.
- Chybalski, F. (2014). Financial Stability of Pension Systems: A Cross Country Analysis, in: Proceedings of the 14th International Conference on Finance and Banking, Karvina, Czech Republic
- Chybalski, F. (2016). The multidimensional efficiency of pension system: Definition and measurement in cross-country studies. Social Indicators Research, 128(1), 15–34. https://doi.org/10.1007/s11205-015-1017-3
- Cook, W. D., & Zhu, J. (2015). DEA cross efficiency. In Zhu (Ed.), Data envelopment analysis: A handbook of models and methods. Springer. (2015)23–43.
- De La Fuente, A., & Doménech, R. (2013). The financial impact of Spanish pension reform: A quick estimate. Journal of Pension Economics and Finance, 12(1), 111–137. https://doi.org/10.1017/S1474747212000182
- Doyle, J., & Green, R. (1994). Efficiency and cross-efficiency in DEA: Derivations, meanings and uses. Journal of the Operational Research Society, 45(5), 567–578. https://doi.org/10.1057/jors.1994.84
- European Commission. (2009). Portfolio of indicators for the monitoring of the European strategy for social protection and social inclusio–2009 update. Employment, Social Affairs and Equal Opportunities DG.
- European Commission. (2018). Country fiches on pension projections, various issues. https://ec.europa.eu/info/topics/economy-finance-and-euro
- Eurostat. (2020a). Pensions strand of the open method of coordination. https://ec.europa.eu/eurostat/web/employment-and-social-inclusion-indicators/social-protection-and-inclusion/pension
- Eurostat. (2020b). Database. https://ec.europa.eu/eurostat/data/database
- Fanti, L., & Gori, L. (2010). Increasing PAYG pension benefits and reducing contribution rates. Economics Letters, 107(2), 81–84. https://doi.org/10.1016/j.econlet.2009.01.001
- Fougere, M., & Merette, M. (1999). Population ageing and economic growth in seven OECD countries. Economic Modelling, 16(3), 411–427.
- Friedman, L., Sinuany-Stern, Z., & Adler, N. (2002). Review of ranking methods in the data envelopment analysis context. European Journal of Operational Research, 140(2), 249–265.
- Gardijan Kedžo, M., & Lukač, Z. (2021). The financial efficiency of small food and drink producers across selected European Union countries using data envelopment analysis. European Journal of Operational Research, 291(2), 586–600. https://doi.org/10.1016/j.ejor.2020.01.066
- Georges, P., Lisenkova, K., Mérette, M., & Zhang, Q. (2016). An overlapping generations computable general equilibrium (OLG-CGE) model with age-variable rate of time preference. NIESR discussion paper 458.
- Holzmann, R., Palacios, R., & Zviniene, A. (2004). Implicit pension debt: Issues, measurement and scope in international perspective. Social Protection Discussion Paper Series, 30153, 1–38.
- İmrohoroglu, A., İmrohoroglu, S., & Joines, D. H. (1995). A life-cycle analysis of social security. Economic Theory, 6(1), 83–114. https://doi.org/10.1007/BF01213942
- International Monetary Fund. (2020). World economic outlook. https://www.imf.org/external/datamapper/datasets/WEO
- Kasek, L., Laursen, T., & Skrok, E. (2008). Sustainability of pension systems in the new EU member states and Croatia: Coping with aging challenges and fiscal pressures. World Bank Publications.
- Kostrzewa, K., Okniński, A., & Radziszewski, B. (2011). Data envelopment analysis without input or output. In: Ł. Piotr (Ed.), Production engineering in making. AGH University of Science and Technology Press.
- Krpan, M., Pavković, A., & Galetić, F. (2019). Comparison of Sustainability Indicators of Pension Systems in the New EU Member States, In D. Tipurić and D. Hruška (Eds.), Proceedings of the 7th International OFEL Conference on Governance, Management and Entrepreneurship: Embracing Diversity in Organisations.
- Krpan, M., Pavković, A., & Žmuk, B. (2020). Cluster analysis of new EU member states' pension systems. Interdisciplinary Description of Complex Systems, 18(2-B), 208–222. https://doi.org/10.7906/indecs.18.2.10
- Kuosmanen, T. (2009). Data envelopment analysis with missing data. Journal of the Operational Research Society, 60(12), 1767–1774. https://doi.org/10.1057/jors.2008.132
- Marcinkiewicz, E., & Chybalski, F. (2014). How to measure and compare pension expenditures in cross-country analyses? Some methodological remarks. International Journal of Business and Management, 2(4), 43–59.
- Mercer. (2019). Melbourne mercer global pension index. Monash Centre for Financial Studies.
- Mercer. (2021). Mercer CFA institute global pension index 2021. Monash Centre for Financial Studies and CFA Institute.
- Moscarola, F. C. (2009). Measuring the sustainability of pension systems through a microsimulation model. The case of Italy. ENEPRI Research Report 66 AIM WP 7.1.
- OECD. (2019). Funded and private pensions: Pension Markets in Focus. http://www.oecd.org/pensions/private-pensions/pensionmarketsinfocus.htm
- Pallares-Miralles, M., Romero, C., & Whitehouse, E. (2012). International patterns of pension provision II: A worldwide overview of facts and figures. Social Protection and Labor Discussion Paper 70319.
- Pavković, A. (2021). An overlapping generations general equilibrium model of croatian pension system . [Doctoral thesis]. University of Zagreb, Faculty of Economics and Business.
- Price, W. J., & Rudolph, H. (2013). Reversal and reduction, resolution and reform—lessons from the financial crisis in Europe and Central Asia to improve outcomes from mandatory private pensions. World Bank.
- Razin, A., & Sadka, E. (1999). Migration and pension with international capital mobility. Journal of Public Economics, 74(1), 141–150. https://doi.org/10.1016/S0047-2727(99)00038-9
- Rhodes, E., Cooper, W. W., & Charnes, A. (1978). Measuring the efficiency of decision making units. European Journal of Operational Research, 3(4), 429–444.
- Sexton, T. R., Silkman, R. H., & Hogan, A. J. (1986). Data envelopment analysis: Critique and Extensions. In Silkman RH (Ed.) Measuring efficiency: an assessment of data envelopment analysis., 32. Jossey-Bass. 73–105.
- Škuflić, L., Krpan, M., & Pavković, A. (2020). Public pension expenditure in the new EU member states: A panel data approach. Finance a úvěr Czech Journal of Economics and Finance, 70(3), 216–243.
- Tone, K. (2001). A slacks-based measure of efficiency in data envelopment analysis. European Journal of Operational Research, 130(3), 498–509. https://doi.org/10.1016/S0377-2217(99)00407-5
- Tone, K. (2002). A slacks-based measure of super-efficiency in data envelopment analysis. European Journal of Operational Research, 143(1), 32–41. https://doi.org/10.1016/S0377-2217(01)00324-1
- United Nations. (2020). Database. https://data.un.org/.
- Verbič, M., Boris, M., & Van Nieuwkoop, R. (2006). Sustainability of the Slovenian pension system: An analysis with an overlapping-generations general equilibrium model. Eastern European Economics, 44(4), 60–81.
- World Bank. (1994). Averting the old age crisis: Policies to protect the old and promote growth. Oxford University Press.