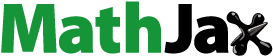
Abstract
In late 2019 and early 2020, a sudden but not unexpected external shock occurred in the tourism industry. This study presents an explorative analysis of the literature review discussing a predicted exogenous shock in tourism caused by the appearance of viruses. Furthermore, the vector autoregressive (V.A.R.) models and panel econometrics is used to analyse and model the impact of the COVID-19 pandemics on tourist arrivals in Slovenia and Croatia. Applied analysis is used to test tourism artefacts in the short term on daily data for 2020. The results of econometric modelling and data plotting confirm significant volatilities in the analysed time-series and their peculiar response to the influence of the COVID-19 pandemic on tourist arrivals. Therefore, innovative tourism policy and management should not expect pre-pandemic values and demand; moreover, tourist expectations will change drastically in a calamitous decade, while several other determinants, not only COVID-19, will influence tourist arrivals. The study is novel in two respects. First, the study adds value to empirical research on the determinants of tourism demand. Moreover, the study can be considered a starting point for further short-, medium- and long-term econometric analyses of sudden shocks affecting tourism demand and government decisions affecting tourism supply.
Introduction
Prior to the twentieth century, there were several speculations about whether the year 2000 would even begin. The combination of numbers two and zero (2, 0) has not been reached until the second decade of the twenty-first century. The year 2020 began as a spellbinding story where people suffered from the viral Coronavirus disease (COVID-19), moreover, it is a decade wrought with a myriad of scourges (Gričar, Baldigara et al., Citation2021). Some authors (Gričar, Citation2019) succeeded to predict the proliferation of viral diseases and demonstrated a decline in tourist arrivals by 20%.
Few scientists use predictable statistical methods; therefore, the majority of research and discussions report well-known and defined problems. Nonetheless, history and recent discussions and research regarding positive COVID-19 cases suggested that the pandemic could last up to four years. Behind that, the economic threat is quite significant and of great impact on several economic aggregates. Rising inflation, which could impede people's life, is scarcer but evident shortly. Behind rising costs of living, including food followed by higher interest rates, manifests new threats (Yang et al., Citation2021). Additionally, overwhelmed stock markets are hazardous (Memon et al., Citation2021; Shaikh, Citation2021; Umar et al., Citation2021). The rising effects of inflation are not supported by the standard of living and a pandemic (Khalid et al., Citation2021).
Overall, contrary to majority wishes, it seems that, according to Sridhar (Citation2020), the COVID-19 pandemic will not be dispelled before 2023. In the meantime, the socio-economic and physical status of residents and non-residents declines, and these adverse afflictions go beyond the health outbreak alone. In November 2019, pioneering research attempted to model and predict tourist arrivals in a pandemic situation. The study was developed by Gričar (Citation2019) and is considered the starting point for the present study, while ex post and ex ante research is of utmost importance. The main objective of the present study is to extend the primary ex ante research that recognised the decline in tourist arrivals before the outbreak of the COVID-19 pandemic. The researcher predicts the pandemic using regression analysis. The regression analysis has been further used by Kostynets et al. (Citation2021). Thus, the objective motivation of this research is to extend the ordinary least squares (O.L.S.) methodology to time series and panel regression steps (Baldigara & Mamula, Citation2015; Karadzic & Pejovic, Citation2020). The use of breaks in time series in vector autoregressive (V.A.R.) model and cointegrated V.A.R. (C.V.A.R.) model are much better diagnostic modelling than other multivariate analyses (Juselius, Citation2009).
The following section presents a historical review of the econometric mirror with two questions related to pandemics and econometrics followed by the literature review. In the central part of the article, the used methodology, data and results of the econometric approach are laid out. Finally, the discussion and conclusion bring a final touch to the research.
Pandemics and econometrics – a historical overview
In economics and econometrics, prediction is based on the proposition that past events predict future events. For example, the main threat in the Middle Ages was the plague, which peaked in the fourteenth and fifteenth centuries. It is worth noting that plagues occurred years earlier, around the tenth century. More recently, there were several earthquakes and volcanic eruptions, e.g., Fagradalsfjall and La Palma volcanoes. Considering that time series econometrics predicts the future from past events could impact the tourism economy as pandemics begin, earthquakes occur, and volcanoes erupt. Firstly, are we approaching the peak of a pandemic and the lowest part of tourist arrivals?
The importance of tourism in national economies, employment, gross domestic product (GDP), small and medium enterprises, different organisations, institutions and stakeholders has been recognised by several scholars (Brida et al., Citation2016; Južnik Rotar et al., Citation2019). Vinogradov et al. (Citation2020) report Airbnb-induced tourism growth. Provenzano and Baggio (Citation2020) propose simple methodological steps in tourism and hospitality industry forecasting.
Secondly, are we facing the new normal as a metaphor? (Ateljevic, Citation2020; Roven, Citation2020). The critical historical events can be a war bell for econometricians to use trending and cycles in econometric modelling without overlooking obstacles, minimum and maximum. History shows us the comparison of past events and future well-being and could be represented econometrically, as proposed by Gričar, Šugar et al. (Citation2021)
The purpose of this research is to present an approach to the analysis of waves in tourist arrivals for Croatia and Slovenia to confirm the misunderstanding of rising events in tourism by multiple stakeholders that do not include reactions to a sudden exogenous event. Researchers have noted an increase in tourist arrivals, albeit domestic or international, for several decades with occasional interruptions. For several years, the World Tourism Organisation (UNWTO, Citation2020a), as the umbrella organisation for tourism development, published its bulletin, which was used countless times by scholars who did not think that every period of maximum has a peak and therefore it is time to turn to a minimum. There were no downward trends in the UNWTO (Citation2020b) bulletin, which is econometrically a misleading story. In addition to the recent paper on the impact of the crisis by Duan et al. (Citation2022), these effects motivated our research on the well-being of volatility in tourist arrivals in time series data. It is of great importance to get the information from the data, the plotted data, while Juselius (Citation2009, Citation2021) stated, a decade ago, that the data should be let speak freely. Based on the previous statements, and objectives two hypotheses were tested. The main thesis is: The tourism industry in Slovenia and Croatia has developed rapidly, and it is predicted its growth even more in a positive direction and year-round (not seasonal). This is an opportunity for positive and more productive growth? Therefore, the Slovenian and Croatian tourism industry offers an exciting research question focusing on a strategic approach using time series:
H1: In the short term, tourist arrivals in Slovenia declines due to the COVID-19 pandemic.
H2: In the short term, tourist arrivals in Croatia declines due to the COVID-19 pandemic.
Literature review
A detailed literature desk-research has evidenced that fewer authors attempted to forecast the rise of the pandemic COVID-19 pandemic ex ante. On the other hand, a pretty significant number of discussions and research are dealing with ex post COVID-19 pandemic analysing and modelling. outlines the research dealing with the COVID-19 pandemic modelling and forecasting; for example, Kaushal and Srivastava (Citation2021) propose a better preparedness for outbreaks in tourism.
Table 1. Coronavirus (COVID-19) literature review desk-research.
Fotiadis et al. (Citation2021) and UNWTO (Citation2020a) are predicting what was already apparent and predicted by Gričar (Citation2019) and Gričar, Baldigara et al. (Citation2021, p. 13), a decline in tourist arrivals. Tourism sharing is an essential generator of economic recognition, according to Sainaghi et al. (Citation2020). Nevertheless, a comprehensive empirical methodology was used, and the prediction is 10 percentage points higher than that of Gričar (Citation2019). Moreover, Sharma et al. (Citation2021) revived the idea of new waves in tourism in post-pandemic tourism. The question, which has not been asked in the research is, when will the recovery start? Škare et al. (Citation2021) predict a more prolonged recovery in tourism than the average recovery from an average crisis is. Additionally, Hu et al. (Citation2021) addressed the evaluations of tourists' experiences during the pandemic collected via the World Wide Web. They predicted a differentiation in tourists' behaviour.
Several countries are studied in the literature based on the COVID-19 pandemic and tourism (Esquivias et al., Citation2021; González-Torres et al., Citation2021; Hu et al., Citation2021; Jeon & Yang, Citation2021; Sobaih et al., Citation2021). For Slovenia and Croatia, on the other hand, there are fewer published articles (Gričar, Citation2019; Perić et al., Citation2021; Williams, Citation2021), so this is one of the first attempts to shed new light on the importance of these two Mediterranean countries among tourism actors. Perić et al. (Citation2021) recognised Slovenia and Croatia as small countries with fewer cases in the first wave of the pandemic. Williams (Citation2021) added a study of entrepreneurial and labour instability during the pandemic.
Additionally, some other researchers recognised other values for waves in tourism. Martini and Buda (Citation2020) offer a deeper layer in death and disaster places. Senbeto and Hon (Citation2020), and Ateljevic (Citation2020) focused on shocks causing peak and through waves, while Çanakçı and Birdir (Citation2020) and Seidell (Citation1995) on food neophobia epidemic. Ferrari et al. (Citation2018) find the change in tourists' income essential for tourism spending and food demand. There were some previous attempts to pandemic effects which were nicely summarised by Duan et al. (Citation2022), leisure vs health nexus discussed by Peel et al. (Citation2021) and outdoor safety perceived by Zanon et al. (Citation2019).
Outline of ex ante research
The time we are living is sufficient and abundant. Nevertheless, it is of primary interest to consider strategic questions and science. Scientific research should be based on data and a quantitative approach in providing guidelines for overcoming external shocks that negatively afflict tourism determinants. Nowadays, the research should be focused on the COVID-19 pandemic situation and tourist arrivals. Many European countries suffer from the pandemic (Dye et al., Citation2020).
Additionally, the COVID-19 pandemic has not yet ended. Based on the normally distributed data, some prognoses show that the virus is still present (The Covid-19 Tracker Slovenia, Citation2021). Researchers recognised, from tourist arrivals and microbial variables in vector correction modelling (Gričar, Baldigara et al., Citation2021), that COVID-19 began in April/May 2019, not December 2019, as indicated by Fong et al. (Citation2021). The wave's peak will be reached after two to two and a half years, starting in April/May 2019, and the trough will be reached in the next two years, ending in spring 2023, for four years (Sridhar, Citation2020).
Secondly, tourism fills moments in our life. Therefore, people living in European Union (E.U.) have a physical need to plan their vacation on the Mediterranean coasts. For E.U. residents, it is customary to find ways to escape from everyday life. This solution is a must that should be offered by policymakers this and next summer. Overall, the first thing that comes to our minds is science based on the COVID-19 epidemic. The second thing that meets their needs is summer vacation. Prognostic models are abundant. In the conclusion of the section, the discussion of a pre-epidemic prognosis by Gričar (Citation2019) on a medium-term and the data of NIJZ (Citation2020) is applied. The research was based on the idea of Provenzano and Baggio (Citation2020) to keep the quantitative analysis credible and straightforward based on 108 monthly time series data, with the twelve most common microbes (viruses and bacterias) included. After including twelve regressors in the O.L.S. model, the reference model showed the association of viral infections on tourist arrivals. The main threats to tourist arrivals were detected viral infections and disease spread. The empirical evidence shows: each such case was associated with a reduction in tourist arrivals of 63.25 persons per month. This result has some important implications for tourism and health policy. For example, looking at the linear regression function, between March 4 and March 28, 2020, related only to the COVID-19 epidemic, this would result in a decrease of 46,615 tourist arrivals () per month on average, out of 314,772 monthly tourist arrivals in Slovenia, or by 17.77%. The result is consistent with the finding of Gössling et al. (Citation2021). They predicted a decrease in international tourist arrivals between 20% and 30%. In addition, the table of Gričar (Citation2019) is translated to be presented in this article as part of the literature review (), where statistically significant
values are in bold numbers.
Table 2. Values (coefficients) of the variables studied, dependent variable number of tourist arrivals, January 2008–December 2016.
The value of the adjusted coefficient of the deterministic coefficient is 0.69, which means that the regression model explains 69% of the variability in tourist arrivals in Slovenia with reported microbial infections.
Secondly, the results suggest that tourist arrivals in Slovenia were not susceptible to the identified bacterial infections. Despite the identified presence of three statistically significant bacterial infections in the country, tourist arrivals increased, influencing the post-pandemic tourist boom. Moreover, the results based on the first differences confirm the results of O.L.S. For the first differences, the adjusted deterministic coefficient is 15%, the statistic of Durbin-Watson is 1.9, and the statistical significance of the regression coefficients is less than 1% for the log variables Campylobacter and viruses, with a constant of 0.01.
However, the short-term shock may be nonlinear, and the decline could be more severe, as was the case in early April 2020 in a broader European and Mediterranean region (Marti & Puertas, Citation2021), as well as in many other parts of the world (Zhang, Gozgor et al., Citation2021). Shocks occur at very short intervals and tend to impact in the short to medium term and then worsen in the long term. Some other models have predicted or showed the impact in a short to medium-term volatility time series for tourism (Batool et al., Citation2021; Gričar, Citation2019).
Therefore, there is a need for greater collaboration and networking between closely linked sectors with the tourism and hospitality industry and urgent organised health measures to reduce, control and manage the spread of infections among tourists (Xue et al., Citation2021). The globalisation of economies and tourism economies have brought rapid economic growth and improvements in the well-being of the population, which should be assured with national, regional and global efforts to control worldwide shocks in global tourism and tourist arrivals, which are faced by many national tourism economies and finances (Gričar, Šugar et al., Citation2021; Liao et al., Citation2021; Li & Xu, Citation2021).
Data and methodology
Every year all the Mediterranean countries suffer from seasonality (Secondi et al., Citation2011). The analysis is based on seasonality as a crucial tourism demand feature of the two Mediterranean countries Slovenia and Croatia. Two hypotheses are considered, involving two variables tourist arrivals and positive COVID-19 cases. The secondary data used for variables were obtained from the two sources: the variable COVID-19 confirmed positive cases, obtained from the European Centre for Disease Prevention and Control (E.C.D.C.) website (European Centre for Disease Prevention and Control (ECDC), Citation2021). Data for the number of tourist arrivals (CBS, Citation2021; SORS, Citation2021) were isolated from the Statistical Offices of Slovenia and Croatia.
Data vector is from March 5 to December 14, 2020, based on daily observations for positive COVID-19 cases (C.O.V.) and average daily values on monthly tourist arrivals data (A.R.R.). Therefore, the data vector is as follows:
(1)
(1)
where 191 is a numeric abbreviation of International Naming Convention for Croatia and 705 is an abbreviation for Slovenia (ISO, Citation2021).
The research relies on several econometric methods. First, in empirical modelling, regression analysis based on O.L.S. is presented in . It contains many lemmas and ideas for distinguishing multiple variables regarding the relationships between the regressed variable and one or more regressors. Nonetheless, this set of statistical procedures points out the importance of how the primary value of a dependent variable changes when one of the independent variables change while the other explanatory variables do not. Regression analysis:
(2)
(2)
is performed for both hypotheses, where
are tourist arrivals as the dependent variable,
is a constant,
is a regression coefficient that shows the direction and dimension of the relationship between the dependent variable and the independent
variable, where
shows the number of regressors,
are model errors based on the distribution of residues over the difference of the time series between
and
The work of Gričar (Citation2019) presents a dependent variable as tourist arrivals, while his independent variables are the 12 most common microbes in Slovenia.
Second, the residuals in a time series have a unit root. It states that the residuals are non-stationary. The null hypothesis is usually determined as having a unit root, and the alternative hypothesis is stationarity, trend stationarity or has an explosive root depending on the test used. The unit root test produces a more accurate approach to the tourism industry on the data obtained. An analysis is performed for hypotheses H1 and H2.
Third, in the diagnostic checking, the autocorrelation and the normality should be jointly considered. Note, however, that it depends on the space (geographically) in which the autocorrelation assumption operates, the framework vicariously can be used, and perhaps an O.L.S.-like transformation, e.g., time series, can be performed. Time series are versions of the model obtained from several sources and the V.A.R. model:
(3)
(3)
is implemented. The
are i.i.d. residuals,
are the regressed coefficients,
are the regressors,
are the integrated parameters, and
is the defined matrix with the coefficients and the constant
The additional test for the panel regression to test the hypotheses,
(4)
(4)
is achieved (Južnik Rotar et al., Citation2019). The balanced panel has, for each cross-sectional unit
the same number of time series observations (capturing everything that remains unexplained about
) or a detour. The number of observations is 286. The remaining disturbance is
which is as high as shocks are delivered.
Finally, in descriptive statistics, a summary of statistical parameters is added to summarise observations and convey most of the information as simply as possible. Summary statistics provide a basic overview of the sample and the conclusions reached. Such summaries can be quantitative, i.e., descriptive statistics, or pictorial, i.e., easy-to-understand drawings in graphs, which is exclusively used in this study.
Results
Descriptive statistics
According to E.C.D.C. data (ECDC, Citation2021), the first COVID-19 case was isolated in Croatia on February 26, 2020, and Slovenia on March 5, 2020. The missing values in the series for Croatia were on days 5, 9 and 10 March 2020 and for Slovenia on March 9, 2020. Therefore, the analysis of COVID-19 cases related to tourist arrivals from March 5, 2020, to the last available source in ECDC (Citation2021), i.e., December 14, 2020, is treated daily. Missing values and zero values are replaced by 1 to obtain a non-zero denominator and avoid miss indexation. The daily values for tourist arrivals are the average monthly values. Since the series are still short, the use of daily values is necessary.
Overall, the part of the descriptive statistics is calculated on the data vector from March 5 to December 14, 2020. Data for Slovenia and Croatia are isolated and presented in the following subsections. It is worth noting that both variables are converted to: (1) the chain base index and (2) to the fixed index
where March 5, 2020 = 100.
Croatia
Descriptive statistics for Croatia confirm the peak month for tourist arrivals, i.e., August 2020. On the other hand, the peak period of positive COVID-19 cases has been isolated for the end of 2020. Nevertheless, the rising effect in the pandemic for Croatia is present without seeing a low point in the epidemic wave. Look at a graph showing a wave because it seems like a diagram of an ocean wave, with the highest point (peak) at the top and the lowest point (trough) before the next wave. Econometrically, this is explained as an i.i.d. distribution of data.
With the onset of winter and colder temperatures in the two countries studied, we have seen a surge in infections, an epidemic tsunami. On the other hand, summer offers an imperative of tourist demand.
Slovenia
The descriptive statistic for Slovenia is plotted in , from which it is apparent that a higher incidence of COVID-19 cases in Slovenia leads to a lower number of tourist arrivals. According to the normal distribution theory, we can expect a decrease in COVID-19 cases for the next two years (e.g., 2022/2023) and the level of tourist arrivals to the level before the pandemic in the next two to three years (e.g., 2022/2024).
Plotting the summary statistics brings an overview of the data transportation and distribution. The analysis of and confirms high volatility in tourism demand. First, for Croatia, it is evident from that the volatility is high and concentrated in the high season; therefore, the main hypothesis is confirmed in a partial agreement that the tourism industry is high and immense. On the other hand, represents that Slovenia had slower growth in tourism demand.
Figure 1. Comparison of COVID-19 cases and tourist arrivals in Croatia for 2020, daily data, fixed index March 5, 2020 = 100.
Source: Author's calculations and rectifications from ECDC (Citation2021) and CBS (Citation2021).
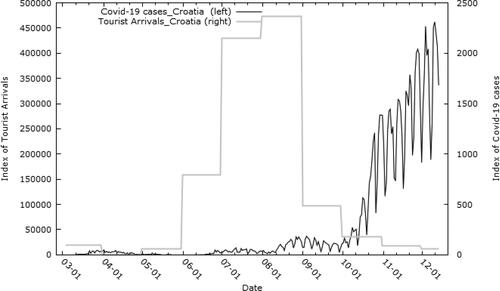
Figure 2. Comparison of COVID-19 cases and tourist arrivals in Slovenia for 2020, daily data, fixed index March 5, 2020 = 100.
Source: Author's calculations and rectifications from ECDC (Citation2021) and SORS (Citation2021).
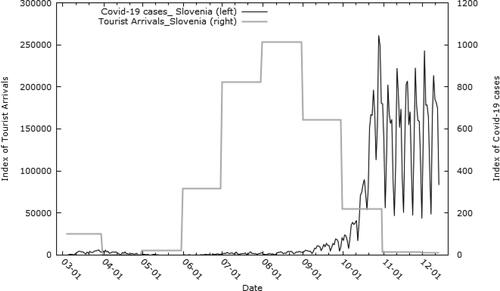
Overall, it can be concluded that both analysed countries are dealing with a more significant number of tourists even in case of a pandemic, be it domestic or international tourist arrivals. In conclusion, Croatia has higher volatility in COVID-19 cases than Slovenia, and consequently, this is reflected in tourist arrivals, which is apparent when overlaying the left parts of and . Therefore, the main hypothesis of using time series in the scientific approach is confirmed.
Regression analysis and V.A.R
It is of central econometric and modelling importance to get an overview of the distribution of the variables. Therefore, the second consideration for the empirical treatment of the study is the regression analysis based on the O.L.S. technique. The result of the regression analysis based on the theoretical consideration of the O.L.S. EquationEquation (2)(2)
(2) for Croatia:
(4)
(4)
claims to be a highly statistically significant result with an
-test value of 26.95. The t statistic value is in a parenthesis, and the p-value is in a brace. On the other hand, the Durbin-Watson statistic (D-W) is 0.04, indicating a high positive autocorrelation between the variables and, as such, it is not very remarkable to be tested as a regression coefficient it is known in time series methodology. The deterministic coefficient is 0.09, indicating that tourist arrivals can only be interpreted in 9% of the variance of COVID-19 positive cases. The adjusted deterministic coefficient is 0.08, eliminating the influence of some explanatory variables.
The result of the regression analysis for Slovenia is:
(5)
(5)
with t statistic value in parenthesis and p-value in a brace. The
-test reliability at 39.94 of the regression equation shows its high statistical significance. The deterministic coefficient and the adjusted one at 0.12 show that the included explanatory variable explains 12% of the dependent variable. Despite a relatively small proportion, there is a sign of some influences; therefore, further investigation is needed.
The not so surprising results for both analysed countries tend to confirm the influence of the COVID-19 pandemic on tourist arrivals with a high significance level of statistics, a low regression coefficient accompanied with a low D-W statistic. On the other hand, the validity of the time series, loosely speaking, is again confronted with the subjective thinking of managers and politicians, which is why the importance of the following three steps in the analysis procedure enters into the econometric decision.
First, related to the results of the regression analyses, the misspecification tests are calculated. From , it is evident that the usual procedure of treating the time series for autocorrelation and normality during the COVID-19 pandemic is econometrically incorrect. The results show that autocorrelation is not present based on Augmented Dickey-Fuller tests (A.D.F.), but there are still non-normalities in the data. This nonnormality is the reason for the further modelling procedure. The second model is V.A.R., and the third is panel regression. Overall, the results of the misspecification test confirm that the volatility in tourist arrivals and positive COVID-19 cases has a giant swing in both directions, followed by a high standard deviation.
Table 3. Misspecification test, daily data, fixed index, March 5, 2020 = 100.
The data distributed in levels are transformed in logarithm, and thus the data vector is obtained:
(6)
(6)
where Δ denotes transformation of the data vector, lower case letters logarithm variables and
denotes the treatment of variables integrated in the first order of integration.
The V.A.R. ‘cays’ that positive COVID-19 cases have no significant impact on tourist arrivals, i.e., the pandemic itself correlates with the procurement of other influences, e.g., political restrictions. Therefore, it can be concluded that the only salvation for tourism is policy, not the pandemic, while it has no direct influence, as shown by the two lagged dimension V.A.R.(2) for Croatia:
(7)
(7)
as its procurement from EquationEquation 3
(3)
(3) , and
statistic values in parenthesis. Lastly, the dimensions are tested in V.A.R.(2) framework for Slovenia:
(8)
(8)
where the results confront the panel regression as another method of investigation.
Panel regression
In a short period, the treatment in the usual time series approach as given above is not highly accurate, while the degrees of freedom could be zero. Since the volatility in the time series is enormous, an additional investigation is necessary, so a panel regression is performed. There are two individual units (Slovenia and Croatia) and two time series tourist arrivals, and positive COVID-19 cases in 2020 spanned in a cross-section. Based on EquationEquation 4(4)
(4) , with the assumption that disturbance term
is constant over time (Brooks, Citation2015, p. 529), the results in first differences for Croatia are:
(9)
(9)
with a D-W statistics 2.02. Additionally, the results for Slovenia are:
(10)
(10)
where
values are in parenthesis and D-W statistics for Slovenia is 2.01. For each country studied, the dependent variable is tourist arrivals. The independent variables are positive COVID-19 cases for individual
at time
Tourist arrivals as a spatial cross-section is a second independent variable.
The panel regression confronts two neighbouring countries in a spatial dimension of tourist arrivals even through the pandemic. The influence on Slovenian tourism has Croatian tourism with a statistically significant coefficient of 2.77 (EquationEquation 9(9)
(9) ). On the other hand, Slovenian tourism has a more minor but significant influence on Croatian tourism with a panel regression coefficient of 0.30 (EquationEquation 9
(9)
(9) ).
Moreover, the spatial dimension shows that the results on Slovenian tourism do not have a compelling impact on the domestic or imported COVID-19 pandemic. On Croatian tourist arrivals, a COVID-19 pandemic also has no significant direct impact. Government and policy decisions could explain the results; econometrically, the data speak: COVID-19 alone has no direct impact on tourist arrivals in the two countries studied. Moreover, it could be concluded that similar results could be obtained by examining adjacent spatial cases during the 2020/2023 pandemic for the E.U.-27, such as Italy versus France, and the European Economic Area (E.E.A.), for example, Sweden versus Norway.
Discussion
The results of this initial trial analysis on daily data are significant. It is important to note that any restriction due to, for example, hospital problems leads to a decrease in tourist arrivals and, more importantly, a change in tourist numbers and habits. If we measure the pure economic impact, the COVID-19 epidemic is probably the most profound breakdown reflecting G.D.P., while tourism contributes about 20% to the entire national economy in Croatia and 5% in Slovenia (Gričar, Šugar et al., Citation2021).
The results of this study suggest that econometric analysis on daily data does not provide satisfactory results, and therefore Gričar's (Citation2019) ex ante research on monthly data provides comparative results. This study is the resonance that COVID-19 research on time series is limited at the moment and should be continued with some other modelling structures. One of the ways is the panel regression done in this study.
The modelled results of this study do not confirm an apparent relationship between COVID-19 infection and the number of tourist arrivals. The conclusion is that COVID-19 does not have as egregious an impact on tourist arrivals as is usually portrayed in newspapers and some scientific articles (). The scientific implications of this study suggest the need for a comprehensive study focused on discovering other determinants affecting tourist arrivals during the pandemic from late 2019 to late 2021. On the other hand, some other methods, such as C.V.A.R. and cointegrated panels, should be used to isolate barriers in the regression. Therefore, C.V.A.R. and random panels are better choices. C.V.A.R. has many ways to nest a multivariate path-dependent process of data emergence and a corresponding dynamic macroeconomic theory. The -th order V.A.R. is:
(11)
(11)
where
is
and
are predetermined. Although, when the reviews of
are actively time-dependent, the conditional process
is independent and O.L.S. estimates of
are Maximum Likelihood estimates. The example V.A.R. is thus an econometric metamorphosis of (time-invariant) data covariances. Therefore, it can be used as a first general approximation to the real process of data formation (Hendry & Mizon, Citation1999).
Conclusions
Shocks in tourist arrivals due to viral infections and emerging diseases are validated events. They are confirmed as one of the significant uncertainties and threats to the tourism industry. This study analyses the relationship between the current pandemic COVID-19 situation and tourist arrivals based on a daily data vector. E.C.D.C. reports the positive cases or diagnoses.
In summary, first, the COVID-19 pandemic has no significant direct impact on tourist arrivals in the short term, but the impact is indirectly significant. There are also other determinants not included in this study, influencing lower tourist arrivals in 2020. The results are based on daily data from March 5, 2020, to December 14, 2020. Second, the ex ante investigation on monthly data for the long-term period provides the information that viruses negatively affect tourist arrivals and bacterial infections positively. The latter could be the next reverberating outbreak in tourist arrivals.
The suggestion to policymakers is to be more concerned with the dissemination of diagnosis, also during the pandemic and in the EU, diagnoses are subject to privacy policy restrictions. On this occasion, sharing this information with the public, tourism management, and the police is a misleading solution. As reported in this study, tourism has suffered and will continue to suffer due to various diseases and policy decisions. The recent epidemic is changing the solution to the welfare of residents, tourists and others across generations. While bacterial infections can be managed in countries as a whole without significant negative impacts on tourist arrivals, this is less the case for viral infections and pandemics with broader governmental policy decisions.
In conclusion, the results propose to study several determinants that influence tourism arrivals while the direct impact on the decline in tourist arrivals due to the COVID-19 epidemic was not confirmed, and therefore, the hypotheses are not verified. The spatial analysis based on the panel regression reveals some differences in Croatian and Slovenian tourism market impacts. Whether public or private, the tourism industry should strongly collaborate and network with the health system and policy/decision-makers. This information can be supported by improving staff awareness and training, accurate information, and first-aid posts for tourists. The historical data presented in this research could improve the thinking of decision-makers and tourists.
The opportunity for future research are delimitations and limitations of the study. The delimitations are that we focus on two Mediterranean countries and a defined data vector. Nevertheless, the data vector is as long as possible for the research time in March 2021, while CBS (Citation2021) does not report tourist arrivals for the period after December 2020. Limitations of the study include testing the hypotheses for waves in a regression function. Therefore, topics for future research could include incorporating integrated time series analysis into a cross-country comparison or random panel data analysis. One possibility could be the application of predicting tourist arrivals with genetic programming models using different explanatory variables.
Overall conclusion
The main hypothesis is that the tourism industry in Slovenia and Croatia has developed rapidly and is expected to grow even more in a positive direction and year-round (not seasonal). This is an opportunity for positive and more productive growth? Therefore, the Slovenian and Croatian tourism industry offers an exciting research question focusing on a strategic approach using time series: H1: In the short run, tourist arrivals in Slovenia decline due to the COVID-19 pandemic. H2: In the short run, tourist arrivals in Croatia decline due to the COVID-19 pandemic.
However, several factors need to be integrated with this overall conclusion:
the models (O.L.S., V.A.R. and Panel) do not prove the direct impact of COVID-19 on tourist arrivals;
the government and policymakers influence the impact on tourist arrivals;
impact studies on other country pairs would yield comparable results of the first (methodology) and second determinant levels (policymakers);
structural breaks were expected; therefore, several methodological steps were introduced from the beginning.
Nevertheless, the time series methodology step is crucial in multivariate analysis.
Disclosure statement
No potential conflict of interest was reported by the authors.
References
- Alsayed, A., Sadir, H., Kamil, R., & Sari, H. (2020). Prediction of epidemic peak and infected cases for COVID-19 disease in Malaysia. International Journal of Environmental Research and Public Health, 17(11), 4076. https://doi.org/10.3390/ijerph17114076
- Ateljevic, I. (2020). Transforming the (tourism) world for good and (re)generating the potential ‘new normal’. Tourism Geographies, 22(3), 467–475. https://doi.org/10.1080/14616688.2020.1759134
- Baldigara, T., & Mamula, M. (2015). Modelling international tourism demand using seasonal ARIMA models. Tourism and Hospitality Management, 21(1), 19–31. https://doi.org/10.20867/thm.21.1.2
- Batool, M., Ghulam, H., Azmat Hayat, M., Zahid Naeem, M., Ejaz, A., Imran, Z. A., Spulbar, C., Birau, R., & Horațiu Gorun, T. (2021). How COVID-19 has shaken the sharing economy? An analysis using Google trends data. Economic Research-Ekonomska Istraživanja, 34(1), 2374–2386. https://doi.org/10.1080/1331677X.2020.1863830
- Brida, J. G., Cortes-Jimenez, I., & Pulina, M. (2016). Has the tourism-led growth hypothesis been validated? A literature review. Current Issues in Tourism, 19(5), 394–430. https://doi.org/10.1080/13683500.2013.868414
- Brooks, C. (2015). Introductory econometrics for finance. Cambridge University Press.
- Çanakçı, S. D., & Birdir, K. (2020). The relation among food involvement, food variety seeking and food neophobia: A study on foreign tourists visiting Turkey. Current Issues in Tourism, 23(8), 917–928. https://doi.org/10.1080/13683500.2019.1602114
- CBS. (2021). CBS databases. Retrieved March 25, 2021 from https://www.dzs.hr/PXWeb/Menu.aspx?px_language=hr&px_type=PX&px_db=Turizam
- Duan, J., Xie, C., & Morrison, A. M. (2022). Tourism crises and impacts on destinations: A systematic review of the tourism and hospitality literature. Journal of Hospitality & Tourism Research, 46(4), 667–695. https://doi.org/10.1177/1096348021994194
- Dye, C., Cheng, R. C.-H., Dagpunar, J. S., & Williams, B. G. (2020). The scale and dynamics of COVID-19 epidemics across Europe. Royal Society Open Science, 7(11), 201726. https://doi.org/10.1098/rsos.201726
- Esquivias, M. A., Sugiharti, L., Rohmawati, H., & Sethi, N. (2021). Impacts and implications of a pandemic on tourism demand in Indonesia. Economics & Sociology, 14(4), 133–150. https://doi.org/10.14254/2071-789X.2021/14-4/8
- European Centre for Disease Prevention and Control (ECDC). (2021). COVID-19 Coronavirus data – daily (up to 14 December 2020). Retrieved March 25, 2021 from https://data.europa.eu/euodp/en/data/dataset/covid-19-coronavirus-data.
- Ferrari, G., Mondéjar Jiménez, J., & Secondi, L. (2018). Tourists’ expenditure in Tuscany and its impact on the regional economic system. Journal of Cleaner Production, 171(10), 1437–1446. https://doi.org/10.1016/j.jclepro.2017.10.121
- Fong, S. J., Dey, N., & Chaki, J. (2021). An introduction to COVID-19. In Artificial intelligence for coronavirus outbreak. Springer briefs in applied sciences and technology (pp. 1–22). Springer. https://doi.org/10.1007/978-981-15-936-5_1
- Fotiadis, A., Polyzos, S., & Huan, T-C. T C. (2021). The good, the bad and the ugly on COVID-19 tourism recovery. Annals of Tourism Research, 87, 103117. https://doi.org/10.1016/j.annals.2020.103117
- Gallego, V., Nishiura, H., Sah, R., & Rodriguez-Morales, A. J. (2020). The COVID-19 outbreak and implications for the Tokyo 2020 Summer Olympic Games. Travel Medicine and Infectious Disease, 34, 101604. https://doi.org/10.1016/j.tmaid.2020.101604
- González-Torres, T., Rodríguez-Sánchez, J.-L., & Pelechano-Barahona, E. (2021). Managing relationships in the tourism supply chain to overcome epidemic outbreaks: The case of COVID-19 and the hospitality industry in Spain. International Journal of Hospitality Management, 92, 102733. https://doi.org/10.1016/j.ijhm.2020.102733
- Gričar, S. (2019). Tourist as a patient. In N. Kregar-Velikonja & L. Leskovic (Ed.), Holistic Approach to the Patient: International Scientific Conference (pp. 137–145). University of Novo mesto, Faculty of Health Sciences. Retrieved March 24, 2021 from https://fzv.uni-nm.si/uploads/_custom/03_unmfzv/konferenca/zbornik/zbornik_prispevkov_unmfzv_konferenca_2019_www.pdf
- Gričar, S., Baldigara, T., & Šugar, V. (2021). Sustainable determinants that affect tourist arrival forecasting. Sustainability, 13(17), 9659. https://doi.org/10.3390/su13179659
- Gričar, S., Šugar, V., & Bojnec, Š. (2021). The missing link between wages and labour productivity in tourism: Evidence from Croatia and Slovenia. Economic Research-Ekonomska Istraživanja, 34(1), 732–753. https://doi.org/10.1080/1331677X.2020.1804427
- Gössling, S., Scott, D., & Hall, C. M. (2021). Pandemics, tourism and global change: A rapid assessment of COVID-19. Journal of Sustainable Tourism, 29(1), 1–20. https://doi.org/10.1080/09669582.2020.1758708
- Hendry, D. F., & Mizon, G. E. (1999). Exogeneity, causality, and co-breaking in economic policy analysis of a small econometric model of money in the UK. In H. Lütkepohl & J. Wolters (Eds.), Money demand in Europe. Studies in empirical economics (pp. 1–28). Physica-Verlag Heidelberg. https://doi.org/10.1007/978-3-662-12539-7_1
- Hu, F., Teichert, T., Deng, S., Liu, Y., & Zhou, G. (2021). Dealing with pandemics: An investigation of the effects of COVID-19 on customers' evaluations of hospitality services. Tourism Management, 85(August), 104320. https://doi.org/10.1016/j.tourman.2021.104320
- Hugo, N. (2021). The strength of the industry during the coronavirus pandemic. Journal of Hospitality & Tourism Research, 45(5), 931–933. https://doi.org/10.1177/10963480211000825
- ISO. (2021). The International Standard for country codes and codes for their subdivisions. Retrieved March 30, 2021 from https://www.iso.org/obp/ui/#search
- Jeon, C.-Y., & Yang, H.-W. (2021). The structural changes of a local tourism network: Comparison of before and after COVID-19. Current Issues in Tourism, 24(23), 3324–3338. https://doi.org/10.1080/13683500.2021.1874890
- Juselius, K. (2021). Searching for a theory that fits the data: A personal research odyssey. Econometrics, 9(1), 5. https://doi.org/10.3390/econometrics9010005
- Juselius, K. (2009). The cointegrated VAR model. Oxford University Press.
- Južnik Rotar, L., Kontošić Pamić, R., & Bojnec, Š. (2019). Contributions of small and medium enterprises to employment in the European Union countries. Economic Research-Ekonomska Istraživanja, 32(1), 3302–3314. https://doi.org/10.1080/1331677X.2019.1658532
- Karadzic, V., & Pejovic, B. (2020). Tourism demand forecasting using ARIMA model. Transformations in Business & Economics, 19(2B), 731–745.
- Kaushal, V., & Srivastava, S. (2021). Hospitality and tourism industry amid COVID-19 pandemic: Perspectives on challenges and learnings from India. International Journal of Hospitality Management, 92, 102707. https://doi.org/10.1016/j.ijhm.2020.102707
- Khalid, U., Okafor, L. E., & Burzynska, K. (2021). Does the size of the tourism sector influence the economic policy response to the COVID-19 pandemic? Current Issues in Tourism, 24(19), 2801–2820. https://doi.org/10.1080/13683500.2021.1874311
- Kostynets, I., Kostynets, V., & Baranov, V. (2020). Pent-up demand effect at the tourist market. Economics & Sociology, 13(2), 279–288. https://doi.org/10.14254/2071-789X.2020/13-2/18
- Kostynets, V., Kostynets, I., & Olshanska, O. (2021). Pent-up demand’s realization in the hospitality sector in the context of COVID-19. Journal of International Studies, 14(1), 89–102. https://doi.org/10.14254/2071-8330.2021/14-1/6
- Li, B., & Xu, Z. (2021). A comprehensive bibliometric analysis of financial innovation. Economic Research-Ekonomska Istraživanja, 1–24. https://doi.org/10.1080/1331677X.2021.1893203
- Liao, J., Luo, L., Xu, X., & Wang, A. (2021). Perceived macroeconomic uncertainty and export: Evidence from cross-country data. Economic Research-Ekonomska Istraživanja, 1–19. https://doi.org/10.1080/1331677X.2021.1890176
- Marti, L., & Puertas, R. (2021). European countries’ vulnerability to COVID-19: Multicriteria decision-making techniques. Economic Research-Ekonomska Istraživanja, 1–12. https://doi.org/10.1080/1331677X.2021.1874462
- Martini, A., & Buda, D. M. (2020). Dark tourism and affect: Framing places of death and disaster. Current Issues in Tourism, 23(6), 679–692. https://doi.org/10.1080/13683500.2018.1518972
- Memon, P. A., Md-Rus, R., & Ghazali, Z. B. (2021). Adjustment speed towards target capital structure and its determinants. Economic Research-Ekonomska Istraživanja, 34(1), 1966–1984. https://doi.org/10.1080/1331677X.2020.1860792
- NIJZ. (2020). Data. National Institute of Public Health. https://podatki.nijz.si/Selection.aspx?px_path=NIJZ%20podatkovni%20portal__1%20Zdravstveno%20stanje%20prebivalstva__06%20Nalezljive%20bolezni&px_tableid=NB2.px&px_language=sl&px_db=NIJZ%20podatkovni%20portal&rxid=ec3b8862-5c90-43ab-bbc1-4145269638bc
- Peel, N., Maxwell, H., & McGrath, R. (2021). Leisure and health: Conjoined and contested concepts. Annals of Leisure Research, 24(3), 295–309. https://doi.org/10.1080/11745398.2019.1682017
- Perić, M., Wise, N., Heydari, R., Keshtidar, M., & Mekinc, J. (2021). Getting back to the event: Covid-19, attendance and perceived importance of protective measures. Kinesiology, 53(1), 12–19. https://doi.org/10.26582/k.53.1.2
- Provenzano, D., & Baggio, R. (2020). Quantitative methods in tourism and hospitality: A perspective article. Tourism Review, 75(1), 24–28. https://doi.org/10.1108/TR-07-2019-0281
- Roven, I. (2020). The transformational festival as a subversive toolbox for a transformed tourism: Lessons from burning man for a COVID-19 world. Tourism Geographies, 22(3), 695–702. https://doi.org/10.1080/14616688.2020.1759132
- Sainaghi, R., Köseoglu, M. A., d’Angella, F., & Mehraliyev, F. (2020). Sharing economy: A co-citation analysis. Current Issues in Tourism, 23(8), 929–937. https://doi.org/10.1080/13683500.2019.1588233
- Secondi, L., Meseguer-Santamaría, M. L., Mondéjar-Jiménez, J., & Vargas-Vargas, M. (2011). Influence of tourist sector structure on motivations of heritage tourists. The Service Industries Journal, 31(10), 1659–1668. https://doi.org/10.1080/02642069.2010.485641
- Seidell, J. C. (1995). Obesity in Europe: Scaling an epidemic. International Journal of Obesity and Related Metabolic Disorders, 19(3), 1–4.
- Senbeto, D. D., & Hon, A. H. (2020). The impacts of social and economic crises on tourist behaviour and expenditure: An evolutionary approach. Current Issues in Tourism, 23(6), 740–755. https://doi.org/10.1080/13683500.2018.1546674
- Shaikh, I. (2021). Impact of COVID-19 pandemic disease outbreak on the global equity markets. Economic Research-Ekonomska Istraživanja, 34(1), 2317–2336. https://doi.org/10.1080/1331677X.2020.1863245
- Sobaih, A. E. E., Elshaer, I., Hasanein, A. M., & Abdelaziz, A. S. (2021). Responses to COVID-19: The role of performance in the relationship between small hospitality enterprises' resilience and sustainable tourism development. International Journal of Hospitality Management, 94(April), 102824. https://doi.org/10.1016/j.ijhm.2020.102824
- Sharma, G. D., Thomas, A., & Paul, J. (2021). Reviving tourism industry post-COVID-19: A resilience-based framework. Tourism Management Perspectives, 37(January), 100786. https://doi.org/10.1016/j.tmp.2020.100786
- Sridhar, D. (2020). COVID-19: What health experts could and could not predict. Nature Medicine, 26(12), 1812. https://doi.org/10.1038/s41591-020-01170-z
- SORS. (2021). SISTAT. Retrieved March 25, 2021 from the Statistical Office of the Republic of Slovenia: https://pxweb.stat.si/SiStat/en/Podrocja/Index/155/turizem
- Škare, M., Soriano, D. R., & Porada-Rochoń, M. (2021). Impact of COVID-19 on the travel and tourism industry. Technological Forecasting and Social Change, 163, 120469. https://doi.org/10.1016/j.techfore.2020.120469
- The Covid-19 Tracker Slovenia. (2021). Tracking data on the COVID-19 spread in Slovenia. Retrieved 24 March 2021 from Covid-19 sledilnik: https://covid-19.sledilnik.org/en/tables
- Umar, M., Xu, Y., & Sikandar Mirza, S. (2021). The impact of Covid-19 on gig economy. Economic Research-Ekonomska Istraživanja, 34(1), 2284–2296. https://doi.org/10.1080/1331677X.2020.1862688
- UNWTO. (2020a). International tourism to plunge up to 30% due to virus: UNWTO. United Nation World Tourist Organisation. https://economictimes.indiatimes.com/news/international/business/international-tourism-to-plunge-up-to-30-due-to-virus-unwto/articleshow/74849024.cms?from=mdr
- UNWTO. (2020b). World Tourism Barometer, 18(1). United Nation World Tourist Organisation. https://webunwto.s3.eu-west-1.amazonaws.com/s3fs-public/2020-01/UNWTO_Barom20_01_January_excerpt_0.pdf
- Vinogradov, E., Leick, B., & Kivedal, B. K. (2020). An agent-based modelling approach to housing market regulations and Airbnb-induced tourism. Tourism Management, 77(4), 104004. https://doi.org/10.1016/j.tourman.2019.104004
- Wang, Y., Fang, Z., & Gao, W. (2021). COVID‐19's impact on China's economy: A prediction model based on railway transportation statistics. Disasters, 45(S1), S76–S96. https://doi.org/10.1111/disa.12476
- Williams, C. C. (2021). Impacts of the coronavirus pandemic on Europe's tourism industry: Addressing tourism enterprises and workers in the undeclared economy. International Journal of Tourism Research, 23(1), 79–88. https://doi.org/10.1002/jtr.2395
- Wong, K. K. F., Song, H., Witt, S. F., & Wu, D. C. (2007). Tourism forecasting: To combine or not to combine? Tourism Management, 28(4), 1068–1078. https://doi.org/10.1016/j.tourman.2006.08.003
- Xue, W., Xu, Z., Mi, X., & Ren, Z. (2021). Dynamic reference point method with probabilistic linguistic information based on the regret theory for public health emergency decision-making. Economic Research-Ekonomska Istraživanja, 1–35. https://doi.org/10.1080/1331677X.2021.1875254
- Yang, E., Park, H., Choi, Y., Kim, J., Munkhdalai, L., Musa, I., & Ryu, K. (2018). A simulation-based study on the comparison of statistical and time series forecasting methods for early detection of infectious disease outbreaks. International Journal of Environmental Research and Public Health, 15(5), 966. https://doi.org/10.3390/ijerph15050966
- Yang, L., Yi, Y., & Wang, S. (2021). Banks’ maturity mismatch, financial stability, and macroeconomic dynamics. Economic Research-Ekonomska Istraživanja, 1–21. https://doi.org/10.1080/1331677X.2020.1867212
- Yue, X.-G., Shao, X.-F., Li, R. Y. M., Crabbe, M. J. C., Mi, L., Hu, S., Baker, J., & Liang, G. (2020a). Risk management analysis for novel coronavirus in Wuhan, China. Journal of Risk and Financial Management, 13(2), 22. https://doi.org/10.3390/jrfm13020022
- Yue, X.-G., Shao, X.-F., Li, R. Y. M., Crabbe, M. J. C., Mi, L., Hu, S., Baker, J., Liu, L., & Dong, K. (2020b). Risk prediction and assessment: Duration, infections, and death toll of the COVID-19 and its impact on China’s economy. Journal of Risk and Financial Management, 13(4), 66. https://doi.org/10.3390/jrfm13040066
- Zanon, D., Curtis, J., Lockstone-Binney, L., & Hall, J. (2019). Examining future park recreation activities and barriers relative to societal trends. Annals of Leisure Research, 22(4), 506–531. https://doi.org/10.1080/11745398.2018.1500923
- Zhang, H., Song, H., Wen, L., & Liu, C. (2021). Forecasting tourism recovery amid COVID-19. Annals of Tourism Research, 87(March), 103149. https://doi.org/10.1016/j.annals.2021.103149
- Zhang, X., Gozgor, G., Lu, Z., & Zhang, J. (2021). Employment hysteresis in the United States during the COVID-19 pandemic. Economic Research-Ekonomska Istraživanja, 34(1), 3343–3354. https://doi.org/10.1080/1331677X.2021.1875253