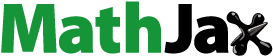
Abstract
We examine the impacts of a public education subsidy on the wage differential in the presence of automation by establishing general equilibrium models with two-stage skill formation. We show that for an economy with full employment, a public education subsidy will increase the wage differential in the first stage of skill formation, but in the second stage, the wage differential may be reduced if the ratio of unskilled labor to skilled labor is large enough. For an economy with unemployment, a public education subsidy will narrow down the wage differential in the first stage, but in the second stage, the wage differential may be widened if that ratio is large enough.
1. Introduction
The race between education and technology has shaped the distribution of earnings for at least the last 100 years (Acemoglu & Autor, Citation2012; Goldin & Katz, Citation2008). Through much of the 20th century, rapidly rising education levels were an important equalizer: Although technological change usually raised the demand for skills, the average years of schooling also increased continuously, countervailing against the skill premium (Goldin & Katz, Citation2008). However, since the early 1980s, the education levels have grown much more slowly and failed to keep pace with technological progress. Autor (Citation2014) shows that the rate of intercohort increase was around 1%, while after 1982, the rate fell by almost half from 0.87% to 0.47%.Footnote1 The deceleration in the skilled labor supply notwithstanding, the past three decades of computerization have greatly raised the demand for college-educated workers, resulting in rising wage inequality (Autor et al., Citation2006).Footnote2
More recently, the remarkable advances in automation technology (e.g., 3 D printers, artificial intelligence, robotics) have raised concerns that the lack of educational attainment for many workers will be a major challenge in the labor market (Autor, Citation2015; Levy & Murnane, Citation2013). Although automation cannot replace all the tasks, it tends to take over repetitive and routine tasks performed by labor on a scale not previously observed, and gives rise to the employment polarization in the sense that unskilled labor is reallocated into service occupations and undertakes manual tasks (Autor et al., Citation2006, Citation2008). Although the wage in low-skill service occupations can outperform other low-skill occupations, it has still grown sluggishly compared to the skilled wage in recent decades (Autor & Dorn, Citation2013, p. 1556). In the presence of automation, unskilled labor would be less likely to find jobs paying decent wages if they cannot update their skill levels in time (e.g., Brynjolfsson & McAfee, Citation2014; Lankisch et al., Citation2019; Levy & Murnane, Citation2013).
This paper aims to explore the impacts of a public education subsidy on skilled-unskilled wage inequality in an economy with automation. We examine such relationships in the canonical specific-factor framework that is widely adopted in the literature on wage inequality (e.g., Anwar, Citation2006, Citation2008; Beladi et al., Citation2010; Chaudhuri et al., Citation2018; Marjit & Kar, Citation2005; Pi & Fan, Citation2019; Pi & Zhou, Citation2012; Pi & Zhang, Citation2018, Citation2020; Tawada & Sun, Citation2010). Similar to Prettner (Citation2019) and Zhang (Citation2019a), we model robots as automation capital, which is a perfect substitute for unskilled labor in the robot-using sector. Skill formation consists of two stages: In the first stage, unskilled workers can decide whether to acquire skills; in the second stage, workers that finished an education in the first stage will acquire skills and will not be replaced by robots, and they can now find jobs in high-skill occupations. We find that a public education subsidy can decrease the wage differential conditionally. In the presence of automation, when we want to ensure full employment, a public education subsidy can only decrease the wage differential in the second stage. When we allow unemployment, wage inequality may be reduced in both stages.
On the one hand, there exist many studies paying attention to the challenge of automation. For example, Acemoglu and Restrepo (Citation2018b) hold that the jobs creation can go hand-in-hand with automation if the long-run return to capital is not too low. Prettner (Citation2019) introduces automation into a standard growth model, and finds that it can explain the decline of the U.S. labor share. Lankisch et al. (Citation2019) establish an R&D driven model of automation to explain the coexistence of increasing per capita output, declining unskilled wage rate, and rising skilled wage rate. Zhang (Citation2019a) argues that automation does not necessarily deteriorate wage inequality. Okada (Citation2020) finds that an equilibrium without automation can be achieved if the government invests enough resources in education. Bentaouet Kattan et al. (Citation2021) construct an overlapping-generations model with automation to investigate how education impacts inequality. Prettner and Strulik (Citation2020) hold that using standard policy suggestions to battle against the challenge of automation may engender unintended side effects. For example, they find that an education subsidy can slow down growth and redistribute income to the rich, thereby raising inequality. However, this paper is different from Prettner and Strulik (Citation2020) and Bentaouet Kattan et al. (Citation2021) who investigate how education can impact inequality in the presence of automation in three aspects. First, we adopt a different analytical framework, and concentrate on the wage differential between the skilled and unskilled. Second, we consider the case with unemployment, which enables us to compare the effect of a public subsidy policy in the full employment economy and that in the economy with unemployment. Third, we use comparative static analysis, and thus, what we focus is the effect of a marginal increase in the education subsidy on wage inequality, rather than the aggregate effect.
On the other hand, rising wage inequality has attracted great attention of scholars, and the existing literature has put forward different theories to explore the determinants of wage inequality. There are two strands of the literature using general equilibrium approach to study wage inequality that are mostly related to our study. The first strand of the literature emphasizes the role of education in affecting wage inequality in the general equilibrium framework (e.g., Beladi et al., Citation2011; Chaudhuri et al., Citation2018; Gupta & Dutta, Citation2010; Kar & Guha-Khasnobis, Citation2006; Pan, Citation2014; Yabuuchi & Chaudhuri, Citation2009; Zhang, Citation2019b). This strand of the literature also takes international factor mobility,Footnote3 trade liberalization, upstream-downstream linkages, capital adjustment cost, and environmental pollution into consideration when investigating how education affects wage inequality, but this strand of the literature does not take the role of automation into consideration. The second strand of the literature holds that technological change is a crucial determinant of rising wage inequality (e.g., Pi & Zhang, Citation2018; Zhang, Citation2019a), but this strand of the literature fails to consider the interaction between technology and education. This paper contributes by incorporating the interaction between automation and education into two-stage general equilibrium models to explore the distributional effect of a public education subsidy.
We show that in the presence of robotic automation, a public education subsidy can narrow down the wage differential conditional on different stages of skill formation, and on the ratio of unskilled labor to skilled labor. To provide an economic explanation, we compare our results of the basic model with Chaudhuri et al. (Citation2018), who investigate the distributional effect of an education subsidy in a two-stage setup, albeit in the absence of automation. In the first stage, Chaudhuri et al. (Citation2018) find that unskilled workers withdrawing from the labor market will raise both the skilled and unskilled wage rates, an implication similar to ours, but how the wage differential will change is indefinite. In our model, however, the first stage of skill formation will widen the wage differential definitely, because in the presence of automation, a higher unskilled labor cost will also drive up the price of robots, resulting in a higher revenue of the robot-producing sector, and consequently a higher skilled wage rate. We find that although the first stage of skill formation increases both the skilled and unskilled wage rates, the wage differential will be widened unconditionally. Similarly, in the second stage, skilled workers entering the labor market will reduce the wage differential unambiguously. This is because a lower unskilled labor cost will also drive down the price of robots, resulting in a lower revenue of the robot-producing sector, and consequently a lower skilled wage rate. When we consider both effects of the unskilled labor supply and the skilled labor supply, the wage differential in the second stage will be improved if the ratio of unskilled labor to skilled labor is large enough.
The rest of this paper is organized as follows. Section 2 establishes the basic model, which is further analyzed in Section 3. Section 4 extends the basic model by incorporating unemployment into the labor market. Section 5 concludes.
2. The basic model
2.1. The production sectors
There are three sectors using technologies characterized by constant returns to scale in the economy: a robot-producing sector, a robot-using sector, and a non-automatable sector (see e.g., Zhang, Citation2019a).
The robot-producing sector utilizes skilled labor (denoted by ) and capital of type
(denoted by
) to produce robots (denoted by
). The production function of the robot-producing sector is characterized by:
(1)
(1)
The robot-using sector utilizes unskilled labor (denoted by ), robots (denoted by
), and capital of type
(denoted by
) to produce good
Following Prettner (Citation2019) and Zhang (Citation2019a), we interpret capital of type
as traditional capital, and robots as automation capital.Footnote4 Traditional capital such as assembly lines can only imperfectly substitute for unskilled labor, while automation capital in the sense of robots acts as a perfect substitute. Since this sector uses both robots and unskilled labor, we can define the amount of effective labor
as follows:
(2)
(2)
The production function of the robot-using sector is characterized by:Footnote5
(3)
(3)
The non-automatable sector utilizes unskilled labor (denoted by ) and capital of type
(denoted by
) to produce good
This sector is introduced to reflect the phenomenon that the work in some industries cannot be easily automated.Footnote6 As is pointed out by Levy and Murnane (Citation2013, p. 14), building and grounds cleaning, food preparation, and personal care and services are jobs that cannot be easily automated. The production function of the non-automatable sector is characterized by:Footnote7
(4)
(4)
Profit maximization of the above three sectors can be characterized by:
(5)
(5)
(6)
(6)
(7)
(7)
where
and
are the prices of robots and good
respectively. The good of the non-automatable sector
is regarded as the numeraire.
denotes the amount of factor
necessary for the sector to produce one unit of product
(e.g.,
).
and
stand for the skilled wage rate, the unskilled wage rate, the rental rate of capital of type
and the rental rate of capital of type
respectively.
The unskilled wage rate can also be regarded as the unskilled labor cost, and thus, the robot-using sector will compare the unskilled wage rate and the price of robots when it decides the usages of unskilled labor and robots. In equilibrium, we have
2.2. The public education subsidy policy
The government implements a public education subsidy policy for unskilled labor. Similar to Chaudhuri et al. (Citation2018) and Zhang (Citation2019b), we assume that there are two stages of skill formation. In the first stage, unskilled labor can decide whether to get an education and receive the government’s subsidy. If an unskilled worker gets educated in the first stage, then in the second stage, the worker will become skilled and will not be replaced by robots. After finishing the education, the worker can be employed by the robot-producing sector.
We assume that the economy has numbers of unskilled families, and each family has one unit of unskilled labor. In the first stage, the government provides a subsidy
for each unit of unskilled labor that plans to have an education.Footnote8 Hence, if an unskilled family decides that
units of its unskilled labor should get an education, then the family can receive
amount of subsidy from the government to compensate the loss of wage income. The rest of unskilled labor in the family,
goes to work and earns the unskilled wage rate. Thus, in the first stage, the family’s income is
Footnote9 In the second stage,
units of labor become skilled. Now, they can be employed by the robot-producing sector, and earn the skilled wage rate. The rest of labor in the family is still employed by the robot-using or the non-automatable sectors, and earns the unskilled wage rate. Thus, in the second stage, the family’s income is
The representative unskilled family maximizes the total income in the two stages:
(8)
(8)
where
is the time discount factor. We assume that the public subsidy on education
will not be too large or too small, ensuring that there exists an inner solution
By solving the problem, we have
Footnote10 Then, the total amount of unskilled labor available in both stages can be given by:
(9)
(9)
where we can calculate that
and
With these results, we define
and
as the (absolute) elasticities of the unskilled labor supply with respect to
and
respectively.
These elasticities show that when the public subsidy on education or the skilled wage rate increases, unskilled workers will be encouraged to engage in skill formation, and thus the unskilled labor supply will decrease, while when the unskilled wage rate increases, skill formation will be impeded, and thus the unskilled labor supply will increase.
2.3. The market-clearing conditions
In both stages, the unskilled labor supply is among which
amount is utilized by the robot-using sector, and
amount is utilized by the non-automatable sector. For the robot-using sector, the demand for effective labor is
which includes unskilled labor and robots, while for the non-automatable sector, the demand for unskilled labor is
Hence, the unskilled labor market clearing conditions yield:
(10)
(10)
(11)
(11)
Denote as the endowment of skilled labor. Then, in the first stage, the skilled labor supply is exactly
In the second stage, since
amount of unskilled labor acquires skills, the skilled labor supply will become
For the robot-producing sector, the demand for skilled labor is
Thus, the skilled labor market clearing condition in the first stage is described by:
(12)
(12)
In the second stage, the above condition becomes:
(13)
(13)
The capital market clearing conditions in both stages are the same, which are characterized by:Footnote11
(14)
(14)
(15)
(15)
where
and
are the endowments of two types of capital.
3. Comparative static analysis
Section 2 has completed the establishment of the basic model characterizing a full employment economy. In the first stage, EquationEqs. (5)-(7) (10)-(12) (14)(14)
(14) , and Equation(15)
(15)
(15) determine
and
In the second stage, EquationEq. (12)
(12)
(12) should be replaced by EquationEq. (13)
(13)
(13) , and EquationEqs. (5)-(7) (10) (11)
(11)
(11) , and Equation(13)-(15) determine the same variables. The public education subsidy (i.e.,
) is regarded as an exogenous variable decided by the government.
3.1. Comparative static analysis on the first stage
We use the matrix form to characterize the economic system in the first stage. We totally differentiate EquationEqs. (5)-(7) (10)-(12) (14)(14)
(14) , and Equation(15)
(15)
(15) , substitute the results of EquationEqs. (10) (12) (14)
(14)
(14) , and Equation(15)
(15)
(15) into the result of EquationEq. (11)
(11)
(11) , and rearrange EquationEqs. (5)-(7) and Equation(11)
(11)
(11) . Then, we have:Footnote12
(16)
(16)
where
and
Similar to the notations adopted by Jones (Citation1965, Citation1971), we denote
as the cost share of factor
utilized by the sector producing good
(e.g.,
),
as the allocative share of capital of type
utilized by the sector producing good
(e.g.,
), and
as the elasticity of substitution between the related factors utilized by the sector producing good
(e.g.,
). We also use the hat notation to denote the relative change (e.g.,
).
Proposition 1 summarizes the first stage results of how a public education subsidy policy can affect the skilled and unskilled wage rates, and the wage differential in the presence of automation.
Proposition 1:
For a full employment economy with automation, a public education subsidy will increase both the skilled and unskilled wage rates, and widen the wage differential in the first stage.
Proof:
See Appendix A.
A public education subsidy policy will encourage unskilled labor to have an education in the first stage, which will reduce the unskilled labor supply. The fall in the unskilled labor supply will increase the unskilled wage rate, but as the unskilled labor cost increases, the sector that uses robots will want to increase the input of robots in order to dampen the negative impact of the reduction in the unskilled labor supply.
Moreover, the fall in the unskilled labor supply can also reduce the capital productivity in the sector using robots, which will then reduce the payment for capital, as a result of which a part of its capital will flow into the sector that produces robots. The reallocation of capital will raise the capital-labor ratio of the sector that produces robots, and thus increase the skilled labor productivity, resulting in a higher skilled wage rate.Footnote13
Although both the skilled and unskilled wage rates will increase, in the presence of automation the skilled wage rate will be higher than the unskilled one, and thus contribute to the expansion of the wage differential.Footnote14 The reason is that the sector that uses robots needs to increase the usage of robots to offset the relative shortage of unskilled labor. The higher unskilled wage rate will drive up the price of robots, resulting in a higher revenue of the sector that produces robots. More robots will be produced, which suppresses the unskilled wage rate, leading to the expansion of the wage differential.
3.2. Comparative static analysis on the second stage
We use the matrix form to characterize the economic system in the second stage. We totally differentiate EquationEqs. (5)-(7) (10) (11)(11)
(11) , and Equation(13)-(15), substitute the results of EquationEqs. (10)
(10)
(10) and Equation(13)-(15) into the result of EquationEq. (11)
(11)
(11) , and rearrange EquationEqs. (5)-(7) and Equation(11)
(11)
(11) . Then, we have:
(17)
(17)
where
and
which is the ratio of unskilled labor to skilled labor.
Proposition 2 describes the second stage results of how a public education subsidy policy can affect the skilled and unskilled wage rates, and the wage differential in the presence of automation.Footnote15
Proposition 2:
For a full employment economy with automation, when the ratio of unskilled labor to skilled labor is small enough (i.e., ), a public education subsidy will increase the skilled and unskilled wage rates, and widen the wage differential in the second stage. When that ratio is large enough (i.e.,
), then the opposite occurs.
Proof:
See Appendix A.
In the second stage, labor that had an education in the first stage can be regarded as skilled labor, and thus will not be replaced by robots. They can now be employed by the robot-producing sector. On the one hand, a public education subsidy will decrease the unskilled labor supply in the second stage as in the first stage, and its economic mechanism behind has been analyzed in Proposition 1. On the other hand, the skilled labor supply will increase in the second stage, and its economic explanation is given as follows.
More skilled labor available for the sector that produces robots will decrease the skilled wage rate paid by this sector. More skilled labor available for the sector will also increase the capital productivity in this sector. Thus, the sector will increase the payment for capital, and it will make a part of capital utilized in the sector that uses robots move to the sector that produces robots. The less usage of capital in the sector that uses robots will reduce the unskilled labor productivity, resulting in a lower unskilled wage rate. Since a lower unskilled wage rate will make the sector that uses robots to employ more unskilled labor, the price of robots will also become lower. Thus, the unit revenue of robots will fall, which will decrease the skilled wage rate, resulting in an improvement in the wage differential.
When taking both economic mechanisms in the second stage into consideration, we find that if the ratio of unskilled labor to skilled labor is small enough (i.e., ), then both the skilled and unskilled wage rates will be increased, and the wage differential will be expanded. If instead that ratio is large enough (i.e.,
), then the opposite will occur.
4. The extended model
In this section, we introduce unemployment among unskilled labor into the model. When the price of robots falls, replacing unskilled labor with robots may generate unemployment if the unskilled wage rate cannot be downwards adjusted. To reflect this situation, we assume that a regulated unskilled wage rate exists in the robot-using sector, which is downwards rigid and higher than
determined by the labor market. The gap between
and
suggests that in equilibrium, there must exist unemployment among labor who plans to work in the sector that uses robots.
Denote the Harris-Todaro type unemployment rate (Harris & Todaro, Citation1970) as and let
represent the amount of unskilled labor aiming to work in the sector that uses robots. Then, EquationEq. (10)
(10)
(10) should be replaced by:
(18)
(18)
Here, robots will not become unemployed because the price of robots can be adjusted flexibly. However, since the sector is willing to use more robots as long as the price of robots
is lower than
In equilibrium, this implies
The existence of unemployment also reduces the expected payoff for working in the robot-using sector. In equilibrium, the expected return to working in either the robot-using sector or the non-automatable sector should be the same. This implies:
(19)
(19)
Profit maximization of the robot-using sector is now described by:
(20)
(20)
The establishment of the extended model with unemployment has been completed. In the first stage, nine equations, EquationEqs. (5) (7) (11) (12) (14) (15)(15)
(15) , and Equation(18)-(20), determine nine endogenous variables, namely,
and
In the second stage, EquationEq. (12)
(12)
(12) should be replaced by EquationEq. (13)
(13)
(13) , and nine equations, EquationEqs. (5) (7) (11) (13)-(15), and Equation(18)-(20), determine the same variables. The public education subsidy (i.e.,
) is still considered as an exogenous variable decided by the government.
We can easily find that the average unskilled wage rate is exactly equal to Thus,
that is determined by the labor market can also be used to explore the wage differential in the presence of automation.
4.1. Comparative static analysis on the first stage
We use the matrix form to characterize the economic system in the first stage. We totally differentiate EquationEqs. (5) (7) (11) (12) (14) (15)(15)
(15) , and Equation(18)-(20), substitute the results of EquationEqs. (12) (14) (15) (18)
(18)
(18) , and Equation(19)
(19)
(19) into the result of EquationEq. (11)
(11)
(11) , and rearrange EquationEqs. (5) (7) (11)
(11)
(11) , and Equation(20)
(20)
(20) . Then, we have:
(21)
(21)
where
and
Proposition 3 gives the impacts of a public education subsidy on the skilled and unskilled wage rates, and the wage differential in the first stage.Footnote16
Proposition 3:
For an economy with unemployment and automation, a public education subsidy will not change the skilled wage rate, but it will increase the unskilled wage rate, and narrow down the wage differential in the first stage.
Proof:
See Appendix A.
A public education subsidy will reduce the unskilled labor supply. However, since there exists unemployment among unskilled labor, the sector that uses robots can still maintain the same employment level of unskilled labor as before at This suggests that the sector can also maintain the same usage level of capital. As a result, the sector that produces robots can maintain the same levels of inputs. Thus, the skilled wage rate will be unaffected. However, the unemployment rate will become lower, and thus, the expected payoff for unskilled labor will increase. The non-automatable sector will also need to increase the unskilled wage rate so as to attract unskilled labor. In sum, the wage differential will be narrowed down.
When unemployment is taken into account, one can find that Proposition 3 is in contrary to Proposition 1. In Proposition 1, the robot-using sector will use more robots to offset the fall in the unskilled labor supply. Thus, the robot-producing sector will increase the supply of robots. In Proposition 3, however, the robot-using sector will not alter its input levels of unskilled labor and robots. Thus, it will not affect the robot-producing sector. In particular, the skilled wage rate will be unaffected, resulting in an unconditional improvement in wage inequality.
4.2. Comparative static analysis on the second stage
We again use the matrix form to characterize the economic system in the second stage. We totally differentiate EquationEqs. (5) (7) (11) (13)-(15), and Equation(18)-(20), substitute the results of EquationEqs. (13)-(15) (18)(18)
(18) , and Equation(19)
(19)
(19) into the result of EquationEq. (11)
(11)
(11) , and rearrange EquationEqs. (5) (7) (11)
(11)
(11) , and Equation(20)
(20)
(20) . Then, we have:
(22)
(22)
where
Proposition 4 concludes the impacts of a public education subsidy on the skilled and unskilled wage rates, and the wage differential in the second stage.
Proposition 4:
For an economy with unemployment and automation, a public education subsidy will not change the skilled wage rate, but it will increase the unskilled wage rate and reduce the wage differential if the ratio of unskilled labor to skilled labor is small enough (i.e., ) in the second stage. If that ratio is large enough (i.e.,
), then the opposite occurs.
Proof:
See Appendix A.
In the second stage, the unskilled labor supply will decrease, while the skilled labor supply will increase. The former has also appeared in the first stage and has been explained in Proposition 3. Here, we provide economic intuition for the latter.
The higher skilled labor supply for the sector that produces robots will make the skilled wage rate decrease, while more skilled labor used in the sector will raise the capital productivity. Thus, the sector will tend to increase the payment for capital, resulting in the inflow of capital from the robot-using sector. Less capital utilized in the sector that uses robots will encourage the sector to cut down the usages of unskilled labor and robots. This will in turn reduce the capital productivity in this sector, resulting in lower demand for capital. Then, capital is further channeled towards the robot-producing sector. Owing to the existence of the regulated unskilled wage rate, the capital reallocation will alter the return to capital so that this return can finally remain unchanged. More capital used in the sector that produces robots will increase the skilled labor productivity, and will finally alter the skilled wage rate so that it can still remain the same level as before. As for the unskilled wage rate, since more unskilled labor are reallocated to the non-automatable sector, the unskilled wage rate will decrease. As a result, the wage differential will be expanded.
When taking both effects in the second stage into consideration, we find that if the ratio of unskilled labor to skilled labor is small enough (i.e., ), then the wage differential will be decreased. If instead that ratio is large enough (i.e.,
), then the opposite will occur.
Note that under the same conditions of Propositions 1 to 4, we obtain the opposite results regarding wage inequality. We summarize this observation in Proposition 5.
Proposition 5:
For an economy with full employment (resp. unemployment) and automation, a public education subsidy will expand (resp. narrow down) the wage differential in the first stage. In the second stage, a public education subsidy will expand (resp. reduce) the wage differential if the ratio of unskilled labor to skilled labor is small enough, and it will narrow down (resp. expand) the wage differential if the ratio is large enough.
The proof of Proposition 5 is straightforward.
Proposition 5 implies that whether there exists unemployment in the economy with automation plays a crucial role in how a public education subsidy can affect the wage differential.
5. Concluding remarks
By establishing general equilibrium models with two-stage skill formation, this paper examines the impacts of a public education subsidy on the wage differential in the presence of automation. A public education subsidy will reduce the unskilled labor supply in both stages, and will raise the skilled labor supply in the second stage. For a full employment economy, the wage differential will be widened in the first stage. In the second stage, if the ratio of unskilled labor to skilled labor is small (resp. large) enough, then the wage differential will be expanded (reps. narrowed down). For an economy with unemployment, the wage differential will be reduced in the first stage. In the second stage, if that ratio is small (resp. large) enough, then the wage differential will be narrowed down (reps. expanded). We can find that when there exists unemployment, the wage differential may be reduced in both stages.
There are several possible extensions for this paper. First, we could consider the ownership of robots, and investigate how an education subsidy can affect wealth inequality in the presence of automation. Second, the robot-using sector might also employ skilled labor. In this case, automation capital might complement skilled labor although it displaces unskilled labor (Acemoglu & Restrepo, Citation2018a; Prettner & Strulik, Citation2020). Third, unskilled labor might have heterogeneous innate ability, which might determine whether they would acquire skills. Fourth, rising inequality may also in turn impede skill formation (Taresh et al., Citation2021).
Disclosure statement
No potential conflict of interest was reported by the author.
Notes
1 Although in 1990s the demand for skilled labor has slowed, the race between education and technology as a simple but powerful supply-demand framework can still be warranted by the data (Autor, Citation2014).
2 In addition to technological change, there exist other factors that may contribute to the rising skill premium, such as rising international competition, declining bargaining power of labor unions, and inadequate redistribution policy.
3 International factor mobility is an important cause of skilled-unskilled wage inequality. Wang (Citation2019) investigates the impacts of international factor mobility on wage inequality when there exists a public sector in the economy.
4 There are also other ways modeling automation. For example, we can distinguish between automatable tasks and human tasks (Acemoglu & Restrepo, Citation2018a, Acemoglu & Restrepo, Citation2018b).
5 Prettner (Citation2019) adopts the Cobb-Douglas form (e.g., ). In this paper, we do not require a specific production form.
6 Workers replaced by robots usually go to the service sector (Autor & Dorn, Citation2013). In this sense, the non-automatable sector may be regarded as a service sector. In principle, the existence of Polanyi’s paradox limits the range of technologically automatable tasks (Autor, Citation2015).
7 We can hold that the sector mainly provides unstructured services (e.g., Levy & Murnane, Citation2013). Thus, following Chaudhuri (Citation2014) and Chaudhuri et al. (Citation2017), we assume that the sector employs another type of capital.
8 It is identical to assume that the government increases education investment so that the unskilled family can reduce its opportunity cost when part of unskilled labor exits from the labor market in order to receive an education in the first stage.
9 Here, we follow Chaudhuri et al. (Citation2018) and assume that unskilled families do not save.
10 Chaudhuri et al. (Citation2018, p.173) present the detailed results.
11 Here, we have shut down the impact of capital accumulation.
12 According to the linear homogeneity property of production functions, we can simplify Take the robot-producing sector as an example.
implies
Totally differentiating
yields
which can be used to simply the differentiating result of Eq. (2).
13 A higher unskilled wage rate does not imply that capital will withdraw from the robot-producing sector and be employed in the sector that uses robots, then leading to a higher return to capital. Actually, totally differentiating Eq. (6), we have which shows that a higher unskilled wage rate will accompany a lower return to capital. It is because that a higher unskilled wage rate raises the production cost for the sector using robots. Although the sector can substitute capital for labor, the production scale will shrink, which will reduce the demand for capital aggregately, resulting in a lower return to capital.
14 Chaudhuri et al. (Citation2018) do not consider automation, and they find that wage inequality changes conditionally in the first stage. Different from Chaudhuri et al. (Citation2018), we obtain an unconditional result in the first stage when considering automation.
15 Following Chaudhuri et al. (Citation2018) and Zhang (Citation2019b), we compare the skilled and unskilled wage rates in the second stage with those in the initial stage. If we compare the wage rates with those in the first stage, then this analysis can be conducted by replacing Eq. (11) with where
is exogenous in the second stage.
16 Although our focus is on wage inequality, the impacts of a public education subsidy on unemployment can be easily investigated. From Eq. (19), we can find that the unemployment rate changes reversely with the unskilled wage rate.
17 We do not display the matrix here in order to save space.
References
- Acemoglu, D., & Autor, D. (2012). What does human capital do? A review of Goldin and Katz’s The race between education and technology. Journal of Economic Literature, 50(2), 426–463. https://doi.org/10.1257/jel.50.2.426
- Acemoglu, D., & Restrepo, P. (2018a). Low-skill and high-skill automation. Journal of Human Capital, 12(2), 204–232. https://doi.org/10.1086/697242
- Acemoglu, D., & Restrepo, P. (2018b). The race between man and machine: Implications of technology for growth, factor shares, and employment. American Economic Review, 108(6), 1488–1542. https://doi.org/10.1257/aer.20160696
- Anwar, S. (2006). Factor mobility and wage inequality in the presence of specialisation-based external economies. Economics Letters, 93(1), 88–93. https://doi.org/10.1016/j.econlet.2006.03.042
- Anwar, S. (2008). Labor inflow induced wage inequality and public infrastructure. Review of Development Economics, 12(4), 792–802. https://doi.org/10.1111/j.1467-9361.2008.00453.x
- Autor, D. H. (2014). Skills, education, and the rise of earnings inequality among the “other 99 percent". Science (New York, N.Y.), 344(6186), 843–851. https://doi.org/10.1126/science.1251868
- Autor, D. H. (2015). Why are there still so many jobs? The history and future of workplace automation. Journal of Economic Perspectives, 29(3), 3–30. https://doi.org/10.1257/jep.29.3.3
- Autor, D. H., & Dorn, D. (2013). The growth of low-skill service jobs and the polarization of the US labor market. American Economic Review, 103(5), 1553–1597. https://doi.org/10.1257/aer.103.5.1553
- Autor, D. H., Katz, L. F., & Kearney, M. S. (2006). The polarization of the U.S. labor market. American Economic Review, 96(2), 189–194. https://doi.org/10.1257/000282806777212620
- Autor, D. H., Katz, L. F., & Kearney, M. S. (2008). Trends in U.S. wage inequality: Revising the revisionists. Review of Economics and Statistics, 90(2), 300–323. https://doi.org/10.1162/rest.90.2.300
- Beladi, H., Chakrabarti, A., & Marjit, S. (2010). Skilled-unskilled wage inequality and urban unemployment. Economic Inquiry, 48(4), 997–1007. https://doi.org/10.1111/j.1465-7295.2009.00247.x
- Beladi, H., Chaudhuri, S., & Yabuuchi, S. (2008). Can international factor mobility reduce wage inequality in a dual economy? Review of International Economics, 16(5), 893–903. https://doi.org/10.1111/j.1467-9396.2008.00751.x
- Beladi, H., Marjit, S., & Broll, U. (2011). Capital mobility, skill formation and polarization. Economic Modelling, 28(4), 1902–1906. https://doi.org/10.1016/j.econmod.2011.03.019
- Bentaouet Kattan, R., Macdonald, K., & Patrinos, H. A. (2021). The role of education in mitigating automation’s effect on wage inequality. LABOUR, 35(1), 79–104. https://doi.org/10.1111/labr.12187
- Brynjolfsson, E., & McAfee, A. (2014). The second machine age: Work, progress, and prosperity in a time of brilliant technologies. W. W. Norton & Company.
- Chaudhuri, S. (2014). Foreign capital, non-traded goods and welfare in a developing economy in the presence of externalities. International Review of Economics & Finance, 31, 249–262. https://doi.org/10.1016/j.iref.2014.02.003
- Chaudhuri, S., Ghosh, A., & Banerjee, D. (2018). Can public subsidy on education necessarily improve wage inequality? International Review of Economics & Finance, 54, 165–177. https://doi.org/10.1016/j.iref.2017.08.005
- Chaudhuri, S., Kumar Dwibedi, J., & Biswas, A. (2017). Subsidizing healthcare in the presence of market distortions. Economic Modelling, 64, 539–552. https://doi.org/10.1016/j.econmod.2017.04.011
- Goldin, C., & Katz, L. (2008). The race between education and technology. Harvard University Press.
- Gupta, M. R., & Dutta, P. B. (2010). Skilled–unskilled wage inequality, nontraded good and endogenous supply of skilled labour: A theoretical analysis. Economic Modelling, 27(5), 923–934. https://doi.org/10.1016/j.econmod.2010.05.013
- Harris, J. R., & Todaro, M. P. (1970). Migration, unemployment and development: A two-sector analysis. American Economic Review, 60, 126–142.
- Jones, R. W. (1965). The structure of simple general equilibrium models. Journal of Political Economy, 73(6), 557–572. https://doi.org/10.1086/259084
- Jones, R. W. (1971). A three factor model in theory, trade, and history. In J. N. Bhagwati, R. W. Jones, R. A. Mundell & J. Vanek (Eds.), Trade, balance of payments and growth: Papers in international economics in Honor of Charles P. Kindleberger (pp. 3–21). North-Holland.
- Kar, S., & Guha-Khasnobis, B. (2006). Economic reform, skill formation and foreign capital. The World Economy, 29(1), 79–94. https://doi.org/10.1111/j.1467-9701.2006.00759.x
- Lankisch, C., Prettner, K., & Prskawetz, A. (2019). How can robots affect wage inequality? Economic Modelling, 81, 161–169. https://doi.org/10.1016/j.econmod.2018.12.015
- Levy, F., & Murnane, R. (2013). Dancing with robots: Human skills for computerized work. NEXT report, Third Way.
- Marjit, S., & Kar, S. (2005). Emigration and wage inequality. Economics Letters, 88(1), 141–145. https://doi.org/10.1016/j.econlet.2005.02.003
- Okada, K. (2020). Dynamic analysis of education, automation, and economic growth. Graduate School of Economics and Osaka School of International Public Policy (OSIPP). Osaka University Discussion Papers in Economics and Business, 20, 1–31.
- Pan, L. (2014). The impacts of education investment on skilled–unskilled wage inequality and economic development in developing countries. Economic Modelling, 39, 174–181. https://doi.org/10.1016/j.econmod.2014.02.040
- Pi, J., & Fan, Y. (2019). Urban bias and wage inequality. Review of Development Economics, 23(4), 1788–1799. https://doi.org/10.1111/rode.12603
- Pi, J., & Zhang, P. (2018). Skill-biased technological change and wage inequality in developing countries. International Review of Economics & Finance, 56, 347–362. https://doi.org/10.1016/j.iref.2017.11.004
- Pi, J., & Zhang, P. (2020). Organized crime and wage inequality. Scottish Journal of Political Economy, 67(3), 344–361. https://doi.org/10.1111/sjpe.12238
- Pi, J., & Zhou, Y. (2012). Public infrastructure provision and skilled-unskilled wage inequality in developing countries. Labour Economics, 19(6), 881–887. https://doi.org/10.1016/j.labeco.2012.08.007
- Prettner, K. (2019). A note on the implications of automation for economic growth and the labor share. Macroeconomic Dynamics, 23(3), 1294–1301. https://doi.org/10.1017/S1365100517000098
- Prettner, K., & Strulik, H. (2020). Innovation, automation, and inequality: Policy challenges in the race against the machine. Journal of Monetary Economics, 116, 249–265. https://doi.org/10.1016/j.jmoneco.2019.10.012
- Taresh, A. A., Sari, D. W., & Purwono, R. (2021). Analysis of the relationship between income inequality and social variables: Evidence from Indonesia. Economics & Sociology, 14(1), 103–119. https://doi.org/10.14254/2071-789X.2021/14-1/7
- Tawada, M., & Sun, S. (2010). Urban pollution, unemployment and national welfare in a dualistic economy. Review of Development Economics, 14(2), 311–322. https://doi.org/10.1111/j.1467-9361.2010.00554.x
- Wang, D. (2019). International labour movement, public intermediate input and wage inequality: A dynamic approach. Economic Research-Ekonomska Istraživanja, 32(1), 1–16. https://doi.org/10.1080/1331677X.2018.1546123
- Yabuuchi, S., & Chaudhuri, S. (2009). Skill formation, capital adjustment cost and wage inequality. Review of Urban & Regional Development Studies, 21(1), 2–13. https://doi.org/10.1111/j.1467-940X.2009.00156.x
- Zhang, P. (2019a). Automation, wage inequality and implications of a robot tax. International Review of Economics & Finance, 59, 500–509. https://doi.org/10.1016/j.iref.2018.10.013
- Zhang, P. (2019b). Skill formation, environmental pollution, and wage inequality. The Annals of Regional Science, 62(2), 405–424. https://doi.org/10.1007/s00168-019-00901-6
Appendix A:
Proofs
Proof of Proposition 1: We denote the determinant of the coefficient matrix of EquationEq. (16)(16)
(16) as
According to Appendix B,
should be positive in order to ensure the local stability of the economic system. Then, by solving EquationEq. (16)
(16)
(16) , we have:
Wage inequality is expressed as follows:
Proof of Proposition 2: We denote the determinant of the coefficient matrix of EquationEq. (17)(17)
(17) as
The adjustment processes that are similar to Appendix B show that
should be positive in order to ensure the local stability of the economic system. Then, by solving EquationEq. (17)
(17)
(17) , we have:
Accordingly, wage inequality can be described as follows:
Recall that Hence, if
then
and
and if
then
and
Note that
represents the ratio of unskilled labor to skilled labor.
Proof of Proposition 3: By calculating the determinant of the coefficient matrix of EquationEq. (21)(21)
(21) (denoted as
), we have:
Then, by solving EquationEq. (21)(21)
(21) , we have:
Furthermore, wage inequality can be described as follows:
Proof of Proposition 4: We denote the determinant of the coefficient matrix of EquationEq. (22)(22)
(22) as
The adjustment processes similar to Appendix B show that
should be positive in order to ensure the local stability of the economic system. Then, by solving EquationEq. (22)
(22)
(22) , we have:
Accordingly, wage inequality can be described as follows:
Hence, if then
and
and if
then
and
Note that
represents the ratio of unskilled labor to skilled labor.
Appendix B:
The adjustment processes
Similar to the methodology used by Beladi et al. (Citation2008) and Zhang (Citation2019b), the adjustment processes of EquationEqs. (2) (5) (7) (10)-(12) (14)(14)
(14) , and Equation(15)
(15)
(15) can be specified as follows:
(B-1)
(B-1)
(B-2)
(B-2)
(B-3)
(B-3)
(B-4)
(B-4)
(B-5)
(B-5)
(B-6)
(B-6)
(B-7)
(B-7)
(B-8)
(B-8)
where
represents the adjustment speed, and the dot over a variable denotes the time derivative (e.g.,
).
We solve for linear approximations of Eqs. (B-1)-(B-8), and denote the corresponding Jacobian matrix as Footnote17
is an
matrix. Since
has even rows,
is required for the local stability. By calculation and rearrangement, we can obtain
where
is a positive parameter. Hence, to ensure that the economy system is locally stable,
should be positive.