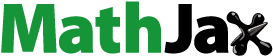
Abstract
The coronavirus outbreak in 2020 shattered economies, public health and public well-being worldwide literally overnight. In response to the pandemic, most countries implemented a delicate balance of policy stringency and economic support to ensure public health, social security and a vibrant economy. With the pandemic slowly phasing out, our article explores the effectiveness of various governmental strategies for ensuring economic growth. The proposed econometric model is tested using panel quarterly data for 49 (37 OECD + 12 non-OECD) countries for all four quarters of 2020 and the first quarter of 2021. Our findings show that policy stringency and economic support are both negatively associated with economic growth. We also find that the stringency was largely responsive, oriented to preventing the collapse of health systems after infections had already become widespread, not towards saving human lives by preventing soaring levels of infection. While our findings appear to lend support for the view that a trade-off between human lives and the economy was inevitable, we also challenge this view by evidence that some countries were able to secure a double dividend of maintaining public health and a vibrant economy by a prudent far-sighted stringency policy of preventing the virus outbreak.
1. Introduction
In early March 2020, COVID-19 was declared a global pandemic. The world has encountered an unprecedented situation in modern history with exponentially rising COVID infections and human deaths, national health systems being overloaded and widespread economic upheaval (European Investment Bank, Citation2021). Unprepared to deal with this situation, governments around the world have taken different paths in an attempt to solve the health crisis by imposing policies of varying stringency (Hale et al., Citation2020).
On one extreme, high-stringency countries imposed complete lockdowns and minimized human contact in an effort to prevent the virus’ spread, with China being a typical representative. On the other extreme, low-stringency countries imposed minimal measures to prevent human contact, where Sweden is a good representative. Yet, most countries opted for a middle way by striking a delicate balance of measures of varying stringency as concerns the lockdown and the fiscal/financial dimension (public and private social welfare) to simultaneously protect public health, social security and ensure a vibrant economy (Fetzer et al., Citation2021; König & Winkler, Citation2021).
The stringency measures imposed by most countries entailed restrictions on the movement of people and goods, declarations of a state of emergency, travel and working bans and so forth. In turn, these measures effectively led to lower overall demand, reduced capacity, business closures and job cuts in many sectors and economies as a whole (Charlton & Castillo, Citation2022). Economic output further suffered when in early spring 2020 the situation triggered panic among the population, which held back on purchasing any items not seen as absolutely necessary. For a brief moment in spring 2020, it seemed as if the world had ground to an economic halt (OECD, 2021).
Alongside these stringent measures, many governments introduced economic support (fiscal/financial) measures to ease the adverse effects of the stringent measures. The lower effective demand due to the stringent measures saw many companies cut jobs, put workers on furlough and reschedule/renegotiate their loan obligations in order to ensure their liquidity and long-term viability. Governments provided aid via two channels. One involved increasing social transfers to vulnerable residents and the other postponing companies’ imminent financial obligations to sometime later (König & Winkler, Citation2020).
Despite these endeavours, the global COVID-19 death count long ago surpassed 5 million, with the world economy on average having contracted by around 5% in 2020 (IMF, Citation2021a, OECD, Citation2021). While COVID-19 has had devastating global effects, one cannot say that all countries have been affected the same (Hu et al., Citation2021). For example, Spain’s GDP contracted 21.59% in the second quarter of 2020 and 10.8% in 2020 (BBVA Research, 2021) in conjunction with one of the highest death rates per capita. On the contrary, Taiwan’s GDP contracted by less than 1% in the second quarter of 2020 and grew 3.11% in 2020 (National Statistics Bureau Taiwan, 2021), which occurred together with one of the lowest death per capita rates.
The observation in the above paragraph motivated an interesting research question: Is there an inevitable trade-off between public health and the economy, as politicians and economists often tell us (Carrieri et al., Citation2021; Marinč et al., Citation2021; McKee & Stuckler, Citation2020), or can these two goals be accomplished simultaneously? If the former is true, policymakers then have no other choice than to make a delicate trade-off between protecting both human life and the economy. If the latter is true, policymakers can achieve the double dividend of protecting human life and the economy at once.
To answer this question, the article reported herein empirically explores the effectiveness of governmental strategies to protect economic growth during the COVID-19 pandemic. The proposed panel model is tested using quarterly data for 49 (37 OECD + 12 non-OECD) countries for all four quarters of 2020 and the first quarter of 2021.
The article provides several important contributions to the literature. From a theoretical and practical perspective, we distinguish between protective and responsive policy stringency. While the measures deployed are the same in either case, they differ with respect to their purpose. In our sample, stringency was predominantly responsive, oriented to preventing the collapse of health systems after infections had already become widespread.
Second, we challenge the conventional view that trade-off between policy stringency and economic growth was inevitable (Havrlant et al., Citation2021). Although we do find a negative relationship between policy stringency and economic growth in our sample, we identify also countries that have achieved a double dividend of protecting both human life and a vibrant economy. These countries have imposed protective stringency before virus became widespread, meaning that prudent and foremost far-sighted policy was able to deliver such outcome.
Third, we find that economic support is also negatively associated with economic growth. Ideally, economic support should reconcile the negative economic and employment dynamics caused by COVID-19 pandemic (Havrlant et al., Citation2021; Su et al., Citation2021), but apparently transfers to the population and businesses were not effective in reversing this dynamics. The positive effect of economic support is helping many individuals and businesses to survive this unprecedented crisis, although Boratyńska (Citation2021) points to a problem of saving also zombie companies whose economic viability is questionable even without the pandemics.
Fourth, we also make a methodological contribution by deploying a novel approach to policy stringency and economic support measurement. This approach was inevitable to secure a meaningful comparison between variables in our panel model as policy variables are measured on a daily basis while economic variables are measured on a quarterly basis.
The rest of the article is structured as follows. Section 2 provides a literature review and develops the hypotheses. Section 3 outlines the method including data collection and modelling. Section 4 sets out the findings, while the article concludes with a discussion section.
2. Policy review and hypothesis development
2.1. COVID-related governmental policies
In response to COVID-19, governments around the world have taken measures to facilitate the lives of people and businesses (Bouri et al., Citation2021; Kaplan et al., 2022; König & Winkler, Citation2020; Pan & Yue, Citation2021). The policy tracker provided by the International Monetary Fund (IMF, Citation2021a), including the responses to the COVID-19 pandemic of 197 countries, is perhaps the most comprehensive overview in this regard (IMF, Citation2021a). Governmental responses may be classified in two main groups: stringent measures to limit the virus’ spread and economic support to help people and businesses survive the pandemic (Hu & Zhang, Citation2021).
Policies of stringency (i.e., lockdowns) have been imposed with the aim to minimize human contact and thereby the spread of the virus. Measures of varying stringency have been deployed to different extents in different countries and time periods. The most extreme stringency entails complete lockdowns where people are not allowed to leave home for purposes other than getting medical help or purchasing essential supplies (Goel et al., Citation2021; González-Bustamante, Citation2021). Lower levels of stringency allow most businesses to continue operating but still prevent large gatherings of people (e.g., sports events, cultural events, university lectures, on-site schooling, etc.) (Tartavulea et al., Citation2020). The least severe levels of stringency also allow larger gatherings of people, provided that minimum security measures are upheld (masks, distance, disinfection) (Coccia, Citation2021; Wilkins et al., Citation2021).
Although the very essence of such stringent measures is to prevent the negative effects of the COVID pandemic, conceptually we can distinguish two types of prevention. One type of prevention focuses on minimizing infections and deaths before they gather pace. We label this type as protective stringency. Typical representatives include China, Taiwan and New Zealand. The second type of prevention concentrates on preventing the collapse of the health system after infections and deaths have already taken off. We label this type as responsive stringency. Representatives include Italy, Spain, the UK and the United States. While the measures deployed are the same for both types, the goals they pursue are distinct (Bajra & Čadež, Citation2019).
presents a list of the most and least stringent jurisdictions in the second quarter of 2020 for the 49 countries sampled in this study, showing that countries varied greatly in the stringency of their measures. In the second quarter of 2020, Argentina topped the list with a stringency index of 92.59 while Japan was found at the bottom with a stringency index of 38.51.
Table 1. List of the most and least stringent jurisdictions sampled in this study in the second quarter of 2020.
Economic support policies, the second group of responses, were typically deployed to offset the negative impacts of the stringency measures introduced for both businesses and citizens. Stringent (lockdown) measures effectively led to the complete closure of some businesses or saw them contract considerably. To help survive the crisis citizens were often being supported with direct social transfers while businesses were being relieved with direct subsidies, postponements of the payment of their liabilities, taxes and pension contributions, loan reschedulings and so forth. Most countries have given particular attention to liquidity in the banking sector and thus it is no surprise that the financial sector has survived the pandemic relatively intact (International Monetary Fund, Citation2021b, Citation2021d). This has enabled banks to push back businesses’ and individuals’ instalment payments to later time periods as state governments have guaranteed these actions. One negative result of these measures is a significant rise in public debt (Rant et al., Citation2021), in some countries reaching unprecedented levels of up to 250% of GDP (e.g., Japan) (Ahrens & Ferry, Citation2020; Hotle & Mumbower, Citation2021).
Despite these measures, many countries have suffered greatly both in terms of human life and economic output (Hulpke & Fronmueller, Citation2021). The global COVID-19 death toll long ago surpassed the 3 million mark and the average quarterly growth rate for the world economy in 2020 entailed a contraction of 5% (OECD, Citation2021). In the EU, Italy was among the hardest hit by high death rates, followed by the UK, France and Spain. For a while, Italy was also one of the few developed countries to have experienced the temporary collapse of its health system due to the system being overloaded by COVID-19 patients (Jones et al., Citation2020).
Although faced with very similar circumstances, the economic consequences have differed widely across countries (Jordà et al., 2022; Spash, 2021). Some countries have seen economic contractions unprecedented in modern history. For example, in Spain, the UK, India and Mexico, the gross output in the second and third quarters of 2020 was more than 15% lower than in the same period of 2019, which is 4 to 5 times larger than any other quarterly decline recorded for these countries. In Peru, for instance, the year-on-year decline even exceeded 30% as a result of the almost complete lockdown during the second quarter of 2020. It is worth noting that all the countries mentioned in this paragraph also fared poorly in terms of the COVID-19 death toll (Feng et al. Citation2021; International Monetary Fund, Citation2021c).
In contrast, the economic impact of COVID-19 in some countries has been much more modest. Taiwan’s gross output, for example, was less than 1% lower in the second quarter of 2020 than in the same period of 2019. Finland, Lithuania and South Korea experienced GDP declines of some 2% or less over the same period of 2019. Again, it worth noting that all the countries mentioned in this paragraph also managed to keep their COVID-19 death rates low (Erokhin & Gao, Citation2020; Jeong et al., Citation2020; Lai et al., Citation2020). Evidence also shows that countries with similar GDP falls have experienced very different levels of COVID-19 deaths. For instance, a comparative analysis between the United States, Sweden, Denmark and Poland reveals that all countries encountered a similar economic contraction (about 3–4.5%), but their death rates varied significantly: the United States and Sweden recorded 5 to 10 times more deaths per million than other countries in the analysis (Gordon et al., Citation2021; Verma et al., Citation2021).
2.2. Hypothesis development
We are interested how policy stringency and economic support (predictor variables) have influenced economic growth (criterion variable) during COVID-19 pandemic. Furthermore, we are also interested in the prevalent type of stringency, i.e., responsive vs. protective.
As for the policy stringency–economic growth relationship, two competing rationales can be found in the literature (Clark & Lepinteur, Citation2021; Jiang et al., Citation2021). The conventional wisdom holds that stringency is detrimental to economic output (Carrieri et al., Citation2021). Stringency means business restrictions and closures that prevent products, services and money from freely circulating in the economy and between economies (König & Winkler, Citation2020). In turn, corporate revenues decline, workers are furloughed or laid off, governments collect less tax on one side and need to increase their spending on the other to maintain social security and help companies hit by the measures (Su et al., Citation2021). This vicious cycle creates lower output and higher public debt.
The competing rationale holds that stringency benefits the economy as a whole, although some sectors reliant on close human contact (tourism, hospitality, etc.) are disproportionally badly affected (Pappas, Citation2021; Traskevich & Fontanari, Citation2021). This rationale assumes that IT technology has made it possible for most sectors to operate relatively undisturbed online. Moreover, greater stringency reduces the uncertainties for businesses compared to less stringency, which needs to adopt policies in line with public health conditions literally on a daily basis to avoid a breakdown of the health system. Finally, higher stringency means that fewer people become infected/die, meaning they temporarily/permanently cease to participate in the economic system as either workers or customers (Bajra & Čadež, Citation2018a; Garzillo et al., 2022; Kartseva & Kuznetsova, Citation2020).
Given these two competing rationales revealing different hypothetical directions in the relationship, we formulate the following hypothesis:
Hypothesis 1: COVID-19 policy stringency is significantly associated with quarterly GDP growth.
As concerns economic support, once again two competing rationales can be detected in the literature. One rationale holds that social security transfers to people on furlough increase effective demand and, in turn, corporate revenues and profits. Further, financial aid to companies helps them to postpone payments and allows them to direct scarce resources towards productive use that will generate revenues and profits. Taken collectively, fiscal and financial aid is seen as beneficial for economic output (International Monetary Fund, Citation2021c; König & Winkler, Citation2020).
The competing rationale maintains that economic support leads to negative output. This is because the recipients of social security transfers cannot spend these funds due to the stringent measures in place or do not want to spend these funds in the presence of longer-term job and health uncertainties (Bajra, Citation2021). In effect, the economy as a whole would be better off if governments were to increase their spending directly rather than channelling funds to residents who do not put these funds to productive use (Cadez et al., Citation2019). Again, given two competing rationales showing different hypothetical directions in the relationship, we formulate the hypothesis as follows:
Hypothesis 2: Economic support is significantly associated with quarterly GDP growth.
Concerning the prevalent type of stringency, i.e., responsive vs. protective, we do not posit a hypothesis formally. We argue however that responsive stringency would me manifested by a positive relationship between stringency and COVID infections/deaths while protective stringency would be manifested by a negative relationship between stringency and COVID infections/deaths.
3. Methodology
3.1. Sample
Testing the impact of government responses to the COVID-19 pandemic on economic growth is made challenging by the short time series of data available since its outbreak. Our approach focuses on testing a panel econometric model that uses quarterly data for variables of interest for 2020 and the first quarter of 2021 for 49 countries, making a total of 245 country quarter observations.
The core of the sample is 37 OECD countries. OECD countries were selected due to data availability and their representativeness of the global economy. The area generates well over 50% of world GDP and has also reported high figures of lives lost due to the COVID-19 pandemic. In addition, in order to increase sample size, we added 12 OECD partner countries for which we could obtain the data.
3.2. Model specification
The hypotheses are tested using the following fixed effects panel regression model, including two test variables and four control variables:
(1)
(1)
Gross fixed capital formation (GFCF) growth is included to control for how much of the total factor income is reinvested in new fixed assets rather than consumed. GFCF includes land improvements (fences, ditches, drains and so on); plant, machinery and equipment purchases; the construction of roads, railways and the like, including schools, offices, hospitals, private residential dwellings and commercial and industrial buildings for country (i) at time (t).
The balance of payments (BOP) macroeconomic indicator is important because it affects the state's ability to make international payments and indirectly shows the stability of the financial system in the country as the difference between the import and export of goods and services during the period under study (Bajra et al., in press; König & Winkler, Citation2020). The BOP shows the difference in total value between payments into and out of country (i) in period (t).
COVID infections growth and the incidence of COVID deaths are included in the model to control whether the rates of infection and deaths have affected the stringency index and thereby the economic output in country (i) at time (t). We expect a higher number of infected and/or deaths to be associated with a higher stringency index and vice versa.
Finally, the model employs both time fixed-effects (uit) and country fixed-effects (eit). εit stands for error terms.
3.3. Data collection and variable measurement
Data were collected from a range of sources. Policy stringency and economic support, the key test variables of inquiry, were measured by the stringency and economic support indices developed by Oxford University (The Oxford COVID-19 Government Response Tracker (OxCGRT)), which highlights the efforts made by countries in response to the pandemic. These measures have been validated and used widely in other studies (Hale et al., Citation2020; Jiang et al., Citation2021).
The stringency index is a composite measure based on nine indicators: school closures; workplace closures; cancellation of public events; restrictions on public gatherings; closures of public transport; stay-at-home requirements; public information campaigns; restrictions on internal movements; and international travel controls. The index assigns scores between 0 (no measures) and 100 (highest stringency). Since the index is calculated on a daily basis, we computed an average value for each quarter from daily data.
The economic support index is also a composite measure based on four indicators: direct transfers to people not working due to the pandemic; debt relief for households; fiscal spending to stimulate the economy; and international support. The index gives scores between 0 (no aid) and 100 (highest aid).
Real GDP growth, the dependent variable in this study, compares GDP in a particular country over the same period in the previous pre-corona year, adjusted for the inflation rate. The source of data is the OECD (Citation2021).
Gross fixed capital formation (GFCF) growth is measured as a percentage change of capital formation over the same period in a previous pre-corona year. The stock variable represents the acquisition of produced assets (including purchases of second-hand assets) and the production of such assets by producers for their own use, minus disposals (European Investment Bank, Citation2021, pp. 55). The source of data is the OECD (Citation2021).
Balance of payments (BOP) is measured as a percentage of GDP. The source of data is once again the OECD (Citation2021).
The COVID infections growth variable shows the relative increase in infections from quarter to a quarter per 100,000 inhabitants for country i in quarter t. The variable is represented as a ratio. Value 1 means that infections in period t are exactly the same as in the preceding period, values greater than 1 indicate the growth of infections, while values below 1 indicate a drop in infections.
The COVID deaths incidence is the number of COVID-related deaths per million people for country i in quarter t. Data for infections and deaths were retrieved from Our World in Data (Hale et al., Citation2020) and John Hopkins University.
4. Results
4.1. Descriptive statistics
presents descriptive statistics for the test and control variables. All variables are per quarter (not the entire year).
Table 2. Descriptive statistics.
As evident in , the average quarterly decline of GDP in the five quarters under examination is −3.65%, yet with strong variability. The largest negative decline in the sample was −23.47% (India in the second quarter of 2020) whereas the highest growth was a commendable 8.93%.
As for the stringency index, the mean recorded index is 53.66, with the lowest observed value being 6.85. This clearly shows that all governments have taken at least some measures to prevent the spread of the virus. The highest variable observed is 92.59 (Argentina in the second quarter of 2020), meaning that some countries were in almost full lockdown for a whole quarter. The economic support mean index is 55.78, although with greater variability than the stringency index. While some countries have the highest possible value of 100, some countries also score 0.
The mean growth of gross fixed capital formation is 2.29%, but with considerable variability. The minimum value was −65.29% whereas the maximum value was 104.50%. The mean value for the BOP variable was 0.90%, with the minimum value of −15.3% and maximum value of 14.87%.
The mean COVID infections growth ratio is 9.29, meaning that on average COVID infections had raised almost tenfold compared to the preceding period. The highest recorded value is 418.23, namely, that infections had risen more than 400-fold (i.e., by 40,000%) over the previous period. It was recorded in the second quarter of 2020 in India which had a relatively low base in Q1.
The mean COVID deaths incidence is 19.23 people per million. The highest recorded value of 956.23 was found in Mexico in the second quarter of 2020, which means that almost 1 in 1000 people died from COVID.
4.2. Model testing
It is important to meet the required econometric/statistical assumptions when deploying a panel regression econometric model. In the first instance, we performed diagnostic tests related to multicollinearity and heteroskedasticity. Multicollinearity is directly related to the correlation between independent variables and thus we present the Pearson correlation matrix and variance inflation factors (VIFs) in . We also applied the Bruch–Pagan test of heteroskedasticity. The test revealed that heteroskedasticity is not present in our data and detailed results are hence not reported. Next, we performed the Hausman test to determine whether the fixed effects model is more suitable for our data than a random effects model. The Hausman test showed that the fixed effects model is appropriate. Finally, we ran a panel regression analysis (whose results are presented in ).
Table 3. Pearson correlation matrix and variance inflation factors.
Table 4. Fixed effects panel regression analysis (dependent variable GDP growth).
As shows, the stringency index (–0.41) and economic support index (–0.27) are both negatively correlated with GDP growth. The correlation coefficient between the stringency index and the economic index is positive and fairly high, i.e., 0.63. This is the highest pairwise correlation between independent variables and contributes to the moderate but not excessive correlation between variables, as shown by the mean variance inflation factor. We therefore dismissed multicollinearity as a serious threat to our data analysis. Interestingly, there is no correlation between growth in infections and the incidence of deaths.
Of great relevance, indicates that the stringency index is positively related to both COVID infections and COVID deaths. This occurrence clearly suggests that countries deployed the responsive type of stringency, i.e., preventing the health system from collapsing after the infections and deaths had already gathered pace, and not preventing human life by preventing the infections from soaring in the first place.
The regression results are presented in . Including only direct effects, Model 1 shows that stringency index is negatively and significantly associated with GDP growth. On the contrary, the relationship between the economic support index and GDP growth is not significant. As for the control variables, COVID infection growth and COVID death incidence had a negative impact on GDP growth.
In model 2, we also included the interaction between the stringency index and the economic support index (stringency*economic). While the interaction term statistically significantly positively influences GDP growth, the direct impact of economic support on GDP growth also becomes statistically significant in model 2. Otherwise, all the other coefficients remain robust in model 2 compared to model 1. The negative direct effects of policy stringency and economic policy in conjunction with their positive interaction effect suggest that a well-designed combination of both policies to some (but relatively small) extent mitigates the negative impact of both policies working in isolation. In both models, the fixed time effects are reported to change over time, as individual fixed effects vary between countries.
5. Discussion and conclusion
This article has attempted to shed light on whether there is a trade-off between public health and the economy as politicians and economists often say (Baker et al., Citation2020; Carrieri et al., Citation2021; McKee & Stuckler, Citation2020) or whether policymakers can secure a double dividend. To answer this question, we collected macroeconomic data for 49 countries, representative of the global population and economy and tested a comprehensive econometric model with GDP growth as criterion variable.
Concerning our first hypothesis, while we provide theoretical reasoning for expecting both a positive and negative relationship between policy stringency and GDP growth, our results clearly show that the relationship is negative in both models (one with direct effects only and one including the interaction effect of two policies). In other words, more stringent jurisdictions on average suffered greater economic contractions than less stringent jurisdictions. This result is consistent with the conventional wisdom that policy stringency is detrimental to economic output (Baker, Citation2020). Stringency leads to business restrictions and closures, thereby preventing products, services and money from freely circulating in the economy and between economies (König & Winkler, Citation2020). Although IT technology has enabled many sectors to operate relatively undisturbed or even to flourish online, such technology could not offset the fact that some important business sectors that rely on close human contact (i.e., tourism, hospitality, aviation, culture) came to almost a complete halt for long periods of time (Garzillo et al., 2022; Kartseva & Kuznetsova, Citation2020) and have still not recovered.
Concerning our second hypothesis, we did not detect a significant relationship between the economic support and GDP growth in the direct effects only model, but we did find a statistically significant negative effect in the model including the interaction effect. We provide theoretical reasoning for expecting both a positive and negative relationship, raising the possibility that these opposite effects cancel each other out in the direct effects only model. One rationale holds that social security transfers to people on furlough increase effective overall demand while financial aid to companies helps them to direct scarce resources to productive use. Taken collectively, fiscal and financial aid should be beneficial for economic output (International Monetary Fund, Citation2021c; König & Winkler, Citation2020). Yet, the competing rationale acknowledges that residents/citizens were unable to spend the funds they had received due to either the stringent restrictions or in the presence of long-term job and health uncertainties, making the economy worse off compared to the classic Keynesian alternatives of direct governmental spending. It appears that the latter rationale prevails when the interaction effect of two policies is taken into account.
The control variables also warrant some interpretation. Both macroeconomic indicators examined in this study, i.e., gross fixed capital formation and balance of payments, are not related to GDP growth. This means that governments of the sampled countries were unsuccessful in offsetting the negative effects of their policy stringency with either greater investment activity or greater transfers from abroad.
As concerns COVID infections and COVID deaths, we detect that both variables have a negative relationship with GDP growth. This may be attributed to several factors. First, high rates of infection and death led to more stringent policies which, in turn, led to lower output. Second, high rates of infection and death inevitably mean that patients/victims either temporarily/permanently cease to participate in the economic system as either workers or customers (Garzillo et al., 2022; Kartseva & Kuznetsova, Citation2020). Third, high rates of infection and death result in an imminent rise in public spending on health instead of directing budget expenditure to other areas with bigger economic multipliers. Next, fear of infection and death can lead to voluntary withdrawal from consumption, even if not imposed by the state.
The significant positive interaction effect of policy stringency and economic support suggests that a well-designed combination of both policies was able to some extent mitigate the negative impact of both policies working in isolation. Still, it should be acknowledged that the interaction effect size, albeit statistically significant, is very small compared to the sizes of direct negative effects of policy stringency and economic support.
Of high relevance for the interpretation of results, the stringency in our sample was largely responsive, oriented to preventing the collapse of health systems after infections had already become widespread, rather than protective, oriented towards saving human life by preventing the virus’ spread.
From a holistic perspective, our econometric model findings appear to lend support for the view that policymakers have faced an inevitable and delicate trade-off between protecting both human life and the economy, a common occurrence in policy making (Bajra & Čadež, Citation2019). In light of economic declines and generous transfers one might (wrongly) conclude that in this crisis Homo sapiens has opted for a social change (Cadez, Citation2013) from Homo economicus towards Homo solidarietatis.
We challenge however the inevitability of trade-off by evidence that some countries were able to successfully circumvent the trade-off path and appear to have secured a double dividend of public health and economic growth. Taiwan, China, Finland and South Korea are some examples of countries that not only managed to keep their GDP relatively stable in 2020 but their COVID-19 death rates low as well (Erokhin & Gao, Citation2020; Jeong et al., Citation2020; Lai et al., Citation2020). These countries have introduced stringent measures before infections started to soar as opposed to the dominant choice of pursuing stringency after the infections had already taken off. It appears fair to conclude that decision makers in these countries were successful in mitigating myopic biases inherent in human decision making (Ličen & Slapničar, Citation2022) by a prudent far-sighted stringency policy of preventing the virus outbreak. The finding that protective stringency is the preferred choice in terms of both protecting human life and maintaining a vibrant economy serves as a useful pointer for policy makers to achieve their goals in the future (Bajra & Čadež, Citation2018b).
The findings should be interpreted in light of this study’s limitations. The very short time since COVID-19 first appeared made it difficult to empirically test effects. The decision to use quarterly data was not because it is the best option but because not many other choices existed. Further, the measures of policy stringency and economic support were developed literally overnight and may suffer from limited validity and reliability. Third, since at the time of writing the pandemic is continuing, we might be somewhat biased in our own interpretations. Nevertheless, although we do not claim that this study is perfect, we believe that it is interesting and robust enough to help policymakers understand whether their stringency and economic support responses have yielded the desired balance in terms of both public health and the economy.
The study also offers interesting pointers for future inquiry. With the pandemic coming to an end, stringent measures are gradually being relaxed around the world and the economy is starting to recover. This raises the interesting question of whether the countries most devastated by the COVID-19 pandemic will also be the most prolific in the economic recovery or will continue to lag behind their more successful counterparts. We leave this interesting question for future inquiry.
Disclosure statement
No potential conflict of interest was reported by the authors.
Additional information
Funding
References
- Ahrens, T., & Ferry, L. (2020). Financial resilience of English local government in the aftermath of COVID-19. Journal of Public Budgeting, Accounting & Financial Management, 32(5), 813–823. https://doi.org/10.1108/JPBAFM-07-2020-0098
- Bajra, U., & Čadež, S. (2018a). The impact of corporate governance quality on earnings management: Evidence from European companies cross-listed in the US. Australian Accounting Review, 28(2), 152–166. https://doi.org/10.1111/auar.12176
- Bajra, U., & Čadež, S. (2018b). Audit committees and financial reporting quality: The 8th EU Company Law Directive perspective. Economic Systems, 42(1), 151–163. https://doi.org/10.1016/j.ecosys.2017.03.002
- Bajra, U., & Čadež, S. (2019). Alternative regulatory policies, compliance and corporate governance quality. Baltic Journal of Management, 15(1), 42–60. https://doi.org/10.1108/BJM-11-2018-0373
- Bajra, U. Q. (2021). The interactive effects of remittances on economic growth and inequality in western Balkan countries. Journal of Business Economics and Management, 22(3), 757–775. https://doi.org/10.3846/jbem.2021.14587
- Bajra, U. Q., Krasniqi, A., & Podvorica, A. (in press). Privatisation of socially owned enterprises, the methods used and the impact on economic growth: Empirical evidence from Kosovo. Economic Research-Ekonomska Istraživanja. https://doi.org/10.1080/1331677X.2021.1893201
- Baker, S. R., Farrokhnia, R. A., Meyer, S., Pagel, M., & Yannelis, C. (2020). How does household spending respond to an epidemic? consumption during the 2020 COVID-19 pandemic. The Review of Asset Pricing Studies, 10(4), 834–862. https://doi.org/10.1093/rapstu/raaa009
- BBVA Research. (2021). Spain Economic Outlook 2Q21. https://www.bbvaresearch.com/en/publicaciones/spain-economic-outlook-second-quarter-2021/
- Boratyńska, K. (2021). A new approach for risk of corporate bankruptcy assessment during the COVID-19 pandemic. Journal of Risk and Financial Management, 14(12), 590. https://doi.org/10.3390/jrfm14120590
- Bouri, E., Naeem, M. A., Nor, S. M., Mbarki, I., & Saeed, T. (2021). Government responses to COVID-19 and industry stock returns. Economic Research-Ekonomska Istraživanja, 1–24. https://doi.org/10.1080/1331677X.2021.1929374
- Cadez, S. (2013). Social change, institutional pressures and knowledge creation: A bibliometric analysis. Expert Systems with Applications, 40, 6885–6893. https://doi.org/10.1016/j.eswa.2013.06.036
- Cadez, S., Czerny, A., & Letmathe, P. (2019). Stakeholder pressures and corporate climate change mitigation strategies. Business Strategy and the Environment, 28(1), 1–14. https://doi.org/10.1002/bse.2070
- Carrieri, V., De Paola, M., & Gioia, F. (2021). The health-economy trade-off during the COVID-19 pandemic: Communication matters. PLoS One, 16(9), e0256103. https://doi.org/10.1371/journal.pone.0256103
- Charlton, D., & Castillo, M. (2022). Potential impacts of a pandemic on the US farm labor market. Applied Economic Perspectives and Policy, 43(1), 39–57. https://doi.org/10.1002/aepp.13105
- Clark, A. E., & Lepinteur, A. (2021). Pandemic Policy and Life Satisfaction in Europe. Review of Income and Wealth, 1–16. https://doi.org/10.1111/roiw.12554
- Coccia, M. (2021). The relation between length of lockdown, numbers of infected people and deaths of COVID-19, and economic growth of countries: Lessons learned to cope with future pandemics similar to COVID-19 and to constrain the deterioration of economic system. Science of the Total Environment, 775, 145801. https://doi.org/10.1016/j.scitotenv.2021.145801
- Erokhin, V., & Gao, T. (2020). Impacts of COVID-19 on trade and economic aspects of food security: Evidence from 45 developing countries. International Journal of Environmental Research and Public Health, 17(16), 5775–5728. https://doi.org/10.3390/ijerph17165775
- European Investment Bank. (2021). European Investment Bank Investment Report: Building a smart and green Europe in the COVID-19 era. https://doi.org/10.2867/904099
- Feng, G.-F., Yang, H.-C., Gong, Q., & Chang, C.-P. (2021). What is the exchange rate volatility response to COVID-19 and government interventions? Economic Analysis and Policy, 69, 705–719. https://doi.org/10.1016/j.eap.2021.01.018
- Fetzer, T., Hensel, L., Hermle, J., & Roth, C. (2021). Coronavirus perceptions and economic anxiety. The Review of Economics and Statistics, 103(5), 968–978. https://doi.org/10.1162/rest_a_00946
- Garzillo, E. M., Monaco, M. G. L., Spacone, A., Inglese, E., Lamberti, M., & Pompei, D. (2022). SARS-CoV-2 emergency in the workplace: Are companies ready to protect their workers? A cross-sectional survey. International Journal of Occupational Safety and Ergonomics, 28(2), 683–690. https://doi.org/10.1080/10803548.2020.1810457
- Goel, R. K., Saunoris, J. W., & Goel, S. S. (2021). Supply chain performance and economic growth: The impact of COVID-19 disruptions. Journal of Policy Modeling, 43(2), 298–316. https://doi.org/10.1016/j.jpolmod.2021.01.003
- González-Bustamante, B. (2021). Evolution and early government responses to COVID-19 in South America. World Development, 137, 105180. https://doi.org/10.1016/j.worlddev.2020.105180
- Gordon, D. V., Grafton, R. Q., & Steinshamn, S. I. (2021). Cross-country effects and policy responses to COVID-19 in 2020: The Nordic countries. Economic Analysis and Policy, 71, 198–210. https://doi.org/10.1016/j.eap.2021.04.015
- Hale, T., Petherick, A., Phillips, T., Webster, S., & Kira, B. (2020). Oxford COVID-19 Government Response Tracker, Blavatnik School of Government. Working Paper. www.bsg.ox.ac.uk/covidtracker
- Havrlant, D., Darandary, A., & Muhsen, A. (2021). Early estimates of the impact of the COVID-19 pandemic on GDP: A case study of Saudi Arabia. Applied Economics, 53(12), 1317–1325. https://doi.org/10.1080/00036846.2020.1828809
- Hotle, S., & Mumbower, S. (2021). The impact of COVID-19 on domestic U.S. air travel operations and commercial airport service. Transportation Research Interdisciplinary Perspectives, 9, 100277. https://doi.org/10.1016/j.trip.2020.100277
- Hu, J., Yue, X.-G., Teresiene, D., & Ullah, I. (2021). How COVID19 pandemic affect film and drama industry in China: An evidence of nonlinear empirical analysis. Economic Research-Ekonomska Istraživanja, 1–19. https://doi.org/10.1080/1331677X.2021.1937262
- Hu, S., & Zhang, Y. (2021). COVID-19 pandemic and firm performance: Cross-country evidence. International Review of Economics & Finance, 74, 365–372. https://doi.org/10.1016/j.iref.2021.03.016
- Hulpke, J. F., & Fronmueller, M. P. (2021). What’s not to like about evidence-based management: A hyper-rational fad? International Journal of Organizational Analysis, 30(7), 95–123. https://doi.org/10.1108/IJOA-06-2020-2278
- International Monetary Fund. (2021a). Policy Tracker. https://www.imf.org/en/Topics/imf-and-covid19/Policy-Responses-to-COVID-19
- International Monetary Fund. (2021b). Global financial stability report: Preempting a legacy of vulnerabilities. SSRN Electronic Journal. https://www.imf.org/en/Publications/GFSR/Issues/2021/04/06/global-financial-stability-report-april-2021
- International Monetary Fund. (2021c). World economic outlook: Managing divergent recoveries (Issue April). International Monetary Fund, Publication Services. https://www.imf.org/en/Publications/WEO/Issues/2021/03/23/world-economic-outlook-april-2021
- International Monetary Fund. (2021d). World economy outlook. In International Monetary Fund. (Issue April). International Monetary Fund, Publication Services. https://www.imf.org/en/Publications/WEO/Issues/2021/03/23/world-economic-outlook-april-2021
- Jeong, E., Hagose, M., Jung, H., Ki, M., & Flahault, A. (2020). Understanding South Korea’s response to the COVID-19 outbreak: A real-time analysis. International Journal of Environmental Research and Public Health, 17(24), 9571–9518. https://doi.org/10.3390/ijerph17249571
- Jiang, B., Gu, D., Sadiq, R., Mohsan Khan, T., & Chang, H.-L. (2021). Does the stringency of government interventions for COVID19 reduce the negative impact on market growth? Evidence from Pacific and South Asia. Economic Research-Ekonomska Istraživanja, 1–19. https://doi.org/10.1080/1331677X.2021.1934058
- Jones, B. A., Sergejeff, K., Sherriff, A., Teevan, C., & Veron, P. (2020). The challenge of scaling up the European Union’s global response to COVID-19. In ECDPM Making Policies Work (Issue Briefing Note No.116). www.ecdpm.org/bn116
- Jordà, Ò., Singh, S. R., & Taylor, A. M. (2022). Longer-run economic consequences of pandemics. The Review of Economics and Statistics, 104(1), 166–175. https://doi.org/10.1162/rest_a_01042
- Kaplan, S., Lefler, J., & Zilberman, D. (2022). The political economy of COVID-19. Applied Economic Perspectives and Policy, 44(1), 477–488. https://doi.org/10.1002/aepp.13164
- Kartseva, M. A., & Kuznetsova, P. O. (2020). The economic consequences of the coronavirus pandemic: Which groups will suffer more in terms of loss of employment and income? Population and Economics, 4(2), 26–33. https://doi.org/10.3897/popecon.4.e53194
- König, M., & Winkler, A. (2020). COVID-19 and economic growth: Does good government performance pay off? Inter Economics, 55(4), 224–231. https://doi.org/10.1007/s10272-020-0906-0
- König, M., & Winkler, A. (2021). COVID-19: Lockdowns, fatality rates and GDP growth: Evidence for the first three quarters of 2020. Inter Economics, 56(1), 32–39. https://doi.org/10.1007/s10272-021-0948-y
- Lai, C. C., Wang, C. Y., Wang, Y. H., Hsueh, S. C., Ko, W. C., & Hsueh, P. R. (2020). Global epidemiology of coronavirus disease 2019 (COVID-19): Disease incidence, daily cumulative index, mortality, and their association with country healthcare resources and economic status. International Journal of Antimicrobial Agents, 55(4), 105946. https://doi.org/10.1016/j.ijantimicag.2020.105946
- Ličen, M., & Slapničar, S. (2022). Can process accountability mitigate myopic biases? An experimental analysis. Journal of Management Control, 33(1), 1–26. https://doi.org/10.1007/s00187-021-00330-7
- Marinč, M., Massoud, N., Ichev, R., & Valentinčič, A. (2021). Presidential candidates linguistic tone: The impact on the financial markets. Economics Letters, 204, 109876. https://doi.org/10.1016/j.econlet.2021.109876
- McKee, M., & Stuckler, D. (2020). If the world fails to protect the economy, COVID-19 will damage health not just now but also in the future. Nature Medicine, 26(5), 640–642. https://doi.org/10.1038/s41591-020-0863-y
- National Statistics Bureau Taiwan. (2021). News Release. https://eng.stat.gov.tw/point.asp?index=1
- OECD. (2021). Quarterly national accounts. OECD National Accounts Statistics (Database). https://doi.org/10.1787/data-00017-en
- Pan, K., & Yue, X.-G. (2021). Multidimensional effect of COVID-19 on the economy: Evidence from survey data. Economic Research-Ekonomska Istraživanja, 1–28. https://doi.org/10.1080/1331677X.2021.1903333
- Pappas, N. (2021). COVID19: Holiday intentions during a pandemic. Tourism Management, 84, 104287. https://doi.org/10.1016/j.tourman.2021.104287
- Rant, V., Marinč, M., & Porenta, J. (2021). Debt and convergence: Evidence from the EU member states. Finance Research Letters, 39, 101617. https://doi.org/10.1016/j.frl.2020.101617
- Spash, C. L. (2021). The economy’ as if people mattered: Revisiting critiques of economic growth in a time of crisis. Globalizations, 18(7), 1087–1104. https://doi.org/10.1080/14747731.2020.1761612
- Su, C.-W., Dai, K., Ullah, S., & Andlib, Z. (2021). COVID-19 pandemic and unemployment dynamics in European economies. Economic Research-Ekonomska Istraživanja, 1–13. https://doi.org/10.1080/1331677X.2021.1912627
- Tartavulea, C. V., Albu, C. N., Albu, N., Dieaconescu, R. I., & Petre, S. (2020). Online teaching practices and the effectiveness of the educational process in the wake of the Covid-19 pandemic. Amfiteatru Economic, 22(55), 920–936. https://doi.org/10.24818/EA/2020/55/920
- Traskevich, A., & Fontanari, M. (2021). Tourism potentials in post-COVID19: The concept of destination resilience for advanced sustainable management in tourism. Tourism Planning & Development, 1–25. https://doi.org/10.1080/21568316.2021.1894599
- Verma, P., Dumka, A., Bhardwaj, A., Ashok, A., Kestwal, M. C., & Kumar, P. (2021). A statistical analysis of impact of COVID19 on the global economy and stock index returns. SN Computer Science, 2(1), 27. https://doi.org/10.1007/s42979-020-00410-w
- Wilkins, P., Gilchrist, D., & Phillimore, J. (2021). Independent review of emergency economic stimulus measures: Global Financial Crisis and COVID-19. Australian Journal of Public Administration, 80(1), 12–28. https://doi.org/10.1111/1467-8500.12437