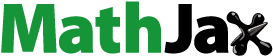
Abstract
Although inflation expectations are pivotal variables for central banks, they are not directly observable. Therefore, central banks use qualitative survey results to proxy consumer expectations, and their quantification in this manner is often criticized. In this study, we investigate and identify an optimal quantification procedure for survey results based on a set of regression and probabilistic models. Specifically, we seek to identify the method that returns time series that are most highly correlated with an unbiased representative of survey-based expectation: balance statistics. We place additional constraints on this criterion to identify the procedure that returns expectations that are most closely related to consumer intentions (directional co-movements and forecast accuracy). Our sample covers the European Union member states over the period of January 2002 to June 2019. We also test a post-crisis subsample. Our results suggest that different procedures may be optimal for different economies, in line with previous findings on cross-country divergences of expectations formation. However, we find that the most applied assumption of normal distribution does not prove to be the best one. Our recommendation is to apply probabilistic procedures rather than regression methods.
1. Introduction
This paper search for an individualized, country-level procedure for consumer inflation expectations quantification that closely reflects consumer intentions expressed in qualitative surveys. We identify this procedure due to the novel optimization path we propose.
Expectations are subjectively held beliefs by individuals about uncertain future economic outcomes (Pesaran & Weale, Citation2006) and are heuristic-based rather than model-based. It is indicated that expectations are the key variable from the point of view of price stability, which is the priority of monetary policy (Woodford, Citation2003). Thus, modern monetary policy should focus on expectations, and central banks need their accurate proxy. The approximation of inflation expectations and their use in monetary policy is an important problem for academics and policymakers.
Consumer inflation expectations are examined through qualitative surveys. The most applied methods of quantification (used to transformed qualitative responses into numbers) are probabilistic methods and regression methods (Berk, Citation1999; Carlson & Parkin, Citation1975; Pesaran, Citation1984). All the methods used have some advantages and drawbacks. Our investigation is motivated by the drawbacks of existing approaches, which leave room for arbitrary choice of the quantification procedure.
In this work, we offer a comparative study of several existing quantification methods to identify the most suitable one for each economy and offer three novel criteria to detect an optimal, individualized method of quantification. We propose the path to identify the optimal quantification procedure. Three evaluation criteria are provided, each of which is characterized by different assumptions regarding the quality of the applied method of quantifying inflation expectations. For all three evaluation criteria, we made the priority assumption of maximization the correlation between raw expression of expectation—the balance statistics of survey responses—and quantified expectation. The first evaluation criterion considers only correlation. The second evaluation criterion consists of two optimization assumptions that consider the strongest correlation and the best directional co-movement of expectations and balance statistics. The third evaluation criterion is about the correlation between expectations and balance statistics and minimization of forecast errors. We find this choice of criteria a novelty as the majority of other studies discuss the best proxy of expectations in terms of the most accurate one only. In our opinion, this is not applicable to consumer expectations, what we explain in Data and Methods section.
Our optimization procedure provides no remedy for econometrical doubts we can have against any of the quantification methods. What we offer (the novelty of this study) is an optimization path that returns for a country dataset the optimal procedure identified according to a set of optimization criteria. The procedure could be applied for economies besides our sample. Our point of view for this paper is that each quantification procedure has drawbacks; we can identify the one that promises the best representation of raw responses (qualitative) regardless of the drawbacks of a particular method.
Our sample covers 19 member states of the European Union: ten economies that adopted the euro when the currency union had been launched (including Greece) and nine economies that have not adopted euro yet,Footnote1 from the 2002–mid-2019 period. We analyze the post-crisis arrival subsample (2010–mid-2019) to investigate whether the optimal procedure changed after the 2008 global financial crisis. If it did, the examination results would be in line with the majority of studies confirming a post-crisis change in consumer expectations properties: their forward-lookingness (Łyziak & Mackiewicz-Łyziak, Citation2014; Szyszko, Rutkowska, & Kliber, Citation2020) or reaction to monetary policy shocks (Andreou, Eminidou, & Zachariadis Citation2016). However, we do not claim that the crisis is the (only) reason for this change, as our methodology does not allow for such conclusions. This study rather only considers the crisis as a good turning point for economic studies.
The study contributes to the literature on inflation expectations as it furthers the discussion on how to quantify expectation and why “the most commonly applied” method is not always the best option. The novelty is also related to our optimization path and criteria that are not about the most accurate forecast. The empirical examination carried out allowed us to conclude why some methods do not work and are merely a methodological possibility for empirical investigations.
The paper is organized as follows: the next section briefly characterises expectations and their approximation options, the third section presents existing procedures of quantification and their critics; the fourth section describes the data and methods of the study. Section four includes the results and analysis. Finally, we discuss our results and conclude.
2. Related literature
Inflation expectations are pivotal variable from monetary policy point of view. Mainstream hypotheses explaining their formation indicated milestones of monetary theories starting with adaptive expectations (Cagan, Citation1956) that supported monetarism to rational expectations (Muth, Citation1961) introduced to macroeconomics by Lucas (Citation1972). The obvious disadvantages of the latter, that dominates in macroeconomics for over fifty years, initiated the intensive search for more realistic models of expectations formation. In the nineties of the 20th century bounded rationality hypotheses were proposed with their multiple variants of adaptive learning (Sargent, Citation1993), sticky information (Mankiw & Reis, Citation2002), and epidemiological model (Carroll, Citation2003). Due to the limited length of this paper, we refrain from their detailed presentation. Nowadays the majority of central banks consider the rational expectations model as the most relevant one; however, hybrid specifications and bounded rationality models are also applied to macro modelling.
Regardless of the theoretical frameworks that contribute to mainstream economics, expectations are not directly observable variable. The question on their approximation, that we aim to respond, arises. Prior to discussing quantification of consumer expectations derived from qualitative surveys, we present existing options to approximate expectations. The existing literature distinguishes two main groups of methods to derive expectations (Pesaran & Weale, Citation2006): indirect (model-based) and direct (survey-based). First, model based or theoretical approach uses macro models and identifies the way how expectations are formulated being the immanent part of the story of the economy’s functioning. Then, the results are juxtaposed with one of the theoretical models of expectations formation and verified (Łyziak & Stanisławska, Citation2006). Direct methods apply surveys held among different groups of economic agents: professionals, enterprises, and consumers. If prepared for professionals and business, the survey questions are expressed quantitatively, therefore there is no discussion about the optimal quantification procedure. Survey-based expectations of consumers and their transformation from qualitative to quantitative proxy are in the scope of this study.
Alternatively to direct and indirect methods, expectations could be derived from financial markets yields. The term structure of yields reveals expected inflation by market participants for different horizons. Term structure models could include linear, non-linear, and arbitrage-free specifications (Ang, Bekaert, & Wei, Citation2007). The market proxies of expectations could be used for economies with developed financial markets.
Finally, expanding body of the literature presents the experimental exercises as the method to derive expectations (Cornand & Heinemann, Citation2018; Cornand & Hubert, Citation2020). Due to small sampling, this approach does not return results for generalization, however, it is a powerful tool of examining expectations proprieties.
All the existing methods have advantages and disadvantages. Due to the limited length of this paper, we could not discuss them in detail. We decided to study survey-based method as this one is commonly used, proves to return the most accurate results for some groups of market participants (Ang et al., Citation2007) and creates the additional problem with expectations quantification that is of our interest in this study.
3. Methods
3.1. Existing quantification methods
Qualitative surveys that are designed to assess economic agents’ sentiments are broadly applied to proxy consumer expectations. As it was mentioned in the previous section, surveys are relatively good sources of expectations, compared to other methods. They are conducted by national entities and, in the case of the EU member states, under the auspices of the European Commission by the Directorate-General for Economic and Financial Affairs. The Business and Consumers Surveys (BCS) are regular, harmonized surveys, conducted monthly, which we use in this paper due to the methodological identity for countries covered. However, the quantification methods that we refer to in this paper could be applied to any survey designed in an analogous way. A similar method of analysis of consumer expectations is applied in numerous countries beyond the EU.
The BCS contains two relevant questions once we quantify expectations (European Commission, Citation2020), each of them offering five options ranging from the most positive to the most negative and “do not know” options:
Question about the inflation perception (Q5): “How do you think that consumer prices have developed over the last 12 months? They have…: risen a lot, risen moderately, risen slightly, stayed about the same, fallen, don't know.”
Question about the expected change of the prices (Q6): “By comparison with the past 12 months, how do you expect that consumer prices will develop in the next 12 months? They will….: increase more rapidly, increase at the same rate, increase at a slower rate, stay about the same, fall, don’t know.”
The Business and Consumers Surveys are conducted monthly in the EU member states and official candidate states. Aggregate level results—the percentage of responses to the survey’s questions—are published and could be used to obtain a direct, quantitative proxy of inflation expectations. Usually, such a proxy is needed even if a few analyses based directly on survey responses exist (Acedański & Włodarczyk Citation2016; Claveria, Citation2021). The authors’ decision on “to quantify or not” depends on the goal of the study. If quantification could be avoided, balance statistics are considered to be enough as a raw representation of expectations.
There are two acknowledged and commonly applied types of methods for quantifying expectations: probabilistic methods and regression methods (). However, neither of them avoids the criticisms related to their setup and reliability. We present shortly the main roots of criticism related to each of them. Our proposal is not a remedy for all of them; it is rather a procedure that suggests which method is found to be optimal for a given economy. Nonetheless, these methods and their extensions are widely used to quantify expectations by scholars and policymakers. We avoid giving detailed description of each of them as they are presented elsewhere (see Berk, Citation1999; Łyziak, Citation2010).
Table 1. Expectation quantification methods.
The methods described above are the most used ones to quantify consumer expectations. However, their applicability and acceptance are accompanied by strong criticism and a search for an alternative. Objections have both: economic and econometric roots. Economically-rooted objections are about the insufficient reliability of the results in the light of modern economic development. The example could be an empirical study applying the probabilistic approach to stock market expectations, suggesting that quantified expectations do not correlate with the results of quantitative surveys (Breitung & Schmeling, Citation2013). Similar results were found for the University of Michigan’s Survey of Consumers – the survey provides both qualitative and quantitative results (Lahiri & Zhao, Citation2015). Due to the absence of quantitative surveys conducted for consumers (results are not published), this argumentation cannot be verified for our dataset. The other study suggests that under the price stability condition, agents perceive “moderate” inflation through the inflation targeting policy. Thus, a more realistic version of the probability method would be assumption-free and acknowledge that consumers are governed by the official central bank inflation targeting policy in discriminating between moderate and extreme inflation tendencies (Lolić & Sorić, Citation2018).
A caveat regarding the shortcomings of surveys as a source of data about expectations should also be noted, as doubts often occur about the correlation of respondents’ answers and actual behaviour. Some studies confirm that they are correlated (Armantier et al., Citation2015). However, survey questions may be imprecise, perceived emotional, and their ordering may affect the responses. The last fact means that the responses would depend on the context of questionnaire presentation (framing effect) (Berk, Citation1999). Moreover, the questionnaire structure should be stable over time and at the same time, should flexibly adjust to the situation. The deflation period is the best example of the mismatch of survey questions, which focus mostly on a general increase in the price level. Despite these objections toward surveys, we find them the most useful tool for examining consumer expectations.
3.2. Proposed optimization procedure
The optimization procedure that we developed to identify the quantification method tailored to replicate to a large extent consumers’ actual expectations covers four steps:
Determining the set of potential methods of quantifying inflation expectations.
Quantification of expectations according to chosen methods.
Introduction and application of evaluation criteria.
Selecting the optimal quantification method or set of methods based on evaluation criteria.
The applicability of the proposed procedure is tested on an empirical example of inflation expectations for 19 EU member states in the years 2002–2019: Austria, Belgium, Germany, Spain, Finland, France, Greece, Italy, Netherlands, and Portugal, and nine non-Euro economies: Bulgaria, Croatia, Czechia, Denmark, Hungary, Poland, Romania, Sweden, and the UK.Footnote2 The sample covers the 2002–mid-2019 period and we also distinguish a subsample for the 2010–mid-2019 period. To quantify expectations, we used Business and Consumers Surveys releases on a percentage of responses given on perceived inflation (Q5) and expected price change (Q6) questions as well as the sets of scaling factors: actual inflation, food prices, fuel prices, and energy prices and own indices, being their averages, as alternative versions. Inflation and its subindices are derived from Eurostat. The data used in the study could be found on the open data repository (see Rutkowska et al., 2022).
In compliance with step 1 of our optimization procedure, we adopted the preliminary set of methods of quantification, consisting of six regression methods and 35 probabilistic methods for different scaling factors and distributions. We applied six regression models described: Anderson (Citation1952), Pesaran (Citation1984), Smith and McAleer (Citation1995), and Cunningham (Citation1997). Two models by Anderson were estimated: his primary proposal and its narrow version that exploits only balance statistics. Further, the other models are based on the Anderson’s. Smith and McAleer (Citation1995) provided a two-model setup including a time-varying parameters model. Equations of estimated models are presented in . Our starting point for the choice of an estimator was OLS. The OLS estimator is consistent when the regressors are exogenous, and is optimal in the class of linear unbiased estimators when the errors are homoscedastic and serially uncorrelated. Post-estimation diagnostics covered standard tests for linear or non-linear models. The logic that underlies the regression methods of quantification is the same, regardless of the version of the model applied as summarized in . To derive the expectation proxy, the dataset from both survey questions (Q5 for parameters estimation and Q6 for calculating the expected inflation rate) are used.
Table 2. Regression methods applied.
The probabilistic methods that we applied are based on the canonical work of Carlson and Parkin (Citation1975). We used Batchelor and Orr's (Citation1988) model extension that adjusts the original procedure to a polychotomous survey structure (five-question survey). The probabilistic method offers the possibility to explore various assumptions about the scaling factor and distributions. Firstly, we distinguish subjectified and objectified versions of quantification. Subjectified quantification is a two-step procedure: the structure of answers to Q5 and a representation of “normal” inflation (we used the 36 M moving average of inflation) are used for the quantified perceived inflation rate. The divergence between inflation perception and the official inflation statistics is well documented in the economics literature (Hałka & Łyziak, Citation2015; Stanisławska, Citation2019). This is why, when seeking to identify a scaling factor, the value consumers perceive as the inflation rate could be applied. Quantified perception of inflation is a scaling factor used to identify an expectations proxy together with Q6 answers. The objectified version of quantification relies only on official inflation statistics and the structure of Q6 responses. However, there is room for the adjustment of a scaling factor. In this analysis, we used six scaling factors:
Official statistics on inflation rate (i); we used 2M-lagged inflation as surveys precede announcements of the latest inflation figure and consumers, as the least qualified economic agents, require time to process economic news.
2M-lagged: food prices inflation (ii), electricity prices (iii) and fuel prices change (iv); they represent the highest weights in the consumption basket and are front-page news when they increase; we consider each of them separately.
An average evolution of food, energy, and fuel prices (v); we use also a weighted averaged (vi) of these three components using the approximation of weight provided by Hałka and Łyziak (Citation2015).
Finally, when applying the probabilistic method, we have to choose the distribution of expectations. The most common choice here is quite standard: normal distribution prevails.Footnote3 It is also possible to use alternative distributions to the normal distribution. We applied uniform, logistic, Student’s t and skewed Student’s t distributions. lists all versions of the probabilistic approach that we used.
Table 3. Probabilistic methods applied.
Valuation criteria adoption (step 3 of this procedure) allows for the selection of the optimal method of quantifying consumer expectations for each economy. We propose three separate criteria applicable for different conditions, depending on the goal of the study that could apply quantified time series (see ). For each of them, our intention was to focus mostly on the dependence between expectations and a raw representation of survey results, biased neither by the choice of quantification method nor by the assumption about the scaling factor or distribution. Such a raw representation of results is expressed in terms of balance statistics (BS):
(1)
(1)
where PP represents the percentage of respondents who chose the “most positive” answer (e.g., for Q5: “prices have risen a lot” and for Q6: “prices will increase more rapidly”); P is the percentage of positive options; M stands for the percentage of respondents who chose the negative option; and MM represents the percentage of “most negative” answer.
Table 4. Evaluation criteria setup.
In our opinion the novelty of this examination lies in the selected evaluation criteria for the optimal quantification procedure. We avoid prioritizing the commonly applied criterion of expectation accuracy as the most suitable method because this approach has little to do with consumers’ capabilities to express their expectations. It is quite common for consumers to have a low degree of economic knowledge and limited awareness of ongoing and future situations. Thus, even central banks refrain from analysing consumer inflation expectations quantitatively as consumers are simply not ready to give sound answers if the survey questions are too difficult. Quantitative survey results are volatile, register high dispersion, and have nothing in common with the actual economic situation. Consumers are entitled to be wrong in their opinions and estimates. If increased accuracy of expectation in a consumer survey is related to the method applied, this does not necessarily reflect what consumers mean. This is confirmed by the results of previous studies that document consumers’ inability to take official inflation statistics for what they are (Stanisławska, Citation2019). Forecasting inflation is a much more challenging task. Previous papers exhibit a lower degree of forward-lookingness in consumer expectations than in that of professionals (Gerberding, Citation2001). According to Ranyard et al. (Citation2008), consumers have limited ability to store and recall prices and their perceptions and expectations of price changes diverge from what is observed. However, they do seem to have some instinct for, and ability to judge and forecast, inflation. Thus, the assumption that more accurate expectations represent actual expectations expressed by consumers is misleading.
Moreover, our justification for not relying on the “most accurate forecasts” criterion is also related to the application of time series with quantified proxies of expectations. Once the expectations are tested for their rationality, the most accurate forecast will more probably show unbiasedness and, if not fully rational, more forward-lookingness. Considering this, studies on expectations properties should certainly not be affected by a priori choices that give a handicap to some quantification procedures. This is why indirect methods of quantifying expectations that we shortly presented in Section Related Literature are not overcoming the other options.
Following the approach that does not prioritize expectations accuracy, the first evaluation criterion assumes the selection of the optimal method only on the basis of the Pearson correlation coefficient between expectations and balance statistics BS (see ).
We acknowledge that this first valuation criterion could be extended. Therefore, the second valuation criterion also considers the directional co-movement of expectations and BS. This is because the same degree of linear correlation may occur for different degrees of alignment of time series over time. In the second criterion, apart from correlation, we also take into account the directional co-movements coefficient (DC) of expectations and the balance statistics. It is based on signs’ accordance for both variables. Thus, we check and count the number of cases for which the first differences of the balance and expectation, calculated with different methods, have the same signs:
(2)
(2)
where
represents the i-th first differences of inflation expectation,
is the i-th first differences of balance statistics, sign is the signum function, and n refers to number of observations. The values of DC statistics vary from −1 to 1, where a value closer to 1 is preferred because detects the more directional co-movement of variables.
In the third evaluation criterion, the assumption regarding forecasts errors is additionally taken into account – here, the accuracy of expectations (see ). Although we aim to prioritize correlations, we would like to compromise with existing literature and consider forecast errors as well. This means that in the third criterion, in addition to correlation, we also take into account forecast accuracy measured using Theil’s coefficient of inequality (U) (EquationEq. 3(3)
(3) ). Theil’s coefficient was applied to indicate the forecast accuracy of the outcomes of the quantification procedures. It proxies the error of the forecast, where it is expressed as a share of the deviations of forecast the series from their realization (Bliemel, Citation1973). Theil’s coefficient of inequality values varies from zero to one. The closer the value is to zero, the better is the accuracy of forecasts returned by a quantification procedure.
(3)
(3)
where:
is the inflation realization,
represents the inflation expectation, and n stands for the number of observations.
The evaluation criteria adopted in step 3 allow for the selection of the optimal methods for each economy separately. While in the case of the first evaluation criteria it is possible to unequivocally choose one optimal method with the highest value of the correlation coefficient, then in the case of the second and third criteria, the response is more complicated. The set of quantification methods for which the value of the correlation coefficient is in the assumed percentile are considered optimal.Footnote4 This is due to the fact that the above-mentioned evaluation criteria take into account two assumptions at the same time. For both assumptions, arbitrarily appropriate percentile values are assumed, and the method selects all that have reached the appropriate percentile values for both assumptions. Narrowing and broadening percentiles might lead to the preferred method of quantification.
The advantage of our optimization procedure arises from the option of filtering from the set of potential methods of quantification only the methods meeting certain criteria. Otherwise, the choice of a specific method of quantifying expectations is arbitrarily carried out by academics or policymaker. The decision is based on the specificity of the research problem.
Regardless of the procedure, we seek an individualized procedure for each economy. Thus, the optimal results might be captured for different degrees of correlation and different percentile sets for each procedure and economy. We always aim at detecting the narrowest percentile that meets our conditions for the two-criterion procedure.
4. Results and discussion
We obtained quantified proxies of expectations for 41 versions, 19 economies, and 2 sample time spans. Full quantification results could be found in open data repository (Rutkowska et al., Citation2022). While commenting on the results, we refer to the regression methods first, where estimations were obtained for 190 equations. To a large extent, our results explain why the majority of authors prefer probabilistic methods of quantification: The estimation results from regression methods could be easily challenged. A large portion of equations (38% of total estimation, 36% for euro subsample and 42% for non-euro economies) does not have statistically significant parameters. The removal of a statistically insignificant variable does not improve the result, it only undermines the economic interpretation of this particular approach. Additionally, in most of our models, the model assumption applicable for estimators are not satisfied: our models fail to meet post-estimations diagnostic tests. Regression methods require individualized econometric procedures for each case depending on the time series properties, and the model and estimator applied. The application of an alternative estimator does not guarantee success but makes the models less comparable.
Additionally, we observe the disadvantage of regression methods from more of an economic rather than an econometric source. Regression models have developed over the years, starting with the simplest, that is, Anderson’s proposal (adjusted for three options of responses: positive, neutral, and negative), to more closely reflect the economic reality of the dataset and consumer behaviour. We can easily identify the shortcomings of the original proposal and its early inspirations, including the assumption about the constant perception of price dynamics. Nevertheless, in our estimation, the simplest models prove to have the best econometric properties, and we are not able to compromise between an economic and econometric approach. The simplest models are closely linked to the balance statistics of survey responses. It reduces unfortunately their applicability to our set up of evaluation criteria.
Our conclusions on regression methods confirm the results of other studies - regression methods are mostly applied by papers that discuss the methodology of quantification in a comparative manner (for examples see (Berk, Citation1999; Łyziak, Citation2010)). Therefore we decide to perform optimization with probabilistic procedures only. The application of regression methods is a possible alternative to the probabilistic approach as it does not require any assumption about the distribution of expected inflation. However, the applicability is limited due to other econometrical limitations, as discussed above.
The second group of methods that we test, the probabilistic approach, requires two decisions: an assumption on the distribution of expected inflation and on the scaling factor that consumers refer to while responding to survey questions. For each economy and the two research periods, we ran 35 quantification procedures and analyzed the results with three valuation methods. Based on each of the evaluation criteria, we chose the optimal methods for each economy individually, where our results are presented in . In order to maintain the readability of the presented results, for each of the criteria one optimal method was presented, which had the highest percentiles values for the assumptions made. For ful quantification results and our statistical analyses see supplementary material and Rutkowska et al. (Citation2022).
Table 5. The first evaluation criterion: a summary of results.
Table 6. The second evaluation criterion: a summary of results.
Table 7. The third evaluation criterion: a summary of results.
Our first evaluation criterion indicates the methods that returns the strongest correlation between quantified expectations and raw balance statistics, where a summary of results is presented in . The first observation is that expectations proxies obtained under the assumption of normal distribution do not prove to be the most correlated with BS for any case. None of the remaining distributions dominates in terms of the number of cases with the strongest correlation. Similarly, the choice of the scaling factor also does not show evidence of a particular one dominating. However, the promising observation is about consumers’ perception of inflation. The results suggest that consumers do not refer to one-factor price changes (like food prices, energy prices, or fuel prices).Footnote5 CPI inflation is referred to, or an average/weighted average of food, fuel, and energy price change. As the majority of observations register the strongest correlation of BS with the averaged or weighted-average index change of price change for these latter three components, we may surmise that consumers do not necessarily perceive official inflation measure as their reference value.
Based on the results of the second evaluation criterion, we identified cases of methods that prove to jointly have the strongest correlation of expectations and BS and the strongest co-movements of these time series (see ). Our intuition for this criterion was that the same Pearson’s correlation could be found for cases with different alignments over time, even with periodical desynchronization. A few economies display high alignments, which simply means that we found the optimal results within high percentiles. Moreover, when the second criterion is added to the examination, the shortened sample results diverge from the full sample results. The post-crisis arrival period was more turbulent in terms of economic shock than the beginning of our research period, a few economies experienced deflation or extraordinary low inflation, and unconditional economic policy measures were applied. Thus, the rationale for desynchronization exists.
Again, normal distribution does not prevail. Second evaluation criterion indicated a greater importance for subjectified procedures. The detection of food prices as a scaling factor for three developed economies is an unexpected result, especially for the UK. Greek and Italian consumers underwent more severe economic crises and could refer to food prices more when adjusting their price expectations.
The discussion on the third evaluation criterion could begin with the observation that normal distribution is more significant in the case of the results obtained if we allow for forecast errors to affect the choice (for summary of the results see ). The other conclusions are similar as for the first (correlations only) evaluation criterion. None of the distributions or scaling factors seems to prevail for the majority of these cases. The number of established methods that indicate the same method as an optimal one for the full sample and shortened subsample is lower than in the first evaluation criterion. This could be due to increased risk uncertainty after the Great Recession, which could lead to less accurate forecasts.
A comparison of the methods indicated by the three evaluation criteria suggests that there are three quantification methods that outperform the others in terms of due to the adopted assumptions: probabilistic methods based on uniform, logistic, and skewed Student’s-t distributions for lagged inflation as a scaling factor. Note that logistic and Student’s-t distributions resemble the normal distribution in shape but have heavier tails, indicating higher kurtosis, whereas the skewed Student’s-t distribution incorporates a skewness parameter that considers the 12-month average actual inflation. Each of them is found to be the most relevant ca. 20 times (for different economies and time spans). This is about half of our cases, for a total of 112 (with the exclusion of Denmark for the shortened subsample). These methods could be an alternative for the standard normal distribution assumption of the probabilistic method.
The set of recommendation regarding the application of our procedure finishes the results discussion. Firstly, the obtained results undermine the most applied solution in other studies: the primary choice for quantification is the standard probabilistic method for normal distribution in subjectified or objectified versions (for examples of the most common practice see (Łyziak & Mackiewicz-Łyziak, Citation2014; Szyszko et al., Citation2020). Actual inflation is commonly considered to be a scaling factor. Alternatively, if the subjectified version is applied, its moving average, which represents a “normal” level of inflation, serves as a scaling factor for inflation perception quantification. Our analysis indicates that this standard setup rarely reflects consumers intentions closely; however, lagged inflation serves as a scaling factor for the most relevant method in about half of our cases.
Secondly, the final choice of the quantification method should be made by the data users: academic staff or central bank staff that need quantified time series to conduct qualitative or quantitative analysis. The users could choose the best evaluation criteria aligned for their goals. Criteria applicability depends on the purpose of examination for which expectations are to be used. We prefer the first evaluation criterion that captures the strongest correlation of expectations and balance statistics and recommend the application of this option if the study’s aim is to test the rationality of expectations. Unbiasedness is the first condition tested to verify the rationality of expectation and relates to the systematic forecast errors made by economic agents (Muth, Citation1961). It needs to be highlighted that the second criterion concerning the alignment of expectations and BS, and the third one about expectations accuracy react stronger to turbulent economic circumstances, which results from the fact that increased uncertainty has a significant impact on market entities, especially in the case of consumers.
If the optimal quantification procedure is needed to proxy expectations used for comparative studies, the priority should be given to criterion that returns the same (or the closest) methods for all economies considered.
We recommend that for policy analysis, central banks apply the quantification procedure based on the second and third valuate criteria. Thanks to this, central banks will take into account expectations quantified on the basis of double criteria in their decisions. Moreover, central banks have at their disposal staff and tools to provide computational exercises with combined version to check for its closer corelation to BS.
Eventually, the differentiation of optimal methods selected within the quantification procedure, confirms our intuition about consumer expectations behaviour, that there are cross-country differences in their formation (Bräuning & van der Cruijsen, Citation2019) and the patterns of behaviour (Łyziak & Mackiewicz-Łyziak, Citation2014). Expected inflation could also have different macro effects, including diverging inflation dynamics in monetary union (Lagoa, Citation2017). This implies that adopted evaluation criteria for the quantification procedure could change over time. Considering that the current hypotheses of expectations formation reflect the modification or step changes of expectations formation patterns (adaptive learning, bounded rationality), our results are not surprising. The obtained results indicate that for the majority of cases, subsample results diverge from the full-sample optimal procedure. However, for Spain, Croatia, Hungary, Sweden, and the UK the same method proved to be optimal at least four out of six times. This means that for a group of countries with institutionally similar we can find some universality over time and evaluation criterion for the most relevant method.
5. Conclusion
Central banks use qualitative research to quantify inflation expectations. The process of quantifying expectations is not an easy task, because the appropriate quantification method must be selected. The studies presented in the literature indicate the possibility of using many different quantification methods, mostly based on the basic probabilistic method or the regression method. This fact indicates the need for developing a procedure allowing for the selection of the optimal quantification method.
Therefore, the article proposes the novel optimization procedure, the use of which will allow for the selection of the optimal method of quantifying inflation expectations due to the adopted evaluation criterion. The evaluation criteria that we consider novelty of this study, were based on the assessment of the degree of correlation between the balance statistics and quantified expectation, the evaluation of directional co-movement of expectations and balance statistics and minimization of forecast errors.
The application of the optimization procedure is presented on the empirical example of inflation expectations for the selected 19 member states of the European Union in the two research periods. For each economy, we ran 6 regression methods and 35 probabilistic methods for quantifying inflation expectations. In the case of regression models, we found that their applicability is limited due to econometric constraints, and ultimately focused only on probabilistic methods. Then, based on three evaluation criteria, we chose the optimal probabilistic methods for each country and we discussed the obtained results.
The study contributes to the existing literature by providing the novel tool to identify the optimal method of expectations quantification in terms of reflecting responder intention. We referred to two groups of quantification methods and our empirical study revealed the econometrical difficulties that undermine the applicability of regression methods. The obtained results also indicated that in the probabilistic methods based on the Carlson and Parkin procedure, different scaling factors should be assumed and alternative distributions to the normal distribution should be taken into account.
In order to maintain the readability of the presented results, for each of the criteria one optimal method was presented, which had the highest percentile values for the assumptions made. As it has already been emphasized, in the case of the second and third criteria, these criteria lead to a set of optimal methods. We find this feature being the most important limitation of our study. Another limitation is about the procedure complexity: before identifying an individualized method for an economy large computational exercises are required. As expectations formation patterns change over time, the optimal procedure of quantification could become stale and needs to be reverified.
Ultimately, this research on finding the optimal quantification procedure for the given three evaluation criterions showed us the direction of further research in the form of developing a novel quantification method. Undoubtedly, the results of this study will become a solid reference point for framing an innovative, fuzzy approach to the quantification of inflation expectations.
Supplemental Material
Download MS Excel (293.5 KB)Disclosure statement
No potential conflict of interest was reported by the author(s)
Additional information
Funding
Notes
1 We exclude the Eurozone new-joiners, as their addition to the club was a game-changer for expectations formation.
2 The UK was the EU member state once we launched our examination.
3 This assumption finds is justified in light of the Central Limit Theorem and in the simplicity of calculation. Moreover, it was developed in Carlson and Parkin (Citation1975) and Bachelor and Orr (1988), which grounded the method in the literature. The argument that empirical proxies do not mimic normal distribution could be easily challenged as consumer expectations should not be juxtaposed with market-based measures of expectations. Regardless of a broad argument in favor of normal distribution, probabilistic extensions for other distributions exist and are tested in this study.
4 Depending on the type of data and the research problem undertaken, one may assume an arbitrary percentile. We suggest that the baseline percentile values are above 90%.
5 Romania is the exception here. For full sample estimations, food prices are the most relevant scaling factor. The explanation could be linked to the unusually large weight of food prices in the Romanian consumption basket (35%). The changes in food and energy prices explain about half of the total CPI changes till 2011. Additionally, food prices registered shocks related to harvest that put both upward and downward pressure on inflation (IMF Citation2012). The result could also be explained by the relatively high volatility of inflation rates and interrupted disinflation process in Romania: in such circumstances, consumers tend to adhere to the most important prices.
References
- Acedański, J., & Włodarczyk, J. (2016). Dispersion of inflation expectations in the European Union during the global financial crisis. Equilibrium, 11(4), 737–749. https://doi.org/10.12775/EQUIL.2016.033
- Anderson, O. (1952). The business test of the IFO-institute for economic research, Munich, and its theoretical model. Revue de l’Institut International de Statistique/Review of the International Statistical Institute, 20(1), 1–17. https://doi.org/10.2307/1401826
- Andreou, E., Eminidou, S., & Zachariadis, M. (2016). Inflation expectations and monetary policy in Europe. (No. CEPR Discussion Paper11306).
- Ang, A., Bekaert, G., & Wei, M. (2007). Do macro variables, asset markets, or surveys forecast inflation better? Journal of Monetary Economics, 54(4), 1163–1212. https://doi.org/10.1016/j.jmoneco.2006.04.006
- Armantier, O., Bruine de Bruin, W., Topa, G., van der Klaauw, W., & Zafar, B. (2015). Inflation expectations and behavior: Do survey respondents act on their beliefs? International Economic Review, 56(2), 505–536. https://doi.org/10.1111/iere.12113
- Batchelor, R. A., & Orr, A. B. (1988). Inflation expectations revisited. Economica, 55(219), 317. https://doi.org/10.2307/2554010
- Berk, J. M. (1999). Measuring inflation expectations: A survey data approach. Applied Economics, 31(11), 1467–1480. https://doi.org/10.1080/000368499323337
- Bliemel, F. (1973). Theil’s forecast accuracy coefficient: A clarification. https://doi.org/10.1177/002224377301000413
- Bräuning, C., & van der Cruijsen, C. (2019). Learning dynamics in the formation of European inflation expectations. Journal of Forecasting, 38, 122–135. https://doi.org/10.1002/for.2553
- Breitung, J., & Schmeling, M. (2013). Quantifying survey expectations: What’s wrong with the probability approach? International Journal of Forecasting, 29(1), 142–154. https://doi.org/10.1016/j.ijforecast.2012.07.005
- Cagan, P. (1956). The monetary dynamics of hiperinflation. In M. Friedman (Ed.), Studies in the quantity theory of money. University of Chicago Press.
- Carlson, J. A., & Parkin, M. (1975). Inflation Expectations. Economica, 42(166), 123–138. https://doi.org/10.2307/2553588
- Carroll, C. D. (2003). Macroeconomic expectations of households and professional forecasters. The Quarterly Journal of Economics, 118(1), 269–298. http://www.jstor.org/stable/25053904 https://doi.org/10.1162/00335530360535207
- Claveria, O. (2021). Uncertainty indicators based on expectations of business and consumer surveys. Empirica, 48(2), 483–505. https://doi.org/10.1007/s10663-020-09479-1
- Cornand, C., & Heinemann, F. (2018). Experiments on macroeconomics: Methods and applications.
- Cornand, C., & Hubert, P. (2020). On the external validity of experimental inflation forecasts: A comparison with five categories of field expectations. Journal of Economic Dynamics and Control, 110, 103746. https://doi.org/10.1016/j.jedc.2019.103746
- Cunningham, A. (1997). Quantifying Survey Data (No. Q3). Retrieved June 10, 2019, from https://www.bankofengland.co.uk/quarterly-bulletin/1997/q3/quantifying-survey-data
- European Commission. (2020). The joint harmonised EU programme of business and consumer surveys. User guide (pp. 1–53). https://ec.europa.eu/info/sites/info/files/bcs_user_guide_2020_02_en.pdf
- Gerberding, C. (2001). The Information Content of Survey Data on Expected Price Development for Monetary Policy (No. 9). Frankfurt am Mein.
- Hałka, A., & Łyziak, T. (2015). How to define the consumer perceived price index? An application to polish data. Eastern European Economics, 53(1), 39–56. https://doi.org/10.1080/00128775.2015.1033291
- IMF. (2012). Staff country report 12/76.
- Lahiri, K., & Zhao, Y. (2015). Quantifying survey expectations: A critical review and generalization of the Carlson–Parkin method. International Journal of Forecasting, 31(1), 51–62. https://doi.org/10.1016/j.ijforecast.2014.06.003
- Lagoa, S. (2017). Determinants of inflation differentials in the Euro area: Is the new Keynesian Phillips curve enough? Journal of Applied Economics, 20(1), 75–103. https://doi.org/10.1016/S1514-0326(17)30004-1
- Lolić, I., & Sorić, P. (2018). A critical re-examination of the Carlson–Parkin method. Applied Economics Letters, 25(19), 1360–1363. https://doi.org/10.1080/13504851.2017.1420880
- Lucas, R. E. (1972). Expectations and the neutrality of money. Journal of Economic Theory, 4(2), 103–124. https://doi.org/10.1016/0022-0531(72)90142-1
- Łyziak, T., & Mackiewicz-Łyziak, J. (2014). Do consumers in Europe anticipate future inflation? Eastern European Economics, 52(3), 5–32. https://doi.org/10.2753/EEE0012-8775520301
- Łyziak, T., & Stanisławska, E. (2006). Current inflation and different measures of consumer inflation expectations in selected countries. Bank & Credit, 37(10), 17–30.
- Łyziak, T. (2010). Measurement of perceived and expected inflation on the basis of consumer survey data. (No. Irving Fisher Committee Working Papers 5).
- Mankiw, N. G., & Reis, R. (2002). Sticky information versus sticky prices: A proposal to replace the New Keynesian Phillips Curve. The Quarterly Journal of Economics, 117(4), 1295–1328. https://doi.org/10.1162/003355302320935034
- Muth, J. F. (1961). Rational expectations and the theory of price movements. Econometrica, 29(3), 315. https://doi.org/10.2307/1909635
- Pesaran, M. H. (1984). Expectations formation and Macroeconometric Modelling. In P.-A. Muet (Ed.), Contemporary Macroeconomic Modelling (pp. 27–53). Oxford: Basil Blackwell.
- Pesaran, M. H., & Weale, M. (2006). Survey expectations. In Handbook of economic forecasting. OSF. https://doi.org/10.1016/S1574-0706(05)01014-1
- Ranyard, R., Del Missier, F., Bonini, N., Duxbury, D., & Summers, B. (2008). Perceptions and expectations of price changes and inflation: A review and conceptual framework. Journal of Economic Psychology, 29(4), 378–400. https://doi.org/10.1016/j.joep.2008.07.002
- Rutkowska, A., Szyszko, M., & Pietrzak, M. B. (2022, February 7). Inflation expectation—survey data. OSF. https://doi.org/10.17605/OSF.IO/P5GTA
- Rutkowska, A., Szyszko, M., & Pietrzak, M. B. (2022, February 7). Estimation of inflation expectation. https://doi.org/10.17605/OSF.IO/FWKHV
- Sargent, T. (1993). Bounded rationality in macroeconomics. Oxford University Press.
- Simmons, P., & Weiserbs, D. (1992). Consumer price perceptions and expectations. Oxford Economic Papers, 44(1), 35–50. https://www.jstor.org/stable/2663423
- Smith, J., & McAleer, M. (1995). Alternative procedures for converting qualitative response data to quantitative expectations: An application to Australian manufacturing. Journal of Applied Econometrics, 10(2), 165–185. https://doi.org/10.1002/jae.3950100206
- Stanisławska, E. (2019). Consumers’ perception of inflation in inflationary and deflationary environment. Journal of Business Cycle Research, 15(1), 41–71. https://doi.org/10.1007/s41549-019-00036-9
- Szyszko, M., Rutkowska, A., & Kliber, A. (2020). Inflation expectations after financial crisis: Are consumers more forward-looking? Economic Research-Ekonomska Istrazivanja, 33(1). https://doi.org/10.1080/1331677X.2019.1595083
- Theil, H. (1952). On the time shape of economic microvariables and the Munich business test. Review of the International Statistical Institute, 20, 105–120.
- Woodford, M. (2003). Interest and prices: Foundations of a theory of monetary policy. Princeton University Press. https://doi.org/10.1111/j.0013-0427.2005.430_2.x