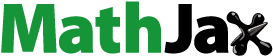
Abstract
This study employs a prominent model comparison criterion, namely the Bayes factor, to compare three commonly used GARCH models with their stochastic volatility (SV) counterparts in modelling the dynamics of inflation rates. By using consumer price index (CPI) data from 18 developed countries to evaluate these models, we find that the GARCH models are generally outperformed by their stochastic volatility counterparts. Furthermore, the stochastic volatility in mean (SV-M) model is shown to be the best for all 18 countries considered. The paper also examines which model characteristics play a main role in modelling inflation rates. It turns out that inflation volatility feedback is a crucial feature that we should take into consideration when modelling inflation rates. The relevance of a leverage effect, however, is found to be rather ambiguous. Finally, the forecasting results using the log predictive score confirm these findings.
1. Introduction
Inflation and its volatility have received increasing attention in the economic literature due to their potential adverse impacts on the real economy. Theoretical studies demonstrate that high volatile inflation will cause an inefficient allocation of resources and thus may decrease economic growth and raise the unemployment rate (see, e.g., Friedman, Citation1977; Lucas, Citation2000). Given the cost of high volatile inflation, understanding the interaction between inflation and inflation uncertainty plays a key role in implementing an effective monetary policy.Footnote1
To document this relationship, empirical studies have to model inflation uncertainty. Two popular methods have been used extensively in the literature. In a conventional approach, this uncertainty can be modelled by a class of generalised autoregressive conditional heteroscedastic (GARCH) models, where the inflation volatility is a deterministic function of past data and the model parameters (see, e.g., Daal et al., Citation2005; Grier & Perry, Citation1998; Keskek & Orhan, Citation2010; Kontonikas, Citation2004). Alternatively, recent studies have employed stochastic volatility models, under which inflation uncertainty is treated as a latent variable that follows an autoregressive model of order one, AR(1), process (see, e.g., Berument et al., Citation2012; Chan, Citation2017; Stock & Watson, Citation2007). Unfortunately, these two types of volatility models are non-nested and their implied inflation volatilities demonstrate very different characteristics. Therefore, classical econometric methods cannot be used to compare these two models. Since modelling inflation volatility plays a crucial part in documenting the nexus between inflation and its uncertainty, it is of great importance to straightly evaluate the model fit of these two types of volatility models by carrying out a formal model comparison. Yet, such a comparison is rarely performed in the literature.
The present paper fills the gap by comparing the model fit of commonly used GARCH models with that of their stochastic volatility counterparts in modelling the dynamics of inflation rates. We also penalise the complex models to avoid over-fitting. To this end, we employ a commonly used Bayesian model comparison approach, namely the Bayes factor, to investigate the evidence in support of the GARCH models against their stochastic volatility counterparts given the observed data. The Bayes factor is computed as the ratio of a likelihood of one particular model to that of another, and it can be used to assess the strength of evidence in favour of one model among two competing two models. Therefore, we need to calculate a marginal likelihood for each model first, and then use them to compute the Bayes factor. The marginal likelihood can be referred to as the data density, which indicates how likely it is that the observed data occurs given the model.
More specifically, for the Bayesian comparison exercise, we consider three GARCH specifications that are commonly used for modelling inflation volatility in empirical studies: (1) the standard GARCH, (2) GARCH with an asymmetric (or leverage) effect, and (3) GARCH in mean. We then select three stochastic volatility models which are closely parallel to GARCH models: (1) standard stochastic volatility, (2) stochastic volatility with a leverage effect, and (3) stochastic volatility in mean. First, by using pairwise comparison between GARCH models and their stochastic volatility counterparts (standard GARCH versus standard stochastic volatility, GARCH with a leverage effect versus stochastic volatility with a leverage effect, and GARCH in mean versus stochastic volatility in mean), we can evaluate which model (GARCH or stochastic volatility) is more strongly supported by the observed data. Second, we investigate which model features play a crucial role in modelling the inflation dynamics by directly comparing the more complex GARCH specifications with the standard one (and also the more complex stochastic volatility variants with the standard one). Finally, we examine the impact of inflation uncertainty on inflation.
The main findings, using the CPI data from 18 advanced economies, are obtained as follows. First, the stochastic volatility specifications generally outperform their GARCH counterparts, which demonstrates that inflation uncertainty is better documented as a latent variable under stochastic volatility models than as a deterministic conditional variance under GARCH models. This finding is consistent with the results in both the energy economic literature (see, e.g., Chan & Grant, Citation2016a) and finance literature (see, e.g., Kim et al., Citation1998) that favour the stochastic volatility models. Second, for all series considered, the inflation uncertainty feedback under both the stochastic volatility in mean and GARCH in mean is empirically important for modelling the dynamics of inflation rates. The relevance of the leverage effect, on the other hand, is found to be ambiguous under both classes of time-varying volatility models. Third, we find that inflation uncertainty has a positive impact on inflation, which confirms a hypothesis proposed by Cukierman and Meltzer (Citation1986).Footnote2 Fourth, stochastic volatility in mean is the best model for all 18 series, followed by the GARCH in mean, which again confirms the importance of inflation volatility feedback. Finally, the forecast-based comparison results using the log predictive score for both the expanding and rolling samples confirm these findings.
There have been other studies that investigate the relationship between inflation and inflation uncertainty. However, to the best of our knowledge, this is the first study to compare the performance of GARCH models with that of their stochastic volatility counterparts in modelling inflation dynamics. Grier and Perry (Citation1998) investigate the linkage between inflation and inflation uncertainty for G7 countries using the GACH models. They show that inflation Granger-causes inflation uncertainty for all G7 countries. However, mixed evidence on the impact of inflation volatility on inflation is found. Daal et al. (Citation2005) employ the asymmetric power GARCH (PGARCH) model to explore the link between inflation and inflation volatility for 22 countries. They find that positive shocks to inflation have stronger effects on inflation volatility for Latin American countries. Berument et al. (Citation2012), using the stochastic volatility in mean model to examine the interaction between inflation and inflation uncertainty for the United States, demonstrate that an innovation in inflation volatility results in an increase in inflation rates. Using data from Germany, the United States, and the United Kingdom, Chan (Citation2017) introduces the time-varying parameter stochastic volatility in mean specification to model the inflation rates. He demonstrates that inflation volatility has a positive effect on the inflation rate for all three countries considered. Furthermore, the results clearly show that the volatility-related coefficients exhibit significant time-variation.
The remainder of this study is organised as follows. In Section 2, we outline two kinds of volatility models in modelling inflation dynamics, which are stochastic volatility and GARCH models. Section 3 gives a brief introduction of model comparison using the Bayes factor and introduces an importance sampling algorithm to compute marginal likelihoods with a view of evaluating these two classes of models. In Section 4, we provide the empirical findings which include the descriptive statistics, unit root tests, the Bayesian model comparison, and the estimation results of the two classes of time-varying volatility models. Section 5 presents the forecast-based comparison results using the log predictive score for both expanding samples and rolling samples. Finally, Section 6 concludes.
2. Models
2.1. GARCH models
In this section, we introduce three common generalised autoregressive conditional heteroscedasticity (GARCH) models that are employed to model inflation uncertainty.Footnote3 First, we consider a standard one, namely the GARCH(1,1) model (referred to as GARCH hereinafter):
(1)
(1)
(2)
(2)
where πt is the inflation rate,
is a constant, and
To make sure the variance process is always stationary, we impose the restriction
It can be clearly seen that the conditional variance
representing a proxy for the inflation volatility is determined by past data and the model parameters.
Another common GARCH model that is widely used in modelling inflation uncertainty is the GARCH-GJR model developed by Glosten et al. (Citation1993). The GARCH-GJR model accounts for asymmetric (leverage) effects of positive and negative disturbances on the conditional variance. To be more specific, the conditional variance equation is defined as follows:
(3)
(3)
where
denotes an indicator function. The parameter θ captures the asymmetric effect: if
a negative shock would have a greater impact on inflation uncertainty; if
a negative shock would lower inflation uncertainty; and if θ = 0, there is no asymmetric effect documented, and thus this specification becomes the standard GARCH model.
The last one we consider is the GARCH in mean model (referred to as GARCH-M) that accounts for potential volatility feedback on the inflation rates:
(4)
(4)
(5)
(5)
The effect of inflation volatility on inflation itself is captured by the parameter λ: when inflation uncertainty has a positive impact on the inflation rate; when
inflation uncertainty has a negative impact on the inflation rate; and when λ = 0, inflation uncertainty has no impact on the inflation rate, and thus this specification reduces to the standard GARCH model.
2.2. Stochastic volatility models
In this section, we consider three stochastic volatility variants which are fairly close parallels to the three GARCH specifications just mentioned. In contrast to the GARCH specifications, the inflation uncertainty under the stochastic models is a latent variable following a stochastic process. The first model we consider is the standard stochastic volatility model, which is referred to as SV:
(6)
(6)
(7)
(7)
Here, the inflation uncertainty is specified in a logarithmic form ht that follows an AR(1) process. To make sure this process is always stationary, we impose the restriction Note that the parameter
captures the uncertainty of future inflation volatility and that the two innovations
and
are assumed to be uncorrelated under the standard stochastic volatility model.
Next, we consider the counterpart of the GARCH-GJR specification, which is the stochastic volatility model with a leverage effect (see, e.g., Omori et al., Citation2007). Specifically, we accommodate a potential correlation between the two disturbances and
as follows:
To model the potential correlation, we assume that and
jointly follow a bivariate normal distribution. The correlation parameter ρ captures the leverage effect: if
a negative shock to inflation rate at time t − 1 tends to decrease the inflation uncertainty at time t; if
a negative shock at time t − 1 tends to increase the inflation uncertainty at time t; and if ρ = 0, there is no leverage effect documented, and this variant becomes the standard SV. We refer to this specification as SV-L.
Similar to the GARCH-M, the stochastic volatility in mean model proposed by Koopman and Hol Uspensky (Citation2002) accommodates the possibility of volatility feedback:
(8)
(8)
(9)
(9)
The parameter λ here captures the impact of inflation volatility on the inflation rate: when inflation volatility has a positive effect on the inflation rate; and when λ = 0, there is no volatility feedback documented.
3. Model comparison
In this section, we provide a brief introduction of model comparison employing a prominent Bayesian criterion named the Bayes factor. In addition, we outline an adaptive importance sampling algorithm introduced in Chan and Eisenstat (Citation2015) to compute the Bayes factor.
3.1. Bayes factor
Suppose we have a set of L models and need to compare them. Let
be actual observed data, where T is the number of observations. Then, each model Ml, with
is constituted by two components: (1) a prior density
and (2) a likelihood function
which relies on the parameter vector
To perform a model comparison exercise, we employ a prominent Bayesian criterion, namely the Bayes factor, that is given by
(10)
(10)
where
is the marginal likelihood under the model
and is computed as
(11)
(11)
From this definition, we can simply interpret the marginal likelihood as a density of the data given the model Ml evaluated with the actual data Thus, if the data is highly likely under the model Ml, the implied log marginal likelihood would be relatively small in absolute value and vice versa. In other words, if the Bayes factor BFij > 1, the model Mi is more favoured by the observed data
than the model Mj.Footnote4 Jeffreys (Citation1998) provides a scale for a more concrete interpretation of the Bayes factor BFij: a Bayes factor in the interval (3, 10) indicates moderate evidence to support the model Mi; a Bayes factor in the interval (10, 30) provides strong evidence; a Bayes factor in the range (30, 100) provides very strong evidence; and if a Bayes factor is greater than 100, we have extreme evidence in favour of model Mi.
To calculate the Bayes factor, we need to compute the marginal likelihoods. In what follows, we outline an efficient method to compute the marginal likelihoods for both the GARCH-type and SV-type models.
3.2. Importance sampling for marginal likelihoods
One main challenge for calculating the marginal likelihood is to evaluate the integral in EquationEquation (11)(11)
(11) since it is often non-standard and of high dimension and thus cannot have an analytic solution. Following Chan and Eisenstat (Citation2015), we compute the marginal likelihoods for both the stochastic volatility and GARCH models using an adaptive importance sampling algorithm. To this end, let
be the proposal density. The marginal likelihood can then be rewritten as follows:Footnote5
Let for all
be an independent draw obtained from the proposal density
then the estimated marginal likelihood is computed as
(12)
(12)
and is shown to be unbiased and simulation consistent. It is clear that the performance of this estimator depends heavily on the choice of the proposal density
Chan and Eisenstat (Citation2015) provide a way of obtaining an optimal proposal density by minimising the Kullback-Leibler divergence (or cross-entropy distance) to the zero-variance density.Footnote6 Once the proposal density
is obtained, we can quickly construct the importance sampling estimator for the GARCH models as the corresponding likelihoods
are available analytically, and thus can be evaluated easily. As an example, the log-conditional likelihood
under the standard GARCH model is given as follows:
Unfortunately, we do not have an analytical form for the likelihoods under the stochastic volatility models. Thus, we need to evaluate them by employing an importance sampling algorithm. More specifically, recall that the integrated (or observed-data) likelihood under the stochastic volatility models is given as follows:
where
is the joint density of
and h,
is the prior density of the log-inflation volatilities
and
is the conditional likelihood. Let
be a proposal density; the integrated likelihood can then be rewritten as
(13)
(13)
Suppose …,
are N independent draws from the proposal density
then the integrated likelihood
can be approximated by
(14)
(14)
4. Empirical findings
4.1. Data
In this paper, we use quarterly CPI data for advanced economies obtained from the Federal Reserve Economic Data.Footnote7 All data series are seasonally adjusted by the X-13-ARIMA SEAT (autoregressive integrated moving average, seasonal extraction in ARIMA time series) method developed by the U.S. Census Bureau. Inflation is then computed as the first difference of the log of CPI: displays the summary statistics and unit root tests for 18 advanced countries. From the table, we find that (for all data series): (1) the inflation distribution exhibits positive (right) skewness; (2) the inflation distribution tends to be leptokurtic owing to high excess kurtosis; (3) the Jarque-Bera (JB) test confirms these results: the test rejects the null hypothesis that the inflation rate follows a normal distribution; (4) both the augmented Dickey-Fuller (ADF) and Phillips-Perron (PP) tests reject the null hypothesis, implying that all the data series are stationary.Footnote8
Table 1. Summary statistics and unit root tests.
4.2. Model comparison findings
In this exercise, we perform the model comparisons between the three commonly used GARCH variants and their SV counterparts using the algorithm presented in Section 3. The results are shown in .Footnote9 Some broad overviews are obtained from this exercise. On the whole, the best model for all 18 countries is the SV-M model, which is followed by the GARCH-M model. Second, with only a few exceptions of GARCH-vs-SV and GARCH-GJR-vs-SV-L pairs, the GARCH variants are outperformed by their SV counterparts. For example, let us consider the results for Canada. The log marginal likelihoods under the GARCH and SV specifications are −569.8 and −562.5, respectively, indicating a Bayes factor of 1480.30 in support of the SV model against its GARCH competitor. According to Jeffreys (Citation1998), this demonstrates decisive evidence for choosing the former model. The Bayes factors for the two remaining pairs are even larger, which again indicates decisive evidence in favour of the SV-type models. This finding is consistent with the result in the energy economic literature that the stochastic volatility variants generally outperform their GARCH counterparts in modelling energy price dynamics (see, e.g., Chan & Grant, Citation2016a). Furthermore, stochastic volatility specifications have been shown to perform better in modelling financial returns (see, e.g., Kim et al., Citation1998; Yu, Citation2002).
Table 2. Log marginal likelihood of two classes of volatility models for 18 rich OECD countries’ inflation. The numbers in parentheses are numerical standard errors.
Exceptions to this overall trend are the two pairs GARCH-vs-SV and GARCH-GJR-vs-SV-L for four countries. Interestingly, these two pairs follow the same pattern: whenever the SV model outperforms its GARCH counterpart, the SV-L model dominates the GARCH-GJR one and vice versa. However, the SV-M models outperform their GARCH-M counterparts for all the countries. For instance, let us consider the results for the United States. The log marginal likelihood for the GARCH and SV models are −513.8 and −520.0, respectively. This demonstrates a Bayes factor of 492.75 in support of the GARCH model against its SV competitor, showing decisive evidence for the former model. A similar conclusion is drawn for the GARCH-GJR-vs-SV-L pair with a smaller, but still relatively large Bayes factor of 221.41 in favour of GARCH-GJR. In contrast, the GARCH-M model is overwhelmed by the SV-M one with a Bayes factor of to support the latter model. Similar findings are found when this is applied to three other countries (Germany, Belgium, and Switzerland).
Now, we turn to examine which model characteristics play a crucial role in explaining the dynamics of inflation rates. First, we investigate the importance of the leverage effect by juxtaposing GARCH with GARCH-GJR and SV with SV-L. For both the GARCH-type and SV-type models, the results are rather mixed. In essence, accounting for the asymmetric effect increases the marginal likelihood for countries like Canada, Australia, and the United States, whereas the Netherlands, France, and the United Kingdom experience a decrease in their marginal likelihoods. These findings may seem surprising; since the GARCH-GJR nests the GARCH (the SV-L nests the SV), the former would be assumed to provide a better fit. However, remember that the marginal likelihood is in fact a density evaluation, and thus it suffers a penalty for the complexity of model. Therefore, these findings demonstrate that the cost of model complexity could exceed its benefit when referring to the leverage effect.
Finally, we compare the GARCH with GARCH-M and the SV with SV-M to explore the relevance of volatility feedback for modelling the inflation rates. It is clear that the volatility feedback plays a key role in explaining the dynamics of the inflation rates. More specifically, the Bayes factors for all the countries’ data in support of the GARCH-M against GARCH are extremely large (for instance, and 1636 for Canada and France, respectively), which implies decisive evidence in favour of the former. Similar findings are achieved for the SV models.
4.3. Bayesian estimation results
This section provides estimated results of model-specific parameters for both GARCH-type and SV-type specifications.Footnote10 Because of space limits, we only report the posterior estimates for the G7 countries, which largely represent the findings for the remaining countries.
4.3.1. Bayesian estimation for GARCH models
The estimated results for the GARCH models are presented in . It can be easily checked that most of the parameter estimates across the three models for all countries are statistically different from zero. For instance, let us consider the results for Canada. In the GARCH model, the parameter α is estimated at 2.78, and its 95% credible interval is estimated to be which excludes zero, indicating the estimate is statistically different from zero. A similar result is obtained by the GARCH-GJR model while the GARCH-M model experiences a relatively smaller estimate of α. This is due to the effect of the volatility feedback on the mean equation. The parameters describing the persistence of the inflation volatility equation (δ and γ) have quite similar estimated results across the three models and are statistically different from zero. More specifically, the inflation volatility equation is highly persistent for all three variants with the sum of the two parameters δ and γ ranging from 0.88 to 0.96, which is consistent with previous literature (see, e.g., Grier & Perry, Citation1998). Similar findings are found when this is applied for the remaining six countries.
Table 3. Bayesian estimation for the GARCH models: Estimated posterior means (posterior standard error in parentheses).
We then further explore the dynamics of the inflation rates through model features. First, we consider the leverage effect. For Canada, the posterior estimate of θ is −0.39 and is statistically different from zero due to its credible interval excluding zero, implying that a negative shock at time t − 1 would lower the inflation volatility at time t. A similar result is found for the United States with the leverage effect θ being estimated at −0.43.Footnote11 However, the posterior estimate of θ is insignificant for countries like France, Germany, and Italy, showing no asymmetric effect. These findings support the ranking of marginal likelihood shown in the previous section.Footnote12 In other words, the leverage effect is found to be mixed. This finding is in line with previous literature. For example, Daal et al. (Citation2005) employ the PGARCH model to capture the asymmetric effect of inflation volatility for 22 countries and show that there are mixed results regarding the relevance of the leverage effect.
Finally, we investigate the impact of inflation volatility on the inflation rate. It is clearly shown that the volatility feedback plays a crucial role in modelling the inflation rates. As an example, let us consider the results for France. The volatility parameter λ is estimated at 0.46 and is statistically significant, implying that inflation volatility has a positive effect on the inflation rate. The same conclusions are drawn for the remaining countries. The finding is consistent with the ranking of marginal likelihood that favours GARCH-M over GARCH.
4.3.2. Bayesian estimation for stochastic volatility models
provides results for the three stochastic volatility variants. Similar to the findings from the GARCH models, most of the posterior estimates across the three variants are statistically significant. Also, all the models imply high persistence of the inflation volatility equation with the posterior estimate of ρh ranging from 0.92 to 0.98, which is in line with previous literature. For instance, Chan (Citation2017) proposes the time-varying parameter stochastic volatility in mean (TVP-SVM) variant to model inflation dynamics and shows high persistence of 0.963 for the transition of inflation volatility.
Table 4. Bayesian estimation for the stochastic volatility models: Estimated posterior means (posterior standard error in parentheses).
Next, we consider the importance of the leverage effect for modelling inflation rates. Similar to the GARCH-GJR results, the posterior estimate of the leverage parameter ρ under SV-L is consistent with the findings from the marginal likelihoods. More specifically, recall that Canada experiences an increase in the marginal likelihood when adding the leverage effect, and thus we would expect that little mass around zero is observed in the posterior distribution of ρ. This is indeed the case since the 95% credible interval of ρ excludes zero. In addition, a positive correlation indicates that a negative shock at time t − 1 decreases the volatility at time t, which is in line with the GARCH-GJR findings. Similarly, the posterior estimate of ρ for the United States is 0.37 and is statistically significant from zero. In the instance of France, the posterior estimate of ρ under SV-L is 0.15, but is insignificant, indicating that no leverage effect is found. Same conclusions are drawn for Italy, Japan and the United Kingdom. Contrary to the finding from the GARCH-GJR model, the asymmetric effect for Germany under the SV-L is estimated to be 0.44 and its 95% credible interval excludes zero. This is, however, consistent with the finding from the model comparison using the log marginal likelihood.
Finally, we investigate the relevance of the inflation volatility feedback in explaining the dynamics of the inflation rates. Similar to the GARCH-M findings, the volatility feedback parameter λ under SV-M is estimated to be positive and is significantly different from zero for all countries, implying that the volatility feedback is relevant in modelling the inflation rates. This finding is in line with the empirical work of Berument et al. (Citation2012), who employ the SV-M model and demonstrate that an innovation in inflation volatility generates an increase in inflation. Furthermore, the estimate of the volatility parameter λ under SV-M is considerably larger than that under the GARCH-M, which shows that a relatively stronger volatility feedback is found under the SV-M. These findings can be generalised to all the remaining countries considered.
5. Forecast-based comparison
In this exercise, we perform the forecast-based comparisons between the GARCH specifications and their SV counterparts. More specifically, we compare these models by employing the log predictive score for both expanding samples and rolling samples.Footnote13 A greater value of the log predictive score demonstrates better prediction performance, and vice versa.
5.1. Expanding samples
First, we calculate the one-step-ahead density forecast under a certain model. Clearly, it is the predictive density for
computed at time t by employing the data from periods 1, 2,…, t. This predictive density is then evaluated at the actual observed data
by computing the log predictive likelihood
). It is apparent that this log likelihood will be large when the actual value
is highly likely under the predictive density, and vice versa. Second, we repeat the above exercise using the data up to time t + 1, t + 2, and so forth. Lastly, the log predictive score for the expanding samples is computed as the sum of the log predictive likelihoods:
Here, …, T are evaluation periods. The one-step-ahead predictive likelihood
can be computed as follows:
Suppose …,
are N draws from the posterior distributions of the parameters, then the predictive likelihood can be approximated by:
5.2. Rolling samples
While the expanding samples make use of the entire sample, the rolling samples employ the most recent data of CPI, and the log predictive score is computed as:
Similarly, the one-step-ahead predictive likelihood for the rolling samples can be approximated using draws
from the posterior distributions of the parameters:
5.3. Forecasting results
This section provides the forecasting results of the two exercises for Canada.Footnote14 The evaluation period for both the expanding samples and rolling samples is from 2009Q1 to 2018Q4. The results are presented in . The forecast-based comparison findings are widely similar to the model comparison findings using the Bayes factor. More specifically, the SV specifications produce better forecast performance than their GARCH competitors. In addition, the volatility feedback improves the predictive density significantly for both the GARCH and SV variants. Yet, the importance of the leverage effect is found to be rather ambiguous. For instance, let us consider the forecasting results of the expanding sample. The log predictive score for the GARCH and SV models are −84.15 and −75.16, respectively. This demonstrates better density predictions of the SV model against its GARCH counterpart. A similar conclusion is drawn for the GARCH-GJR-vs-SV-L and the GARCH-M-vs-SV-M pairs. Moreover, we find that the volatility feedback is important for modelling the inflation dynamics by comparing the GARCH with GARCH-M and the SV with SV-M. Next, we investigate the relevance of the leverage effect by comparing the forecast performance of the GARCH and SV specifications with that of the GARCH-GJR and SV-L models. The numbers show that the GARCH-GJR and SV-L specifications give better density forecasts than the GARCH and SV models, respectively, which demonstrates the importance of the leverage effect for Canada.Footnote15 These findings are confirmed when we employ the rolling samples.
Table 5. Log predictive score of two classes of volatility models for both the expanding samples and rolling samples (Canada).
6. Conclusion
In this paper, we have performed a Bayesian estimation to evaluate three widely used GARCH specifications and their stochastic volatility counterparts in modelling inflation rates for 18 advanced countries. By employing a formal Bayesian comparison criterion–the Bayes factor–to compare a variety of models, we find that the GARCH variants are generally surpassed by their stochastic volatility counterparts in modelling inflation dynamics. In addition, the stochastic volatility in mean model is shown to be the best one for all 18 countries considered. The forecast-based comparison results using the log predictive score for both the expanding samples and rolling samples confirm these findings.
The study also investigates which model characteristics are important in modelling inflation rates. We show that the inflation volatility feedback is a crucial feature that we should take into consideration when modelling the inflation rates. Moreover, inflation volatility has a positive impact on the inflation rate, which confirms a hypothesis introduced by Cukierman and Meltzer (Citation1986). However, we find mixed results when taking the leverage effect into consideration.
For future research, it would be of considerable interest to allow for time-varying parameters in both GARCH and stochastic volatility specifications and evaluate the effectiveness of these models in modelling inflation dynamics. In addition, the interaction between CPI inflation and macroeconomic variables has been a topic of great interest. As a result, incorporating macroeconomic variables into present models and extending them to multivariate GARCH and stochastic volatility variants would also be desirable.
Acknowledgments
I would like to thank Shinichi Nishiyama and Shuhei Takahashi for helpful comments and suggestions. I am also grateful to the editors and two anonymous referees for their useful comments and suggestions. All the remaining errors are, of course, my own.
Disclosure statement
No potential conflict of interest was reported by the authors.
Notes
1 Since no consensus on the terminologies between inflation uncertainty and inflation volatility has been reached in the literature, these two terms will be used interchangeably in this article.
2 They demonstrate that due to a lack of commitment, the monetary authorities are highly likely to generate inflation surprises by carrying out an expansionary monetary policy to stimulate the economy when facing a high inflation uncertainty environment. Thus, an increase in inflation uncertainty raises inflation. In contrast, Holland (Citation1995) argues that in such a high inflation uncertainty environment, the state bank, owing to its stabilization incentive, should implement a tightening monetary policy to diminish the welfare cost of high volatile inflation, and thus lower the inflation rate.
3 The GARCH model is proposed by Bollerslev (Citation1986) to generalise the earlier study on autoregressive conditional heteroscedasticity model by Engle (Citation1982).
4 Note that another widely used model selection, namely the Bayesian information criterion (BIC) introduced by Schwarz (Citation1978) is shown to be asymptotically convergent to the the logarithm of the Bayes factor (see, e.g., Kass & Raftery, Citation1995). In other words, both the Bayes factor and BIC asymptotically choose the same candidate model. More specifically, it can be easily checked that
converges to zero as T goes to infinity. Here T is the number of observations, and the BIC under the model Ml with is computed as
where nl is the number of estimated parameters, and is the maximum likelihood estimate value.
5 For simplicity, we drop out the notation M for the model in the expression.
6 Chan and Eisenstat (2015) show that the posterior density is the zero-variance density for estimating the marginal likelihood. Unfortunately, we cannot use this density as a proposal due to its unknown normalizing constant.
7 The advanced economies, as defined in this study, are ones with GDP per capita over 40,000 US dollars under the IMF’s list of countries by nominal GDP per capita. In addition, we only include countries with at least 50 consecutive years of data for CPI. The resulting data contains 18 economies: the G7 countries (Canada, France, Germany, Italy, Japan, the United Kingdom, and the United States), Australia, Austria, Belgium, Denmark, Finland, Luxembourg, the Netherlands, New Zealand, Norway, Sweden, and Switzerland. This is almost the same list considered by Uribe and Schmitt-Grohé (Citation2017). In their study, they characterise the rich economies as all of those with purchasing power parity (PPP)-converted GDP per capita in 2005 U.S. dollars above 25,000.
8 We also perform: (1) an ADF test with drift; (2) an ADF test with both drift and trend; and (3) a PP test with trend. The findings also suggest that the inflation rates are stationary.
9 We report the marginal likelihoods of the models because the Bayes factor is computed based on the marginal likelihoods. This is also a common method used by researchers.
10 The estimation method is presented in Appendix A and Appendix B.
11 The negative estimate of the asymmetric effect is also found in the previous literature (see, e.g., Abbas Rizvi et al., Citation2014).
12 Similar conclusions are drawn for the remaining countries. This means the posterior estimates of an asymmetric effect parameter θ is in line with the ranking of the marginal likelihoods. The results can be found in the Appendix C.
13 Interested readers are referred to Geweke and Amisano (Citation2011) for a more in-depth consideration of the log predictive score using the expanding samples and rolling samples.
14 The results for the other countries can be found in the Appendix D.
15 We found no improvement in the density prediction of the GARCH-GJR (SV-L) over the GARCH (SV) for countries like France, Germany, Italy. This finding is in line with the result from the model comparison using the log marginal likelihood. To summarise, the relevance of the asymmetric effect is shown to be mixed.
16 This approach has been used recently, for example, by Chan and Jeliazkov (2009) and McCausland et al. (Citation2011) for linear state space models, and by McCausland (Citation2012) and Djegnéné and McCausland (Citation2015) for nonlinear state space models.
17 We choose the mode of conditional density as a point to expand since it can be quickly computed by the Newton-Raphson method. Interested readers are referred to Kroese et al. (Citation2013) for a more detailed explanation.
18 From EquationEquation (6)(6)
(6) , we can derive the log-conditional density of π given the parameter α and the volatility ht as:
19 Interested readers are referred to Kroese and Chan (Citation2014) for a detailed proof.
References
- Abbas Rizvi, S. K., Naqvi, B., Bordes, C., & Mirza, N. (2014). Inflation volatility: An Asian perspective. Economic Research-Ekonomska Istraživanja, 27(1), 280–303. https://doi.org/10.1080/1331677X.2014.952090
- Berument, M. H., Yalcin, Y., & Yildirim, J. (2012). Inflation and inflation uncertainty: A dynamic framework. Physica A: Statistical Mechanics and Its Applications, 391(20), 4816–4826. https://doi.org/10.1016/j.physa.2012.05.003
- Bollerslev, T. (1986). Generalized autoregressive conditional heteroskedasticity. Journal of Econometrics, 31(3), 307–327. https://doi.org/10.1016/0304-4076(86)90063-1
- Chan, J. C. (2017). The stochastic volatility in mean model with time-varying parameters: An application to inflation modeling. Journal of Business & Economic Statistics, 35(1), 17–28. https://doi.org/10.1080/07350015.2015.1052459
- Chan, J. C., & Eisenstat, E. (2015). Marginal likelihood estimation with the cross-entropy method. Econometric Reviews, 34(3), 256–285. https://doi.org/10.1080/07474938.2014.944474
- Chan, J. C., & Grant, A. L. (2016a). Modeling energy price dynamics: GARCH versus stochastic volatility. Energy Economics, 54, 182–189. https://doi.org/10.1016/j.eneco.2015.12.003
- Chan, J. C., & Grant, A. L. (2016b). On the observed-data deviance information criterion for volatility modeling. Journal of Financial Econometrics, 14(4), 772–802. https://doi.org/10.1093/jjfinec/nbw002
- Chan, J. C., & Jeliazkov, I. (2009). Efficient simulation and integrated likelihood estimation in state space models. International Journal of Mathematical Modelling and Numerical Optimisation, 1(1/2), 101–120. https://doi.org/10.1504/IJMMNO.2009.030090
- Cukierman, A., & Meltzer, A. H. (1986). A theory of ambiguity, credibility, and inflation under discretion and asymmetric information. Econometrica, 54(5), 1099–1128. https://doi.org/10.2307/1912324
- Daal, E., Naka, A., & Sanchez, B. (2005). Re-examining inflation and inflation uncertainty in developed and emerging countries. Economics Letters, 89(2), 180–186. https://doi.org/10.1016/j.econlet.2005.05.024
- Djegnéné, B., & McCausland, W. J. (2015). The HESSIAN method for models with leverage-like effects. Journal of Financial Econometrics, 13(3), 722–755. https://doi.org/10.1093/jjfinec/nbt027
- Engle, R. F. (1982). Autoregressive conditional heteroscedasticity with estimates of the variance of United Kingdom inflation. Econometrica, 50(4), 987–1007. https://doi.org/10.2307/1912773
- Friedman, M. (1977). Nobel lecture: Inflation and unemployment. Journal of Political Economy, 85(3), 451–472. https://doi.org/10.1086/260579
- Geweke, J., & Amisano, G. (2011). Hierarchical Markov normal mixture models with applications to financial asset returns. Journal of Applied Econometrics, 26(1), 1–29. https://doi.org/10.1002/jae.1119
- Glosten, L. R., Jagannathan, R., & Runkle, D. E. (1993). On the relation between the expected value and the volatility of the nominal excess return on stocks. The Journal of Finance, 48(5), 1779–1801. https://doi.org/10.1111/j.1540-6261.1993.tb05128.x
- Grier, K. B., & Perry, M. J. (1998). On inflation and inflation uncertainty in the G7 countries. Journal of International Money and Finance, 17(4), 671–689. https://doi.org/10.1016/S0261-5606(98)00023-0
- Holland, A. S. (1995). Inflation and uncertainty: Tests for temporal ordering. Journal of Money, Credit and Banking, 27(3), 827–837. https://doi.org/10.2307/2077753
- Jeffreys, H. (1998). The theory of probability. Oxford University Press.
- Kass, R. E., & Raftery, A. E. (1995). Bayes factors. Journal of the American Statistical Association, 90(430), 773–795. https://doi.org/10.1080/01621459.1995.10476572
- Keskek, S., & Orhan, M. (2010). Inflation and inflation uncertainty in Turkey. Applied Economics, 42(10), 1281–1291. https://doi.org/10.1080/00036840701721273
- Kim, S., Shepherd, N., & Chib, S. (1998). Stochastic volatility: Likelihood inference and comparison with ARCH models. Review of Economic Studies, 65(3), 361–393. https://doi.org/10.1111/1467-937X.00050
- Kontonikas, A. (2004). Inflation and inflation uncertainty in the United Kingdom, evidence from GARCH modelling. Economic Modelling, 21(3), 525–543. https://doi.org/10.1016/j.econmod.2003.08.001
- Koopman, S. J., & Hol Uspensky, E. (2002). The stochastic volatility in mean model: Empirical evidence from international stock markets. Journal of Applied Econometrics, 17(6), 667–689. https://doi.org/10.1002/jae.652
- Kroese, D. P., & Chan, J. C. (2014). Statistical modeling and computation. Springer.
- Kroese, D. P., Taimre, T., & Botev, Z. I. (2013). Handbook of Monte Carlo methods. John Wiley & Sons.
- Lucas, R. E. (2000). Inflation and welfare. Econometrica, 68(2), 247–274.
- McCausland, W. J. (2012). The HESSIAN method: Highly efficient simulation smoothing, in a nutshell. Journal of Econometrics, 168(2), 189–206. https://doi.org/10.1016/j.jeconom.2011.12.003
- McCausland, W. J., Miller, S., & Pelletier, D. (2011). Simulation smoothing for state–space models: A computational efficiency analysis. Computational Statistics & Data Analysis, 55(1), 199–212. https://doi.org/10.1016/j.csda.2010.07.009
- Omori, Y., Chib, S., Shephard, N., & Nakajima, J. (2007). Stochastic volatility with leverage: Fast and efficient likelihood inference. Journal of Econometrics, 140(2), 425–449. https://doi.org/10.1016/j.jeconom.2006.07.008
- Schwarz, G. (1978). Estimating the dimension of a model. The Annals of Statistics, 6(2), 461–464. https://doi.org/10.1214/aos/1176344136
- Stock, J. H., & Watson, M. W. (2007). Why has U.S. inflation become harder to forecast? Journal of Money, Credit and Banking, 39, 3–33. https://doi.org/10.1111/j.1538-4616.2007.00014.x
- Uribe, M., & Schmitt-Grohé, S. (2017). Open economy macroeconomics. Princeton University Press.
- Yu, J. (2002). Forecasting volatility in the New Zealand stock market. Applied Financial Economics, 12(3), 193–202. https://doi.org/10.1080/09603100110090118
Appendix A.
Hyper-parameters setting
A.1. GARCH-type models
We assume independent priors for α and the group of parameters as follows:
We impose the inequality to induce stationarity. For the GARCH-GJR, the coefficient of leverage ρ is assumed to have a uniform prior conditional on Δ. As for the volatility feedback parameter λ under the GARCH-M, we assume a normal distribution:
Following Chan and Grant (Citation2016a), we consider the noninformative priors as follows:
and
A.2. SV-type models
Similar to the GARCH-type models, we use independent priors for α, αh, ρh, and as follows:
Here is the inverse-gamma distribution. We also impose a restriction
to induce stationarity. As for the SV-L, we assume a uniform prior for the leverage parameter ρ. For the SV-M, we assume that the volatility feedback parameter λ follows a normal distribution
To obtain similar dynamics for the inflation volatility as in the GARCH variants, we also set noninformative priors for the parameters of the SV models:
and
Appendix B.
Bayesian estimation
In this section, we provide a brief discussion of Bayesian estimation for our models. Two classes of time-varying models are estimated by the Markov chain Monte Carlo (MCMC) methods. More specifically, we generate Markov samplers to draw from the posterior distributions and use these independent draws to calculate moments of interest such as the log marginal likelihoods, the posterior means, and standard deviations.
B.1. GARCH models
For the GARCH models, following Chan and Grant (Citation2016a), we draw from the posterior distributions using the Metropolis-Hastings (MH) algorithm. We group parameters into blocks and sequentially sample from conditional distributions. As an example, let us consider the standard GARCH model. We first divide the parameters into two blocks: (1) α and (2) We then sequentially sample from the two conditional distributions
and
To this end, we resort to the Metropolis-Hasting algorithm for sampling as these conditional distributions are not standard,. More specifically, we use a Gaussian distribution centred at the sample mean
with the variance
where s2 is the sample variance, to draw α. For a block κ, we use a Gaussian distribution with the mean and the covariance matrix being set to be the mode of
and the outer product of the scores, respectively. For the two remaining GARCH variants, the algorithm remains unchanged, but additional blocks are required to deal with additional parameters.
B.2. Stochastic volatility models
For stochastic volatility variants, a main challenge is to jointly draw the log-inflation volatilities h = conditional on the observed data and model parameters. To this end, we employ the acceptance-rejection Metropolis-Hastings algorithm proposed by Chan (Citation2017) to draw h. A main feature of this approach is the use of fast band and sparse matrix routines which take advantage of the specialty of the problem, namely, that the Hessian of the log-conditional density of h contains only a few non-zero elements along the diagonal band. In general, this approach has proved to be more efficient than the conventional Kalman filter.Footnote16
B.2.1. Standard stochastic volatility model
First, we discuss the algorithm for the standard stochastic volatility specification. For convenience, we refer to this algorithm as the baseline one. Let =
be the observed data. The posterior draws can then be attained by sequentially sampling from:
In the first step, we need to jointly draw log-inflation volatilities h, which is a key ingredient to implement the acceptance-rejection Metropolis-Hastings algorithm. The fundamental idea is to approximate the target using a Gaussian density. Note that from Bayes’ Theorem, we have:
Hereinafter, we derive the explicit expressions for the two conditional densities, and
The former density
can be approximated by a Gaussian distribution in h. To this end, we approximate the conditional density
around a point
which is chosen to be the mode of
by using a second-order Taylor expansion to obtain:Footnote17
(15)
(15)
where a1 is a constant, and
and
are the gradient and the negative Hessian of the log-conditional density of πt evaluated at
respectively. Ft and Gt for all
are computed as follows:Footnote18
Next, we consider the conditional density It is proved that this density is Gaussian (see, e.g., Chan & Grant, Citation2016b). Let
be the following matrix:
Then, we can rewrite the volatility equation of the standard stochastic volatility model in (7) as follows:
(16)
(16)
where
and
Since the determinant of
is one, the matrix is invertible regardless of the value of ρh. Therefore, from EquationEquation (16)
(16)
(16) , we obtain:
where
Hence, the log-conditional density can be written as follows:
(17)
(17)
where a2 is a constant parameter independent of h. Finally, combining EquationEquations (15)
(15)
(15) and Equation(17)
(17)
(17) , we obtain the following result:
(18)
(18)
where a3 and a4 are constant parameters independent of h,
and
The expression in (18) can be shown as the log-kernel of
with
Footnote19 In other words, we can approximate the joint conditional density
by the Gaussian density with the mean vector
and variance vector
It is easy to check that
is a tridiagonal matrix, and hence, we can quickly obtain
by solving the linear system
for x without computing the inverse matrix
Moreover, it is quite fast to sample from the density
by using the precision sampler introduced in Chan and Jeliazkov (Citation2009).
The posterior draws for α, αh, and can be easily obtained as their corresponding conditional distributions are Gaussian:
where
and
where
and
Here,
and
are defined as
and
where
Finally, we can draw from by employing an independence-chain Metropolis-Hastings algorithm with the proposal density
where
and
with
and
B.2.2. Stochastic volatility in mean model
Some modifications of the baseline algorithm are required in order to estimate the stochastic volatility in mean model. First, the log-conditional density of πt given parameters and the volatility ht now become:
Hence, the first and second derivatives of this log-conditional density with respect to ht are as follows:
We can then sample h from the joint distribution as in Step 1 of SV model. A second adjustment is that in Step 2 of the baseline algorithm, we need to jointly draw from
This can be done easily since the joint conditional distribution is Gaussian. Specifically, we define
as
The conditional distribution is shown as follows:
where
and
Here,
and
are defined as
B.2.3. Stochastic volatility with leverage
To fit this stochastic volatility specification, a few modifications are required. First, we need to draw from where h now is defined as
which means h is of length T + 1. The conditional density of πt given parameters and ht,
is as follows:
Hence, the log-conditional density is as follows:
To sample h, we go through a similar procedure as in the baseline algorithm with only slight changes. Second, we need one extra step to sample ρ from the conditional distribution It can be checked that the log-conditional distribution of ρ is as follows:
Here, is a prior distribution; m1, m2, and m3 are defined as
and
The remaining parameters can be drawn similarly as the standard stochastic volatility model.
Appendix C.
Estimation results for all 18 countries
In this section, we present the estimated results for the all 18 countries. Because of space limit, we only report the posterior estimates for the leverage effect and volatility feedback. The results are presented in . It is clear that the posterior estimates of volatility feedback λ for both the GARCH-M and SV-M are all positive and statistically significant, which demonstrates that inflation volatility has a positive impact on the inflation rate. However, we find mixed results when taking the leverage effect into account. These findings are in line with the results of the model comparison using the marginal likelihood.
Table C.1. The posterior estimates of the leverage effect and volatility feedback for other countries. The numbers in parentheses are numerical standard errors.
Appendix D.
Forecasting comparison results for all 18 countries
This section provides the forecasting comparison results for all 18 countries. The results are presented in for the expanding samples and for the rolling samples. It is clear that the SV specifications surpass their GARCH counterparts in performing the density forecast of the inflation rate for both the expanding samples and rolling samples. This result is consistent with the finding of the model comparison using the log marginal likelihood.
Table D.1. Log predictive score of two classes of volatility models for all 18 countries (Expanding samples).
Table D.2. Log predictive score of two classes of volatility models for all 18 countries (Rolling samples).