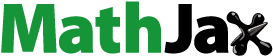
Abstract
Tourism is a rapidly rising sector that contributes significantly to economies. In this paper, we apply a bootstrap full-sample causality test, parameter stability test, and quantile-on-quantile approach test so as to examine the relationship between consumer confidence index (CCI) and outward tourism expenditure (OTE) for the period between 1998:Q1 to 2021:Q4. The results of the findings reflect that CCI tends to exert a positive impact on the OTE in most of the quantiles. This essentially implies that consumer confidence in China can act as a compound for outbound tourism expenditure in nature. Therefore, it can be affirmed that the OTE tends not to have an effect on the CCI, thus extending the implication that an alteration in the private outbound tourism spending in most likely to be insufficient for the modification of the factor of consumer confidence. To ensure the robustness of the results, we have employed the quantile-based granger causality to investigate the causal relationship in quantile between CCI on the OTE. The results of this study tend to educate the foreign tourism policymakers, while at the same time making forecasts of the tourism arrivals from China. Policymakers can then plan their tourism strategies, ideally including the CCI.
1. Introduction
In the last few decades, tourism is becoming more significant as revenue and foreign exchange earnings. The tourism demand literature is skewed toward studies that focus exclusively on inbound tourism, ignoring outbound tourism. This is due to the economic benefits accrued from inbound travel receipts instead of the capital flight incurred from outbound tourism. However, it is critical for domestic and international tourism stakeholders to understand the factors that influence outbound tourism demand to be well-versed in external competition. Tourism is also referred to as a “smokeless business”, as it provides a considerable boost to the socioeconomic development of a country (Athari et al., Citation2021; Huang & Wei, Citation2018). As the perception of the consumers tends to fluctuate and change over time, the confidence index also reflects these changes and shifts according to an individual’s financial condition and the corresponding macroeconomic environment (Chatziantoniou et al., Citation2016; Tachochalalai & Romprasert, Citation2019). It is noteworthy that the consumer confidence shows changes primarily due to the uncertainty in the economic or political conditions and particularly evident with the preservation of safety, e.g., epidemiological situation.
In addition to this, the variations in the consumer confidence index (CCI) might also influence the demand for travel (Teyssier, Citation2012). The presence of consumer confidence in the present and the future conditions of an economy would ideally provide critical insights into why people decide to pursue travelling (Dogru, Citation2016). It is observed that consumers will tend to increase their spending on tourism particularly when they are optimistic about their financial position, as well as the macroeconomic situation (Godil et al., Citation2020; Li et al., Citation2010). On the other hand, visitors who have little confidence in their career prospects are likely to spend less on vacations, as well as shopping, thus resulting in a decline in the spending on tourism (Almeida & Garrod, Citation2017). In addition to this, the emotional side of things, or in other words the ‘animal spirits’, tend to exert an influence on the decision making capabilities of the economic agents. This can essentially predict the trends that are to follow in the aspect of consumer spending as well as tourism expenditure (Dogru, Citation2016; Ludvigson, Citation2004).
One must acknowledge that the economic implications presented by the tourism industry, in both the origin and the destination countries are of the utmost importance to the society (Jin et al., Citation2018). Therefore, the expansion of the tourism industry has given way to a considerable amount of economic growth, that too on a global level, specifically when it comes to the emerging economies that can bank on their ample natural features when attracting the attention of tourists. Thus, we can affirm that consumer confidence tends to have a definite impact on the demand for outbound tourism (Jucan & Jucan, Citation2013; Pham et al., Citation2017). Due to this, we can make a calculated deduction that consumer confidence may very likely be linked to the associated with outbound tourism expenditures (OTE) (Gholipour et al., Citation2022; Su et al., Citation2022). Therefore, when taking into consideration the implementation of the tourism growth strategies and its relevant policy measures, this particular study puts forth the suggestion that the authorities dedicated to travel in different counties must pay heed to the consumers’ confidence in the country of origin.
Since the year 2012, China has managed to establish itself as the world’s top market for tourism. Therefore, in this regard, its impact on the expenditures associated with outbound tourism are such that cannot be taken for granted (Xie & Tveterås, Citation2020). Sources of information have also affirmed that China has superseded the United States as the world’s largest consumer of outbound travel (Cavlek, Citation2020), and OTE went up to USD 254 billion in 2019. This is primarily due to the fact that Chinese tourists have exsperienced an increase in their purchasing power, and several tourist spots and locations have gone an extra mile to attract these tourists (Dogru, Citation2016). Having observed this, it has also been noticed that there is little research that has been undertaken regarding the impact of the CCI on the OTE. Moving on with the same context, the factor of CCI is deemed to be a viable economic indicator that is impacted by the current as well as the future state of the economy. Besides, the demand for tourism has shown a tremendous decline in different time periods that have been defined by a high CCI in China (Xie et al., Citation2021). It has also been observed that consumer confidence has reached an all-time low in periods where the global financial crisis has taken over the economy, specifically referring to the 2008 and COVID-19 pandemic (Gössling et al., Citation2020).
This study contributes to the extant literature in many ways. The first one of these is that even though there has been a plethora of research conducted on the effects of consumer confidence on the outbound tourism (Cavlek, Citation2020; Dragouni et al., Citation2016; Gholipour & Tajaddini, Citation2018), there has been negligible research that has been undertaken on the functionality of CCI, specifically in China’s outbound tourism market. Moreover, after the crisis that have been experienced in the past few years, the role and function of CCI has become even more critical for the policymakers, primarily because the customers have become increasingly irrational, solely relying on their personal psychological prompts and understanding when making holiday purchases (Kock et al., Citation2020; Lahiri et al., Citation2016). Therefore, as a result, this particular research tends to look into the relationship dynamics between CCI and OTE, specifically keeping China as a case in point, so as to better address this gap in the literature. Second, a vast chunk of the extant literature written on this discipline has primarily observed the causal relationship between CCI and OTE in a full-sample causality (Cavlek, Citation2020; Dragouni et al., Citation2016; Gholipour & Tajaddini, Citation2018; Gunter & Smeral, Citation2017). However, it is noteworthy that when the structural relationships of the considered variables tends to change or alters, the linkages between CCI and OTE may end up becoming time variant.
The purpose of this was to fill the gaps that reveal a dynamic causal relationship, and confirm the presence of structural changes in the series. Therefore, First, we have resorted to the employment of the bootstrap full-sample causality test, parameter stability test, and quantile-on-quantile approach test so as to examine the relationship between of CCI and OTE. This approach has led to the identification of the relationship between the variables placed in the different quantiles of the data. Moreover, the choice of this specific approach is strongly validated by the CCI data structure. Because CCI data is only provided monthly, there is a risk that high-frequency data fluctuations may be disregarded. Thus, studying high-frequency crescendos of the link between CCI and OTE is lost, especially when other conventional methodologies are used. Second, to ensure the robustness of the results, we have employed the quantile-based granger causality to investigate the causal relationship in quantile between CCI on the OTE. In this regard, the empirical results of the study have indicated that CCI tends to exert a positive impact on the OTE, that too in most of the samples that have been taken into consideration. This is then affirmed by the gravity model, this pointing towards the indication that the two variables that have been considered essentially move in the same direction. This has resulted in the realization that OTE tends to have no effect on the CCI in the economic situation of China which may occur in the future.
The remaining paper has been structured as follows. The literature review of the study has been displayed in segment 2. Next, model, methodology and data has been presented in segment 3, in order to explicate the association between CCI and OTE. Following this, empirical results have been presented in segment 4 and segment 5 provided detailed discussion of the results. Moving further, segments 5 presented conclusion, policy implication, and future research of the paper.
2. Literature review
Extensive literature has investigated tourism determinants of tourism but the literature between CCI and OTE is limited and lacks research consensus. According to (Wang et al., Citation2006), the consumption behavior of tourists is influenced by a wide range of elements that can be divided into economic restrictions, sociodemographic factors, trip-related factors, and psychographic traits. The evidence on economic restrictions overwhelmingly supports that income has a positive and considerable impact on visitor expenditure (Almeida & Garrod, Citation2017; Bernini et al., Citation2021). Other factors such as prices, ownership of economic assets, indicators of financial distress, and healthcare costs may have a substantial impact on spending, contributing to the formation of limits on leisure-time choices (Arain et al., Citation2020; Belenkiy & Riker, Citation2013; Gössling et al., Citation2020). Personal income, measured by GDP per capita, or average earnings, is one of the most significant motivations to take a vacation trip in the outbound tourism demand literature (Chi, Citation2016). (Motamed-Jahromi et al., Citation2017) found that capital earnings have a major impact on the decision to travel to other regions of the world for vacation. Household members tend to take fewer vacations abroad when the CCI is low, which reduces OTE
(Gunter & Smeral, Citation2017) observed that during the time periods when the economy was facing distress and the CCI was believed to be on the lower side, European consumers tended to avoid planning holidays abroad, and rather preferred to increase their consumption of basics and at the same time save up for the rainy days as well. Moreover, Gholipour and Tajaddini (Citation2018) also discovered that when the European people are going through a period of low confidence in the economic situation, they tend to cut back on the OTE, and vice versa. In this regard, the issue of a positive or favorable impact from CCI to the OTE, is not always highlighted or brought into the lime light. In a study, (Latzko, Citation2005) found that the consumers’ confidence does not highly correlate with the movements of tourism, especially when taking into account similar movements from Japan to Hawaii. In the same context, (Heim, Citation2010) also gave an explanation that the consumption of non-durable and perishable products and services, and the advent of outbound tourism, were factors that are essentially not driven by a strong consumer confidence. It is also noteworthy that the inbound tourism to places such as Greece has been unaffected by the changes in the consumer confidence in the origin countries of the tourists (Gounopoulos et al., Citation2012).
Furthermore, (Claveria & Torra, Citation2014) suggested that the consumer confidence in the countries of origin, such as the likes of France, United Kingdom, Germany, and Italy, has no influence or comportment on the demand for outbound tourism in Catalonia. Also, (Gorlevskaya, Citation2016) also gave evidence that the consumer confidence tends to leave a rather miniscule impact on tourism spending and expenditures on a global level. Data pertaining to travel and tourism also shows that the Chinese outbound tourists tend to decrease by a significant amount during the periods where there has been low disposable incomes and as a result low economic growth paired with a low CCI (Raza & Shah, Citation2017). At the same time, a study by (Zuo & Lai, Citation2020) propagated that when Chinese consumers tend to develop a more trusting relationship with their financial positions, they are able and willing to spend more on tourism related activities. According to a recent study by Gholipour et al. (Citation2022), a progressive improvement in China’s CCI leads to a positive impact on the outbound travel to 25 African countries. Nonetheless, in China, the perception of a positive association between CCI and OTE does not always stand accurate. For instance, it was observed that during the global financial crisis, CCI on a global level was at an all-time low, however, the Chinese outbound tourism told another story. At that time as well, not only the OTE experienced an increase, but the RMB also appreciated, thus making outbound and overseas travel more approachable for consumers (Li et al., Citation2010). Due to this high CCI, an increasing number of consumers in China have been looking to save and invest their money(Zipser et al., Citation2016), primarily because this may lead to a significant reduction in the expenses that they incur on outbound travelling. During periods of high CCI, the demand for Chinese tourism has also been observed to decline considerably (Xie et al., Citation2021).
It can be affirmed that despite the fact that there has been little research that has shown linkages between consumer confidence and outbound tourism (Cavlek, Citation2020; Dragouni et al., Citation2016; Gholipour & Tajaddini, Citation2018; Gunter & Smeral, Citation2017), there is no evidence of a study that has investigated this very correlation in the context of China. Therefore, we have set out to investigate the correlation between CCI and OTE in this study in order to analyze how the consumer confidence impacts the Chinese outbound tourism. Furthermore, a significant chunk of the extant literature has overlooked the structural breakdowns that exist in the time series, thus making it difficult to study the dynamic causal linkages between the CCI and OTE. Therefore, as a result, this research aims to fill in the gaps presented by the existing literature. For this purpose we have applied the quantile-on-quantile method and quantile-based granger causality tests to investigate the factor of causality between CCI and OTE, by taking into consideration the inconstant parameters. We can therefore make use of this test in order to evaluate if the Chinese consumer confidence behaves as a stimulating factor for outbound tourism spending and expenditures in China.
3. Model, methodology, and variable description
3.1. Model
For the purpose of this paper, the gravity model with its suitable extensions, has also been taken into consideration in order to thoroughly investigate the determinants that define the flow of tourism, while the independent variables comprise of the various features of the origin and destination countries, which are deemed to be either fixed or variable in nature (Athari et al., Citation2021). In addition to this, the model has been presented to be a stable, as well as a reliable measure for investigating the outbound travel (Balli et al., Citation2020; Ullah et al., Citation2019). This research also makes use of Rodrigue's gravity model, a measure that has been altered accordingly in order to assess China’s outbound tourism. As a result, the basic outlines of the gravity model for outbound tourism are as follows:
(1)
(1)
Here, China is characterized by the symbol the country of destination has been denoted by the symbol
while the time index is denoted by the symbol t. Moreover,
is shown as a constant, while the flow of tourists from China have been denoted by
to the destination country
during the time period denoted by
Moreover, the elements that can be used to generate the flows of outbound tourism from China have been articulated by
specifies the factors that are capable of attracting the inflows of international tourism to the destination country that is denoted by the symbol
In addition to this, the distance between China and the destination country that is chosen by the consumers is denoted by the symbol
It can be affirmed that CCI is a significantly critical factor that is capable of boosting the outbound tourism flows in the source country (Huang & Wei, Citation2018). The hypothesis for CCI and OTE is developed based on literature review and model. The hypothesis is developed as below:
H1: CCI might have a positive impact on OTE and vice versa.
3.2. Methodology
The methodology that this particular research has undertaken is twofold. First, we have resorted to the employment of the bootstrap full-sample causality test, parameter stability test, and quantile-on-quantile approach test so as to examine the relationship between CCI and OTE. This approach has led to the identification of the relationship between the variables placed in the different quantiles of the data. Moreover, the choice of this specific approach is strongly validated by the CCI data structure. Because CCI data is only provided monthly, there is a risk that high-frequency data fluctuations may be disregarded. Thus, studying high-frequency crescendos of the link between CCI and OTE is lost, especially when other conventional methodologies are used. Second, to ensure the robustness of the results, we have employed the quantile-based granger causality to investigate the causal relationship in quantile between CCI on the OTE. An in-depth analysis of each set of the methodologies taken into consideration has been provided henceforth.
3.2.1. Bootstrap full-sample causality test
The Granger causality test statistics are developed on the basis of the vector autoregression (VAR) model, and do not typically adhere to the traditional asymptotic distributions. As a result, when using the VAR model, Granger causality tests are often confronted with difficulty. (Toda & Yamamoto, Citation1995) suggested an improved Wald test that typically uses an expanded VAR model with I (1) variables, so as to achieve the usual asymptotic distribution of the Wald test. The Granger causality test, according to (Shukur & Mantalos, Citation2000), thus fails to produce effective and reliable results, especially when the residual-based bootsrap (RB) approach is not eligible to be applied. Furthermore, the likelihood ratio (LR) test tends to be completely applicable in small samples. Moreover, (Mantalos, Citation2000) examined the properties of the Wald, corrected-LR, and the bootstrap tests, in cointegration and non-cointegration processes using the Monte Carlo methods. Following this, they demonstrated that, regardless of the type of the cointegration, the bootstrap test performed best in the majority of the circumstances. As a result, the modified LR tests based on the RB method have been used in this study to investigate the causality between CCI and OTE. EquationEquation (1)(1)
(1) can therefore be used to construct the VAR (p) model, with a lag value of p that has been determined using the Schwarz Information Criterion (SIC):
(2)
(2)
Here, the function, is a white noise process. The VAR is extended with exogenous variables, so as to allow for the addition of unmodeled variables that are decided outside of the VAR (Nicholson et al., Citation2017). In the econometrics literature, these models are known as ‘‘VARX", but they are also known as the ‘‘transfer function" or the ‘‘distributed lag" models. Taking this into account, the primary goal of this paper is to closely investigate the interactions that occur between the CCI and OTE. EquationEquation (1)
(1)
(1) can thus be expressed as EquationEquation (2)
(2)
(2) , by translating the variable
to
and
(3)
(3)
3.2.2. Parameter stability test
One of the assumptions that has been designed for the full-sample test is that the parameters in the VAR model are constant. As a result, the outcomes will be zero, and the causality will be unstable, especially when the time series' structure tends to show changes (Balcilar & Ozdemir, Citation2013). It is therefore crucial to check whether the settings are stable. On one hand, in order to check the stability of the short-run parameters, we have used the tests of
and
These tests can be used to observe the short-term stability of the parameters. The
statistic, on the other hand, has been used to examine the long-term stability of the parameters (Nyblom, Citation1989).
3.2.3. Quantile on quantile approach
It has been sometime since the researchers studying this discipline have come to the realization that the Quantile Regression Analysis, originally coming from the teachings imparted by of (Koenker & Bassett, Citation1978), carries a significant amount of importance, particularly in terms of its comparative preeminence and competency over the linear regression model. However, even though it is able to make estimations and analyze the heterogeneous association at different quantiles of the conditional distribution, it is affirmed that the QRA is incapable of catering to any probability of varying relationships that are most likely caused by the level and type of uncertainty. Therefore, in order to concern specifically, Sim and Zhou suggested a unique approach, i.e., the Quantile on Quantile (QQ). Therefore, taking this very approach forward, we then demonstrated the association between CCI and OTE while using the QQ methodology for this paper. It is noteworthy that this approach is deemed to be proficient in terms of providing a detailed analysis at varying quantiles of the conditional distribution. Therefore, the nonparametric model that is primarily based on the quantile regression must ideally be put forth in the following function;
(4)
(4)
Here, denotes the outward tourism expenditure during the time period t; while
can take that represent the variable consumer confidence index, which can be captured through (CCI), at day
Moreover,
is the
th quantile of the conditional distribution of
while
is a quantile error term, whose conditional
th quantile may be deemed to be equal to zero. For further details on the methodology, interested readers can pursue.
3.2.4. Quantile-based granger causality test
Our analysis based on the Wavelet based quantile on the Quantile analysis has been further complimented by taking into account the quantile-based granger causality. Based on the (Granger, Citation1996), if a series does not make a viable contribution towards predicting another series
then it is affirmed that
does not granger-cause
In more mathematical terms, let there be an explanatory vector
and
which is the prior information set of
The mathematical equation for the null hypothesis of granger non-causality from
to
is therefore given as follows:
(5)
(5)
3.3. Variable description
When taking into account the data pertaining to this research, we have taken monthly data of consumer confidence index (CCI) and outbound tourism expenditure (OTE) from 1998:Q1 to 2021:Q4. It was observed that after the Asian financial crisis that was initiated from Thailand in July 1997, there was a slowdown in the Chinese economy, which then lead to widespread uncertainity amongst the people. This eventually resulted in a dramatic decline in the consumer confidence. CCI has been referred to in this study as a measure to people’s approach and attitudes, along with their expectations about the economy, paired with their income levels as well. The index that has been developed ranges from 0 to 200, with 0 showing a “very pessimistic” scenario, while 200 representing “very optimistic” circumstances. In this regard, the greater the extent of CCI, the more positive the consumer is expected to be regarding the future of the economic conditions and the amount of income that they may have to spend, and vice versa. The Chinese OTE hit a considerably low point in the year 1998, as it was a newly emerging outbound travel market back then. This could primarily be due to the unstable economic situation that China was in at that point in time, as stated by the historians keeping who analyzed the economic conditions in China at the time (Zhang & Heung, Citation2002).
The trends and transform series of the selected variables that have been observed in and . It can be observed that the CCI experiences a dramatic increase after China took oath with the World Trade Organization (WTO), in the year 2001. However, at the same time, the OTE still shows a slow rising trend (Guo et al., Citation2007). One of the factors influencing this slow rise could be the outbreak of the Severe Acute Respiratory Syndrome (SARS) disease in the year 2002, which caused a drop in the OTE, and the consumer trust was severely damaged. According to (Ayob & Masron, Citation2014), concerns regarding the wellbeing and safety contributed to the drop in the level of OTE at the time. Then, in the year 2004, the stock market in China experienced a considerable level of instability. It was at this time that the Shanghai and Shenzhen component indexes experienced a sharp decline, thus causing the consumers to become cautious about how and where to spend their money, ultimately faltering their confidence in the economic conditions of the country. At the same time, the OTE remained at a low level as well. Then, in the year 2013-2014, the OTE from China was observed to be more prominent and evident.
As the CCI experienced an increase, surpassing that of Germany and the United States in terms of the OTE, it can be affirmed that it was perhaps catapulting to a top position around the globe. Then in the year 2015, due to the economic uncertainity that was experienced on a global level, it was seen that China’s economic development also started to decline. At the same time, the OTE was also showing a declining trend, along with a low CCI. Both the CCI and the OTE during this time period, were observed to decline which then continued towards the Sino-US trade war in 2017–18. After this time period, the emergence of the novel Covid-19 virus also shattered the consumer confidence, causing a tremendously dramatic decline in the OTE in the year 2020 (Gössling et al., Citation2020). The CCI and the OTE on the other hand do not move at the same rate. That is to say that when the global financial crisis began in the fall of the year 2008, and the global economy went into a recessionary phase, the CCI also fell rather quickly during this time period. However, because there was so appreciation of the RMB during this time period, travelling outside of China was a relatively inexpensive phenomenon, and at the same time, the OTE kept on rising even amidst a global crisis (Li et al., Citation2010). Following this era, the economic development was again hampered by the European debt crisis, which began in the year 2010 (Hou et al., Citation2012), thus resulting in a slowdown of the CCI. The OTE climbed up again the year 2011-2012, as the RMB experienced an appreciation. As a result of these developments, the value of RMB might have affected the OTE, and its eventual appreciation would have caused the OTE to incline.
The presents a summary of the values and figures for the CCI and OTE. Due to the element of positive skewness, the CCI and OTE both are observed to have right-skewed distributions. The Jarque-Bera test demonstrates that the CCI and OTE have non-normal distributions at a 1% significance level. In order to avoid any factor of heteroscedasticity, the variables are transformed by using natural logarithms (Qin et al., Citation2020; Su et al., Citation2020).
Table 1. Descriptive statistics for CCI and OTE.
4. Empirical results
In order to assess the factor of stability of the CCI and OTE, we have resorted to the use various tests, such as the Augmented Dickey-Fuller test (Dickey & Fuller, Citation1981), the Phillips-Perron test (Phillips & Perron, Citation1988), and the Kwiatkowski-Phillips-Schmidt-Shin (Kwiatkowski et al., Citation1992). The results of these extensive tests have been shown in , where it is evident that the four variables after the first differencing are deemed to be stable under the ADF, PP, and KPSS tests, and they are also I(1).
Table 2. Unit root tests.
As a result of these developments, we have established the VAR model by taking into account the first – order difference of all the variables and performing the Granger causality test in accordance. The above mentioned VAR model has been referred to in order to investigate, as well as analyze the causal relationships that exist between the variables under consideration, which are CCI and OTE. The optimal lag length of the two variables, according to SIC, is affirmed to be at a value of 4. Moreover, the null hypothesis which states that CCI does not Granger cause OTE, cannot be rejected at a 10% significant level, as also evident in . Due to these results, we can conclude that there is no Granger causality present from CCI to OTE, which refutes the previous research that has been carried out in this regard (Dogru, Citation2016; Su et al., Citation2022).
Table 3. Full-sample Granger causality tests.
Moving on, we can also observe that the entire sample is presumed to have a lack of structural changes in the time series, and also the casual linkages between the two variables is such that is consistent and stationary over the entire time period that has been taken into consideration (Balcilar et al., Citation2010). However though, when the structural breaks do experience an exit in the series, the level of causality between the CCI and the OTE is not consistent and reliable anymore. The parameter stability test can be used to govern the constancy of time series data. Therefore, as mentioned before, we resort to the use of the Sup-F, Ave-F, Exp-F, and L c tests in order to ensure parameter stability. According to , the null hypothesis of the parameter constancy is thus rejected for CCI significance level of 1% for the Sup-F and Exp-F tests, and at a 5% level of significance for the Ave-F tests. Moreover, the null hypothesis is also overruled and rejected at a 1% significance level for CCI when using the Sup-F, Ave-F, and Exp-F tests. Thus, as a result, the time series of the CCI and OTE can be therefore be deemed to be non-constant in nature. Furthermore, the significant Lc statistics show that the time series in the VAR model are unstable in nature. As a result, we can conclude that the full-sample causality test is unreliable due to the structural changes and the non-constant parameters.
Table 4. The parameter stability test.
When taking into account the structural changes, quantile-on-quantile approach aids in the establishment of a reliable bidirectional causal relationship between CCI and OTE. The values observed on the z-axis capture the effect of the nth quantile of CCI (OTE), on the nth quantile of OTE (CCI), at varying scaling coefficients. shows that CCI's strong positive effect on OTE is found in the upper quantiles. The positive effects are consistent with those that were keenly observed by Gholipour and Tajaddini (Citation2018) and Gholipour et al. (Citation2022).
Figure 3. (a): Q-Q estimates (CCI and OTE); (b): Q-Q estimates (CCI and OTE).
Source: Author's Estimation.
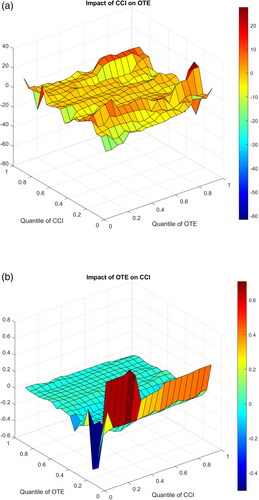
shows that OTE's does not induce CCI is most of the quantiles. Moreover, the changes observed in the OTE are not entirely able to give a plausible explanation for the way the CCI is moving, thus implying that the alterations in the outbound tourist expenditure have a negligible impact on the consumers’ expectations for the economic conditions in the future. This realization is in accordance with the observations shared by Heim (Citation2010), who was of the view that private consumption tends to have a negligible impact on the consumer confidence.
This study also uses the quantile-based Granger causality approach to ensure robustness to assess the causation between the variables. This technique enables us to analyze data for causality measures evaluated on multiple quantiles. describe the findings of the joint significance test in this respect. According to the test, the non-causal association is rejected by most of the quantiles [0.05 ∼ 0.95, 0.60 ∼ 0.80, 0.80 ∼ 0.95], demonstrating that one way relationship between CCI and OTE.
Table 5. Quantile causality test: CCI and OTE.
5. Discussion
The results of the study show us that the positive effects that CCI exerts on the OTE reflect that the consumer confidence in China is capable of acting as a catalyst for the outbound tourist expenditure. Data shows that after quite an unstable period in time, the stock market in China experienced a sharp rise since the first half of the year 2005. Moreover, Since January 2006, it has been witnessing an astonishingly positive bull market as well. It is widely believed that the bull stock market tends to seep in the element of consumer confidence in the economy and the income levels of the consumers (Chen, Citation2012). Therefore, during such a situation where the consumer confidence is high, households usually tend to possesses more disposable income, and at the same time spend more as well that too on luxury items as well as services such as the outbound tourism (Gholipour & Tajaddini, Citation2018).
In the year 2010, the Chinese outbound tourism was still continuing to experience growth, and the OTE was also at a position that would be considered to be at an all-time high. Now, because both CCI and OTE experienced a rise in the time period pertaining to 2010:Q1 to 2010:Q2, the positive relationship between the CCI and OTE can be recognized. Due to the stability in the economic growth, as well as the efficacious execution of the supply-side structural reforms in the year 2016, the consumers from China became increasingly confident regarding the macroeconomic situation that they came face to face with, back in the year 2016 (Khan et al., Citation2021). Due to the fact that the GDP has been high, the disposable income per Chinese resident has reached till 23,800 yuan, which is a staggering 6.3 percent from the preceding year. In addition to this, the CCI also increased by a significant amount between the time periods pertaining to 2016:Q2 and 2016:Q4, primarily because of the positive and favorable macroeconomic as well as personal financing conditions of the consumers. Typically, when the CCI is on the higher side, households will tend to reconsider and re-evaluate their disposable incomes and at the same time increase their spending on luxury items and services, which includes the need for tourist activities. As a result, an increase in the OTE, as propagated by Dragouni et al. (Citation2016). In the year 2016, the OTE coming from China experienced a sharp and significant decline. The value reached to a staggering 109.8 USD, which was a 5.1 percent increase as compared to the previous year. In the year 2019, the total GDP coming from China was still considered to be the highest in the world, with the GDP per capita reaching to a staggering 71,000 Yuan. In addition to this, the stable macroeconomic environment paired with the encouraging financials also remained unchanged and unhinged. At the same time, the CCI also remained at a high level during this time. With regards to this, according to Gholipour et al. (Citation2022), the Chinese consumers are such that they are usually willing to travel abroad when they feel optimistic about the economy of their country. In the year 2019, China experienced the highest number of outbound tourists, with the highest outbound tourism expenditure in the world.
Meanwhile, the results of OTE on CCI is also noteworthy that the confidence of the Chinese consumers varies, and mostly depends upon several factors. This includes the expectations regarding the present economic situation, the economic prospects, the level of income, and the expectation (Xie et al., Citation2021). Records and studies also tell us that after the global financial crisis, China’s consumer confidence continued to remain strong and resilient, a phenomenon that was mostly true due to the increase in the wages, along with a low level of unemployment (Zipser et al., Citation2016). As a result of these intricacies, we may affirm in the conclusion that a shift in the level of outbound tourism spending in China tends to have a little effect on the individuals’ consumer confidence, and hence, CCI is linked to the macroeconomic climate and a private financial situation. After analyzing the results, it can be concluded that the entire sample result accept the model hypothesis, as well as the earlier investigations in this regard (Gholipour et al., Citation2022;; Su et al., Citation2022). It makes logical sense to explain that when the parameters are not constant in nature, the bidirectional quantile-on-quantile approach is effective, as observed by the study of causality between the variables CCI and OTE.
6. Conclusion, policy implication and future research
For the purpose of this research, the study used the bootstrap full-sample causality test, parameter stability test, and quantile-on-quantile approach test so as to examine the relationship between CCI and OTE. Second, to ensure the robustness of the results, we have employed the quantile-based granger causality to investigate the causal relationship in quantile between CCI on the OTE. The result of full sample causality show that there is in no link between the CCI and OTE. Due to the realization that this result might be deemed to be unreliable and unstable because of the structural changes have been taken into account. Moving on, the quantile-on-quantile approach reveals that a positive trend from CCI to OTE. Therefore, we can come to a conclusion that the CCI is the main driver that stands sturdy behind the concept of outbound travel. That is to say that when the CCI is at a high level, consumers will raise their spending on outbound travel, and vice versa. Moreover, the conclusion is deemed to be generally consistent with the gravity model, which suggests that the CCI and OTE change in a lockstep. However, during the times where the economy has been under financial distress, the CCI and OTE have had a negative link with each other, primarily because a lower value of the CCI is unable to lead to a decline in the outbound tourism spending as the RMB has appreciated during such times. On the other hand, the empirical ultimatum of the result shows that the OTE has no effect on the CCI, which essentially means that the changes experienced in the outward tourism expenditure tend to have no impact on the consumer confidence. Moreover, the consumer confidence is not entirely influenced by the private consumption of the consumers, as it is a perspective of the future microeconomic environment and the financial conditions that are being faced at the time. It is noteworthy that is a foreign tourism administration, authority or market wants to gain some financial strength from a Chinese tourist, they should thoroughly study the relationship between CCI and OTE, particularly in China.
To begin with, when the development and the implementation of a targeted tourist policy is underway, especially for Chinese consumers, the consumer confidence in the macroeconomic scenario, and the financial standing should be a factor that must be taken into consideration. In more specific terms, during the times when the CCI is high, the government can extend its support for the protection and promotion of tourism, and at the same time create a unique travel plan for Chinese tourism. Secondly, during the times when the CCI is high, foreign markets can also chalk out specific and targeted marketed strategies for goods and services that are specifically aimed towards the travel interests of Chinese tourists. These essentially include encouraging entertainment and various shopping related activities. This also has certain consequences for Chinese travel agencies which deal with outbound tourism, specifically in terms of planning international holiday packages. That is to say that when there is a high level of CCI in China, these agencies may create appealing and exclusive travel packages for Chinese consumers only.
This study's findings need to be interpreted in the context of its limitations. For the purposes of this analysis, we solely employ macro (or country-level) datasets, which often do not include controls for individual variables. Using survey data acquired from individual visitors, it may be possible to investigate the link between the consumer confidence and outgoing tourism expenditures in future study. Aside from that, due to a lack of solid data, the econometric model only incorporated a restricted number of variables. Market research components, political considerations, especially health policy, which is a significant influencer of tourism demand, were excluded from the model. Researchers are invited to replicate the study in the future, assuming that a complete data collection spanning a greater number of nations becomes accessible. This will allow them to get a deeper knowledge of the factors that influence outbound tourism.
Disclosure statement
No potential conflict of interest was reported by the authors.
Correction Statement
This article has been republished with minor changes. These changes do not impact the academic content of the article.
References
- Almeida, A., & Garrod, B. (2017). Insights from analysing tourist expenditure using quantile regression. Tourism Economics, 23(5), 1138–1145. https://doi.org/10.1177/1354816616668108
- Arain, H., Han, L., Sharif, A., & Meo, M. S. (2020). Investigating the effect of inbound tourism on FDI: The importance of quantile estimations. Tourism Economics, 26(4), 682–703. https://doi.org/10.1177/1354816619859695
- Athari, S. A., Alola, U. V., Ghasemi, M., & Alola, A. A. (2021). The (Un) sticky role of exchange and inflation rate in tourism development: Insight from the low and high political risk destinations. Current Issues in Tourism, 24(12), 1670–1685. https://doi.org/10.1080/13683500.2020.1798893
- Ayob, N. M., & Masron, T. (2014). Issues of safety and security: New challenging to Malaysia tourism industry. In SHS Web of Conferences (Vol. 12, p. 01083).
- Balcilar, M., & Ozdemir, Z. A. (2013). The export-output growth nexus in Japan: A bootstrap rolling window approach. Empirical Economics, 44(2), 639–660. https://doi.org/10.1007/s00181-012-0562-8
- Balcilar, M., Ozdemir, Z. A., & Arslanturk, Y. (2010). Economic growth and energy consumption causal nexus viewed through a bootstrap rolling window. Energy Economics, 32(6), 1398–1410. https://doi.org/10.1016/j.eneco.2010.05.015
- Balli, F., Balli, H. O., Hasan, M., & Gregory-Allen, R. (2020). Economic policy uncertainty spillover effects on sectoral equity returns of New Zealand. Journal of Economics and Finance, 44(4), 670–686. https://doi.org/10.1007/s12197-020-09508-6
- Belenkiy, M., & Riker, D. (2013). Modeling the international tourism expenditures of individual travelers. Journal of Travel Research, 52(2), 202–211. https://doi.org/10.1177/0047287512461180
- Bernini, C., Emili, S., & Vici, L. (2021). Are mass tourists sensitive to sustainability? Tourism Economics, 27(7), 1375–1397. https://doi.org/10.1177/1354816620923212
- Cavlek, N. (2020). Chinese outbound tourism to central, eastern and southeast European countries. Südosteuropa Mitteilungen, 03, 51–60.
- Chatziantoniou, I., Degiannakis, S., Eeckels, B., & Filis, G. (2016). Forecasting tourist arrivals using origin country macroeconomics. Applied Economics, 48(27), 2571–2585. https://doi.org/10.1080/00036846.2015.1125434
- Chen, S.-S. (2012). Consumer confidence and stock returns over market fluctuations. Quantitative Finance, 12(10), 1585–1597. https://doi.org/10.1080/14697688.2011.565363
- Chi, J. (2016). Research note: Employment and wage sensitivity to tourism activities–the case of US tourist arrivals and expenditure in Hawaii. Tourism Economics, 22(1), 171–178. https://doi.org/10.5367/te.2014.0422
- Claveria, O., & Torra, S. (2014). Forecasting tourism demand to Catalonia: Neural networks vs. Time series models. Economic Modelling, 36, 220–228. https://doi.org/10.1016/j.econmod.2013.09.024
- Dickey, D. A., & Fuller, W. A. (1981). Likelihood ratio statistics for autoregressive time series with a unit root. Econometrica, 49(4), 1057–1072. https://doi.org/10.2307/1912517
- Dogru, T. (2016). Chinese propensity to purchase a vacation: The role of financial behavior and confidence in economy. Tourism: An International Interdisciplinary Journal, 64(2), 149–158.
- Dragouni, M., Filis, G., Gavriilidis, K., & Santamaria, D. (2016). Sentiment, mood and outbound tourism demand. Annals of Tourism Research, 60, 80–96. https://doi.org/10.1016/j.annals.2016.06.004
- Gholipour, H. F., Nunkoo, R., Foroughi, B., & Daronkola, H. K. (2022). Economic policy uncertainty, consumer confidence in major economies and outbound tourism to African countries. Tourism Economics, 28(4), 979–994. https://doi.org/10.1177/1354816620985382
- Gholipour, H. F., & Tajaddini, R. (2018). Sub-dimensions of consumer confidence and outgoing tourist expenditures. Anatolia, 29(3), 445–455.
- Godil, D. I., Sharif, A., Rafique, S., & Jermsittiparsert, K. (2020). The asymmetric effect of tourism, financial development, and globalization on ecological footprint in Turkey. Environmental Science and Pollution Research International, 27(32), 40109–40120.
- Gorlevskaya, L. (2016). Building effective marketing communications in tourism. Studia Commercialia Bratislavensia, 9(35), 252–265. Article 35. https://doi.org/10.1515/stcb-2016-0025
- Gössling, S., Scott, D., & Hall, C. M. (2020). Pandemics, tourism and global change: A rapid assessment of COVID-19. Journal of Sustainable Tourism, 29, 1–20.
- Gounopoulos, D., Petmezas, D., & Santamaria, D. (2012). Forecasting tourist arrivals in Greece and the impact of macroeconomic shocks from the countries of tourists’ origin. Annals of Tourism Research, 39(2), 641–666. https://doi.org/10.1016/j.annals.2011.09.001
- Granger, C. W. (1996). Can we improve the perceived quality of economic forecasts? Journal of Applied Econometrics, 11(5), 455–473. https://doi.org/10.1002/(SICI)1099-1255(199609)11:5<455::AID-JAE408>3.0.CO;2-E
- Gunter, U., & Smeral, E. (2017). European outbound tourism in times of economic stagnation. International Journal of Tourism Research, 19(3), 269–277. https://doi.org/10.1002/jtr.2108
- Guo, Y., Seongseop Kim, S., & Timothy, D. J. (2007). Development characteristics and implications of Mainland Chinese outbound tourism. Asia Pacific Journal of Tourism Research, 12(4), 313–332. https://doi.org/10.1080/10941660701760995
- Heim, J. (2010). The impact of consumer confidence on consumption and investment spending. The Journal of Applied Business and Economics, 11(2), 37–54.
- Hou, S., Li, B., Li, J., & Mao, H. (2012). Coping with European debt crisis: China’s fiscal policy effect and orientation. Strategic Management, 17(2), 13–21.
- Huang, S., & Wei, X. (2018). Chinese outbound travel: Understanding the socioeconomic drivers. International Journal of Tourism Research, 20(1), 25–37. https://doi.org/10.1002/jtr.2150
- Jin, C., Cheng, J., Xu, J., & Huang, Z. (2018). Self-driving tourism induced carbon emission flows and its determinants in well-developed regions: A case study of Jiangsu Province. China. Journal of Cleaner Production, 186, 191–202. https://doi.org/10.1016/j.jclepro.2018.03.128
- Jucan, C. N., & Jucan, M. S. (2013). Travel and tourism as a driver of economic recovery. Procedia Economics and Finance, 6, 81–88. https://doi.org/10.1016/S2212-5671(13)00117-2
- Khan, K., Su, C.-W., Xiao, Y.-D., Zhu, H., & Zhang, X. (2021). Trends in tourism under economic uncertainty. Tourism Economics, 27(4), 841–858. https://doi.org/10.1177/1354816620909608
- Kock, F., Nørfelt, A., Josiassen, A., Assaf, A. G., & Tsionas, M. G. (2020). Understanding the COVID-19 tourist psyche: The evolutionary tourism paradigm. Annals of Tourism Research, 85, 103053.
- Koenker, R., & Bassett, G. (1978). Regression quantiles. Econometrica, 46(1), 33–50. https://doi.org/10.2307/1913643
- Kwiatkowski, D., Phillips, P. C. B., Schmidt, P., & Shin, Y. (1992). Testing the null hypothesis of stationarity against the alternative of a unit root. How sure are we that economic time series have a unit root? Journal of Econometrics, 54(1–3), 159–178. https://doi.org/10.1016/0304-4076(92)90104-Y
- Lahiri, K., Monokroussos, G., & Zhao, Y. (2016). Forecasting consumption: The role of consumer confidence in real time with many predictors. Journal of Applied Econometrics, 31(7), 1254–1275. https://doi.org/10.1002/jae.2494
- Latzko, D. A. (2005). Economic conditions and Japanese tourism to Hawaii. Asia Pacific Journal of Tourism Research, 10(2), 151–156. https://doi.org/10.1080/10941660500135936
- Li, S., Blake, A., & Cooper, C. (2010). China’s tourism in a global financial crisis: A computable general equilibrium approach. Current Issues in Tourism, 13(5), 435–453. https://doi.org/10.1080/13683500.2010.491899
- Ludvigson, S. C. (2004). Consumer confidence and consumer spending. Journal of Economic Perspectives, 18(2), 29–50. https://doi.org/10.1257/0895330041371222
- Mantalos, P. (2000). A graphical investigation of the size and power of the Granger-causality tests in integrated-cointegrated VAR systems. Studies in Nonlinear Dynamics & Econometrics, 4(1), 1–18. https://doi.org/10.2202/1558-3708.1053
- Motamed-Jahromi, M., Fereidouni, Z., & Dehghan, A. (2017). Effectiveness of positive thinking training program on nurses’ quality of work life through smartphone applications. International Scholarly Research Notices, 2017, 1–6. https://doi.org/10.1155/2017/4965816
- Nicholson, W. B., Matteson, D. S., & Bien, J. (2017). VARX-L: Structured regularization for large vector autoregressions with exogenous variables. International Journal of Forecasting, 33(3), 627–651. https://doi.org/10.1016/j.ijforecast.2017.01.003
- Nyblom, J. (1989). Testing for the constancy of parameters over time. Journal of the American Statistical Association, 84(405), 223–230. https://doi.org/10.1080/01621459.1989.10478759
- Pham, T. D., Nghiem, S., & Dwyer, L. (2017). The determinants of Chinese visitors to Australia: A dynamic demand analysis. Tourism Management, 63, 268–276.
- Phillips, P. C., & Perron, P. (1988). Testing for a unit root in time series regression. Biometrika, 75(2), 335–346. https://doi.org/10.1093/biomet/75.2.335
- Qin, M., Su, C.-W., Xiao, Y.-D., & Zhang, S. (2020). Should gold be held under global economic policy uncertainty? Journal of Business Economics and Management, 21(3), 725–742. https://doi.org/10.3846/jbem.2020.12040
- Raza, S. A., & Shah, N. (2017). Tourism growth and income inequality: Does Kuznets Curve hypothesis exist in top tourist arrival countries. Asia Pacific Journal of Tourism Research, 22(8), 874–884. https://doi.org/10.1080/10941665.2017.1343742
- Shukur, G., & Mantalos, P. (2000). A simple investigation of the Granger-causality test in integrated-cointegrated VAR systems. Journal of Applied Statistics, 27(8), 1021–1031. https://doi.org/10.1080/02664760050173346
- Su, C.-W., Meng, X.-L., Tao, R., & Umar, M. (2022). Chinese consumer confidence: A catalyst for the outbound tourism expenditure? Tourism Economics, 1–22. https://doi.org/10.1177/13548166211065250
- Su, C.-W., Qin, M., Tao, R., Moldovan, N.-C., & Lobonţ, O.-R. (2020). Factors driving oil price——From the perspective of United States. Energy, 197, 117219. https://doi.org/10.1016/j.energy.2020.117219
- Tachochalalai, S., & Romprasert, S. (2019). Macroeconomic perspective-chinese president era affecting Chinese tourist demand: Case of Thailand. Asian Administration & Management Review, 2(1). https://ssrn.com/abstract=3368001
- Teyssier, N. (2012). How the consumer confidence index could increase air travel demand forecast accuracy?
- Toda, H. Y., & Yamamoto, T. (1995). Statistical inference in vector autoregressions with possibly integrated processes. Journal of Econometrics, 66(1–2), 225–250. https://doi.org/10.1016/0304-4076(94)01616-8
- Ullah, S., Kiani, A. K., & Imran, M. (2019). Pakistan’s trade and trading partners using gravity model: A static and dynamic panel data. Jinnah Business Review, 7(1), 11–19. https://doi.org/10.53369/NBNE4722
- Wang, F.-C., Hung, W.-T., & Shang, J.-K. (2006). Measuring the cost efficiency of international tourist hotels in Taiwan. Tourism Economics, 12(1), 65–85. https://doi.org/10.5367/000000006776387150
- Xie, G., Qian, Y., & Wang, S. (2021). Forecasting Chinese cruise tourism demand with big data: An optimized machine learning approach. Tourism Management, 82, 104208. https://doi.org/10.1016/j.tourman.2020.104208
- Xie, J., & Tveterås, S. (2020). Economic drivers for the Chinese tourists. Scandinavian Journal of Hospitality and Tourism, 20(2), 110–125. https://doi.org/10.1080/15022250.2020.1734076
- Zhang, H. Q., & Heung, V. C. (2002). The emergence of the mainland Chinese outbound travel market and its implications for tourism marketing. Journal of Vacation Marketing, 8(1), 7–12. https://doi.org/10.1177/135676670200800102
- Zipser, D., Chen, Y., & Gong, F. (2016). The modernization of the Chinese consumer. McKinsey & Company.
- Zuo, B., & Lai, Z. (2020). The effect of housing wealth on tourism consumption in China: Age and generation cohort comparisons. Tourism Economics, 26(2), 211–232. https://doi.org/10.1177/1354816619861843