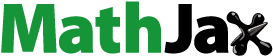
Abstract
The outbreak of the COVID-19 pandemic received widespread media attention, and global financial markets reacted strongly to the pandemic shocks. It is, therefore, worthwhile to detect the influence of media hype about COVID-19 and the pandemic index on stock market price returns. Utilising a newly developed non-linear ARDL model, our empirical outcomes show that the direct effect of the COVID-19 pandemic index on sectoral stock market returns is generally weak and significant only in the Energy, Financials, and Health Care sectors. In contrast to the direct effect of the COVID-19, we find that the media hype index pronouncedly affects sectoral stock market returns, with a significant negative effect in most sectors and with asymmetry. The dynamic asymmetric causality test has been applied for robustness check, where there is time-varying asymmetric causality from media hype to sectoral stock markets. These findings help investors with different investment horizons in emerging markets understand sector-level stock price dynamics and formulate investment strategies during the pandemic. Furthermore, market regulators should consider asymmetric effects over time when formulating strategies and making policy decisions.
1. Introduction
The coronavirus (COVID-19) pandemic outbreak received widespread media attention, and global financial markets reacted strongly to the pandemic shocks (Al-Awadhi et al., Citation2020; Ashraf, Citation2020; Haroon & Rizvi, Citation2020; Phan & Narayan, Citation2020; Su et al., Citation2021, Su, Yuan, et al., Citation2022; Topcu & Gulal, Citation2020; Youssef et al., Citation2021; Zhang et al., Citation2020). For instance, China’s stock market index reached a considerable decline of 7.72% on the first trading day after the Chinese traditional Spring Festival, and more than 3,000 stocks fell by the limit. In particular, the U.S. stock market even experienced 4 ‘circuit breaks’ on March 9, March 12, March 16, and March 18, 2020,Footnote1 which is more than ever in history. Therefore, market panic dominates media headlines at the beginning of the COVID-19 crisis. From the perspective of behaviour finance, news related to infectious diseases reported by the media may cause uncertainty and affect investor sentiment, further changing investors’ investment strategies and then influencing stock market volatility (Nepp et al., Citation2022).
Compared with the SARS pandemic in 2003 and the Global Financial Crisis in 2008, the shock of the COVID-19 pandemic crisis had a broader and deeper impact on financial markets (Youssef et al., Citation2021). Different from the era of past crises, the rapid growth of the internet nowadays allows news items to circulate faster than ever before. The media heavily influences investors’ insight into the current economy and expectations for the future, leading to changes in investment decisions and affecting the stock market’s volatility. For instance, Andrei and Hasler (Citation2015) show that the significance of media news in affecting investor sentiment and asset prices is becoming increasingly crucial. During the COVID-19 crisis, identifying the impact of media hype on financial market fluctuations and effectively avoiding the spread of financial risks by ensuring steady economic development has become a severe problem for policymakers in various countries (Su, Meng, et al., Citation2022). In light of this, this study proposes the hypothesis that media hype impacts the stock market and attempts to examine the effect of media hype on the stock market in the context of China.
This paper also considers four underlying factors, including the composite COVID-19 pandemic index, investor sentiment, monetary policy condition, and stock market panic index, when detecting the impacts of media hype on China’s stock market. Moreover, since stock market participants’ risk aversions and trading strategies differ significantly, their reactions to COVID-19’s negative and positive shocks on the stock market are likely to be heterogeneous (Nepp et al., Citation2022). Furthermore, policymakers might react differently to these shocks during the COVID-19 crisis (Cepoi, Citation2020). Therefore, COVID-19’s shocks and other potential factors may have asymmetric effects on the stock market. Therefore, the non-linear ARDL (NARDL) model proposed by Shin et al. (Citation2014) is utilised to investigate the asymmetric impacts of COVID-19 on the stock market. The main superiority of the NARDL approach is that it is more robust in small sampling cases, which is in line with the current duration of COVID-19. Another advantage of the NARDL estimation is that the cointegration test could be executed when the series is not I (2), which is much different from the traditional method. According to these merits, the NARDL approach can be utilised to investigate the asymmetric impacts of each factor on China’s stock market.
This paper’s main contribution is listed below. First, different from previous literature that often indicates the determinants of stock markets during the pandemic are primarily derived from the COVID-19’s direct impact (Ashraf, Citation2020; Topcu & Gulal, Citation2020), the current study devotes special attention to the role of the media hype in the stock market. To the best of our knowledge, while it has been commonly accepted that the media news plays an important role in information dissemination and the stock market is much sensitive to the new information, little study during the COVID-19 crisis has uncovered the influences of media hypes on the stock market. As a result, this paper presents new evidence on whether the media hype changes may be used to explain stock market price movement. Second, different from previous studies that mainly focus on the overall stock market’s reaction, we consider the industry-level heterogeneity and pay attention to heterogeneous reactions of sectoral stock markets. We do find that the media hypes differentially impact the sectoral stock markets. Third, we apply the NARDL approach to examine the asymmetric influence of the concerned factors on sectoral stock markets, which sheds fresh light on previous research that has only considered symmetric effects. This study’s asymmetric analysis may contribute to a better understanding of the impacts of media hypes on sectoral stock markets, which would have implications for the conduct of monetary policy and financial stability.
The rest of this research is structured below. Section 2 reviews the corresponding literature. The empirical method is presented in Section 3. The data is described in Section 4. The empirical findings are discussed in Section 5. Finally, Section 6 brings this paper to a close.
2. Literature review
Before the outbreak of COVID-19, existing literature examined the impact of public emergencies on economic and financial activities, such as public health incidents and natural disasters (i.e., SARS, H1N1, Ebola, Hurricane Katrina, and ‘3.11’ Earthquake) and claimed that natural disasters or significant public health incidents could cause investor’s panic in the financial market (Chen et al., Citation2018; Nippani & Washer, Citation2004). Therefore, the stock market volatility was significantly increased, and market returns were decreased (Ichev & Marinč, Citation2018).
In a recent study, during the COVID-19 crisis, lots of literature have also considered the impact of these major public health emergencies on the stock market and investigated the relationship between equity returns and the COVID-19 pandemic (Al-Awadhi et al., Citation2020; Ashraf, Citation2020; Topcu & Gulal, Citation2020). For example, He et al. (Citation2020) examine the influences of the COVID-19 pandemic on China’s stock market and claim that different sectors respond heterogeneously to the shock of the COVID-19 crisis. Zaremba et al. (Citation2020) investigate whether government interventions affect stock market returns during the spread of COVID-19, and they prove that interventions with non-pharmaceutical could significantly increase stock market return volatility. Moreover, Al-Awadhi et al. (Citation2020) utilise a panel data model to find that death cases and confirmed cases negatively affect stock returns. However, Ashraf (Citation2020) claim that stock markets are more significantly affected by the growing number of confirmed cases, while the impact of the increasing number of death cases on the stock market is weak.
In addition, there are a few articles that explain the theoretical relationship between media hype and the stock market during an extreme public health emergency. For instance, Andrei and Hasler (Citation2015) and Nepp et al. (Citation2022) highlight that investors’ insight into the current economic scenario and future expectations are primarily shaped by social media news, leading to changes in investment decisions and affecting the volatility of the stock market. Through event study methodology, Donadelli et al. (Citation2017) also find that disease-related news does have a significant influence on the stock prices. Haroon and Rizvi (Citation2020) find that the increasing stock market volatility is related to investors’ panic generated by the media coverage of COVID-19. Cepoi (Citation2020) employs the quantile regression model to examine the impact of the news outlets on the stock market and find that the coronavirus-related news causes stock market returns to decrease in the middle and superior quantiles. Moreover, as unexpected news reports increase, more information is more widely available to the public, and the market will also correct the impact of the news on its own (Phan & Narayan, Citation2020).
Furthermore, several kinds of research also consider the asymmetric reaction of the stock market during the pandemic crisis. It is well known that the presence of diverse investor profiles and asymmetric information in markets can result in differing responses to negative and positive shocks in prices. For example, Nepp et al. (Citation2022) note that since investors’ risk aversions and trading strategies in the stock market differ significantly, their reactions to the negative and positive shocks of COVID-19 media news on the stock market are likely to be heterogeneous. Besides, policymakers might also react differently to these shocks during the COVID-19 crisis (Cepoi, Citation2020). Therefore, COVID-19 shocks and media hype news may have asymmetric effects on the stock market.
Overall, recent studies have contributed to understanding COVID-19’s impact on the stock market. However, a body of studies has only focussed on the symmetric connections between COVID-19 and stock market reaction. Moreover, few researchers have looked into the role of COVID-19 media news in the stock market. Furthermore, many analyses disregard the heterogeneous response of sectoral stock market returns to the COVID-19 shock. These gaps must be explored in the current study. Therefore, this research attempts to use the NARDL approach to detect the underlying asymmetric influences of media hype on sectoral stock markets.
3. Methodology
The empirical methodology applied in this study is based on the NARDL approach (Shin et al., Citation2014), which could capture each explanatory variable’s asymmetric long-term and short-term effect on the explained variable. Taking into account the asymmetric long-term equilibrium equation, as follows:
(1)
(1)
where
is the explained variable,
is a random error term,
and
indicates the asymmetric long-term parameters, and
and
represent the explanatory variable’s cumulative sum processes of the negative and the positive changes, which are in the matrix form (
). The exact calculation procedure is as follows:
(2)
(2)
Moreover, the stock market might react heterogeneously under different levels of attention since that information is only included in prices when the public pays attention to the news information (Andrei & Hasler, Citation2015). Therefore, this study proposes the idea that media hype during the pandemic affects the stock market. Besides, we also follow the existing studies to control the impact of the investor sentiment (Molchanov & Stangl, Citation2018; Ni et al., Citation2015), the interest rate dynamic (Gu et al., Citation2021; Huang et al., Citation2016; Valera et al., Citation2017), and the market panic (Qadan et al., Citation2019) on the stock market.
Therefore, the asymmetric error correction model in the current paper can be created by embedding EquationEquation (1)(1)
(1) into the unconstrained linear ARDL (p, q) model, which can be written explicitly as:
(3)
(3)
where
represents the stock price returns of the industry represent an intercept,
is the aligned COVID-19 pandemic index,
represents the media hype,
stands for the investor sentiment,
denotes the interest dynamics, and
refers to the stock market panic index.
In order to reduce heteroscedasticity, this paper uses the logarithmic transformation of
and
in the empirical estimation. Since some observed data of
is negative,
is not transformed. At this stage, we can obtain the NARDL estimation approach to uncover the asymmetric impact of various factors on sector stock markets in China.
4. Data
The main dataset consists of China’s sectoral stock indices, the COVID-19 pandemic index, media hype index, investor sentiment index, interest rate dynamic, and equity market fear index. The sampling period spans from January 20, 2020 to December 30, 2020 and each data set is collected on a daily frequency. The beginning date depends on the availability of daily new confirmed COVID-19 cases in China. The selection of 11 major sectoral stock indices of China follows the Global Industry Classification Standard (GICS): Consumer Discretionary, Consumer Staples, Energy, Financials, Health Care, Industrials, IT, Materials, Real estate, Telecommunication Services, and Utilities. The motivation for considering the sectoral indices is that stock prices in different sectors may exhibit heterogeneity during pandemics and financial turmoil, depending on the nature of business, the cyclicality or counter-cyclicality of the sector, and the perceived risk of the industry. Therefore, we use 11 major sectoral stock indices in China to uncover the heterogeneous reactions of sectoral stock markets. Moreover, the PCA method separately constructs the COVID-19 pandemic index, investor sentiment index, and equity market fear index.Footnote2 Coronavirus Media Hype Index is employed as a proxy for the media hype. Besides, we use the Shanghai interbank offered rate for the interest rate dynamic. The media hype index is obtained from the Coronavirus Media Monitor (https://coronavirus.ravenpack.com/) and the other datasets are obtained from the Wind Info Economic Database of China. All variables are calculated as the log-returns in the empirical estimation.Footnote3
represents the trend of sectoral stock markets and explanatory variables. Specifically, it can be observed that the stock prices in Consumer Staples, Energy, Financials, Health Care, IT, and Materials sectors varied dramatically, particularly from the end of June to the start of July 2020, which could be attributed to the pandemic being under control and economic recovery is accelerating. We also noticed that the interest rate witnessed a downward trend in the first six months of 2020. The stock market panic gradually declined after reaching its peak in March 2020, indicating that investors were optimistic about the pandemic management. The extremely loose credit conditions and the high investor sentiment during this period make industry returns increasingly prominent. Moreover, the COVID-19 pandemic index showed a downward trend after the national lockdown measures. The media hype is relatively consistent during the data sampling period, suggesting that the COVID-19 spread is still receiving attention from the media ().
shows the summary statistics for each variable. The COVID-19 pandemic index has the largest standard deviation, indicating that COVID-19 changes dramatically during the sampling period. Among sectoral stock indices, the standard deviation of Consumer Staples and Health Care is greater than that of other industries, which implies that these sectors are much more volatile during the COVID-19 crisis. In addition, the kurtosis of COVID-19, Media hype, and VSI is greater than 3, indicating that there are leptokurtic distribution characteristics. Besides, the J-B statistics of most variables are significant at the 1 percent level and the skewness statistic is negative for a great majority of series, which indicates that the probability distributions exhibit non-normality and non-symmetric characteristics.
Table 1. Descriptive statistics for the variables under the current study.
5. Empirical result
5.1. NARDL estimations
Considering that the daily data might have structural fluctuations, we further employ the LM test (Lee & Strazicich, Citation2003) to detect multiple breakpoints to reveal their non-linear characteristics. In addition, modelling nonlinear relationships with linear models might cause spurious results, which leads to misleading economic policy decisions (Su, Pang, Umar, & Lobonţ, Citation2022). Accordingly, we also utilise the linearity test and nonlinear unit root test to examine the nonlinear feature of each variable. The corresponding results are shown in . The Wλ and HM statistics reject the null hypothesis of linearity for all variables, indicating that distributions are non-linearity in most scenarios. Besides, the two-break LM test results reveal break dates for each series, which are typically related to the stock market’s volatility.
Table 2. Linearity test, unit root test with structural breaks, and nonlinear unit root test results under the current study.
shows corresponding statistics and diagnostic results of both bound tests for different NARDL models. The FPSS statistics for most sectors exceed the upper critical value for each NARDL model. Moreover, the results of the tBDM test cannot reject the no cointegration null hypothesis, suggesting that there exists considerable evidence in support of the steady nonlinear cointegrating connections for all situations. Accordingly, the empirical model with asymmetries makes sense to detect dynamic influences of the COVID-19 pandemic index and media hype on the sectoral stock market.
Table 3. Results of bounds test for cointegration in NARDL model.
and present the final result of the NARDL estimation for each scenario, considering the direct influence of COVID-19 and the effect of media hype on 11 sectoral stock markets. Stepwise regression is used to determine the lag order for each equation. Besides, the error correction coefficients in and demonstrate that the model estimate is steady because they are significant and show negative signs. Moreover, the LM test results in both systems suggest that the null hypothesis of no sequence correlation cannot be rejected, showing that there exists no sequence correlation in both model estimations.
Table 4. Estimation result of the NARDL models with considering the impact of COVID-19 on sectoral stock market returns.
Table 5. Estimation result of the NARDL models with considering the impact of the media hype on sectoral stock market returns.
According to , we can notice that a majority of the estimated COVID-19 long-run coefficients are not significant. Unexpectedly, this result is different from existing literature, which claims that the stock market returns are significantly influenced by COVID-19 (Topcu & Gulal, Citation2020). These different conclusions may be related to the empirical method and sample selection. Besides, failing to consider the heterogeneity of the industry in these studies will also lead to different empirical results. However, we can see that the long-term coefficient of COVID-19 is positive signs in Health Care but negative signs in Energy and Financials. The beneficial effect of COVID-19 on Health Care, in particular, means that the demand for medical facilities and healthcare items was high during the COVID-19 crisis. Hospitals, businesses, and individuals have begun to compete for medical protective products such as medical masks (Fuchs et al., Citation2020), causing the Health Care industry to thrive and stock returns to climb. Furthermore, an increasing long-run effect of COVID-19 defeats the decreasing influence, implying that the Health Care sector is more vulnerable to favourable COVID-19 variations.
Likewise, the direct impacts of COVID-19 on Energy and Financials are significantly negative, which means that Energy and Financials sectors could rapidly respond to emergencies (Tao et al., Citation2022). One explanation for the energy sector is that the shutdown policy has led to a reduction in energy consumption during the COVID-19 crisis (IEA Global Energy Review, Citation2020), so the profitability of the energy sector has declined, and the stock price has fallen. An interpretation for the financial industry is that stock market trading becomes more uncertain during the COVID-19 crisis, causing much panic in the stock market. Consequently, ceteris paribus, growing stock market fear could lead to more cautious investments, and the ‘Waiting and seeing’ attitude prevails greater than in other contexts. Investment activities in the market are not active, resulting in a crash in the Financial sector. Besides, the long-term impact of a decrease in COVID-19 defeats the impact of an increase, suggesting that the Financials industry is more sensitive to negative changes in COVID-19 (Su, Pang, Umar, Lobonţ, Moldovan, Citation2022). The easing of the COVID-19 pandemic causes an increase in the financial industry’s stock prices more significantly than the impact of the deterioration on the financial industry.
Moreover, we also notice that the stock market returns are always significantly influenced by the investor sentiment in each sector. The coefficients of investor sentiment are positive, which means that positive changes in investor sentiment (market optimism) will cause stock prices to rise. In contrast, negative fluctuations in investment sentiment (market pessimism) will cause stock prices to fall, which is consistent with the findings of Ni et al. (Citation2015) and Molchanov and Stangl (Citation2018). An increasing long-run influence of investor sentiment outweighs the decreasing influence, which means that high investment sentiments have a greater impact on stock prices. This finding is consistent with several studies; the impact of investor optimism on stock returns is more pronounced than the impact of pessimism (Ding et al., Citation2004; Zhang & Semmler, Citation2009). Besides, the influences of VSI on sectoral stock returns are also significant, and the impact of interest rate is partially significant. Specifically, the influence of decreasing interest rates is more pronounced on sectoral stock returns. This finding is similar to the view of Huang et al. (Citation2016) that a lower interest rate could bloom stock price. In addition, the long-run influence of an increase in VSI beats the declining influence in most sectors, indicating that a higher VSI would cause more damage to the stock market (Qadan et al., Citation2019). In addition, model diagnostic tests are given at the bottom of . The results of the CUSUM test suggest that all model estimations are stable and the results of the heteroscedasticity test show that there is no heteroscedasticity in all models, except for Consumer Staples and Telecom. Serv. sectors.
The discussion above evidence that the direct impact of COVID-19 on sectoral stock market returns varies among industries, and the interpretation of the direct influence of COVID-19 is not significant for most industry stock prices. Next, we further pay attention to the impacts of media hype on sector stock markets. The estimated results are highlighted in , and coefficients of the media hype index are significant in most sectors, which are different from the result in . We can preliminarily conclude that COVID-19 mainly affects stock returns through media hype rather than pandemics directly affecting stock prices. Specifically, the coefficients of media hype on stock returns are negative in most industries, except for the Health Care sector. These significant negative effects of media hype on sectoral markets mean that media coverage may cause panic among most industries by spreading news related to COVID-19, thereby influencing their investment decisions and ultimately decreasing in stock returns. Furthermore, the long-term impact of increased media hype outweighs the impact of a decrease in Energy, IT, Utilities, and Real estate, indicating that continued increases in media hype will cause stock returns to fall more than the benefits brought by decreased media hype in these sectors. That is, increasing media hype will send a stronger signal that the individual’s safety and health have been badly compromised, and there exist severe uncertainties in the economy. Confronted with this predicament, most industries were compelled to halt output, and investors’ panic selling caused herding behaviour, compounding the decrease in stock returns. Besides, the long-term impact of a decline in media hype beats the impact of an increase in Consumer Discretionary, Financials, Materials, Industrials, Consumer Staples, and Telecom. Serv., suggesting that reducing media hype will increase stock returns more than the losses caused by the decline in media hype for these industries.
On the opposite, the coefficients of media hype on stock returns are positive in the Health Care sector. In addition, media hype has a substantial asymmetric long-run influence on stock returns in this sector. We also note that the long-term impact of increased media hype beats the impact of the decrease, suggesting that stock returns in the Health Care sector are more vulnerable to increased media hype. One reason for this is that media hype led investors to expect that the contagion of COVID-19 would trigger an increase in demand for medical devices (Fuchs et al., Citation2020). The demand for medical materials and R&D spending will increase in the Health Care Industry, generating positive investor sentiment in these sectors and increasing stock prices. Besides, the long-term coefficients of investor sentiment are still significantly positive in each scenario. The influence of interest rate is partially significant, and the impact of VSI is also significant, which is consistent with the findings in . Model diagnostic tests given at the bottom of indicate the absence of heteroscedasticity and autocorrelation in the error terms and the in all the models. Furthermore, the stability tests of CUSUM suggest that the estimates have been steady.
A summary of NARDL estimations is registered in to understand better the difference between the asymmetric influence of COVID-19 and the media hype on the Chinese sectoral stock markets. According to the result summary in , we can conclude that when considering the role of the media hype during the COVID-19 crisis, the hidden linkage between the stock market and COVID-19 can be better revealed.
Table 6. Results summary.
5.2. Dynamic asymmetric causality analysis
Asymmetric causality relationships between COVID-19 and each sector are shown in . According to the results of negative and positive shocks of COVID-19, we find that dynamic asymmetric causality does not show continuity. To reveal the results more precisely, shows the number of sub-sample periods with a causality link in both positive and negative shocks.
Figure 2. Time-varying causality analysis in positive shocks of COVID-19.
Source: Authors' calculation.
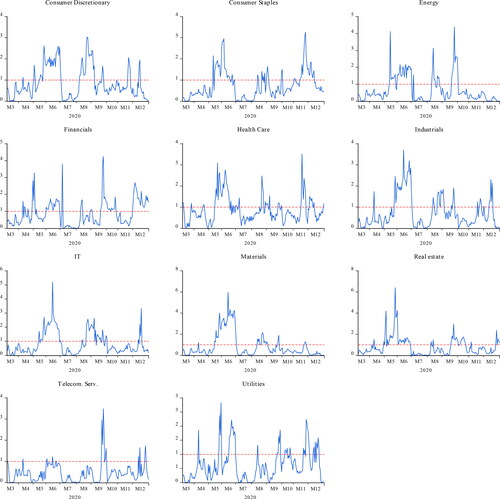
Figure 3. Time-varying causality analysis in negative shocks of COVID-19.
Source: Authors' calculation.
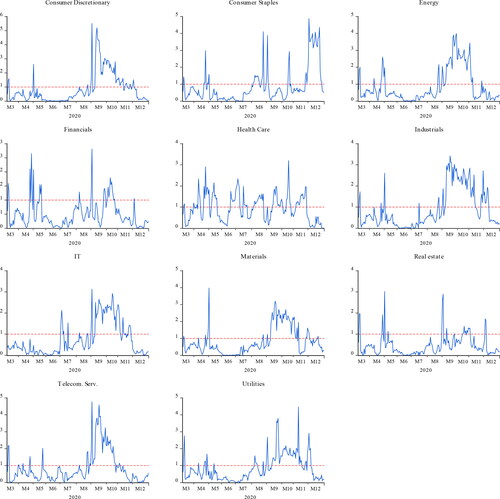
Figure 4. Summary of asymmetric causality test results considering the impact of COVID-19.
Source: Authors' calculation.
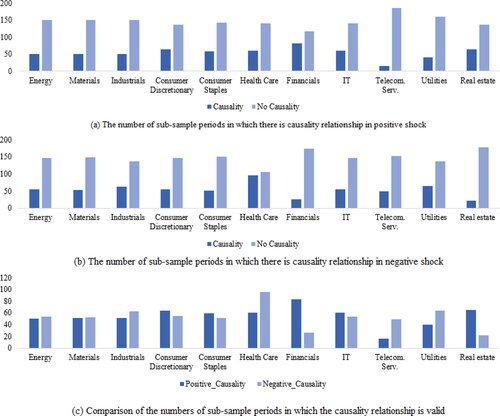
Panel (a) of shows the number of sub-sample periods with a causality linkage in positive shock of COVID-19. We notice that periods with no causality link are significantly more than the periods with a causality. Moreover, Telecom. Serv. sector has the fewest causation linkages and the Financial sector witnessed the most causality links. According to Panel (b), this is also valid for negative shocks, with the Real estate sector having the fewest causality ties and the Health Care sector having the greatest amount of causality linkages. Panel (c) represents the asymmetric features of the influence of COVID-19 on the sector market. In general, there exists no significant asymmetric relationship in most sectors. However, for the Financials and Health Care sectors, the results suggest evidence of the asymmetric influence of COVID-19 on the stock market during the sample period. These findings are consistent with the NARDL estimation.
and represent the findings of time-varying asymmetric causality from media hype to the sectoral stock market, considering positive and negative shocks of media hype. We also find that dynamic asymmetric causality does not show continuity. provides a more complete examination of each industry’s negative and positive shocks. Panel (a) and Panel (b) of depicts the number of sub-sample periods with substantial causation in both positive and negative shocks of media hype. Different from the direct impact of COVID-19, Panel (c) indicates that media hypes have significant asymmetric impacts on sectoral stock markets. The numbers of considerable causality from negative shocks defeat the numbers of significant causality from positive shocks, suggesting that stock returns in each sector are more vulnerable to negative fluctuations in the media hype. Therefore, the authorities should introduce measures to manage media reports to prevent excessive media hype and allow the market to form reasonable expectations, which is essential to the healthy development of the stock market.
Figure 5. Time-varying causality analysis in positive shocks of media hype.
Source: Authors' calculation.
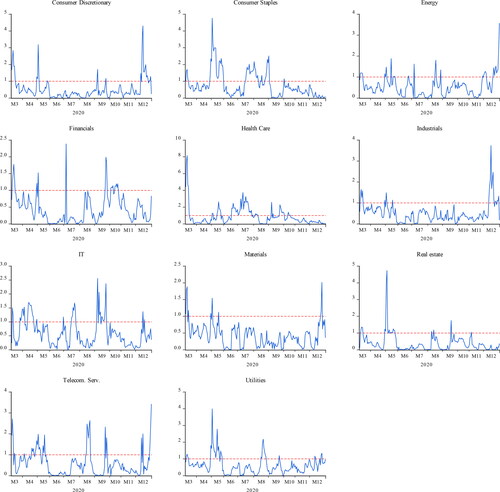
Figure 6. Time-varying causality analysis in negative shocks of media hype.
Source: Authors' calculation.
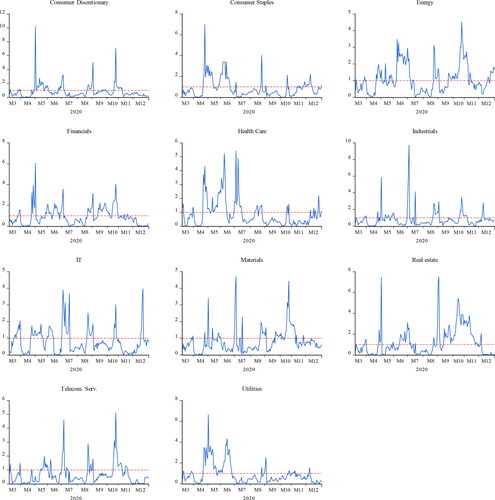
Figure 7. Summary of asymmetric causality test results considering the impact of media hype.
Source: Authors' calculation.
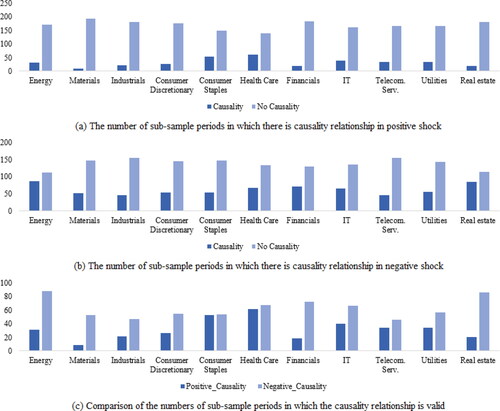
Through the above empirical analysis, this study discovers strong unequal impacts of the several concerned determinants on sectoral market returns in the context of China. We reveal that media hype pronouncedly affects sectoral market returns asymmetrically while the direct influence of COVID-19 on sectoral market returns is weak. These findings provide fresh light on the literature, which ignores the significance of media hype and the nonlinear impact when investigating the stock market’s reaction to COVID-19.
6. Conclusion
This study investigates the impact of media hype about COVID-19 on industry stock returns in the context of China. With the help of the NARDL model, the empirical outcomes show that the impacts of the media hype index are significant on stock returns in most sectors. This finding indicates that the information derived from the hype around COVID-19 might give helpful information to help policymakers and market investors predict the stock price trends. Furthermore, the empirical outcomes also reveal that media hype has a significant uneven influence on Chinese sectoral stock returns, implying that the prior literature’s linear model analyses are insufficient to capture the real reaction of stock returns to COVID-19.
These discoveries have several significant implications. Firstly, as media hype significantly affects stock price movements, while the direct influence of the COVID-19 pandemic index is weaker, policymakers need to pay close attention to the frequency of media releases of COVID-19 news and avoid excessive hype to stabilise public opinion and public sentiment. Appropriate dissemination of COVID-19 pandemic news helps investors make investment decisions based on objective information and actual risks. Secondly, these outcomes have significant implications for developing strategies for investors with different investment horizons. According to the sectoral stock returns showing time-varying heterogeneous reactions to the negative and positive changes of media hype, investors can benefit from various changes in media reports. Depending on changing market conditions, participants in the Chinese stock market should be aware of different industries’ characteristics and promptly diversify their portfolios to reduce industry risks. On the other hand, as the influence of media hype on stock market prices varies not only with negative and positive shocks but also over time, policy enforcers should take this impact into account by making new decisions and formulating strategies over time. Finally, each factor’s asymmetric influence should not be overlooked. As positive changes in media hype and negative changes in interest rates show a more significant influence on sectoral market returns, regulators should pay close attention to increased media coverage and declining interest rates to avoid excessive stock market volatility.
Acknowledgements
We would like to thank the Editor and three anonymous reviewers for their time and efforts in our research. Thanks to the reviewer’s contributions, the quality of this paper has been enhanced. Any remaining errors are our own.
Disclosure statement
No potential conflict of interest was provided by the authors.
Additional information
Funding
Notes
2 The composited COVID-19 pandemic index is calculated as the second principal component of Daily new confirmed cases, Daily suspected cases, Daily new severe cases, daily new deaths, and Daily new cured cases, which are taken from the Wind Info Economic Database of China.
3 The unit test results show that the series is I (1). The results are available upon request to authors.
References
- Al-Awadhi, A., Alsaifi, K., Al-Awadhi, A., & Alhammadi, S. (2020). Death and contagious infectious diseases: Impact of the COVID-19 virus on stock market returns. Journal of Behavioral and Experimental Finance, 27, 100326.
- Andrei, D., & Hasler, M. (2015). Investor attention and stock market volatility. Review of Financial Studies, 28(1), 33–72. https://doi.org/10.1093/rfs/hhu059
- Ashraf, B. (2020). Stock markets’ reaction to COVID-19: Cases or fatalities? Research in International Business and Finance, 54, 101249. https://doi.org/10.1016/j.ribaf.2020.101249
- Banerjee, A., Dolado, J., & Mestre, R. (1998). Error-correction mechanism tests for cointegration in a single-equation framework. Journal of Time Series Analysis, 19(3), 267–283. https://doi.org/10.1111/1467-9892.00091
- Cepoi, C. (2020). Asymmetric dependence between stock market returns and news during COVID-19 financial turmoil. Finance Research Letters, 36, 101658. https://doi.org/10.1016/j.frl.2020.101658
- Chen, M., Lee, C., Lin, Y., & Chen, W. (2018). Did the SARS epidemic weaken the integration of Asian stock markets? Evidence from smooth time-varying cointegration analysis. Economic Research-Ekonomska Istraživanja, 31(1), 908–926. https://doi.org/10.1080/1331677X.2018.1456354
- Ding, D., Charoenwong, C., & Seetoh, R. (2004). Prospect theory, analyst forecasts, and stock returns. Journal of Multinational Financial Management, 14(4–5), 425–442. https://doi.org/10.1016/j.mulfin.2004.03.005
- Donadelli, M., Kizys, R., & Riedel, M. (2017). Dangerous infectious diseases: Bad news for Main Street, good news for Wall Street? Journal of Financial Markets, 35, 84–103. https://doi.org/10.1016/j.finmar.2016.12.003
- Fuchs, A., Kaplan, L. C., Kis-Katos, K., Schmidt, S., Turbanisch, F., & Wang, F. (2020). Mask wars: China’s exports of medical goods in times of COVID-19. http://dx.doi.org/10.2139/ssrn.3661798.
- Gu, G., Zhu, W., & Wang, W. (2021). Time-varying influence of interest rates on stock returns: Evidence from China. Economic Research-Ekonomska Istraživanja, 34, 1–20. https://doi.org/10.1080/1331677X.2021.1966639
- Haroon, O., & Rizvi, S. (2020). COVID-19: Media coverage and financial markets behavior – A sectoral inquiry. Journal of Behavioral and Experimental Finance, 27, 100343.
- Harvey, D. I., & Mills, T. C. (2002). Unit roots and double smooth transitions. Journal of Applied Statistics, 29(5), 675–683. https://doi.org/10.1080/02664760120098739
- He, P., Sun, Y., Zhang, Y., & Li, T. (2020). COVID-19’s impact on stock prices across different sectors – An event study based on the Chinese stock market. Emerging Markets Finance and Trade, 56(10), 2198–2212. https://doi.org/10.1080/1540496X.2020.1785865
- Huang, W., Mollick, A., & Nguyen, K. (2016). U.S. stock markets and the role of real interest rates. The Quarterly Review of Economics and Finance, 59, 231–242. https://doi.org/10.1016/j.qref.2015.07.006
- Ichev, R., & Marinč, M. (2018). Stock prices and geographic proximity of information: Evidence from the Ebola outbreak. International Review of Financial Analysis, 56, 153–166. https://doi.org/10.1016/j.irfa.2017.12.004
- IEA Global Energy Review. (2020). The impacts of the COVID-19 crisis on global energy demand and CO2 emissions.
- Lee, J., & Strazicich, M. (2003). Minimum lagrange multiplier unit root test with two structural breaks. Review of Economics and Statistics, 85(4), 1082–1089. https://doi.org/10.1162/003465303772815961
- Molchanov, A., & Stangl, J. (2018). Investor sentiment and industry returns. International Journal of Finance & Economics, 23(4), 546–570. https://doi.org/10.1002/ijfe.1637
- Nepp, A., Okhrin, O., Egorova, J., Dzhuraeva, A., & Zykov, A. (2022). What threatens stock markets more – The coronavirus or the hype around it? International Review of Economics & Finance, 78, 519–539. https://doi.org/10.1016/j.iref.2021.12.007
- Ni, Z., Wang, D., & Xue, W. (2015). Investor sentiment and its nonlinear effect on stock returns – New evidence from the Chinese stock market based on panel quantile regression model. Economic Modelling, 50, 266–274. https://doi.org/10.1016/j.econmod.2015.07.007
- Nippani, S., & Washer, K. (2004). SARS: A non-event for affected countries’ stock markets? Applied Financial Economics, 14(15), 1105–1110. https://doi.org/10.1080/0960310042000310579
- Pesaran, M., Shin, Y., & Smith, R. (2001). Bounds testing approaches to the analysis of level relationships. Journal of Applied Econometrics, 16(3), 289–326. https://doi.org/10.1002/jae.616
- Phan, D., & Narayan, P. (2020). Country responses and the reaction of the stock market to COVID-19 – a preliminary exposition. Emerging Markets Finance and Trade, 56(10), 2138–2150. https://doi.org/10.1080/1540496X.2020.1784719
- Qadan, M., Kliger, D., & Chen, N. (2019). Idiosyncratic volatility, the VIX and stock returns. The North American Journal of Economics and Finance, 47, 431–441. https://doi.org/10.1016/j.najef.2018.06.003
- Shin, Y., Yu, B., & Greenwood-Nimmo, M. (2014). Modelling asymmetric cointegration and dynamic multipliers in a nonlinear ARDL framework. In R. Sickles, & W. Horrace (eds.), Festschrift in Honor of Peter Schmidt (pp. 281–314). New York, NY: Springer. https://doi.org/10.1007/978-1-4899-8008-3_9
- Su, C. W., Khan, K., Umar, M., & Zhang, W. K. (2021). Does renewable energy redefine geopolitical risks? Energy Policy, 158, 112566. https://doi.org/10.1016/j.enpol.2021.112566
- Su, C. W., Meng, X. L., Tao, R., & Umar, M. (2022). Policy turmoil in China: A barrier for FDI flows? International Journal of Emerging Markets, 17(7), 1617–1634. https://doi.org/10.1108/IJOEM-03-2021-0314
- Su, C. W., Pang, L. D., Umar, M., & Lobonţ, O. R. (2022). Will gold always shine amid world uncertainty? Emerging Markets Finance and Trade, 58, 1–2694. https://doi.org/10.1080/1540496X.2022.2050462
- Su, C. W., Pang, L. D., Umar, M., Lobonţ, O. R., & Moldovan, N. C. (2022). Does gold’s hedging uncertainty aura fade away? Resources Policy, 77, 102726. https://doi.org/10.1016/j.resourpol.2022.102726
- Su, C. W., Yuan, X., Umar, M., & Lobonţ, O. R. (2022). Does technological innovation bring destruction or creation to the labor market? Technology in Society, 68, 101905. https://doi.org/10.1016/j.techsoc.2022.101905
- Tao, R., Su, C. W., Naqvi, B., & Rizvi, S. (2022). Can Fintech development pave the way for a transition towards low-carbon economy: A global perspective. Technological Forecasting and Social Change, 174, 121278. https://doi.org/10.1016/j.techfore.2021.121278
- Topcu, M., & Gulal, S. (2020). The impact of COVID-19 on emerging stock markets. Finance Research Letters, 36, 101691.
- Valera, H., Holmes, M., & Hassan, G. (2017). Stock market uncertainty and interest rate behaviour: A panel GARCH approach. Applied Economics Letters, 24(11), 732–735. https://doi.org/10.1080/13504851.2016.1223817
- Youssef, M., Mokni, K., & Ajmi, A. (2021). Dynamic connectedness between stock markets in the presence of the COVID-19 pandemic: Does economic policy uncertainty matter? Financial Innovation, 7(1), 13. https://doi.org/10.1186/s40854-021-00227-3
- Zaremba, A., Kizys, R., Aharon, D., & Demir, E. (2020). Infected markets: Novel coronavirus, government interventions, and stock return volatility around the globe. Finance Research Letters, 35, 101597.
- Zhang, D., Hu, M., & Ji, Q. (2020). Financial markets under the global pandemic of COVID-19. Finance Research Letters, 36, 101528.
- Zhang, W., & Semmler, W. (2009). Prospect theory for stock markets: Empirical evidence with time-series data. Journal of Economic Behavior & Organization, 72(3), 835–849. https://doi.org/10.1016/j.jebo.2009.08.003